How to build an AI solution for enterprise knowledge management? Implementation steps, benefits, challenges and trends
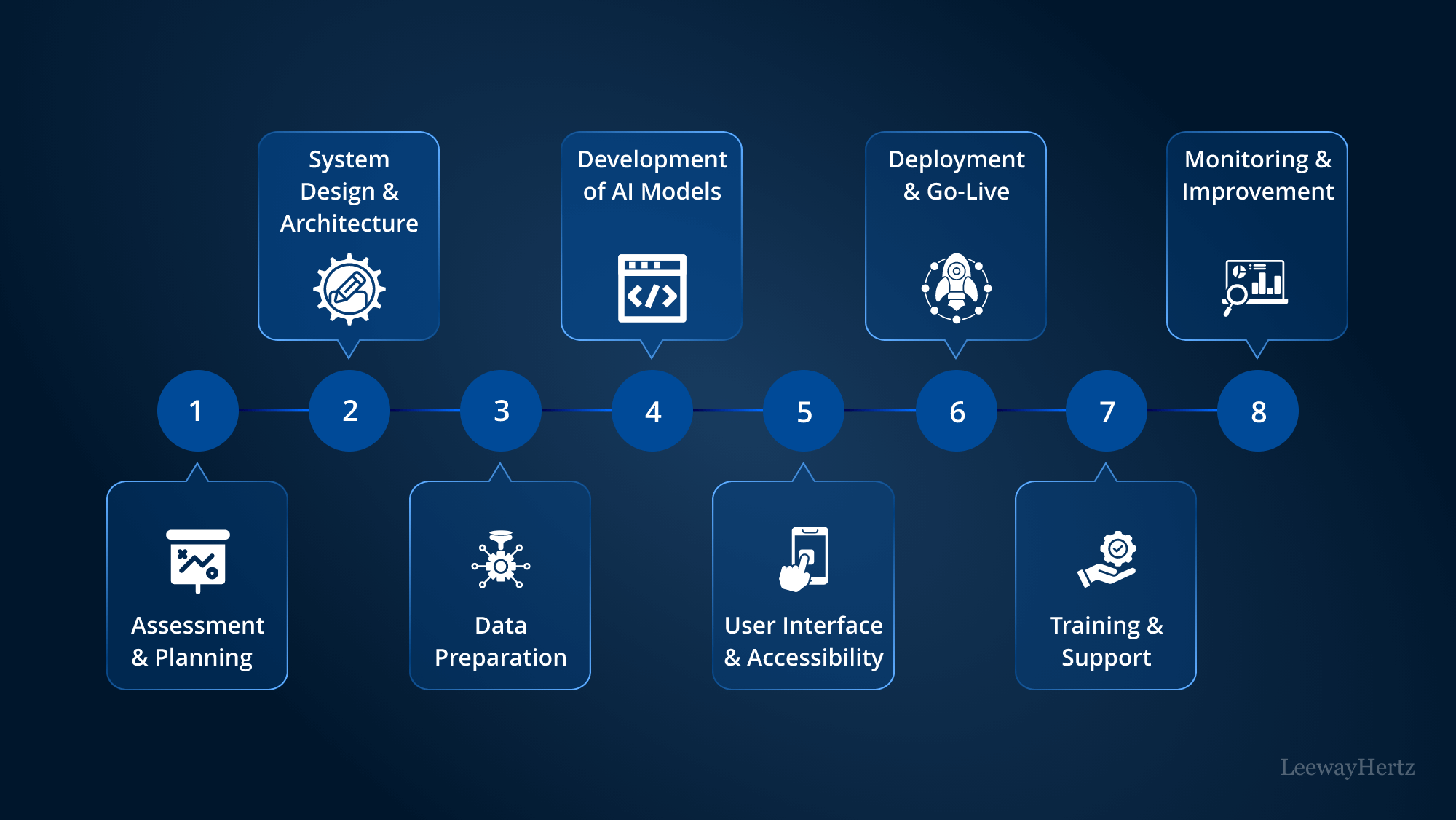
In today’s fast-paced business environment, managing and leveraging knowledge can provide enterprises with a distinct competitive edge. Artificial Intelligence (AI) solutions offer a transformative potential for enhancing knowledge management systems, making them more dynamic, intuitive, and capable of handling complex data landscapes.
Integrating artificial intelligence into knowledge management systems emerges as a crucial strategy. AI-driven knowledge management systems offer a centralized platform for all organizational knowledge—including documents, emails, and multimedia. By leveraging advanced AI capabilities such as natural language processing (NLP) and predictive analytics, these systems can intelligently organize content, anticipate user needs, and facilitate quicker decision-making. Moreover, AI enables enhanced security measures that are beyond human capabilities.
This transformative approach not only streamlines information accessibility but also boosts employee engagement by minimizing challenges associated with traditional systems. With AI, enterprises can not only safeguard their data but also foster a culture of efficiency and innovation. As remote and hybrid work models become the norm, the role of AI in knowledge management is not just beneficial but essential. A report from the McKinsey Global Institute highlights that implementing a robust knowledge management system (KMS) can decrease the time employees spend searching for information by up to 35%, potentially increasing overall productivity by 20-25%.
This article examines the strategic integration of AI into enterprise knowledge management. We will explore AI-powered knowledge management systems, their benefits, implementation, best practices, and future trends in this space.
