A comprehensive guide to Remote Patient Monitoring (RPM) system
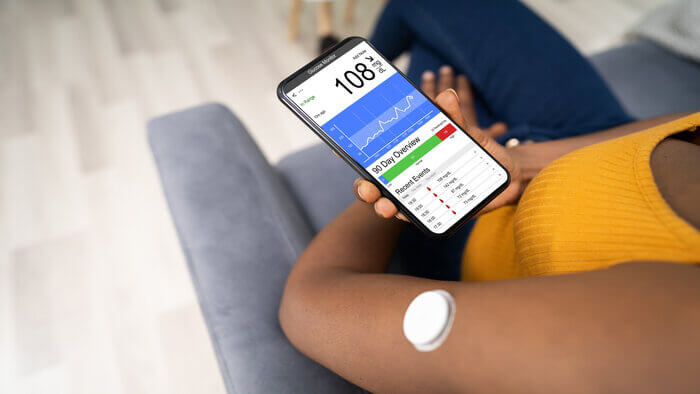
The landscape of healthcare delivery is evolving rapidly, with technological advancements paving the way for innovative approaches to patient care. Among these advancements, Remote Patient Monitoring (RPM) systems have emerged as a transformative solution, transforming the way healthcare is delivered and managed. RPM systems leverage advanced technology to remotely monitor patients’ health status, allowing healthcare providers to track vital signs, symptoms, and other health metrics from a distance. This real-time monitoring capability enables timely interventions, enhances patient outcomes, and reduces the need for frequent in-person visits, particularly for individuals with chronic conditions or those requiring continuous monitoring.
At the core of every RPM system lies a sophisticated infrastructure comprising wearable devices, sensors, mobile applications, and cloud-based platforms. These components work in tandem to collect, transmit, and analyze patient data, providing healthcare providers with valuable insights into patients’ health status and trends. By continuously monitoring key health indicators such as blood pressure, heart rate, glucose levels, and activity levels, RPM systems empower healthcare providers to detect early warning signs of deterioration or exacerbation of chronic conditions, allowing for timely interventions and preventive measures.
This article provides an overview of Remote Patient Monitoring (RPM) and its current landscape in healthcare. It explores the transformative impact of artificial intelligence (AI) in RPM, discussing its applications and advanced techniques. Additionally, the article addresses challenges and considerations for AI implementation in RPM and offers insights into the future of data-driven healthcare within this context.
- What is Remote Patient Monitoring (RPM)?
- The current landscape of remote patient monitoring
- How does remote patient monitoring work?
- The impact of AI in RPM: Transforming care for patients, providers, and the healthcare system
- Key applications of AI in remote patient monitoring
- Advanced AI techniques transforming remote patient monitoring
- Building an AI-powered RPM software: A step-by-step guide
- How LeewayHertz can help in building a custom remote patient monitoring system?
- Challenges and considerations for AI in RPM: Advancing responsibly
- The future of AI in RPM: A glimpse into a data-driven healthcare landscape
What is Remote Patient Monitoring (RPM)?
Remote Patient Monitoring (RPM) is an advanced approach in healthcare that leverages technological advancements to oversee patients’ well-being from a distance, without requiring them to be physically present in a medical facility. Through RPM, patients’ vital signs, symptoms, and other health-related data are continuously gathered using various devices like wearable sensors, mobile applications, and other interconnected gadgets. This collected information is then transmitted securely to healthcare providers, allowing them to monitor patients’ conditions in real-time. RPM enables healthcare professionals to track patients’ health metrics, detect any deviations from the norm, and intervene promptly if necessary, thereby facilitating proactive healthcare management. By providing continuous monitoring and timely interventions, RPM not only enhances patient care but also contributes to reducing hospitalizations, lowering healthcare costs, and improving overall health outcomes.
Types of remote patient monitoring devices
Remote patient monitoring relies on a variety of devices to collect and transmit patient data for continuous monitoring outside of traditional healthcare settings. These devices are designed to monitor various aspects of a patient’s health and can range from simple wearable sensors to sophisticated medical devices. Here are some common types of remote patient monitoring devices:
- Wearable sensors: Wearable sensors are small, lightweight devices that patients can wear on their bodies to monitor vital signs and activity levels continuously. These sensors can track parameters such as heart rate, blood pressure, respiratory rate, oxygen saturation, and physical activity. Wearable devices may include wristbands, patches, chest straps, and smart clothing embedded with sensors. They provide real-time data transmission to healthcare providers, enabling remote monitoring of patients’ health status and activity levels.
- Remote monitoring kits: Remote monitoring kits consist of a set of medical devices and peripherals that patients use to measure and monitor specific health parameters at home. These kits typically include devices such as blood glucose monitors, blood pressure monitors, pulse oximeters, thermometers, and weight scales. Patients can perform regular measurements and transmit the data to healthcare providers using integrated communication technologies, such as Bluetooth or cellular connectivity.
- Implantable devices: Implantable devices are medical devices that are surgically implanted inside the patient’s body to monitor and manage chronic conditions or specific health conditions. Examples of implantable devices used in remote patient monitoring include cardiac implantable electronic devices (e.g., pacemakers, implantable cardioverter-defibrillators), implantable loop recorders, and neurostimulators. These devices continuously monitor physiological parameters and detect abnormal heart rhythms, cardiac events, or neurological conditions, transmitting data to healthcare providers wirelessly.
