Hybrid AI: Components, applications, use cases and development
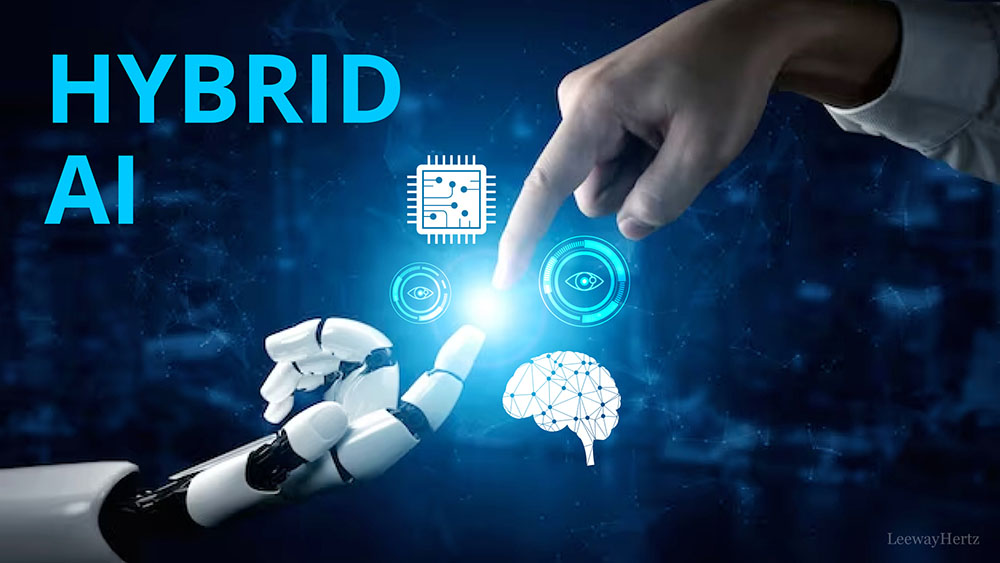
Hybrid AI, an innovative convergence of symbolic and non-symbolic AI, is emerging as a game-changing force in the realm of artificial intelligence. It combines the strengths of two distinct facets of AI, introducing a new wave of transformative solutions allowing real-time decision-making and enhanced creativity. This synergy creates user-friendly and authentic systems, promoting a seamless interaction between humans and their digital environments.
Symbolic AI, which uses logical rule-based systems to link facts and events, enables machine-readable and retrievable knowledge. This approach is vital in adding a layer of semantic understanding and enhancing decision-making processes. On the other hand, non-symbolic AI encompasses machine learning, deep learning, and neural networks, using vast amounts of training data to derive statistically informed conclusions and decisions.
However, the true power of AI lies not in these separate branches but in their integration – a hybrid architecture in AI, called hybrid AI. Hybrid AI represents a structured, comprehensive, and integrated application of both symbolic and non-symbolic AI. With the goal of making all data or knowledge within an organization machine-readable and understandable in natural language, hybrid AI effectively improves overall data comprehension, retrieval, and utilization.
From autonomous vehicles to retail industries, the applications of hybrid AI are diverse and far-reaching. The future of AI looks promising with the advent of hybrid AI, offering a flexible and responsive system set to transform how we interact with technology.
Join us as we delve into the intriguing world of hybrid AI architecture and explore its potential implications across industries, illuminating how this innovative approach is transforming the business landscape.
- What is hybrid AI?
- Why is hybrid AI important?
- Why is hybrid AI seen as a solution to the limitations of purely rule-based or learning-based AI?
- Understanding the components of hybrid AI
- Types of AI that form the hybrid AI ecosystem
- In what ways does the fusion of symbolic AI and neural networks prove beneficial?
- Applications of hybrid AI
- How to get started with hybrid AI?
- Is hybrid intelligence the future?
- Use cases of hybrid AI
- Importance of knowledge graphs in building hybrid AI systems
- How can LeewayHertz help you?
What is hybrid AI?
Hybrid AI is a strategic fusion of human intelligence and artificial intelligence systems designed to leverage the strengths of both entities. It ingeniously combines rule-based systems, known for their speed and reliability, with machine learning models renowned for their adaptability to changing scenarios. In circumstances where an AI system like a bot fails to comprehend certain aspects, such as ‘context,’ hybrid AI introduces human oversight. This human intervention ensures the bot proceeds effectively and responds satisfactorily to end users.
Google’s search function serves as a powerful instance of hybrid AI, melding the latest deep learning methods like Transformers with symbolic AI systems like tools for navigating knowledge graphs. Similarly, AlphaGo, a milestone accomplishment in AI in recent times, illustrates the fusion of symbolic AI and deep learning.
Hybrid AI’s unique composition allows it to deliver superior performance and accuracy, overcoming the limitations of using a single AI approach. This method finds extensive utility in various domains like customer service and natural language processing, where it skillfully handles common as well as complex queries. Other applications of hybrid AI include making informed predictions based on medical data analysis, improving the accuracy of targeted advertisements, and more. Essentially, hybrid AI serves as a robust framework that maximizes the advantages of human and artificial intelligence to deliver precise and contextually relevant outcomes.
Let’s illustrate the concept of hybrid AI model with a scenario. Visualize a machine learning system designed to detect faulty products. The system is taught to differentiate between defective and non-defective items by feeding it hundreds or thousands of samples. Once trained, the system can reliably and swiftly identify faulty products, often outperforming human accuracy. But, the question arises, what happens after a defective product is identified?
Here’s where additional data comes into play. We need answers to several questions to derive meaningful actions from the identification. For instance, which production line did the defective product come from? What is the financial implication of the defect? Who were the suppliers? Which process was responsible for the defect? What information needs to be shared with the managers to address the issue? This is the point where machine learning data needs to be put into context, and that’s where symbolic AI comes in. Symbolic AI helps comprehend and reason semantic knowledge, including establishing rules for extracting relevant insights.
The ultimate aim of AI is to emulate human brain functions as closely as possible. But the truth is, the human brain is far more complex and adaptable than any machine, with unparalleled versatility and interconnectivity. The intricacies of our brains are still unmatched by computers. However, hybrid AI blends the scalability of machine learning with the interpretive ability of symbolic AI. This blend helps mimic human brain functionality more closely and generates actionable insights from data.
Launch your project with LeewayHertz!