- The role of AI in enterprise knowledge management
- Knowledge management challenges in enterprises
- Benefits of AI-powered knowledge management solutions to enterprises
- How do advancements in Generative AI and NLP transform knowledge management in enterprises?
- How to build AI solutions for enterprise knowledge management?
- Best practices for implementing AI solutions for enterprise knowledge management
- Challenges and considerations for building AI Solutions for enterprise knowledge management
- Leveraging LeewayHertz’s expertise for enterprise knowledge management solutions
- Future trends in AI-powered enterprise knowledge management
The role of AI in enterprise knowledge management
Knowledge management (KM) is a comprehensive discipline that integrates processes, strategies, and technologies to effectively manage both explicit and implicit knowledge within an organization. Explicit knowledge includes codified information such as documents and databases, while implicit knowledge comprises personal insights, expertise, and experiential learning. The core objective of KM is to facilitate organizational learning, enhance problem-solving capabilities, and support decision-making processes.
In the age of artificial intelligence, knowledge management (KM) goes beyond traditional limits, serving as a critical foundation for AI systems. It enables these systems to effectively process, analyze, and utilize data. AI for knowledge management involves the use of sophisticated algorithms and models to:
- Identify relevant information from a vast array of data sources.
- Capture this information systematically, ensuring accuracy and accessibility.
- Store data in a manner that supports easy retrieval and efficient management.
- Disseminate knowledge across the organization to promote innovation and efficiency.
By integrating AI in knowledge management not only improves information retrieval and dissemination but also enhances systems’ understanding and reasoning capabilities. This allows AI models to learn continuously from organizational data and apply this learning to optimize outcomes. As a result, AI-powered knowledge management systems can dynamically adapt to new information, refine their operations, and deliver tailored solutions that drive organizational success and strategic advantage.
According to IDC’s report, the typical knowledge worker spends about 2.5 hours per day, or roughly 30% of the workday, searching for information. As enterprises grow and become more complex, finding the right insights and information for impactful decision-making becomes increasingly challenging. This complexity is exacerbated by layered organizational and technological structures. To effectively tackle challenges in customer service and operational efficiency, teams need immediate access to precise and current information.
For example, within the insurance sector, companies manage a high volume of intricate processes, such as specialty claims and underwriting. These tasks require both personnel and technology to collaborate seamlessly for well-informed decision-making. Similarly, banks coordinate across teams, functions, and even different organizations when handling customer service requests for diverse products and offerings. Effective knowledge management systems are critical in these scenarios to streamline processes and enhance decision-making efficiency.
What is an AI knowledge management solution?
An enterprise AI knowledge management solution serves as a centralized platform for storing and organizing various types of knowledge, including documents, files, images, and videos. By leveraging AI algorithms, this solution efficiently categorizes, tags, and retrieves content based on its relevance and context, greatly improving the speed and accuracy with which users can access the information they need.
A key feature of an AI knowledge management platform is its ability to foster knowledge sharing and collaboration within an organization. It provides employees across various departments and locations access to a unified knowledge base, promoting a culture of continuous learning and innovation and enhancing productivity.
Furthermore, AI knowledge management platforms equip organizations to stay ahead of industry trends and developments. By monitoring and analyzing data specific to their sectors, these solutions enable organizations to anticipate changes and adapt their strategies accordingly, ensuring competitiveness.
Knowledge management challenges in enterprises
Before delving into the specifics of building AI-powered KM solution, it’s essential to understand enterprises’ unique challenges with knowledge management. These challenges underscore the need for innovative solutions to streamline and optimize knowledge management processes.
Extensive documentation
Enterprises generate a vast array of documents daily, encompassing areas like production, customer support, legal, HR, sales, and marketing. This documentation becomes more complex and voluminous as a company grows, particularly in industries such as manufacturing, where detailed records of processes and even minor product changes are crucial. With the introduction of new products, additional documentation—such as technical specifications, maintenance manuals, and user instructions—becomes necessary. Over time, managing these documents and their versions becomes unwieldy, turning into a Gordian knot, especially in enterprises with multiple branches.
Complex internal processes
The extensive and scattered documentation affects internal processes by limiting access to essential knowledge. This situation causes delays in sales, maintenance, and production operations. For instance, a production employee might need to consult multiple sources to complete a single task on the production line, or a maintenance technician may have to review various technical manuals before performing repairs.
Tedious searches for information
Navigating through an ocean of documents to find specific information can be a daunting task. The challenge is exacerbated when employees search numerous unconnected knowledge databases to find the necessary information to perform specific tasks, leading to delays, wasted time, and inefficiency.
Steep learning curve
New employees face significant challenges during the initial months as they need to absorb extensive information about the company’s products, services, and internal processes. The onboarding process becomes prolonged and less effective if the knowledge base is vast and poorly organized. Consequently, the enterprise has to invest more resources in training new hires until they are fully operational.
Limited access to organizational knowledge
Documentation and organizational knowledge often reside in multiple, unconnected systems, making it difficult to access and navigate. This fragmentation limits employees’ ability to efficiently retrieve and utilize company knowledge, which impacts their performance and the organization’s overall efficiency. In a world where knowledge is power, ensuring accessible and streamlined knowledge resources is crucial for empowering teams and enhancing organizational performance.