- Smart home devices: Smart home devices are connected devices integrated into the patient’s home environment to monitor their health and well-being. These devices include smart scales, smart blood pressure monitors, smart pill dispensers, and smart environmental sensors. Smart home devices collect data on patient activity, medication adherence, environmental factors (e.g., temperature, humidity), and home safety, providing valuable insights into the patient’s daily life and health status.
- Mobile health applications: Mobile health (mHealth) applications are smartphone or tablet-based applications that enable patients to monitor their health, track symptoms, and communicate with healthcare providers remotely. These applications may include features such as symptom tracking, medication reminders, telemedicine consultations, and secure messaging. Patients can input data manually or connect wearable devices to the app for automated data collection. mHealth apps facilitate patient self-management and engagement while enabling healthcare providers to monitor patients’ health remotely.
- Smartwatches and fitness trackers: Advanced smartwatches and fitness trackers go beyond basic health monitoring to include ECG, stress measurement, and even blood glucose estimation through non-invasive sensors.
- Health monitors integrated with smartphones: Some smartphones now have built-in capabilities or attachable devices that can measure blood pressure, take ECG readings, and more, making remote patient monitoring more accessible.
- Digital stethoscopes: These devices can record and send heart and lung sounds to healthcare providers, allowing for remote auscultation, which is crucial for patients with cardiac or respiratory conditions.
- Smart beds and mattresses: Equipped with sensors, these beds can monitor sleep patterns, heart rate, and even detect movement or lack thereof, which can indicate a fall or other emergency.
- Video monitoring systems: Often used in elder care, these systems can detect falls, track movement, and allow for visual check-ins without invasive monitoring, providing a balance between patient privacy and care.
- Smart inhalers and spirometers: For patients with respiratory conditions, these devices track inhaler use, measure lung function, and can remind patients when it’s time to take their medication or perform breathing exercises.
- Connected contact lenses: These lenses are in development to monitor glucose levels in tears and may eventually provide real-time monitoring for diabetes management.
- Home dialysis machines: These allow patients with kidney conditions to perform dialysis at home, with devices that can transmit session data to healthcare providers.
- Digestible sensors: Pills with ingestible sensors can transmit data from within the body, monitoring medication adherence and reaction to drugs.
- Telehealth kiosks: Standalone kiosks can be placed in community centers and pharmacies, equipped with various devices to measure vital signs and facilitate virtual consultations.
- Smart patches: These patches are placed directly on the skin and can measure a wide range of health indicators, from heart rate and temperature to UV exposure, and even sweat analysis.
- Connected insulin pumps: These pumps manage insulin levels automatically and can communicate data to healthcare providers for diabetes management.
These types of remote patient monitoring devices empower patients to take an active role in managing their health and enable healthcare providers to deliver proactive, personalized care outside of traditional clinical settings. By leveraging these devices, remote patient monitoring systems enhance patient outcomes, improve clinical decision-making, and optimize healthcare delivery.
Importance of RPM in healthcare
Remote Patient Monitoring (RPM) is a pivotal component of modern healthcare systems, offering an array of benefits that significantly enhance patient care and overall healthcare delivery. By facilitating real-time monitoring of patients’ health status, RPM empowers healthcare providers to stay informed about their patients’ well-being, regardless of their physical location. This continuous oversight enables proactive management of chronic conditions, as healthcare professionals can promptly identify any deviations from normal health parameters and intervene before complications arise. Furthermore, RPM enables early detection of health issues, allowing for timely intervention and treatment, which can prevent exacerbation of illnesses and reduce the need for emergency care or hospitalizations.
For example, in a scenario where an elderly patient with heart failure utilizes RPM, healthcare providers can remotely monitor their vital signs and medication adherence. If the patient’s blood pressure begins to rise or they miss taking their prescribed medications, healthcare providers can intervene promptly to adjust their treatment plan or schedule a telemedicine consultation.
Moreover, RPM contributes to improved patient outcomes by fostering patient engagement and adherence to treatment plans through personalized monitoring and feedback. Patients benefit from the convenience of remote monitoring, as it eliminates the need for frequent in-person visits to healthcare facilities, thereby reducing inconvenience and the associated costs. Additionally, by facilitating remote consultations and interventions, RPM promotes patient-centered care and empowers individuals to actively participate in managing their health.
Overall, the integration of RPM into healthcare systems leads to better patient outcomes, reduced healthcare costs, and enhanced efficiency in healthcare delivery. It represents a paradigm shift in healthcare management, emphasizing proactive and personalized care that is tailored to the unique needs of each patient. As technology continues to advance, RPM holds the potential to redefine healthcare delivery by providing continuous, comprehensive monitoring and support to patients, ultimately improving their quality of life and well-being.
The current landscape of remote patient monitoring
Traditional methods of patient monitoring
Traditional methods of patient monitoring have long been the cornerstone of healthcare delivery, relying on periodic visits to healthcare facilities for assessments and interventions. During these visits, patients undergo physical examinations, which include the measurement of vital signs such as blood pressure, heart rate, and temperature. Diagnostic tests may also be conducted to assess specific health conditions or monitor disease progression. While these traditional methods provide valuable insights into patients’ health status during scheduled appointments, they are inherently limited in their ability to offer continuous monitoring and timely intervention.