Embrace the future with hybrid AI. Contact our AI experts to transform your business with advanced hybrid AI solutions!
Why is hybrid AI important?
What challenges does AI currently face?
Contrary to expectations, the implementation of artificial intelligence has presented more challenges than solutions, as the MIT Technology Review reported. Although computers can learn new skills, the struggle lies in teaching them to apply this knowledge to unexpected situations.
AI has remained limited in its applications, primarily due to the difficulty in designing systems capable of adapting to new circumstances and executing both inductive and deductive reasoning effectively.
One fundamental issue is that AI systems are data-driven and lack the inherent knowledge and common-sense reasoning that humans apply naturally. Conventional or narrow AI fails to comprehend the broader world and establish contextual connections between words. As a result, these systems struggle to draw on information from various sources to solve problems or form new connections, an area where humans excel.
While humans are proficient at generalizing, most AI systems are tailored to address specific use cases. Therefore, AI’s reach remains constricted.
The significance of hybrid intelligence
The journey of artificial intelligence spans several decades, and the results of the early efforts are now beginning to surface. However, the reality remains that even with advanced technological progress, computers and software techniques have not reached the level of human intelligence.
This is where the concept of hybrid Intelligence comes into play. Hybrid Intelligence is about humans and machines operating in unison, each leveraging their strengths to create more impactful outcomes.
In this symbiotic relationship, machines provide computational power while humans infuse their creativity, knowledge, and experiential wisdom to guide decision-making processes.
The primary strength of hybrid Intelligence lies in its ability to process and apply knowledge to unfamiliar scenarios. This system can handle unstructured data, typically challenging for machines, and use this information for decision-making. The concept of the Internet of Things (IoT) serves as an apt illustration.
In an IoT framework, machines monitor and collect data from various sensors. However, understanding the significance of this data can be a daunting task for machines. Hybrid intelligence bridges this gap by leveraging human input to comprehend the relevance of this data, enabling informed decision-making. This collaboration between humans and artificial intelligence presents the next frontier in AI development, promising more robust, adaptable, and intelligent systems.
Why is hybrid AI seen as a solution to the limitations of purely rule-based or learning-based AI?
Hybrid AI represents a significant advance in the field of artificial intelligence by bridging two distinct approaches: rule-based and machine learning-based methods. This unique fusion enhances the capacity and performance of AI systems, overcoming the limitations of standalone rule-based or learning-based methods.
Rule-based AI, also referred to as symbolic AI, operates on predefined rules and logic. It’s particularly effective when dealing with clear-cut, well-structured problems where rules can be clearly defined. These systems are quick, reliable, and excellent at performing tasks with consistency and precision. However, rule-based AI’s strength can also be its weakness. It’s rigid and inflexible, struggling to adapt to new or changing conditions since it can only operate within its preprogrammed rules.
On the other hand, machine learning-based AI methods thrive on flexibility and adaptability. They leverage vast amounts of training data to learn, adapt, and make decisions based on statistical analysis. The more data a machine learning algorithm processes, the more it learns and the better it performs. However, machine learning-based AI systems require substantial data to train effectively, and their performance may vary depending on the data quality they are trained on.
Hybrid AI models emerge as an elegant solution to address the limitations of both approaches. By combining rule-based and machine learning methods, it capitalizes on the strengths of both domains. The rule-based component ensures speed and reliability, while the machine learning component offers flexibility and adaptability. Together, they create a more versatile AI system that can efficiently handle complex scenarios, adjust to changing conditions, and improve performance and accuracy.
This integrative approach is incredibly valuable in fields like customer service and language processing, where both rule-based and learning-based methods are beneficial. For instance, in customer service, rule-based methods can swiftly handle common queries, while machine learning-based approaches can learn from past interactions to handle more complex or unusual queries. Similarly, rule-based systems can handle grammar and syntax in language processing, while machine learning systems can understand context, sentiment, and slang.
Hybrid AI also finds application in medical data analysis, advertising, and many other fields where accurate predictions and adaptability are crucial. The fusion of rule-based and learning-based methods provides a comprehensive approach to AI, paving the way for more robust, reliable, and adaptable systems capable of meeting diverse needs.
Understanding the components of hybrid AI
Hybrid AI, as the name suggests, combines different AI techniques and methodologies. The goal is to create a more robust and effective AI system to tackle a wider range of tasks more efficiently. The main components of a hybrid AI system may vary depending on the specific goals of the system, but they often include:
1. Symbolic AI (Declarative AI): This branch of AI involves creating models and systems that can reason logically and follow explicitly defined rules. The system makes inferences based on a set of rules, which human experts often formulate. This branch includes:
- Expert systems: These are computer systems made to think like a human expert. They solve tough problems using a lot of knowledge, mostly in the form of if-then rules rather than the usual computer code. The “knowledge” is explicitly programmed by human experts.
- Business Rules Management Systems (BRMS): BRMS provide a way to manage, automate, and execute business rules. These systems enable companies to make decisions based on rules that can be changed without requiring changes to the system code.
2. Machine learning (Non-declarative AI): Machine learning algorithms develop a mathematical structure using a subset of data referred to as “training data.” This structure is then utilized to make forecasts or decisions, all without the necessity of being directly coded to execute the specific task. This branch includes:
- Decision tree learning: Decision trees are a model used for classification and regression. They model decisions with tree-like structures, where each internal node represents a “test” on an attribute, each branch represents the outcome of a test, and each leaf node represents a class label (a decision).
- Deep learning: A subclass of machine learning, deep learning architectures such as deep neural networks are based on artificial neural networks with multiple layers. These techniques have significantly advanced state-of-the-art areas such as speech recognition, visual object identification, and object detection, among several other fields.
- Neural networks: These are computing systems inspired by the biological neural networks that constitute animal brains. They are a variety of machine learning models which aim to mimic the human brain and are designed to recognize patterns.
- Bayesian networks: A Bayesian network, also known as a belief network, is a probabilistic graphical model representing variables and their conditional dependencies via a Directed Acyclic Graph (DAG). Bayesian networks are great at looking at something that happened and guessing which known reason might have caused it.
- Support vector machines: Support Vector Machines (SVMs) are a set of supervised learning methods used for classification, regression, and outliers detection. SVMs perform well in spaces with many dimensions and offer versatility as various Kernel functions can be chosen for decision-making.