Inadequate data utilization and analytics
Many enterprises fail to fully utilize the vast amounts of data they collect. This underutilization stems from inadequate systems that cannot effectively analyze and interpret complex data sets. Without the proper tools to convert raw data into actionable insights, companies miss out on opportunities to optimize operations and make strategic decisions. AI-powered knowledge management solutions can bridge this gap by incorporating advanced data analytics capabilities, which help transform data into valuable knowledge. This ensures that enterprises not only store but also intelligently use their information to gain a competitive advantage.
Optimize Your Operations With AI Agents
Optimize your workflows with ZBrain AI agents that automate tasks and empower smarter, data-driven decisions.
Benefits of AI-powered knowledge management solutions to enterprises
AI-powered knowledge management solutions are transforming how organizations capture, store, and utilize knowledge, driving substantial benefits across various business functions. Let’s explore how these solutions are transforming businesses and fostering innovation:
Efficient knowledge capture
AI-powered knowledge management enables organizations to efficiently capture knowledge from diverse sources such as documents, emails, and conversations. This comprehensive capture ensures that valuable information is preserved, easily accessed, and shared across the organization, preventing knowledge loss and promoting informational continuity.
Improved knowledge discovery
AI knowledge management systems can analyze, categorize, and tag information, vastly improving the ease with which users can discover relevant knowledge. This not only enhances productivity but also significantly reduces the time employees spend searching for information.
Enhanced collaboration
AI solutions facilitate better collaboration by providing a centralized platform where team members can access and contribute knowledge. This shared access improves communication and collaboration, leading to more informed decision-making and greater innovation.
Personalized knowledge delivery
AI algorithms personalize knowledge delivery based on user preferences and behavior, ensuring that users receive the most relevant and timely information. This targeted delivery enhances user efficiency and effectiveness in their roles.
Increased organizational learning
By capturing and sharing knowledge systematically, AI knowledge management promotes a culture of continuous learning and improvement. This adaptability is crucial for staying competitive in today’s dynamic business environment.
Cost and time savings
These systems reduce the time and resources spent searching for information, leading to significant cost savings and improved operational efficiency. By leveraging existing knowledge, organizations can avoid redundancy and accelerate innovation.
Enhanced decision-making
With easy access to relevant and up-to-date knowledge, AI-powered systems enable better decision-making across all levels of the organization. This leads to smarter decisions and improved outcomes.
Compliance and risk management
AI knowledge management solutions help ensure compliance with regulations and mitigate risks by maintaining easy access to accurate and current information. This is crucial for adhering to industry standards and protecting organizational integrity.
Scalability and adaptability
AI-driven knowledge management solutions are designed to scale with the organization, accommodating increased demand and more complex data sets as the business grows. This scalability ensures that the knowledge management system evolves in line with the organization’s needs.
Multilingual support
Generative AI enhances the accessibility of knowledge by offering multilingual support, allowing global businesses to serve diverse populations effectively. This capability broadens the organization’s reach and facilitates international operations.
Automation of routine tasks
From document categorization to content generation, AI automates routine tasks, freeing up employees to focus on more strategic activities. This automation drives efficiency and reduces the likelihood of human error.
Enhanced security and data protection
AI-powered knowledge management platforms significantly strengthen data security and integrity. By employing sophisticated security algorithms and continuously monitoring access patterns, these platforms proactively identify and address potential threats. This vigilant approach is crucial for preserving the confidentiality and integrity of sensitive business information, ensuring robust protection against threats.
Improved customer insights and engagement
AI-driven knowledge management systems can analyze customer interactions and feedback to provide deeper insights into customer needs and preferences. This information can be used to enhance customer engagement strategies and tailor services to better meet customer expectations. Organizations can offer more personalized experiences by understanding consumer behavior patterns, improving satisfaction and loyalty.
Resource optimization
By automating routine tasks and organizing information efficiently, AI allows enterprises to optimize the allocation of human resources. Employees are freed from mundane tasks and can focus on higher-value activities that require human insight, creativity, and decision-making skills.
How do advancements in Generative AI and NLP transform knowledge management in enterprises?
Generative AI and Large Language Models (LLMs) are redefining the capabilities of knowledge management systems, transitioning them from static data repositories to dynamic, intelligent platforms capable of generating and leveraging knowledge in transformative ways. As organizations face the challenges of navigating vast amounts of data, these advanced technologies provide powerful solutions that streamline processes and enhance decision-making and innovation.
Generative AI and NLP advancements
Generative AI with Natural Language Processing (NLP) has significantly transformed traditional knowledge management systems from static repositories into dynamic, interactive platforms. This technological integration achieves several transformative outcomes:
- Generation of new knowledge: Generative AI can synthesize information by aggregating and integrating diverse data sources. This capability enables KM systems to generate new insights or content that did not previously exist, filling knowledge gaps and extending an organization’s informational reach.
- Context-aware responses: NLP allows enterprise knowledge management systems to understand the context behind queries, enabling them to provide responses that are relevant to the specific needs of the user. This contextual awareness facilitates more accurate and useful interactions with the system, much like interacting with a knowledgeable human assistant.
- Automation of knowledge organization and retrieval: These AI-based knowledge management system can automatically organize large volumes of data, tag them with relevant metadata, and retrieve information swiftly. This automation significantly reduces the time and effort required to manage and navigate through extensive datasets, increasing operational efficiency.
AI-driven efficiency
AI’s capability to automate the categorization and analysis of unstructured data has made knowledge management systems far more efficient and user-friendly:
- Enhanced information retrieval: AI algorithms improve the speed and accuracy of search functions within KM systems, enabling users to find necessary information much faster than traditional methods.