One of the primary drawbacks of traditional patient monitoring is the lack of real-time data collection between visits. Patients’ health status may fluctuate unpredictably between appointments, and crucial changes in vital signs or symptoms may go undetected until the next scheduled visit. This delay in monitoring and intervention can result in missed opportunities for early detection of health issues and timely intervention, potentially leading to worsened outcomes or preventable complications.
Furthermore, traditional patient monitoring relies heavily on patients’ ability to accurately report their symptoms and adhere to treatment plans. However, factors such as forgetfulness, miscommunication, or reluctance to disclose certain symptoms may compromise the reliability of this information. Additionally, the inconvenience and logistical challenges associated with frequent visits to healthcare facilities may deter some patients from seeking timely care, particularly those with chronic conditions or mobility limitations.
In light of these limitations, there is a growing recognition of the need for innovative approaches to patient monitoring that can overcome the constraints of traditional methods. Emerging technologies such as remote patient monitoring (RPM) offer promising solutions by enabling continuous monitoring of patients’ health status outside of conventional healthcare settings. By leveraging wearable sensors, mobile health applications, and other connected devices, RPM systems can provide real-time data on patients’ vital signs, symptoms, and medication adherence, allowing healthcare providers to monitor their health status remotely and intervene promptly when necessary. This shift towards remote monitoring represents a paradigm shift in healthcare delivery, with the potential to improve patient outcomes, enhance access to care, and reduce healthcare costs.
Limitations and challenges faced by conventional RPM systems
Conventional Remote Patient Monitoring (RPM) systems, despite their advantages, encounter several limitations and challenges that impede their effectiveness in delivering comprehensive and seamless care to patients. One prominent challenge lies in the reliance on manual data entry by patients, which introduces opportunities for error and inconsistency. Patients may find the process of inputting data into RPM devices burdensome or may forget to do so regularly, resulting in incomplete or inaccurate data collection. Such inaccuracies can undermine the reliability of the monitoring system and compromise the quality of care provided.
Moreover, interoperability issues pose significant hurdles for conventional RPM systems. The lack of standardized protocols and compatibility among different devices often leads to fragmented data collection and siloed information, hindering seamless communication and data sharing between healthcare providers and across healthcare settings. This fragmentation not only complicates data management but also impedes the integration of RPM data with electronic health records (EHRs), limiting its utility for clinical decision-making and care coordination.
Additionally, conventional RPM systems may struggle to keep pace with the escalating demand for remote monitoring services. As the prevalence of chronic diseases rises and healthcare delivery shifts towards value-based care models, the volume of patient data generated by RPM systems continues to increase exponentially. Conventional systems may encounter scalability issues and resource constraints, hampering their ability to manage and analyze large volumes of data efficiently. Consequently, healthcare providers may face challenges in extracting actionable insights from RPM data and leveraging it to drive meaningful improvements in patient outcomes.
Addressing these limitations requires innovative approaches that prioritize automation, interoperability, and scalability in RPM systems. By harnessing advanced technologies such as artificial intelligence, machine learning, and interoperable standards, healthcare organizations can overcome these challenges and unlock the full potential of RPM to deliver personalized, proactive, and high-quality care to patients, wherever they may be.
The need for innovation in RPM
The evolving landscape of healthcare necessitates continuous innovation in remote patient monitoring (RPM) to address the growing complexities and challenges associated with managing chronic diseases and an aging population. Traditional and conventional RPM systems have demonstrated limitations in providing comprehensive, real-time monitoring of patients’ health status, highlighting the urgent need for innovative solutions.
Furthermore, advancements in AI and machine learning offer unprecedented opportunities to enhance RPM capabilities. AI algorithms can analyze vast amounts of patient data to identify patterns, predict health outcomes, and personalize interventions based on individual patient needs. Predictive analytics powered by AI enable early detection of health issues and proactive interventions, potentially preventing complications and improving patient outcomes.
By embracing innovation in RPM, healthcare providers can overcome the limitations of traditional monitoring methods and deliver more effective, patient-centered care. By leveraging cutting-edge technologies and data-driven insights, healthcare organizations can enhance overall healthcare delivery, improve patient outcomes, and ultimately, transform the way healthcare is delivered in the modern era.
How does remote patient monitoring work?
Remote patient monitoring works by utilizing various technologies to gather and transmit patient data from a distance, allowing healthcare providers to monitor and manage patients’ health remotely. Here’s a basic overview of how RPM typically functions:
- Data collection: Patients use wearable devices or home monitoring equipment to track vital signs, such as blood pressure, heart rate, blood glucose levels, and more. These devices continuously collect and record patient data.
- Data transmission: The collected data is transmitted securely to a centralized platform or cloud-based system using wireless or internet-connected technology. This allows real-time or periodic transmission of data from the patient’s location to the healthcare provider’s system.
- Data analysis: Healthcare providers use specialized software or platforms to analyze the transmitted data. This analysis may involve identifying trends, detecting anomalies, and assessing the patient’s overall health status based on the collected data.
- Alerts and notifications: The RPM system may be programmed to generate alerts or notifications for healthcare providers in case of abnormal or concerning trends in the patient’s data. This enables timely interventions and follow-up care.
- Patient engagement: RPM systems often include features to engage patients in their own care, such as educational resources, medication reminders, and communication tools for interacting with healthcare providers.