Hybrid AI, therefore, uses a combination of the above methodologies to form a more comprehensive and efficient AI system. It benefits from each method’s strengths and mitigates individual systems’ weaknesses. A hybrid AI system can handle a broader array of tasks and is capable of self-learning, adaptation, and problem-solving at a high level of complexity.
Types of AI that form the hybrid AI ecosystem
Below are the types of AI that form part of the Hybrid Intelligence ecosystem:
- Adaptive AI: Adaptive AI refers to an artificial intelligence system that alters operations and responses based on observations and incoming data. Unlike traditional computing systems that strictly follow predefined instructions, adaptive AI has the capability to adjust its activity according to new information it receives. This type of AI doesn’t have all the solutions pre-programmed into it; instead, it evolves its operations and decision-making patterns to achieve objectives, essentially learning from its experiences.
- Learning AI: Learning AI is a branch of artificial intelligence that thrives on data to improve its performance. The distinctive feature of learning AI is that it doesn’t require explicit programming to enhance its capabilities or performance. Rather, it utilizes data it has been fed or has gathered to self-improve, refining its predictions or outputs over time.
- General AI: General AI, also known as Artificial General Intelligence (AGI), refers to a type of artificial intelligence that can perform any intellectual task that a human being can. It’s a theoretical concept at present, denoting a system that could replicate human intelligence aspects, including but not limited to natural language processing, knowledge representation, problem-solving, and planning.
- Narrow AI: Narrow AI, or weak AI, is a type of artificial intelligence specialized in performing a specific task or a set of tasks. Examples could include AI systems that play chess, drive cars, or recommend products online. These systems are designed to excel in their respective areas but lack the versatility of human intelligence.
- Parallel AI: Parallel AI denotes artificial intelligence systems that harness the power of multiple machines working in parallel. By distributing the computational load across multiple machines, these AI systems can process large datasets and complex algorithms more quickly and efficiently.
- Specialized AI: Specialized AI is designed to clearly focus on solving specific problems or tasks. These could range from diagnosing a medical condition based on symptoms and medical imaging, forecasting stock market trends, to interpreting natural languages. Specialized AI excels in its domain but may not perform well outside it.
- Symbiotic AI: Symbiotic AI is a concept where different AI systems collaborate and share knowledge with each other. By working together, these AI systems can achieve more complex tasks or understand broader contexts than they individually can.
- Virtual AI: Virtual AI exists solely within digital environments, such as computer systems or smartphones. Examples of virtual AI include chatbots, virtual assistants like Siri or Alexa, and AI characters in video games. These AI systems interact with users, process data, and perform tasks within their virtual environments.
Launch your project with LeewayHertz!
Embrace the future with hybrid AI. Contact our AI experts to transform your business with advanced hybrid AI solutions!
In what ways does the fusion of symbolic AI and neural networks prove beneficial?
The combination of symbolic AI and neural networks, often called neuro-symbolic AI or hybrid AI, has gained interest in recent years due to its potential to combine the best aspects of both AI approaches. Here’s why it can be beneficial to combine them:
- Comprehensibility: Using explicit rules and logic, Symbolic AI is highly interpretable. This means that it can provide clear and understandable reasoning for its decisions. On the other hand, neural networks, particularly deep learning models, are often described as “black boxes” because their decision-making process can be difficult to understand. By combining the two, we can potentially create AI systems with both the power of neural networks and the interpretability of symbolic AI.
- Learning efficiency: Symbolic AI systems can provide a form of “prior knowledge” or structure that can help guide the learning process of a neural network. This can make the learning process more efficient, potentially requiring less data or fewer iterations.
- Robustness and generalization: Symbolic AI, using explicit rules, can ensure certain invariants or constraints are always met, leading to more robust AI systems. It can also help neural networks to better generalize from the training data to new, unseen data.
- Common sense reasoning: One of the challenges of current neural networks is their lack of “common sense” or their inability to make logical inferences that are obvious to humans. With its roots in formal logic, Symbolic AI is well-suited to this kind of reasoning.
- Integration of knowledge: Symbolic AI can integrate human knowledge in the form of rules or facts into the AI system. This can be useful when data is scarce, or we want to ensure the AI aligns with human expertise or values.
- Data efficiency: While neural networks often require large amounts of data to learn effectively, symbolic AI can learn from smaller amounts of data, making them more data-efficient.
In summary, the combination of symbolic AI and neural networks can lead to AI systems that are more understandable, efficient, robust, capable of common sense reasoning, able to integrate human knowledge and more data-efficient. However, integrating these two very different AI approaches is a challenging and active area of research.
Applications of hybrid AI
Customer experience, service and support
Hybrid AI, which combines different AI techniques like symbolic AI and machine learning, can significantly enhance customer experience, service, and support in the following ways:
- Personalized experiences: Hybrid AI can help businesses offer personalized experiences to their customers. Machine learning algorithms can analyze customer behavior, preferences, and past interactions to understand what each customer might want or need. This allows businesses to tailor their offerings to individual customers, enhancing the customer experience.
- Intelligent virtual assistants: Hybrid AI can power intelligent virtual assistants or chatbots that can handle a wide range of customer service tasks. The combination of neural networks and symbolic AI allows these assistants to understand natural language inputs, respond in a human-like manner, and even understand complex instructions or queries. As a result, customers can get instant, 24/7 support for many common issues, improving customer satisfaction.
- Predictive customer service: Hybrid AI can help predict customer needs before they arise. Machine learning models can forecast future customer behavior or issues by analyzing past data and recognizing patterns. This allows businesses to address these needs, proactively improving customer service.
- Automated support systems: Businesses can automate many customer support tasks with hybrid AI. For instance, AI can analyze incoming support tickets, categorize them based on urgency and subject, and route them to the appropriate department or support agent. This can help reduce response times and improve the efficiency of customer support.
- Understanding customer sentiment: Hybrid AI can also be used to analyze customer feedback and understand customer sentiment. Natural language processing (NLP), a field of AI that helps computers understand human language, can be used to analyze customer reviews, social media posts, and other forms of feedback. This can provide valuable insights into how customers feel about a business and its products or services.
