Generative AI in supply chain: Use cases, benefits and development
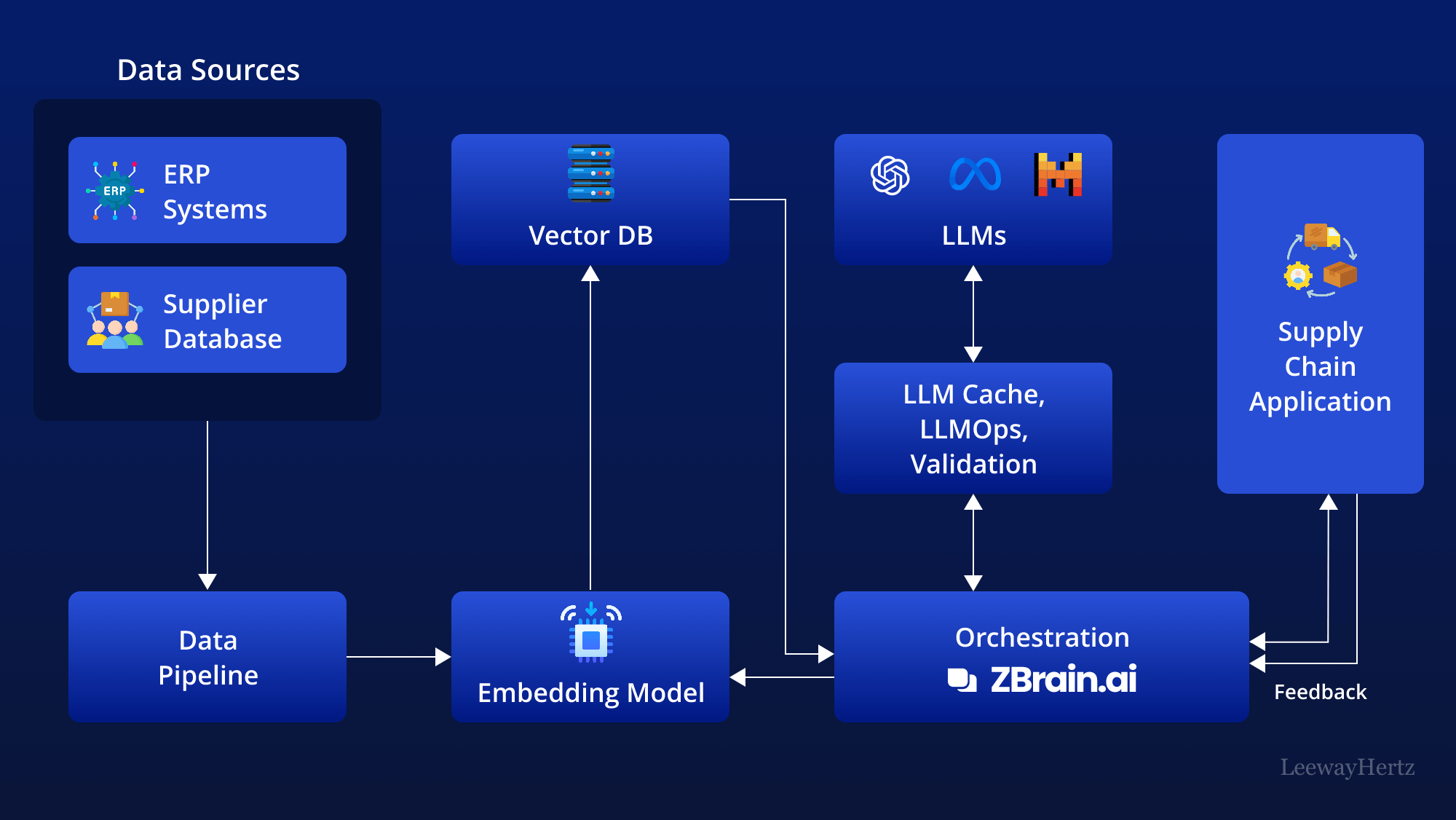
Listen to the article
The supply chain, a vital cog in the success of businesses across various sectors, comprises a complex network involving the production, distribution, and delivery of products and services. With technological advancements, Artificial Intelligence (AI) has emerged as a game-changing tool in refining supply chain operations.
The global supply chain is continuously evolving, aiming to enhance efficiency, reduce costs, and satisfy customers. However, it faces mounting complexities which stem from escalating customer expectations, rapid market changes, and an intensified call for sustainable methods.
This is where generative AI, a subcategory of artificial intelligence, steps in, providing innovative solutions to tackle these challenges. Through generative AI, supply chain stakeholders can process immense volumes of data, extract valuable insights, and streamline decision-making processes.