- Clinical decision support: RPM systems may also provide clinical decision support to healthcare providers by offering insights and recommendations based on the analyzed data. This helps healthcare providers make informed decisions about patient care and treatment plans.
Overall, remote patient monitoring enables healthcare providers to remotely monitor patients’ health status, detect changes or complications early, and intervene promptly to improve patient outcomes and quality of care.
The impact of AI in RPM: Transforming care for patients, providers, and the healthcare system
The integration of AI into RPM is guiding in a new era of healthcare delivery. The impact of AI in RPM extends far beyond technological innovation; it is fundamentally reshaping how patients experience care, how providers deliver it, and how the healthcare system as a whole operates. This section will delve into the diverse impact of AI in RPM, exploring the benefits it offers for patients, providers, and the healthcare system at large.
A. Empowering patients through improved outcomes and personalized care
For patients, AI-powered RPM offers a multitude of benefits that translate to improved health outcomes and a greater sense of empowerment in managing their own health.
- Early detection and intervention: AI’s ability to analyze substantial amounts of patient data allows for the early detection of potential health issues. This translates to earlier intervention, preventing complications and improving the overall effectiveness of treatment.
- Personalized care management: AI personalizes the RPM experience by tailoring it to individual patient needs and preferences. By analyzing data on medication adherence, activity levels, and health goals, AI can recommend personalized interventions and educational resources. This promotes patient engagement and empowers them to take an active role in managing their health.
- Enhanced convenience and reduced burden: Frequent in-person visits can be disruptive and time-consuming for patients. AI-powered RPM allows for continuous monitoring and data collection, reducing the need for frequent appointments. Additionally, AI can automate tasks like medication reminders and symptom tracking, further alleviating the burden on patients.
B. Streamlining workflows and empowering providers
Healthcare providers are facing an ever-increasing workload and pressure to deliver high-quality care. AI-powered RPM offers a powerful set of tools to streamline workflows and improve the way providers deliver care:
- Increased efficiency and productivity: AI automates tedious tasks like data analysis, generating reports, and flagging potential issues. This frees up valuable time for providers, allowing them to focus on more complex emphasis on patient care, such as diagnosis, treatment planning, and patient interaction.
- Proactive and personalized care delivery: AI provides real-time insights into patient health, enabling providers to deliver proactive and personalized care. By analyzing trends and patterns in patient data, AI can identify patients who might need immediate attention or adjustments to their treatment plans. This allows for a more proactive approach to care, potentially preventing complications and improving patient outcomes.
- Reduced workload and burnout: The burden of administrative tasks and data analysis can contribute to provider burnout. AI alleviates this burden by streamlining workflows and automating repetitive tasks. This allows providers to focus on what they do best – building rapport with patients and delivering high-quality care.
C. Transforming the healthcare system: Cost savings and improved population health
The impact of AI in RPM extends beyond individual patients and providers. It has the potential to transform the healthcare system as a whole by promoting cost-effectiveness and improving population health management:
- Reduced healthcare costs: Early detection and intervention through AI-powered RPM can significantly reduce healthcare costs. By preventing complications and hospital readmissions, AI helps to optimize resource allocation and eliminate unnecessary healthcare expenditures. For instance, AI can identify patients at high risk of readmission and provide targeted interventions like remote monitoring or medication adjustments, thereby reducing hospital readmission rates.
- Improved population health management: AI provides valuable data and insights into population health trends. By analyzing data from a large pool of RPM users, healthcare systems can identify areas of need and target resources more effectively. This data-driven approach allows for preventive measures to be implemented at a population level, leading to improved overall population health outcomes.
- Wider adoption of RPM programs: AI-powered RPM systems offer greater effectiveness compared to traditional RPM methods. This can incentivize wider adoption of RPM programs, leading to a larger population of patients benefiting from remote monitoring and personalized care. As AI-powered RPM proves its value, healthcare systems are likely to invest more heavily in these programs, ultimately improving access to care for a broader population.
The impact of AI in RPM is diverse and far-reaching. It empowers patients with greater control over their health, streamlines workflows for providers, and offers significant cost-saving advantages for the healthcare system. As AI technology continues to evolve and integrate seamlessly into RPM systems, we can expect even greater advancements in the way we monitor, manage, and deliver healthcare remotely. The future of healthcare holds immense promise, with AI poised to play a crucial role in shaping a more proactive, personalized, and cost-effective healthcare system for everyone.
Key applications of AI in remote patient monitoring
In advanced remote patient monitoring, artificial intelligence (AI) applications play a crucial role in transforming healthcare delivery. Some key AI applications in advanced RPM include:
Early disease detection and prediction
In the use case of early disease detection and prediction, AI algorithms play a pivotal role in analyzing comprehensive patient data encompassing vital signs, symptoms, and medical history. Through sophisticated data analysis techniques, AI can discern subtle patterns and anomalies indicative of the early stages of disease progression or potential health events. By leveraging this capability, AI can predict the onset of various conditions including diabetes, heart disease, and respiratory illnesses before they manifest clinically. This proactive approach empowers healthcare providers to intervene swiftly and initiate preventive measures, thereby mitigating the risk of complications and improving patient outcomes. By harnessing the insights gleaned from AI-driven analysis, healthcare teams can implement targeted interventions, personalize treatment plans, and optimize patient care strategies, ultimately leading to better health management and reduced healthcare burdens.