- Enhancing omnichannel support: In today’s digital age, customers interact with businesses through various channels – email, phone, social media, live chat, etc. Hybrid AI can help businesses integrate these different channels into a seamless omnichannel support system. Machine learning algorithms can analyze data from all these channels to provide a unified view of each customer. This allows businesses to provide consistent and high-quality support across all channels.
Targeted marketing
Hybrid AI can significantly augment marketing strategies and operations. Here’s how:
- Personalization: Hybrid AI can analyze customer data to understand individual preferences and behaviors. This enables businesses to tailor their marketing messages and offers to each customer, providing a highly personalized experience that can drive engagement and conversions.
- Predictive analytics: Hybrid AI can be used to predict future trends based on past data. For example, it can forecast which products are likely to become popular, when demand for certain items might increase, or how marketing campaigns might perform. These insights can help marketers make informed decisions and strategies.
- Customer segmentation: Through machine learning algorithms, hybrid AI can analyze a vast array of customer data and identify distinct groups or segments based on shared characteristics. Marketers can use this information to create highly effective and targeted campaigns.
- Content creation and curation: Hybrid AI systems can assist in creating and curating content for marketing campaigns. For instance, AI can generate personalized email content, social media posts, or even blog articles. Additionally, AI can help curate the most relevant content for individual customers, improving engagement rates.
- Chatbots and virtual assistants: These can be used for marketing purposes, providing personalized recommendations, and interacting with customers in real time. They can answer queries, provide product recommendations, and even guide customers through the buying process, all of which can enhance the customer experience and potentially increase sales.
- Optimizing ad campaigns: Hybrid AI can analyze the performance of ad campaigns across different platforms and identify what’s working and what’s not. It can adjust parameters in real-time, such as bidding strategies and audience targeting, to optimize the effectiveness and ROI of advertising efforts.
- Social media monitoring: Hybrid AI can monitor social media platforms to understand what customers say about a brand, its products, or its competitors. This can provide valuable insights for marketing strategies and help businesses respond quickly to any issues or opportunities.
- A/B testing: Hybrid AI can automate and enhance A/B testing processes, allowing marketers to more quickly and accurately understand what strategies, messages, or designs are most effective with their audience.
Thus, hybrid AI can provide valuable insights, automate routine tasks, and enhance decision-making in marketing. By leveraging the strengths of different AI techniques, marketers can drive more effective, personalized, and responsive marketing strategies.
Smarter supply chains
Hybrid AI can significantly impact supply chain management by enhancing efficiency, reducing costs, and improving decision-making. Here are some ways it can be utilized:
- Demand forecasting: Hybrid AI can analyze a variety of factors, such as historical sales data and market trends, to make accurate predictions about future demand. This can help businesses plan their production and inventory management more efficiently, reducing storage costs and the risk of stockouts or overstock.
- Supply chain optimization: Hybrid AI can optimize various aspects of the supply chain. For example, it can determine the most efficient routes for transport, optimize warehouse layout for quicker picking and packing, or find the ideal mix of transportation modes to minimize costs and delivery times.
- Risk management: By analyzing data from various sources, hybrid AI can predict potential supply chain disruptions like delays, shortages, or quality issues. This allows businesses to proactively mitigate these risks, for instance, by finding alternative suppliers or adjusting their production schedule.
- Real-time visibility: Hybrid AI can provide real-time visibility into the supply chain by tracking goods from production to delivery. This can help businesses quickly respond to any issues, improve customer service by providing accurate delivery estimates, and reduce theft and loss.
- Supplier selection and management: Hybrid AI can analyze supplier performance based on factors such as cost, quality, reliability, and sustainability. This can help businesses select the best suppliers and manage their relationships more effectively.
- Inventory management: Hybrid AI can predict which items are likely in high demand and which are not, helping businesses optimize their inventory levels. This can reduce storage costs and the risk of obsolescence while ensuring that popular items are always in stock.
- Sustainable supply chain: Hybrid AI can also contribute to creating a more sustainable supply chain by identifying ways to reduce waste, lower energy consumption, or minimize the carbon footprint of transport.
In summary, hybrid AI can significantly enhance supply chain management by providing accurate forecasts, optimizing operations, managing risks, improving visibility, and contributing to sustainability. This can lead to lower costs, improved customer service, and a more resilient and sustainable supply chain.
Safer and smarter operations
Hybrid AI can significantly enhance operations across various industries. Here’s how:
- Process automation: Hybrid AI can automate routine and repetitive tasks, such as data entry, scheduling, or inventory management. This can free employees to focus on more complex and strategic tasks, thereby increasing efficiency and productivity.
- Predictive maintenance: By analyzing data from sensors and machine logs, hybrid AI can predict when equipment will likely fail or need maintenance. This can help businesses prevent downtime and extend the lifespan of their equipment.
- Quality control: Hybrid AI can be used to enhance quality control processes. For instance, machine learning algorithms can analyze images or sensor data to detect defects in products, while symbolic AI can ensure that all quality standards and rules are followed.
- Resource optimization: Hybrid AI can analyze operations data to identify inefficiencies and opportunities for optimization. For example, it can optimize the use of resources in manufacturing, determine the most efficient routes for transport, or schedule staff to maximize productivity.
- Risk management: Hybrid AI can analyze a variety of data to predict potential risks and disruptions, such as supply shortages, equipment failures, or safety incidents, allowing businesses to proactively mitigate risks.
- Decision support: Hybrid AI can support decision-making by providing accurate forecasts and insights. For instance, it can predict the impact of different decisions on key performance indicators, helping managers make more informed decisions.
- Enhancing customer service: Hybrid AI can enhance customer service operations by automating responses to common queries, providing personalized recommendations, and predicting future customer needs, which improves customer satisfaction and loyalty.
- Supply chain management: Hybrid AI can provide real-time visibility into the supply chain, optimize inventory levels based on demand forecasts, and manage supplier relationships more effectively.
Hybrid AI can help businesses automate routine tasks, enhance quality control, optimize resources, manage risks, support decision-making, and improve customer service. By integrating different AI techniques, businesses can achieve higher efficiency, productivity, and resilience in their operations.
Launch your project with LeewayHertz!
Embrace the future with hybrid AI. Contact our AI experts to transform your business with advanced hybrid AI solutions!