- Reduction in manual data handling: By automating routine data processing tasks, AI reduces the need for manual intervention, freeing up human resources for more complex and strategic activities.
- Continuous data optimization: AI-powered knowledge management systems continuously learn and improve from user interactions, enhancing their ability to serve up the most relevant and updated content.
Strategic AI integration
For AI to effectively enhance knowledge management systems, its integration must be strategically aligned with organizational goals:
- Assessment of needs: Organizations need to conduct thorough assessments to identify where generative AI can have the most significant impact, whether it’s improving data accessibility and organization, optimizing knowledge retrieval, or facilitating more informed decision-making.
- Solution selection: When selecting AI solutions for knowledge management, it’s crucial to choose between off-the-shelf or customized solutions based on specific knowledge processes and user needs. Custom solutions can address unique challenges in knowledge management, providing features tailored to enhance information structuring and retrieval.
- Integration with existing infrastructure: AI tools need to be integrated with existing IT infrastructure to maximize their effectiveness and minimize disruption to current processes. Integrating AI into existing knowledge management infrastructure requires careful planning to ensure compatibility and minimal disruption. AI tools should be designed to seamlessly connect with current knowledge bases and management systems to enhance, rather than complicate, knowledge workflows.
Advanced approaches and technologies
Leveraging advanced AI technologies such as self-hosted Large Language Models (LLMs) and Retrieval Augmented Generation (RAG) can significantly boost the capabilities of knowledge management systems:
- Self-hosted LLMs: These models are trained on an organization’s proprietary data, ensuring that the outputs are highly relevant and secure. Self-hosted LLMs can provide customized insights and responses based on the specific corpus of knowledge they have been trained on, making them ideal for organizations with unique or sensitive information.
- Retrieval Augmented Generation (RAG): RAG combines the capabilities of generative models with the ability to fetch information from external databases or documents. This method enhances the quality of responses by providing additional, contextually relevant information that may not be present in the training data.
Integrating Generative AI and NLP into enterprise knowledge management systems represents a paradigm shift in how organizations handle, utilize, and benefit from their information assets. By embracing these technologies strategically, enterprises can create more intelligent, efficient, and adaptable knowledge ecosystems that drive innovation and competitive advantage in the digital age.
How to build AI solutions for enterprise knowledge management?
Implementing an AI-powered solution for enterprise knowledge management (KM) is a complex but rewarding process that can significantly enhance an organization’s ability to capture, organize, and utilize its collective wisdom. This section outlines the key steps and considerations for building such a system.
Initial assessment and planning
The foundation of a successful AI-driven knowledge management solution lies in thorough preparation. Initiate the process by organizing meetings with stakeholders to capture their insights, needs, and expectations throughout the organization. These discussions will help align the project with strategic goals and ensure buy-in from key decision-makers.
Next, evaluate the current knowledge management practices and IT infrastructure. This assessment provides a baseline understanding of existing systems, workflows, and pain points. It also helps identify potential integration points for the new AI solution.
- Identify specific KM challenges in your organization
- Set clear goals for the AI solution (e.g., improving information retrieval, enhancing collaboration)
- Determine the types of knowledge to be managed (e.g., documents, emails, databases)
Based on these insights, define detailed functional and non-functional requirements for the new system. These requirements should address specific challenges in your organization’s knowledge management processes and set clear goals for improvement, such as enhancing information retrieval, fostering collaboration, or streamlining decision-making processes.
System design and architecture
With a clear understanding of requirements, design a robust system architecture incorporating AI technologies such as Natural Language Processing (NLP) and Machine Learning (ML). The technical blueprint should prioritize scalability to accommodate future growth and security to protect sensitive organizational knowledge.
Develop a data architecture plan that outlines how information from various sources will be consolidated and accessed. This includes designing an efficient database schema that supports quick retrieval and maintains data integrity.
Simultaneously, focus on user experience design by creating initial wireframes for the interface. The goal is to ensure the system is intuitive and accessible for employees at all levels, promoting widespread adoption and engagement.
Data preparation and integration
Data is the lifeblood of any AI system. Initiate data integration by compiling information from databases, document management systems, emails, and other relevant organizational sources. This process may involve negotiating access to different departmental silos and addressing any privacy or security concerns.
Once collected, apply data preprocessing techniques to clean, normalize, and structure the data. This step is crucial for ensuring the AI models have high-quality, consistent information to work with.
Implement Extract, Transform, and Load (ETL) processes to consolidate the prepared data into a central repository. This integration should maintain proper formatting and ensure accessibility for the system’s AI components.
- Gather relevant data from various sources across the organization
- Clean and preprocess the data to ensure quality and consistency
- Implement data governance policies to maintain data integrity
As AI plays a bigger role, address ethical considerations around bias in data and algorithms. Ensure fairness and transparency in the AI models used in the KM system.
An organization could benefit from including strategies for capturing and retaining critical knowledge, especially from departing employees or subject matter experts.
When collecting, storing, and using employee data within the KM system, follow data privacy regulations like GDPR and CCPA.
Development of AI models
With clean, integrated data available, focus on developing and training the AI models that will power the enterprise knowledge management solution. Implement NLP models to enhance text classification, automated tagging, and semantic search capabilities. These features will make it easier for employees to find relevant information quickly and accurately.