In March 2023, a significant stride was made when Microsoft announced Microsoft Dynamics 365 Copilot, an AI-driven assistant incorporated into CRM and ERP systems. Moreover, the release of ChatGPT by OpenAI for public users in November 2022 was a groundbreaking event that paved the way for anyone to explore the potential of generative AI.
The expectations from generative AI in the supply chain sector are high. AI-powered technology facilitates the smooth and sustainable flow of goods, components, and materials in a global, data-driven business environment. According to IDC, by 2026, 55% of Forbes Global 2000 OEMs are projected to have revamped their service supply chains with AI. This will enable businesses to take proactive measures, ensuring a more efficient and smooth supply chain operation. This proactive approach can help prevent delays, minimize downtime, and improve overall supply chain performance.
In recent IDC surveys, global organizations have expressed the need for improved supply chain visibility to mitigate challenges like cost increases and demand volatility. Generative AI holds the capability to fulfill these needs and more, aiding businesses in enhancing their transparency, efficiency, and overall resilience.
In this article, we will delve deeper into the applications and impact of generative AI within the supply chain sector.
- Generative AI in the supply chain: a brief overview
- Use cases of generative AI in supply chain
- Demand forecasting and planning
- Inventory optimization
- Managing supplier selection and relationships
- Predictive maintenance
- Optimizing routes and managing logistics
- Fraud detection
- Focusing on sustainability and environmental footprint
- Reverse logistics and returns management
- Document management
- Localization strategies
- Chatbot
- Risk management
- Warehouse layout optimization
- Automated cross-docking strategies
- Delivery time predictions
- Shipment tracking
- Streamlining supply chain workflow with GenAI
- How does GenAI in the supply chain work?
- How does LeewayHertz’s generative AI platform optimize logistics operations?
- LeewayHertz’s AI development services for supply chain
- How to implement generative AI solutions in logistics and supply chain operations?
- Benefits of generative AI in logistics and supply chain
- Difference between traditional and generative AI in the supply chain
Generative AI in the supply chain: a brief overview
Generative AI transforms the supply chain by enhancing efficiency and decision-making processes. Through advanced algorithms, it can analyze vast amounts of data to predict demand patterns, optimize inventory management, and streamline logistics. Generative AI models can generate realistic scenarios, helping businesses simulate various situations and devise robust strategies. This technology enables predictive maintenance for machinery, reducing downtime and improving overall operational resilience. Additionally, by automating routine tasks, such as order processing and invoicing, generative AI frees up human resources for more strategic roles. The integration of generative AI in supply chains not only accelerates decision-making but also fosters adaptability in dynamic market conditions, ultimately enhancing the overall agility and competitiveness of businesses.
Use cases of generative AI in supply chain
Demand forecasting and planning
Generative AI in supply chain transforms demand forecasting in supply chain management, enhancing inventory streamlining, reducing product shortages, and elevating customer satisfaction. It applies intricate algorithms to past data, market shifts, and external factors to augment the precision of demand predictions.
The power of generative models lies in their ability to process multiple variables concurrently, unraveling complex patterns and correlations often overlooked by traditional forecasting techniques. This accuracy boosts a business’s ability to anticipate demand changes, optimize production, and adjust inventory levels, driving operational efficiency and financial gains.
The strength of generative AI shines when digesting vast historical sales data, incorporating cyclical changes and marketing drives. As the AI model learns from this rich data, it becomes proficient at generating accurate demand forecasts. The result? Businesses can expertly manage stock levels, allocate resources tactically, and brace for future market trends.
Inventory optimization
Inventory management is a critical balancing act between averting product shortages and mitigating costs associated with excess inventory. Generative AI in supply chain proves pivotal in maintaining this balance, determining optimal inventory levels using analysis of historical data, demand patterns, and external variables. This technology helps businesses to reduce surplus inventory, prevent overstocking, and enhance supply chain responsiveness.
Generative AI models can identify optimal distribution strategies and storage practices considering delivery times, transportation costs, and demand fluctuations. The result is maximized operational efficiency and substantial cost reduction. By proposing to reorder points and safety stock levels, generative AI aids businesses in improving warehouse management, leading to reduced product shortages, minimized surplus inventory, and lower holding costs.
Managing supplier selection and relationships
By analyzing comprehensive data sets, including performance indicators, quality assessments, and cost structures, generative AI in supply chain allows businesses to identify optimal suppliers, thereby enhancing supply chain resilience. Moreover, it aids in proficiently managing supplier relationships by analyzing past interactions, contracts, and performance records. These insights help identify potential risks, improvement areas and propose negotiation strategies, enabling proactive management of supplier-related issues and fostering beneficial collaborations.