Quality control and quality assurance
Hybrid AI can substantially improve Quality Control (QC) and Quality Assurance (QA) processes. Here’s a detailed breakdown of how it can do so:
- Automated inspections: Hybrid AI can be used to automate visual inspections in manufacturing processes. Machine learning algorithms, particularly deep learning models, can be trained to recognize product defects by analyzing images or videos. These systems can identify issues that may be difficult for human inspectors to detect and can do so quickly and consistently without fatigue.
- Predictive quality control: By analyzing historical process data, hybrid AI can predict potential quality issues before they occur. For example, it can identify patterns or anomalies in production data that could lead to defects. This allows businesses to proactively address these issues, thereby preventing defects and reducing waste.
- Integrating human expertise: Symbolic AI can integrate human expertise into the QC process. For example, rule-based systems can encode the knowledge of experienced human inspectors into the AI system. This can ensure that the AI system adheres to established quality standards and practices.
- Process optimization: Hybrid AI can analyze QC data to identify opportunities for process improvement. For example, it can determine which factors are most likely to impact product quality and suggest changes to the production process to improve quality outcomes.
- Real-time monitoring and feedback: Hybrid AI systems can provide real-time monitoring of production processes, providing instant feedback when quality issues are detected. This allows for immediate corrective action, preventing the production of further defective units and reducing rework costs.
- Improving QA testing: In software development, hybrid AI can enhance QA testing by automating the generation of test cases, predicting where bugs are most likely to occur, and even identifying potential issues in software requirements or design documents.
- Documentation and traceability: Hybrid AI can automate QC activities and outcomes documentation, providing a detailed and accurate record for traceability purposes. This is particularly important in regulated industries, where businesses need to demonstrate compliance with quality standards.
Thus, hybrid AI can enhance QC and QA by automating inspections, predicting quality issues, integrating human expertise, optimizing processes, providing real-time monitoring and feedback, enhancing testing, and improving documentation and traceability. This can lead to higher product quality, improved efficiency, reduced waste, and better compliance with quality standards.
Contextual understanding
Hybrid AI can significantly enhance contextual understanding. Here’s how:
- Natural Language Processing (NLP): NLP, a subfield of AI, encompasses the interface between human language and computer systems. Hybrid AI can improve NLP systems by using machine learning to understand the nuances and complexities of human language and symbolic AI to understand grammar rules, idioms, and other language structures. This can enhance the ability of AI systems to understand and generate human language in a contextually accurate manner.
- Contextual recommendations: Hybrid AI can improve recommendation systems by incorporating more context into their predictions. For example, machine learning algorithms can analyze user behavior to understand their preferences, while symbolic AI can incorporate additional contextual information, such as the time of day, the user’s location, or the device they are using.
- Context-aware computing: In context-aware computing, hybrid AI can be used to enhance the ability of a system to understand and respond to its environment. For instance, a context-aware application might use machine learning to predict user behavior based on past interactions and symbolic AI to understand and apply rules about the user’s context, such as their location or the current time.
- Semantic understanding: Hybrid AI can improve an AI system’s ability to understand the meaning of different words or phrases in different contexts. Machine learning can learn these different meanings from data, while symbolic AI can be used to understand the rules of language and logic that govern these meanings.
- User intent understanding: In tasks such as voice recognition or search query interpretation, hybrid AI can improve the system’s understanding of user intent. Machine learning can predict intent based on past data, while symbolic AI can be used to understand the rules and structures of language that can provide additional context.
- Contextual advertising: In digital advertising, hybrid AI can improve ad targeting by incorporating more context. Machine learning can be used to predict user interests and behaviors, while symbolic AI can incorporate additional context, such as the content of the webpage the user is viewing.
In summary, by combining different AI techniques, hybrid AI can provide a more robust and nuanced understanding of context. This can lead to more accurate predictions, personalized recommendations, and natural and effective interaction between humans and AI systems.
Process optimization
Hybrid AI can substantially enhance process optimization across various industries. Here’s how:
- Predictive analytics: Hybrid AI can analyze historical data and identify patterns that may influence process outcomes. For example, in a manufacturing setting, it could predict which variables in the production process might lead to defects. Businesses can use these insights to adjust their processes, improving quality and reducing waste.
- Real-time process monitoring: By collecting and analyzing real-time data from processes, hybrid AI systems can identify inefficiencies as they occur. For instance, if a piece of machinery starts operating outside its ideal parameters, the AI system could alert operators to this issue, allowing immediate corrective action.
- Automation: Many routine tasks within processes can be automated using hybrid AI. This can reduce the potential for human error, speed up process times, and free up human employees for more complex tasks. Additionally, machine learning algorithms can improve this automation over time by learning from past performance.
- Scheduling and resource allocation: Hybrid AI can optimize the scheduling of tasks and allocation of resources within processes. For instance, in a logistics operation, hybrid AI could determine the optimal routes for delivery trucks, minimizing travel time and fuel consumption.
- Quality control: Hybrid AI can enhance quality control within processes. Machine learning algorithms can analyze data to detect anomalies that might indicate a quality issue, while symbolic AI could ensure that the system adheres to quality standards and rules.
- Process design and simulation: Hybrid AI can assist in designing processes and simulating their performance under different conditions. This can help organizations identify potential bottlenecks or inefficiencies before implementing a new process.
- Decision support: By providing accurate forecasts and insights, hybrid AI can support decision-making related to process optimization. For instance, it could predict the impact of different decisions on key performance indicators, helping managers make more informed decisions.
In summary, hybrid AI can enhance process optimization by providing predictive analytics, real-time process monitoring, automation, optimized scheduling and resource allocation, enhanced quality control, and improved process design and simulation. Integrating different AI techniques enables more efficient, effective, and reliable processes.
Effective learning
Hybrid AI can significantly enhance the effectiveness of learning in various ways. Here’s how:
- Personalized learning: Hybrid AI can analyze a learner’s behavior, performance, and preferences to create personalized learning paths. This personalization can improve engagement and cater to each learner’s unique needs and strengths, thereby enhancing the effectiveness of learning.
- Adaptive learning systems: These systems can adjust the difficulty level of tasks, the type of content delivered, and the pacing of instruction based on the learner’s performance and progress. Hybrid AI is instrumental in building these systems as machine learning algorithms can predict what a learner needs and symbolic AI can apply rules to adapt the content.
- Predictive analytics: Based on historical data, hybrid AI can be used to predict learner outcomes, such as test scores or course completion rates. This can help educators identify learners who may need additional support or intervention.