Construct and refine machine learning algorithms for predictive analytics and personalized recommendations. These models can help surface relevant knowledge to users based on their roles, projects, or past interactions with the system. The following technologies play a crucial role in this process:
- Natural Language Processing (NLP) for text analysis and understanding
- Machine Learning (ML) for pattern recognition and predictive analytics
- Deep Learning for complex data processing and insights generation
Once these technologies are selected, the next step is to develop core AI functionalities such as:
- Intelligent search and retrieval
- Automated categorization and tagging
- Content summarization and recommendations
- Knowledge gap identification
Continuously test the AI models against real-world datasets throughout the development process to ensure their effectiveness and accuracy. This iterative approach allows for refinement and optimization before full-scale deployment.
System implementation and integration
The core development phase involves coding the application layers and integrating the AI models with backend systems and user interfaces. This process should follow best practices in software development, including version control and code review processes.
Develop APIs to enable seamless communication between different system modules and potential integration with third-party applications. This approach ensures flexibility and allows for future expansion of the system’s capabilities.
Conduct comprehensive testing, including unit tests, integration tests, and user acceptance tests. This rigorous testing regime verifies system stability, functionality, and performance under various conditions.
User interface and accessibility enhancement
Refine the user interface based on feedback from the initial UX design phase. The goal is to create an intuitive, user-friendly interface that encourages adoption and regular use of the system.
Implement accessibility features to ensure the system is usable by all users, including those with disabilities. This step is crucial for creating an inclusive knowledge management environment.
Ensure the platform is responsive and functional across various devices, including desktops, tablets, and smartphones. This flexibility allows users to access knowledge resources from anywhere, enhancing productivity and collaboration.
Implement security measures
Implementing security controls such as data encryption, role-based access controls, and compliance with data protection regulations is essential in building an AI solution for enterprise knowledge management:
- Data encryption: Ensures the security and privacy of sensitive information within the knowledge management system, protecting against unauthorized access and data breaches.
- Role-based access controls: Facilitate system access based on user roles to enhance security and operational efficiency, ensuring users access only the information necessary for their duties.
- Compliance with regulations: Maintains adherence to legal standards such as GDPR or HIPAA, avoiding legal penalties and reinforcing the organization’s commitment to data protection.
Deployment and go-live
Develop a detailed deployment plan that minimizes operational disruption. This plan should include strategies for data migration, user onboarding, and contingency measures.
Begin with a pilot test, launching the system to a selected group of users. This controlled rollout allows for gathering initial feedback and making necessary adjustments before full-scale deployment.
Once refined, extend the deployment across the organization. Ensure all data and user connections are smoothly transitioned, and be prepared to address any issues that arise during this critical phase.
More emphasis could be placed on how the new AI-driven KM system will integrate with existing business processes and workflows to ensure seamless adoption.
Building an AI-powered knowledge management system involves a cultural shift within the organization. Include a change management plan to encourage user adoption and address potential resistance.
Training and support
Conduct comprehensive training sessions to familiarize employees with the new system and its features. These sessions should cater to different learning styles and skill levels.
Provide detailed documentation, including user manuals and online help resources, to support self-guided learning and troubleshooting.
Establish a dedicated support desk to address queries and issues promptly. This ongoing support and periodic system updates ensure the solution remains effective and relevant over time.
- Provide comprehensive training for employees
- Encourage knowledge sharing and collaboration
- Recognize and reward active contributors to the KM system
Monitoring and iterative improvement
Implement observability tools to monitor system performance and user engagement. These metrics provide valuable insights into how the system is being used and where improvements can be made.
Create mechanisms to collect user feedback regularly, such as surveys or focus groups. This direct input from users is invaluable for identifying pain points and opportunities for enhancement.
- Track key performance indicators (KPIs) to measure success
- Gather user feedback and address issues promptly
- Continuously update and refine the AI models
Use the gathered data and feedback to drive iterative updates to the system. Continuously improve functionality, user experience, and AI model performance to ensure the knowledge management solution evolves with the organization’s needs.
While KPIs are mentioned, stakeholders could benefit from a more detailed approach to measuring and demonstrating the return on investment of the AI-driven knowledge management solution.
By following this comprehensive approach, organizations can build a robust, AI-powered knowledge management solution that enhances decision-making, improves productivity, and drives innovation across the enterprise. The implementation process typically spans a few months, from initial assessment to full-scale deployment and iterative improvements. However, the benefits of such a system – including improved information accessibility, enhanced collaboration, and more informed decision-making – can provide lasting value to organizations across various industries, from healthcare and finance to education and technology.
Optimize Your Operations With AI Agents
Optimize your workflows with ZBrain AI agents that automate tasks and empower smarter, data-driven decisions.
Best practices for implementing AI solutions for enterprise knowledge management
Core principles to follow
This section outlines the essential principles for developing AI solutions for KM, emphasizing transparency, user-centric design, scalability, and ethical practices to ensure the technology is trustworthy, adaptable, and aligned with user needs.
a) Transparency:
- Documentation: Maintain detailed documentation of AI algorithms and decision-making processes to ensure clarity and accountability.
- User explanations: Provide user-friendly explanations on how AI-driven recommendations and decisions are generated, incorporating visualization of data flows and logic wherever possible.