Personalized care management
AI empowers healthcare providers to customize RPM programs according to the unique needs and preferences of individual patients. Leveraging sophisticated algorithms, AI-powered systems meticulously monitor medication adherence, devise personalized treatment plans, and deliver tailored education and self-management support. This individualized approach not only fosters greater patient engagement but also enhances adherence to treatment regimens, ultimately culminating in improved health outcomes. By catering to the specific requirements of each patient, AI-driven personalized care management transforms the delivery of healthcare, optimizing patient satisfaction and contributing to overall wellness.
Proactive clinical decision support
The application of artificial intelligence in proactive clinical decision support represents a significant advancement in healthcare delivery. AI empowers healthcare providers with real-time insights and alerts, facilitating proactive clinical decision-making. Through sophisticated algorithms, AI-driven systems analyze patient data, including vital signs, laboratory results, and medical history, to stratify risks and identify patients at high risk of deterioration or adverse events. By leveraging this predictive capability, AI enables healthcare providers to prioritize interventions and allocate resources effectively. Timely alerts and recommendations provided by AI-driven systems enhance patient care delivery by enabling proactive interventions, thereby preventing complications and improving clinical outcomes.
Remote monitoring and telemedicine
AI-powered devices and platforms have significantly impacted healthcare delivery by enabling remote monitoring of patients’ health status and facilitating telemedicine consultations. Moreover, AI-driven telemedicine platforms enhance access to care, particularly for patients residing in remote or underserved areas who may face challenges accessing traditional healthcare services. By leveraging AI in remote monitoring and telemedicine, healthcare providers can extend their reach, improve patient outcomes, and enhance the overall efficiency and accessibility of healthcare delivery.
Data analytics and predictive modeling
The application of artificial intelligence in data analytics and predictive modeling represents a transformative advancement in healthcare. AI harnesses the power of machine learning and deep learning algorithms to analyze vast volumes of healthcare data, including Electronic Health Records (EHRs), medical imaging, and genomic data. By processing and interpreting this wealth of information, AI extracts actionable insights and makes predictions about patients’ health outcomes with remarkable accuracy.
Predictive modeling techniques employed by AI algorithms enable healthcare providers to identify patients at risk of complications, anticipate disease progression, and optimize treatment strategies accordingly. By leveraging historical patient data and identifying patterns, AI enables healthcare teams to implement targeted interventions and preventive measures, thereby mitigating risks and improving patient outcomes.
Furthermore, AI-driven predictive modeling facilitates personalized medicine by tailoring treatment plans to individual patient characteristics and needs. By predicting how patients may respond to different interventions, AI empowers healthcare providers to make informed decisions and optimize patient care pathways.
Natural Language Processing (NLP) and clinical documentation
AI plays a crucial role in natural language processing (NLP) and clinical documentation, transforming the way healthcare professionals manage patient information. AI-powered NLP algorithms are designed to extract valuable insights from unstructured clinical notes, enabling automated clinical documentation, coding, and analysis.
Traditionally, healthcare providers spend significant amount of time manually transcribing and organizing clinical notes from patient encounters. However, AI-powered NLP streamlines this process by automatically extracting relevant information from free-text notes, such as patient symptoms, diagnoses, and treatment plans. This automated documentation not only saves time but also improves accuracy and consistency in clinical records.
Moreover, AI-driven NLP enhances the efficiency of healthcare workflows by automating tasks such as clinical coding and billing. By accurately extracting diagnostic codes and other relevant information from clinical notes, NLP algorithms ensure compliance with coding standards and facilitate reimbursement processes.
Additionally, NLP facilitates clinical decision-making by providing actionable insights from free-text notes. By analyzing and summarizing large volumes of clinical data, NLP algorithms help healthcare providers identify trends, patterns, and critical information that may influence patient care decisions.
Overall, the application of AI in NLP and clinical documentation enhances the efficiency of healthcare workflows, improves documentation accuracy, and facilitates clinical decision-making in the context of remote patient monitoring. By automating tedious tasks and extracting valuable insights from clinical notes, AI-powered NLP contributes to better patient care and outcomes.
Overall, AI applications in advanced RPM hold immense potential to redefine healthcare delivery by enabling early detection, personalized care management, proactive clinical decision support, remote monitoring, data analytics, and clinical documentation. These AI-driven innovations have the potential to improve patient outcomes, enhance healthcare efficiency, and reduce healthcare costs.
Advanced AI techniques transforming remote patient monitoring
AI techniques play a crucial role in enhancing the capabilities of Remote Patient Monitoring (RPM) systems, enabling more accurate and personalized healthcare interventions. Here are some key AI techniques commonly used in RPM:
- Machine learning: Machine learning algorithms analyze patient data to identify patterns, trends, and correlations that may be indicative of health risks or changes in health status. Supervised learning techniques, such as classification and regression, are used to predict outcomes or classify patients into risk categories based on historical data. Unsupervised learning methods, such as clustering and anomaly detection, can uncover hidden patterns and outliers in patient data, facilitating early detection of health abnormalities.