Generative AI’s ability to process extensive data like past performance, financial records, and news helps foresee potential disruptions and implement preemptive measures, such as diversifying supplier networks or devising contingency plans. Hence, it helps improve the overall dependability and performance of the supply chain.
Predictive maintenance
Equipment malfunctions and unexpected downtime can significantly disrupt supply chain operations, often leading to considerable financial loss. Generative AI becomes an invaluable asset for implementing predictive maintenance strategies in this context. It achieves this by analyzing information such as sensor readings, historical maintenance documentation, and equipment performance indicators.
Generative AI models can predict when maintenance is needed by identifying data anomalies and patterns. This allows organizations to plan for repairs or replacements proactively, consequently reducing downtime, prolonging equipment life, boosting operational efficiency, and curbing maintenance expenses.
Optimizing routes and managing logistics
Generative AI in supply chain significantly enhances route optimization and logistics management in supply chain operations. It can devise optimal transportation strategies by considering traffic patterns, vehicle capacities, and customer needs, thereby reducing fuel usage and delivery times and increasing customer satisfaction.
Additionally, it can dynamically adapt to unexpected circumstances in real-time, enhancing overall supply chain resilience. Generative AI analyses extensive data from various sources, aiding in route optimization and transportation, resulting in time and cost savings and overall improvements in logistical efficiency. Its capabilities span route enhancement, vehicle and fleet optimization, and dynamic routing, contributing to a more robust and resilient supply chain.
Fraud detection
Generative AI in supply chain significantly elevates fraud detection in supply chain management by analyzing financial data for irregular patterns indicative of fraud. It uses machine learning algorithms, particularly deep learning neural networks, to examine past transactional data, invoice information, shipping details, and more, spotting anomalies that could signal fraud.
Specifically, Generative Adversarial Networks (GANs) are employed, comprising a generator network creating synthetic fraudulent transactions and a discriminator network identifying them. This dual system enhances fraud detection accuracy.
Additionally, these AI models, equipped with predictive capabilities, can forecast potential fraudulent activities using historical data. This helps detect and proactively prevent fraud, bolstering supply chain security and reliability.
Focusing on sustainability and environmental footprint
Generative AI can significantly promote sustainable supply chain management by refining transportation pathways to decrease fuel usage and emissions. Additionally, it can aid in enhancing the use of packaging materials, cutting down waste, and endorsing environmentally conscious practices across the supply chain.
Technically, generative AI works by analyzing vast amounts of transportation, waste management, and resource usage data. It uses machine learning algorithms to identify patterns and predict outcomes that can lead to more sustainable practices.
In transportation, for instance, generative AI in supply chain can analyze traffic data, vehicle capacities, and delivery routes to optimize logistics and minimize environmental impact. Choosing the most fuel-efficient routes and schedules can reduce carbon emissions and contribute to sustainability goals.
Generative AI can analyze product dimensions, fragility, and other factors for packaging optimization to suggest the most efficient and environmentally friendly packaging solutions. This reduces material usage and waste and can lead to cost savings.
Furthermore, generative AI can analyze resource consumption and waste production data across the supply chain. Identifying inefficiencies and suggesting improvements can help companies adopt greener practices and reduce their environmental footprint.
Reverse logistics and returns management
Generative AI in supply chain can streamline the reverse logistics process, which is a crucial aspect of supply chain management, by evaluating data related to returns, repairs, and refurbishments. This AI technology aids in identifying the best routes for returned products, deciding on repair or disposal actions, and optimizing inventory distribution for refurbished items.
For returned goods, AI can evaluate factors like the cost of transportation, the condition of the product, and the demand for refurbished items. This data can predict whether a product should be repaired, refurbished, recycled, or disposed of. This process helps reduce unnecessary costs and waste.
Regarding routing, generative AI can analyze transportation data to determine the most efficient route for returning products. This can minimize transportation costs and time, resulting in a more efficient reverse logistics process.
Furthermore, for inventory management, generative AI can use historical sales data and demand forecasts to optimize the allocation of refurbished items. This can prevent overstocking or stockouts of refurbished goods and help ensure that these items are allocated where they are most likely to sell, improving overall supply chain efficiency.
Document management
Generative AI, particularly utilizing Natural Language Generation (NLG), play a pivotal role in automating the creation of logistics and customs documents within the supply chain. The AI gains a nuanced understanding of the specific language, patterns, and structures inherent in such documents by training on extensive datasets comprising various customs forms, declarations, and regulations.