- Audit trails: Implement systems to log and review AI-driven decisions, facilitating audits and reviews when needed.
b) User-centered design:
- User research: Conduct regular user research and usability testing to gather insights from end-users and refine the AI system to better meet their needs.
- Intuitive interfaces: Design intuitive interfaces that align with existing workflows, reducing learning curves and enhancing user adoption.
- Customization: Offer customizable user experiences to accommodate diverse user roles and preferences, allowing personalization of dashboards and controls.
c) Scalability:
- Modular architecture: Use a modular system architecture that supports easy integration of new features and can be scaled up without major overhauls.
- Cloud solutions: Employ cloud-based solutions to handle increases in data volume and user load dynamically, ensuring performance remains optimal.
- Efficient data pipelines: Design data pipelines that can scale horizontally, allowing for efficient processing and analysis of growing data volumes.
d) Ethical AI:
- Ethics oversight: Establish an ethics board to oversee the development and deployment of AI systems, ensuring ethical considerations are prioritized.
- Bias mitigation: Implement mechanisms to detect and mitigate biases in AI models, including the use of diverse training datasets.
- Regular audits: Conduct regular audits to identify and address unintended consequences or discriminatory outcomes, maintaining ethical integrity.
Best practices for knowledge management
Explore best practices that enhance how organizations capture, manage, and utilize knowledge. Focus areas include cultivating a knowledge-sharing culture, setting governance standards, leveraging technology, and maintaining an ongoing evaluation process to keep content relevant and useful.
a) Establish a knowledge-centric culture:
- Incentives for sharing: Create reward systems that recognize and incentivize knowledge sharing among employees. According to Deloitte research, 37% of businesses lack the incentive to create new knowledge.
- Collaborative platforms: Deploy platforms that facilitate cross-functional collaboration and knowledge exchange, enhancing collective intelligence.
- Knowledge events: To promote knowledge dissemination, host regular events like workshops, seminars, and informal ‘lunch and learn’ sessions.
b) Implement governance policies:
- Data management policies: Develop and enforce policies around data classification, retention, and disposal to maintain information lifecycle management.
- Knowledge roles: Clearly define roles and responsibilities related to knowledge management, ensuring accountability and efficient oversight.
- Intellectual property: Establish protocols to protect intellectual property and maintain confidentiality, securing sensitive organizational knowledge.
c) Leverage advanced technologies:
- Semantic search: Implement semantic search capabilities that go beyond keyword matching, using context and synonyms for enhanced retrieval accuracy.
- Automated categorization: Utilize machine learning for dynamic categorization and tagging of knowledge assets, improving organization and accessibility.
- NLP for analysis: Apply natural language processing tools for extracting themes, sentiments, and patterns from large volumes of unstructured data.
d) Continuous evaluation and refinement:
- KPIs for KM: Define and monitor key performance indicators related to knowledge management activities to gauge effectiveness and identify improvement areas.
- Stakeholder feedback: Regularly collect and analyze feedback from users and stakeholders to refine KM practices and technologies.
- Knowledge audits: Periodically review and refresh knowledge assets to ensure they remain relevant and up-to-date.
Strategies for effective AI integration in knowledge management
This section provides strategies for effectively integrating AI into knowledge management processes, highlighting the importance of aligning AI with expert insights, prioritizing high-quality data, ensuring system compatibility, and continuously refining AI applications to drive organizational knowledge goals.
- Align AI with domain expertise:
- Collaborate closely with subject matter experts when developing AI models.
- Ensure AI solutions complement and enhance human expertise rather than replace it.
- Regularly validate AI outputs against expert knowledge to maintain accuracy and relevance.
- Prioritize data quality and relevance:
- Implement robust data governance practices to ensure high-quality inputs for AI models.
- Regularly update and curate knowledge bases to maintain relevance.
- Develop processes for continuous data validation and cleaning.
- Ensure seamless integration:
- Design AI solutions that integrate smoothly with existing knowledge management workflows.
- Prioritize user-friendly interfaces that don’t require extensive technical knowledge to operate.
- Implement single sign-on and consistent user experiences across all knowledge management tools.
- Foster continuous learning:
- Implement feedback loops to continuously improve AI model performance.
- Encourage users to provide input on AI-generated insights and recommendations.
- Regularly retrain models with new data to keep pace with evolving organizational knowledge.
- Maintain transparency and explainability:
- Ensure AI decision-making processes are traceable and understandable.
- Provide clear explanations for AI-generated recommendations or insights.
- Maintain human oversight for critical decisions supported by AI.
- Balance automation and human touch:
- Use AI to automate routine tasks, freeing human experts for higher-value activities.
- Maintain human moderation for sensitive or complex knowledge areas.
- Develop escalation procedures for when AI encounters uncertainty or potential errors.
- Prioritize scalability and flexibility:
- Design AI systems that can grow with the organization’s needs.
- Ensure AI solutions can adapt to changing business requirements and new types of data.
- Implement modular architectures that allow for easy updates and expansions.
Organizations can create robust, effective, and user-friendly AI solutions for enterprise knowledge management by adhering to these best practices. These systems leverage advanced AI capabilities and align with organizational objectives, ethical standards, and user needs.
Optimize Your Operations With AI Agents
Optimize your workflows with ZBrain AI agents that automate tasks and empower smarter, data-driven decisions.
Challenges and considerations for building AI Solutions for enterprise knowledge management
Implementing AI solutions in enterprise knowledge management presents a few challenges that organizations must navigate carefully. This section explores these challenges and offers strategic considerations for addressing them effectively.