- Deep learning: Deep learning models, particularly neural networks, are used to extract complex features from large-scale patient data, including medical images, biosignals, and textual data from electronic health records (EHR). Convolutional neural networks (CNNs) are employed for image analysis tasks, such as radiology interpretation and pathology detection, while recurrent neural networks (RNNs) and transformer models are used for sequential data analysis and natural language processing (NLP) tasks, such as patient notes and clinical documentation.
- Natural Language Processing (NLP): NLP techniques are applied to extract meaningful insights from unstructured textual data in patient records, clinical notes, and medical literature. NLP algorithms parse and analyze text to extract clinical concepts, identify medical entities (e.g., diseases, symptoms, medications), and analyze sentiment or tone. NLP-powered RPM systems can automate documentation, extract relevant information for decision support, and facilitate semantic interoperability across healthcare systems.
- Predictive analytics: Predictive modeling techniques leverage historical patient data to forecast future health outcomes, anticipate disease progression, and predict adverse events. Time-series forecasting models, such as autoregressive integrated moving average (ARIMA) and exponential smoothing methods, predict trends in patient vital signs and physiological parameters. Survival analysis techniques, such as Cox proportional hazards model and Kaplan-Meier estimator, estimate patient survival probabilities and time-to-event outcomes for chronic diseases.
- Reinforcement learning: Reinforcement learning algorithms learn optimal decision-making policies by interacting with the environment and receiving feedback on their actions. In RPM, reinforcement learning can be used to optimize treatment strategies, medication dosing regimens, and care interventions based on patient responses and outcomes. Reinforcement learning agents may adapt treatment plans over time to maximize patient outcomes while minimizing adverse effects and resource utilization.
- Bayesian inference: Bayesian statistical methods are employed to model uncertainty and incorporate prior knowledge into the analysis of patient data. Bayesian networks and probabilistic graphical models represent causal relationships between clinical variables and enable probabilistic reasoning about patient health states and treatment effects. Bayesian inference facilitates personalized risk assessment, decision support, and treatment planning by quantifying uncertainty and integrating evidence from multiple sources.
- Federated learning: Federated learning frameworks enable collaborative model training across distributed data sources, such as multiple healthcare institutions or patient devices, while preserving data privacy and security. Federated learning algorithms aggregate locally trained models or model updates from participating entities without sharing sensitive patient data. Federated learning empowers RPM systems to leverage diverse patient populations, improve model generalization, and address data heterogeneity and privacy concerns.
By leveraging these AI techniques, Remote Patient Monitoring systems can enhance clinical decision-making, facilitate early intervention, and improve patient outcomes while ensuring scalability, privacy, and regulatory compliance in healthcare settings.
Building an AI-powered RPM software: A step-by-step guide
Developing an AI-powered RPM system demands precise planning, technical proficiency, and a comprehensive grasp of healthcare and AI fundamentals. This roadmap explains the essential stages in constructing such a system:
- Define requirements: Begin by clearly defining the requirements for your AI-powered remote patient monitoring (RPM) software. This includes identifying the specific functionalities, features, and goals of the software.
- Data collection and integration: Collect and integrate relevant patient data from various sources such as medical devices, electronic health records (EHR), wearables, and other health monitoring systems. Ensure that data collection methods comply with healthcare regulations and standards for patient privacy and security.
- Data preprocessing: Cleanse, preprocess, and normalize the collected data to ensure consistency and accuracy. This step may involve data cleaning, filtering, normalization, and transformation to prepare the data for analysis and model training.
- AI Model development: Develop AI models tailored to the requirements of remote patient monitoring. This may involve machine learning algorithms such as supervised learning for predictive analytics, unsupervised learning for clustering and anomaly detection, or reinforcement learning for optimizing treatment recommendations.
- Training and validation: Train the AI models using labeled data and validate their performance using separate datasets to ensure accuracy, reliability, and generalizability. Fine-tune the models as needed to optimize performance and address any issues identified during validation.
- Integration with RPM platform: Integrate the trained AI models into the RPM software platform, ensuring seamless interaction and compatibility with existing systems and workflows. Develop user interfaces and APIs to facilitate data input, model execution, and output visualization.
- Real-time monitoring and analysis: Implement real-time monitoring capabilities to continuously collect and analyze patient data, detect trends, anomalies, and potential health risks. Use AI models to provide predictive insights, early warning alerts, and personalized recommendations for patient care.
- Feedback loop and iteration: Establish a feedback loop to gather feedback from healthcare professionals, patients, and other stakeholders. Use this feedback to iteratively improve the AI models, software features, and user experience based on real-world usage and evolving requirements.
- Regulatory compliance: Ensure compliance with regulatory requirements and standards for healthcare software, data privacy, security, and medical device regulations. Conduct thorough testing, validation, and documentation to meet regulatory obligations and obtain necessary certifications.
- Deployment and maintenance: Deploy the AI-powered RPM software in healthcare settings, providing training and support to users as needed. Establish maintenance procedures to monitor system performance, address issues, and incorporate updates, enhancements, and new features over time.
Building an AI-powered RPM system is a complex undertaking. However, by following these steps and prioritizing user needs, data security, and continuous improvement, you can create a valuable tool that empowers patients, improves healthcare delivery, and contributes to a more data-driven healthcare future.
Additional considerations:
- Interoperability: Ensure your system can integrate with existing Electronic Health Records (EHR) systems used by healthcare providers.
- Scalability: Design your system to handle a growing user base and future expansion.