- Intelligent tutoring systems: These are computer systems designed to offer instant and personalized guidance or feedback to learners, typically without human involvement. Hybrid AI can power these systems, using machine learning to understand the nuances of a learner’s behavior and symbolic AI to provide rule-based tutoring.
- Learning analytics: Hybrid AI can analyze data from learning activities to provide insights into learning processes. These insights can be used to improve instructional design, assess learner performance, and enhance the overall effectiveness of learning.
- Automated assessment: Hybrid AI can automate grading assignments and tests, freeing up time for educators to focus on other tasks. Machine learning can be used for pattern recognition tasks, such as grading multiple-choice questions or assessing written responses, while symbolic AI can ensure that grading follows certain standards and rules.
- Virtual Reality (VR) and Augmented Reality (AR) learning: Hybrid AI can be used in VR and AR learning environments, providing learners with interactive, immersive experiences. AI can adapt to the virtual environment based on the learner’s actions, enhancing engagement and learning effectiveness.
In summary, hybrid AI can enhance learning effectiveness by providing personalization, adaptivity, predictive analytics, intelligent tutoring, learning analytics, automated assessment, and immersive learning experiences. Integrating different AI techniques can create more powerful, efficient, and engaging learning environments.
How to get started with hybrid AI?
Implementing hybrid AI involves careful strategic planning, data collection and management, setting up the necessary tools and infrastructure, building or finding the necessary skills and talent, and an ongoing cycle of experimentation, iteration, and integration.
Let’s elaborate on each of these steps to get started with hybrid AI:
- Lead with strategy: This initial step is all about defining your goals and objectives. What specific problems are you trying to solve with hybrid AI? This could be anything from reducing operational costs to accelerating business growth or improving customer satisfaction. It’s crucial to align your hybrid AI strategy with these objectives. Remember that technology should serve your business needs, not the other way around. Strategic planning should also involve identifying key stakeholders, setting up a timeline, and defining metrics for success.
- Get the data: AI systems, including hybrid AI, require data to function effectively. Identify the types of data you will need to address your defined objectives. This could be structured data (such as databases or spreadsheets), unstructured data (like text documents or images), or streaming data in real time. Depending on your objectives, you might need to collect new data, clean or preprocess existing data, or both. You must also ensure that you comply with all relevant data privacy regulations.
- Implement the tools and infrastructure: This involves setting up the technological foundations for your hybrid AI system. Depending on your needs and resources, you will need to decide whether to use cloud-based solutions, on-premises servers, or hybrid infrastructure. You should choose tools and platforms that allow you to effectively manage and analyze your data, build and train AI models, and integrate these models into your existing workflows. Also, consider whether you will need specialized hardware for tasks such as deep learning.
- Find or build relevant skills and talent: Implementing hybrid AI requires specific skills. You will need data scientists to build and train AI models, data engineers to manage your data infrastructure, and domain experts who understand the specific problems you are trying to solve. Additionally, it’s crucial to have leaders and managers who understand AI and can guide its strategic implementation. If these skills are not available within your organization, consider upskilling your existing workforce, hiring new talent, or partnering with external consultants or AI service providers.
- Experiment and iterate: Once you have defined your strategy, collected your data, set up your infrastructure, and assembled your team, you can start building your hybrid AI system. This will likely be an iterative process involving testing different models, fine-tuning their parameters, and validating their performance. Remember to continuously monitor your system once it’s deployed to ensure it performs as expected and make any necessary adjustments.
- Scale and integrate: After testing and validating your hybrid AI system, the next step is to scale it up and fully integrate it into your business processes. This might involve training your AI models on more data, deploying them on more powerful hardware, or expanding their use to additional areas of your business.
Is hybrid intelligence the future?
Yet, the research also emphasizes that AI systems still face challenges when executing complex activities humans perform intuitively. Examples include conducting a natural human dialogue or planning a trip. The limitations also extend to making errors that can be distressing or harmful to users.
The solution lies in hybrid intelligence for industries where AI deployment is crucial, such as medical diagnosis and autonomous vehicles.
In April 2022, McKinsey, a global management consulting firm, merged with QuantumBlack, a sophisticated analytics startup based in London, known for its team of over 30 data scientists, data engineers, and designers. Post-integration, it is referred to as McKinsey’s “unified AI arm.” Alex Sukharevsky, a leader at QuantumBlack in McKinsey, mentions that despite the integration, the original principle remains unchanged: combining human intellect and domain expertise with innovative technology to address the most challenging problems. This approach, known as hybrid intelligence, is the foundation of each project.
Francois Laborie, a Forbes Technology Council contributor, indicates that hybrid intelligence is particularly crucial in the domain of industrial analytics. While a predictive algorithm’s failure in the consumer industry might not have severe repercussions, the scenario changes dramatically in asset-heavy industries such as oil and gas, power and utilities, and manufacturing. In these high-stakes sectors, AI failure can lead to equipment malfunction, interruption of a process, or even risk to human life.
There are specific reasons why hybrid AI is the solution for these industries:
First, industrial machinery experiences operational changes over time. Although sensors in these industries have been collecting data, much less is usable for AI applications.
Second, the quality of data gathered from sensors and other IoT devices in industrial settings is often low. This is because the data is subject to harsh conditions and noise that introduce bias into the data.
Thus, hybrid AI, combining the strengths of both human intellect and advanced AI technology, is showing great promise as the future of AI, especially in critical industries.
Use cases of hybrid AI
Hybrid AI is an approach that combines rule-based systems (classical AI) and statistical learning methods (modern AI). By combining these techniques, we can use the best features of each. Here are several detailed use cases for hybrid AI.
Healthcare:
- Personalized treatment plans: Hybrid AI can use rule-based AI to adhere to strict treatment protocols while leveraging machine learning to predict patient outcomes based on vast medical data. This allows for tailoring treatment strategies to individual patients, potentially improving care and outcomes.
- Early disease detection: Machine learning algorithms can analyze medical imaging data to identify patterns indicative of early-stage diseases, while rule-based systems can ensure compliance with diagnostic guidelines. This combination can lead to earlier detection and potentially more effective treatment.
- Drug discovery and development: Hybrid AI can analyze vast amounts of data on drug interactions, patient responses, and molecular structures to identify promising drug candidates. Rule-based systems can ensure compliance with regulatory requirements throughout the drug development process.