Data quality and integration
The foundation of any effective AI system is high-quality, well-organized data. However, many enterprises struggle with data inconsistencies, incompleteness, and fragmentation across various sources and formats. For instance, a multinational corporation may face significant hurdles in integrating and standardizing data from regional offices using disparate systems. Organizations should implement robust data management frameworks that standardize collection, validation, and aggregation processes to overcome this, ensuring data uniformity and accuracy across the enterprise.
Data privacy and security
As AI systems handle increasingly sensitive information, maintaining data privacy and security becomes paramount. Compliance with global regulations such as GDPR and HIPAA is non-negotiable. Regular system audits and adopting state-of-the-art security protocols are essential to safeguard sensitive knowledge and maintain regulatory compliance.
Scalability
As organizations grow, their knowledge management needs expand exponentially. AI-driven systems must be designed with scalability in mind to handle increasing data volumes and user demands efficiently. Cloud-based services offer a solution, providing the elasticity and high availability necessary to accommodate growth without compromising performance. This approach particularly benefits rapidly expanding businesses, such as e-commerce platforms dealing with ever-increasing product listings and customer interactions.
Expertise and training
The deployment and maintenance of AI systems require specialized knowledge in areas such as machine learning, natural language processing, and data science. Many organizations face a significant challenge in acquiring or developing this expertise internally. Companies should invest in comprehensive training programs for existing employees to address this skills gap and consider strategic partnerships with third-party consultants to augment their capabilities.
Change management
Integrating AI into existing knowledge management practices often necessitates substantial changes in workflows and organizational culture. Resistance to these changes can significantly impede adoption and effectiveness. A comprehensive change management strategy is crucial, encompassing stakeholder engagement, clear communication plans, and phased implementation to facilitate a smooth transition and encourage user adoption.
Bias and fairness
AI systems are susceptible to perpetuating and amplifying biases in their training data, potentially leading to unfair outcomes. Bias in AI-driven knowledge management solutions can lead to skewed information retrieval and decision-making processes. These biases, often present in the training datasets, can affect the quality and fairness of the knowledge delivered, potentially impacting operational decisions and strategic planning. To address this challenge, organizations must utilize diverse and representative data sets for training their AI models. Regular audits and updates of the AI systems are necessary to identify and mitigate biases, ensuring that the knowledge management solutions remain accurate and equitable across all user interactions.
User adoption
The success of AI-driven knowledge management systems hinges on user acceptance and engagement. Resistance to new technologies can hinder implementation efforts, especially among long-standing employees. Overcoming this challenge requires a focused approach to demonstrating the tangible benefits of the AI system, coupled with comprehensive training programs tailored to different user groups within the organization.
Continuous learning and improvement
AI models require ongoing refinement to maintain relevance and accuracy. This is particularly crucial in dynamic environments where the nature of knowledge evolves rapidly. Establishing protocols for continuous model training and data refreshes is essential to keeping AI systems effective and up-to-date and ensuring they continue to provide value over time.
Integration with existing systems
Many organizations face the challenge of integrating new AI solutions with legacy knowledge management systems. This integration can be complex and resource-intensive, often requiring significant custom development work. Utilizing APIs and middleware to create interoperable layers can facilitate effective communication between AI systems and existing technologies, streamlining the integration process.
Cost and ROI
The initial investment in AI for knowledge management can be substantial, and demonstrating a clear return on investment (ROI) can be challenging, especially for smaller enterprises. Developing a comprehensive business case that outlines potential cost savings, efficiency gains, and strategic advantages is crucial for justifying the expenditure and tracking ROI post-implementation.
Ethical considerations
The use of AI in knowledge management can raise important ethical questions, particularly regarding data usage and decision-making processes. Organizations must implement clear ethical guidelines governing AI use, emphasizing transparency, accountability, and human oversight to ensure responsible deployment of these technologies.
By addressing these challenges thoughtfully and strategically, organizations can harness the full potential of AI in enterprise knowledge management, driving innovation, efficiency, and competitive advantage in an increasingly data-driven business landscape.
Leveraging LeewayHertz’s expertise for enterprise knowledge management solutions
LeewayHertz stands at the forefront of AI development with a seasoned team of AI professionals specializing in creating custom solutions that cater to the specific needs of businesses. Their expertise in implementing advanced AI technologies, such as machine learning and natural language processing, makes them an ideal partner for developing comprehensive enterprise knowledge management solutions. Here’s how LeewayHertz can transform your organization’s KM capabilities:
Specialized AI expertise: LeewayHertz’s team is equipped with in-depth knowledge in machine learning, natural language processing, and other AI disciplines. This expertise is crucial in crafting enterprise AI solutions that enhance knowledge management processes, from automating data categorization to enhancing information retrieval systems. Their nuanced understanding of AI’s application across various industries ensures that solutions are not only innovative but also perfectly aligned with a company’s operational needs.
Tailored KM solutions: Drawing on vast experience, LeewayHertz develops custom AI solutions that transform how organizations manage and leverage knowledge. Whether it’s through developing intelligent search platforms that streamline data access or creating smart chatbots for real-time employee assistance, LeewayHertz tailors each solution to meet the unique challenges and objectives of your business.