- Ethical considerations: Ensure your system adheres to ethical principles of fairness, transparency, and responsible data collection and use.
How LeewayHertz can help in building a custom remote patient monitoring system?
At LeewayHertz, we understand the unique challenges and requirements of implementing a custom remote patient monitoring system. With our extensive expertise in AI, we offer comprehensive support to healthcare organizations seeking to develop tailored RPM solutions that meet their specific needs and objectives.
- Strategic planning and consultation: Our team of experts begins by conducting in-depth consultations to understand your organization’s goals, requirements, and constraints. We work closely with stakeholders to define the scope of the custom RPM system and develop a strategic roadmap for its implementation.
- Custom solution design: Leveraging our deep domain knowledge and technical proficiency, we design custom RPM solutions tailored to your organization’s unique requirements. Whether you need a solution for monitoring chronic conditions, managing medication adherence, or tracking vital signs, we develop intuitive and user-friendly interfaces that prioritize patient engagement and healthcare provider usability.
- AI integration and optimization: As pioneers in AI-driven healthcare solutions, we integrate advanced AI technologies into your custom RPM system to enhance its capabilities. From AI-powered data analytics and predictive modeling to clinical decision support systems, we leverage AI to drive actionable insights and improve patient outcomes.
- Scalable and secure development: Our experienced development team follows industry best practices to build scalable and secure RPM systems that can accommodate the growing needs of your organization. We prioritize data security and compliance with healthcare regulations, ensuring that patient data remains protected and confidential at all times.
- Continuous support and maintenance: Our partnership doesn’t end with the deployment of the custom RPM system. We provide ongoing support and maintenance services to ensure the smooth operation of your solution. Whether it’s troubleshooting technical issues, implementing updates, or scaling the system as your organization grows, we’re here to support you every step of the way.
With LeewayHertz as your trusted technology partner, you can rest assured that your custom RPM system will be built to the highest standards of quality, reliability, and innovation.
Challenges and considerations for AI in RPM: Advancing responsibly
While AI presents immense potential for transforming RPM, its implementation is not without challenges. Addressing these challenges is pivotal for ensuring the responsible development and deployment of AI in RPM. This section will explore key areas of concern, including data security and privacy, algorithmic bias, regulatory hurdles, the human factor in clinical decision-making, and ensuring equitable access to AI-powered RPM.
1. Safeguarding patient data
RPM relies on collecting and analyzing a vast amount of sensitive patient information – physiological data, medication adherence records, and even personal health information. Data security breaches can have devastating consequences, eroding patient trust and potentially leading to identity theft or misuse of health data. Therefore, robust security measures are paramount. Healthcare providers and developers of AI-powered RPM systems must prioritize data encryption, secure storage practices, and strict access controls.
Furthermore, patient privacy is a critical concern. Clear and transparent data governance policies are essential, outlining how patient data is collected, used, and stored. Patients must have full control over their data, with the right to access, modify, or withdraw consent at any time. Building trust and transparency with patients regarding data handling practices is crucial for the widespread adoption of AI-powered RPM.
2. Combating algorithmic bias
AI algorithms are only as good as the data they are trained on. Unfortunately, biased data can lead to biased algorithms. This can have significant consequences in healthcare, potentially leading to inaccurate diagnoses, ineffective treatment recommendations, and disparities in access to care. Mitigating algorithmic bias requires careful consideration of training data sets, ensuring diversity and representation across various demographics and health conditions.
Additionally, transparency and explainability in AI models are crucial. Healthcare providers need to understand how AI arrives at its conclusions to ensure its validity and avoid relying solely on “black box” algorithms. Explainable AI techniques can help shed light on the reasoning behind AI-generated insights, fostering trust and allowing for human oversight.
3. Navigating the regulatory landscape
The regulatory landscape for AI-powered medical devices is still evolving. Healthcare providers and developers need to stay informed of changing regulations and ensure their AI-powered RPM systems comply with all relevant data privacy, security, and safety standards. Collaborating with regulatory bodies during the development and testing phases of AI-powered RPM systems can expedite the approval process and ensure adherence to established guidelines.
4. Human expertise alongside AI
AI is a powerful tool, but it should not replace human expertise in healthcare. Clinicians must remain at the forefront of decision-making, leveraging AI insights to augment their knowledge and inform their assessments. AI can help automate tasks, analyze vast amounts of data, and identify potential issues, but human judgment and experience are crucial for interpreting the data and making critical decisions regarding patient care.
A successful approach involves human-AI collaboration, where AI empowers clinicians, allowing them to focus on higher-level tasks like patient interaction, diagnosis, and treatment planning. The goal is to create a symbiotic relationship – AI serving as a powerful assistant, ultimately enhancing the capabilities of healthcare professionals.
The challenges associated with AI in RPM are real but not insurmountable. By prioritizing data security and privacy, mitigating algorithmic bias, navigating the regulatory landscape, and fostering human-AI collaboration, we can ensure the responsible development and deployment of AI-powered RPM. By addressing these challenges, we can explore the full potential of AI to create a future where RPM empowers patients, improves healthcare outcomes, and ultimately leads to a healthier population.
The future of AI in RPM: A glimpse into a data-driven healthcare landscape
The integration of AI into remote patient monitoring is rapidly reshaping healthcare delivery. As AI technology continues to evolve, exciting new trends are emerging, promising even more profound transformations in how we monitor and manage patient health remotely. This section will explore the potential of AI for mental health and paint a long-term vision for a data-driven healthcare system powered by AI-driven RPM.