Finance:
- Risk management and fraud detection: Rule-based systems can enforce regulatory guidelines and standard procedures, while machine learning algorithms can analyze financial data to identify anomalies and predict potential risks, helping financial institutions proactively mitigate fraud and manage risk.
- Personalized financial advice: Rule-based systems can ensure compliance with financial regulations, while machine learning algorithms can analyze customer spending patterns and financial goals to provide personalized financial advice and recommendations.
Autonomous vehicles:
- Navigation and decision-making: For autonomous vehicles, rule-based AI ensures compliance with traffic laws and road safety protocols, while machine learning algorithms analyze real-time sensor data to identify obstacles, pedestrians, and optimal routes for safe and efficient driving.
- Predictive maintenance: Machine learning can analyze vehicle performance data to predict potential malfunctions, enabling timely repairs and reducing maintenance costs. This can be combined with rule-based systems for managing maintenance schedules and ensuring compliance with safety regulations.
- Adaptive driving: Machine learning can continuously learn and adapt to different driving conditions, such as traffic, and road surfaces, to optimize driving behavior and enhance safety. Rule-based systems can ensure adherence to traffic laws and safety protocols even in complex situations.
Manufacturing:
- Production optimization: In manufacturing processes, hybrid AI can optimize production lines by analyzing data on production processes, resource allocation, and demand forecasts. Rule-based systems can ensure adherence to quality control standards, while machine learning can predict and detect defects, minimizing waste and improving efficiency.
- Predictive maintenance: Machine learning can analyze sensor data from manufacturing equipment to predict potential failures, allowing for proactive maintenance and reducing downtime. Rule-based systems can enforce maintenance schedules and safety protocols.
- Quality control: Machine learning algorithms can detect defects in products by analyzing images and data from various sensors, while rule-based systems can enforce strict quality control guidelines. This combination can ensure consistent product quality and reduce manufacturing defects.
Supply chain and logistics:
- Route optimization: Machine learning algorithms can analyze historical data and real-time traffic conditions to optimize delivery routes, minimizing delivery times and costs. Rule-based systems can ensure compliance with regulations and delivery schedules.
- Inventory management: Machine learning algorithms can analyze historical data and demand forecasts to optimize inventory levels, reducing storage costs and preventing stockouts. Rule-based systems can enforce inventory management policies and safety regulations.
Retail and e-commerce:
- Personalized recommendations: ML algorithms can analyze customer data, including purchase history, browsing behavior, and demographics, to provide personalized product recommendations. Rule-based systems can ensure compliance with business strategies and pricing policies.
- Targeted marketing: Machine learning can analyze customer data to identify target audiences for specific products or promotions, while rule-based systems can ensure compliance with marketing regulations and ethical guidelines.
- Fraud detection: Hybrid AI can analyze transaction data to detect fraudulent activities, such as credit card fraud or account takeover. Rule-based systems can enforce security protocols, while machine learning can identify patterns indicative of fraudulent behavior.
Smart cities:
- Traffic management: Machine learning algorithms can analyze traffic patterns and data from sensors to optimize traffic flow, reduce congestion, and improve public transportation efficiency. Rule-based systems can enforce traffic laws and manage traffic signals.
- Resource optimization: Machine learning can analyze data from smart meters and sensors to optimize energy consumption and water usage, while rule-based systems can ensure compliance with environmental regulations and resource allocation policies.
- Public safety: ML algorithms can assess data from various sources, such as surveillance cameras, sensor networks, and social media, to detect and predict potential security threats and crime patterns. Rule-based systems can enforce safety protocols and respond to emergencies.
Cybersecurity:
- Threat detection: ML algorithms can analyze network traffic patterns, identify anomalies, and detect potential cyberattacks. This can be combined with rule-based systems that enforce security protocols and implement threat response strategies.
- Security incident response: Hybrid AI can analyze security incidents to identify root causes and implement effective mitigation measures. Rule-based systems can ensure compliance with security policies, while machine learning can analyze threat data and identify potential future threats.
- Adaptive security: Machine learning can continuously learn and adapt to evolving cyber threats, identifying new attack patterns and vulnerabilities. This allows for proactive security measures and the development of more effective defense mechanisms.
Legal
- Automated document review: In the legal industry, ML algorithms can analyze large volumes of legal documents, identifying relevant information and extracting key clauses, saving lawyers significant time and effort during due diligence and contract review. Rule-based systems ensure compliance with legal standards and ensure the accuracy of the extracted information.
- Risk assessment and mitigation: Hybrid AI can analyze data on legal risks and potential liabilities, identifying and prioritizing high-risk areas. Rule-based systems can enforce risk mitigation strategies, while machine learning can predict future risks and suggest preventive measures.
- Fraud detection: Machine learning can analyze financial and legal data to identify patterns indicative of potential fraud. Rule-based systems can enforce fraud prevention protocols and alert legal teams to potential misconduct.
Customer service
- Chatbots and virtual assistants: Chatbots can handle routine inquiries and simple issues automatically using pre-defined rules and responses. Machine learning allows them to learn from past interactions and improve their ability to understand and respond to complex queries.
- Ticket routing and prioritization: Machine learning can analyze customer tickets to automatically route them to the appropriate support team based on content and urgency. Rule-based systems can ensure compliance with service level agreements and internal processes.
- Customer sentiment analysis: Machine learning can analyze customer feedback to understand sentiment and identify areas for improvement. Rule-based systems can be used to track and report on customer satisfaction metrics, ensuring a proactive approach to customer service.
HR (Human Resources):
- Automated screening and shortlisting: Machine learning can analyze resumes and job applications, identifying the most qualified candidates based on skills, experience, and other criteria. Rule-based systems can ensure compliance with legal requirements and company policies during the screening process.
- Automated onboarding: Hybrid AI can automate the onboarding process, providing new hires with personalized information and training materials. Rule-based systems ensure compliance with legal requirements and company policies, while machine learning can tailor the experience based on employee roles and needs.
- Automated HR processes: Hybrid AI can automate repetitive HR tasks, such as payroll processing, benefits administration, and performance management. Rule-based systems ensure accuracy and compliance, while machine learning can handle exceptions and adapt to changing needs.