Emphasis on data security: Recognizing the critical nature of data security in knowledge management, LeewayHertz integrates stringent security protocols into every phase of the AI solution development process. By prioritizing the protection of sensitive information, they ensure that the enterprise KM systems are not only effective but also secure against potential cyber threats, fostering trust and reliability in the deployed AI solutions.
Client-centric development approach: LeewayHertz begins every project with a detailed consultation to understand the specific knowledge management needs and challenges faced by their clients. This client-first methodology ensures that the developed AI solutions are highly relevant and effectively address the distinct challenges of managing corporate knowledge, such as improving knowledge accessibility and ensuring compliance with industry regulations.
End-to-end AI solution implementation: With a comprehensive approach to AI development, LeewayHertz supports organizations throughout the entire process of integrating AI into their knowledge management systems. This includes everything from the initial consulting to the final stages of deployment and maintenance. Their end-to-end service ensures that the AI solutions are seamlessly integrated, highly functional, and tailored to enhance knowledge management practices effectively.
By partnering with LeewayHertz, organizations can harness the power of AI to optimize their knowledge management processes and transform them into a strategic asset that drives innovation, efficiency, and competitive advantage in the marketplace.
Future trends in AI-powered enterprise knowledge management
The application of AI in enterprise knowledge management is evolving, with new technologies enhancing how companies capture, process, and utilize knowledge. Here are the key trends driving the future of AI-enhanced knowledge management in enterprises:
- Advanced Natural Language Processing (NLP): NLP technologies are becoming more sophisticated, allowing for deeper understanding and interpretation of human language within enterprise systems. This enables more effective search capabilities, smarter data categorization, and the extraction of meaningful insights from unstructured data sources like emails, documents, and online interactions.
- Integration of chatbots and virtual assistants: AI-powered chatbots and virtual assistants are becoming integral to enterprise knowledge management systems by providing immediate access to information and automating routine inquiries. This not only improves efficiency but also enhances user engagement across various departments.
- Semantic search engines: Beyond keyword-based searches, semantic search technologies understand the context of queries, improving search results’ accuracy and relevance. This is crucial for enterprises dealing with large and diverse data sets, enabling employees to find precise information swiftly.
- Complex data management through knowledge graphs: As data complexity grows, knowledge graphs become indispensable in enterprise knowledge management. By visualizing data relationships and contextualizing information, knowledge graphs enhance search capabilities and decision-making, proving crucial for businesses dealing with vast, interconnected datasets.
- Collaborative KM platforms: The future of knowledge management is inherently collaborative. Emerging KM platforms increasingly integrate with workflow tools, promoting real-time knowledge exchange and innovation. This trend is pivotal in dismantling informational silos and cultivating a culture of continuous learning.
- Enhanced focus on security and compliance: As digital knowledge expands, so does the focus on securing and regulating it. Future KM systems should prioritize robust security measures like end-to-end encryption, sophisticated access controls, and compliance with evolving global data regulations.
- Integration of KM and employee experience platforms: The convergence of knowledge management with employee experience platforms is set to redefine workplace interactions. This integration aims to boost productivity and employee engagement by embedding knowledge access into everyday workflows, offering seamless and immediate access to crucial information.
These trends highlight the dynamic integration of AI into knowledge management processes, illustrating a shift towards more intelligent, efficient, and secure information management within enterprises. As AI technologies evolve, they will unlock new capabilities and drive significant transformations in how knowledge is managed and utilized in the corporate world.
Endnote
The intersection of AI and knowledge management continually evolves, offering innovative solutions that enhance how enterprises handle and utilize vast data landscapes. As we’ve explored the various trends and technologies shaping this field, it’s clear that integrating AI into enterprise knowledge management systems simplifies data processing, enhances security, and fosters a culture of informed decision-making and continuous improvement.
Throughout this exploration of AI-driven enterprise knowledge management, we have explored a multitude of insights into how advanced technologies enhance and modernize the ways organizations manage, access, and leverage information. From the foundational strategies for integrating AI into knowledge management systems to the cutting-edge trends shaping the future of enterprise operations, this discussion underscores the profound impact AI is poised to have across various business sectors.
As we conclude, it is evident that the path forward for enterprises is intricately linked with the innovative use of AI technologies. The integration of AI not only propels the efficiency and effectiveness of knowledge management systems but also ensures that enterprises remain resilient, agile, and competitive in an increasingly digital world.
By harnessing the potential of AI, enterprises can unlock unprecedented levels of productivity and innovation, ensuring they not only survive but thrive in the ever-evolving landscape of global business.
Ready to elevate your enterprise knowledge management with AI? Discover LeewayHertz’s AI development services that can transform your business operations and enhance efficiency. Let AI take your enterprise’s knowledge management to the next level—Contact us today!
Start a conversation by filling the form
All information will be kept confidential.
Insights
GenAI in marketing: Unleashing unprecedented creativity and precision
From enhancing content creation and SEO optimization to driving customer engagement and reshaping market research, generative AI empowers marketers to leverage data-driven insights and automation for more effective and personalized campaigns.
AI in predictive maintenance: Use cases, technologies, benefits, solution and implementation
AI offers numerous applications in predictive maintenance across a variety of industries, leveraging its capabilities in data analysis, machine learning, and predictive analytics to improve equipment reliability and operational efficiency.
AI in accounting and auditing: Blazing new trails in financial management
AI has made significant inroads into the fields of accounting and auditing, redefining how financial data is processed, analyzed, and audited.