1. The rise of conversational AI in RPM
The future of RPM is likely to see seamless integration with chatbots and virtual assistants powered by AI. These intelligent agents can provide patients with 24/7 access to information, answer basic questions about their health conditions, and offer personalized support based on their real-time data. Chatbots can also play a pivotal role in medication adherence by sending reminders, tracking progress, and escalating concerns to healthcare providers if necessary.
Furthermore, virtual assistants can conduct preliminary health assessments through chat interfaces or voice commands. This can improve early detection of potential issues and streamline communication between patients and providers. Imagine a patient experiencing chest pain at home. A virtual assistant can guide them through a series of questions and collect vital signs data remotely, potentially prompting an immediate call to emergency services.
2. Advanced analytics for complex diseases
As AI technology matures, we can expect to see the development of advanced analytics specifically tailored for complex diseases. These AI models will be able to analyze not only physiological data but also integrate genetic information, environmental factors, and social determinants of health. This comprehensive approach will allow for a more holistic understanding of individual patient risk factors and disease progression.
For instance, AI models could analyze a patient’s genetic profile along with their blood sugar levels and activity data to predict the risk of developing diabetes complications. This would allow for the implementation of highly personalized preventive measures before complications arise. Similarly, AI could analyze a patient’s brain scans, combined with data from wearable devices tracking sleep patterns and mood, to identify early signs of neurodegenerative diseases like Alzheimer’s.
3. AI and remote mental health monitoring
Mental health is an area ripe for the transformative potential of AI in RPM. Traditional mental health monitoring often relies on subjective patient reporting during infrequent in-person visits. AI can analyze data from wearable devices tracking sleep patterns, activity levels, and heart rate variability to identify potential indicators of depression, anxiety, or other mental health conditions. Additionally, AI-powered chatbots can be used for mental health screening, providing basic cognitive assessments and offering emotional support through carefully designed conversational interfaces.
The future holds the promise of AI-powered virtual therapists capable of conducting therapeutic conversations, offering cognitive behavioral therapy (CBT) techniques, and monitoring patient progress remotely. This could redefine access to mental healthcare, particularly in underserved communities or for patients with mobility limitations.
4. A data-driven future of healthcare
Looking towards the long term, we can envision a future healthcare system built upon a foundation of real-time patient data collected through RPM and analyzed by powerful AI engines. This data-driven approach will enable proactive, preventative, and truly personalized care. Healthcare providers will have access to a comprehensive view of each patient’s health, allowing for early intervention and targeted treatment plans.
Imagine a scenario where a patient’s AI-powered RPM system detects a gradual decline in their activity levels and sleep quality. The system can then alert healthcare providers, who can intervene early, potentially identifying undiagnosed depression or a developing chronic condition. This proactive approach has the potential to enhance overall population health and reduce healthcare costs significantly.
The future of AI in RPM is brimming with possibilities. As AI continues to develop, we can expect to see seamless integration with conversational AI, advanced analytics for complex diseases, and a revolution in remote mental health monitoring. Ultimately, these advancements pave the way for a data-driven healthcare system focused on prevention, personalization, and improved patient outcomes across the entire spectrum of healthcare.
Endnote
The integration of AI into remote patient monitoring has transformed healthcare delivery, enhancing patient outcomes and provider efficiency. By leveraging AI-powered remote patient monitoring systems, healthcare professionals can efficiently monitor patients’ vital signs from afar, swiftly detect any anomalies, and intervene promptly. This proactive approach significantly reduces potential health risks and enhances patient care. Moreover, AI-driven predictive analytics enable proactive care management, predicting potential health complications before they escalate, thus reducing hospitalizations and healthcare costs.
Furthermore, AI in RPM facilitates personalized healthcare by analyzing vast amounts of patient data to tailor treatment plans based on individual needs and historical trends. This personalized approach not only improves patient satisfaction but also fosters better treatment adherence and long-term health outcomes.
However, challenges such as data privacy concerns, interoperability issues, and the need for regulatory frameworks must be addressed to maximize the potential of AI in RPM fully. Despite these challenges, the future of healthcare undoubtedly lies in the synergy between AI and RPM, promising to transform patient care and empower healthcare providers with invaluable insights for informed decision-making. As technology continues to evolve, embracing AI in RPM holds the promise of a healthier, more connected future for patients and providers alike.
Empower patients, enhance care delivery, and reduce costs with custom RPM solutions. Connect with LeewayHertz experts to learn how we can design and develop a tailored RPM software system, incorporating AI, ML, and the latest in digital health technology to meet your specific needs.
Start a conversation by filling the form
All information will be kept confidential.
Insights
Healthcare Supply Chain Management
Healthcare supply chain management regulates the flow of medical supplies from manufacturer to patient.
AI in healthcare: Use cases, applications, benefits, solution, AI agents and implementation
AI-powered predictive analytics identifies high-risk patients and prevents disease before it becomes severe. It can transform drug development processes for timely delivery and care.
How to improve communication in healthcare with technology?
Technology is assisting every industry in moving towards high efficiency and healthcare industry is no exception to it. With the help of various technologies, healthcare communication can be immensely improved and made effective.