IT (Information Technology):
- Automated incident response: Hybrid AI can analyze logs and system data to detect and respond to security incidents automatically. Rule-based systems can trigger predefined actions, while machine learning can identify new threat patterns and adapt response strategies.
- Automated ticket routing: Machine learning algorithms can analyze IT support tickets, identifying the most relevant team or individual for resolution. Rule-based systems can enforce service level agreements and ensure ticket prioritization.
- Automated code review: Machine learning can analyze code for potential vulnerabilities and coding errors, improving code quality and security. Rule-based systems can enforce coding standards and best practices.
Hospitality
- Personalized recommendations: Machine learning algorithms can analyze guest data, including booking history, preferences, and social media activity, to provide personalized recommendations for dining, activities, and other services. Rule-based systems can ensure compliance with hotel policies and pricing strategies.
- Automated concierge services: Machine learning-based chatbots can provide guests with 24/7 assistance, answering questions, booking services, and addressing requests. Rule-based systems can ensure consistent responses and adherence to brand messaging.
- Targeted marketing: Machine learning algorithms can analyze guest data to identify segments with specific needs and preferences, allowing for targeted marketing campaigns. Rule-based systems can ensure compliance with marketing regulations and privacy laws.
- Guest relationship management: Machine learning algorithms can analyze guest data to understand their preferences and needs, allowing for personalized communication and nurturing long-term relationships. Rule-based systems can ensure consistent branding and communication across touchpoints.
Importance of knowledge graphs in building hybrid AI systems
A knowledge graph plays a crucial role in developing a hybrid AI system. It serves as the backbone for data management and organization, helping the system to understand the relationships and connections between various pieces of information.
Here are the key aspects of the significance of a knowledge graph in creating a hybrid AI system:
- Data structuring and contextualization: Knowledge graphs help structure and organize complex data from multiple sources. It provides a framework for contextualizing information, which is critical in understanding and interpreting data correctly. This is particularly useful for hybrid AI systems, which often have to deal with vast amounts of diverse data.
- Semantic understanding: Knowledge graphs give meaning to data by defining the relationships and connections between different entities. This semantic understanding is vital for hybrid AI systems as it enables them to better comprehend and interact with the data, leading to more accurate results and more sophisticated interactions.
- Enhanced machine learning: With a well-structured knowledge graph, hybrid AI systems can improve their machine learning capabilities. The knowledge graph can guide the learning process, focusing on relevant relationships and reducing the noise in the data. This leads to more efficient learning and improved performance.
- Supporting rule-based systems: The organization and clarification provided by knowledge graphs are critical for the rule-based component of hybrid AI systems. By defining the entities and their relationships, knowledge graphs provide a clear framework for applying rules, making the system more effective and consistent.
How can LeewayHertz help you?
LeewayHertz employs a comprehensive and organized approach to creating knowledge graphs, a critical component in developing AI-based solutions. The entire process of knowledge graph creation involves five significant steps:
- Data organization and digitization: Recognizing that organizations must be able to bypass the digitization and structuring of their data, LeewayHertz assists in this critical initial step. They ensure the organization’s data is ready for further processing and inclusion in the knowledge graph.
- Knowledge graph development: With the digitized data, LeewayHertz proceeds to develop the knowledge graph, which they regard as the data structure of the future. This knowledge graph becomes the backbone of all subsequent AI-based applications, including chatbots and voice assistants.
- Process implementation: LeewayHertz continues beyond merely creating a knowledge graph. They implement organizational processes and workflows necessary for maintaining and updating the knowledge graph in the future. This way, your knowledge graph remains up-to-date and effective in driving AI functionalities.
- Expert input: The LeewayHertz team brings several decades of combined experience in knowledge graph development. Their vast experience is invaluable in refining the knowledge graph to suit specific use cases and ensure optimal performance in AI applications.
- Continuous support: LeewayHertz offers an end-to-end solution, providing continuous support throughout the process. They offer a hybrid AI platform where the knowledge graph can be easily managed, updated, and adapted at any time, thereby offering maximum convenience to businesses.
By following these steps, LeewayHertz provides a holistic solution that ensures the successful development of a comprehensive and effective knowledge graph, setting the stage for advanced, AI-driven solutions.
Endnote
It is indisputable that the future lies in hybrid intelligence – a convergence of human ingenuity and artificial intelligence. This fusion of AI and human intelligence will significantly transform our daily lives, societal norms, and perhaps even biological existence.
By leveraging the power of hybrid AI, we are stepping into a new digital age where raising children will involve navigating a world deeply intertwined with intelligent technology. Consider the significant impact of this on education, social interactions, and skill development. This transformation goes beyond merely improving efficiency; it has the potential to redefine what it means to be human.
Further, the role of hybrid intelligence in problem-solving is immensely promising. Hybrid AI isn’t limited to learning tasks explicitly programmed by humans. Instead, it has the potential to adapt to new environments, learning and solving problems autonomously. This self-driven creativity and autonomy will prove invaluable in tackling significant global challenges, such as climate change and health care.
One of the key elements enabling hybrid AI’s capabilities is the knowledge graph. By providing a structured framework that allows AI to understand the context and relationships within data, a knowledge graph empowers hybrid AI systems to perform more efficiently and with better comprehension. LeewayHertz, for instance, has built its success around developing comprehensive knowledge graphs for its clients, providing a foundation for advanced, AI-driven solutions.
So, as we progress into a future powered by hybrid intelligence, it’s essential to harness its potential responsibly and ethically. The key to unlocking its power lies in understanding its components, like the knowledge graph, and developing strategies that ensure it benefits humanity as a whole.
Step into the future with hybrid AI. Get in touch with LeewayHertz’s AI experts and transform your business with advanced hybrid AI solutions!
Start a conversation by filling the form
All information will be kept confidential.
Insights
Generative AI architecture for enterprises: Development frameworks, tools, implementation, and future trends
By understanding the architecture of generative AI, enterprises can make informed decisions about which models and techniques to use for different use cases.
From diagnosis to treatment: Exploring the applications of generative AI in healthcare
Generative AI in healthcare refers to the application of generative AI techniques and models in various aspects of the healthcare industry.
How to build a generative AI model for image synthesis?
With tools like Midjourney and DALL-E, image synthesis has become simpler and more efficient than before. Dive in deep to know more about the image synthesis process with generative AI.