This technology mimics human writing, allowing it to generate coherent and contextually appropriate content for customs and logistics paperwork. The AI system ensures compliance with the intricate regulations governing these documents, minimizing the risk of errors and misinterpretations.
By automating the document creation process, generative AI significantly reduces the manual effort required, leading to increased efficiency and speed in handling paperwork. Moreover, it contributes to a more reliable and consistent documentation process, reducing the likelihood of errors and ensuring that all generated documents adhere to the necessary legal and regulatory standards. Overall, integrating generative AI in document creation is valuable for enhancing accuracy, efficiency, and compliance in supply chain logistics.
Localization strategies
Generative AI in localization strategies enables businesses to adapt their supply chain operations to diverse markets. By analyzing regional preferences, regulations, and cultural nuances, the technology assists in customizing product assortments, distribution networks, and marketing approaches. This ensures businesses meet specific market demands, comply with local regulations, and resonate with cultural expectations. Through real-time data processing, generative AI allows for agile adjustments, promoting a responsive supply chain that aligns seamlessly with the unique requirements of each region. Ultimately, this personalized approach enhances customer satisfaction, optimizes resource allocation, and fosters sustained success in global markets.
Chatbot
Generative AI-powered chatbots are crucial in optimizing supply chain operations and streamlining supplier communication. These intelligent chatbots excel in managing a spectrum of tasks, such as tracking orders, facilitating real-time updates, and addressing common queries related to inventory, shipments, and delivery schedules.
In supplier communication, these chatbots act as efficient intermediaries, automating routine interactions such as order placements, invoice inquiries, and product availability checks. By swiftly providing accurate information, they enhance the overall efficiency of supplier relationships, reducing response times and fostering better collaboration.
Moreover, generative AI empowers these chatbots to adapt to various languages, regional preferences, and industry-specific terminology, ensuring seamless communication in the diverse landscape of the global supply chain. The technology’s ability to learn from past interactions enables continuous improvement, refining responses based on historical data and feedback. Integrating generative AI-powered chatbots into supply chain operations and supplier communications accelerates information exchange and contributes to increased accuracy, transparency, and collaboration throughout the supply chain network.
Risk management
Generative AI’s role in risk management within the supply chain is pivotal. By analyzing a multitude of variables, including geopolitical events, market trends, and potential natural disasters, generative AI can provide predictive insights into possible disruptions. This foresight empowers businesses to proactively implement contingency plans and mitigate the impact of unforeseen events.
AI’s ability to process vast amounts of real-time data allows for a dynamic assessment of risk factors, enabling quicker response times and strategic decision-making. Generative AI models can identify patterns and correlations that may be challenging for traditional risk management methods to discern, thereby enhancing the precision of risk predictions.
In the face of uncertainties, businesses can leverage generative AI to simulate different scenarios, allowing them to develop robust strategies and contingency plans. This forward-looking approach contributes to an overall improvement in risk resilience, ensuring supply chain continuity and minimizing the potential negative impact of disruptions. Generative AI, therefore, emerges as a valuable tool in fortifying the resilience of supply chain operations against a range of unpredictable risks.
Warehouse layout optimization
Generative AI optimizes warehouse layout optimization by analyzing historical order patterns and adapting storage configurations dynamically. Through this analysis, the AI identifies the most frequently accessed products and strategically places them closer to packing and shipping areas, reducing retrieval times and expediting order fulfillment. By considering factors like product size, weight, and demand frequency, generative AI creates layouts that maximize storage capacity while minimizing travel distances for warehouse staff. This enhances operational efficiency and significantly reduces labor costs associated with order picking. The ability of generative AI to continuously learn and adapt ensures that the warehouse layout remains optimized in response to evolving order patterns, contributing to sustained improvements in speed, accuracy, and overall cost-effectiveness in supply chain operations.
Automated cross-docking strategies
Automated cross-docking strategies, facilitated by AI, streamline supply chain operations by minimizing storage time and enhancing agility. Generative AI analyzes real-time data on incoming shipments and existing inventory to develop optimal cross-docking plans. By identifying products with high demand and prioritizing their quick transfer from inbound to outbound shipments, the AI reduces the need for long-term storage. This minimizes storage costs and accelerates order fulfillment, contributing to improved customer satisfaction. The generated strategies consider factors such as product characteristics, order volume, and destination, ensuring a seamless and efficient cross-docking process. As a result, businesses can adapt swiftly to changing market demands, reduce handling and storage expenses, and foster a more agile and responsive supply chain.
Delivery time predictions
Generative AI transforms delivery time predictions by leveraging historical data and real-time conditions to provide accurate estimates. The AI analyzes past delivery performances, considering variables such as traffic patterns and order complexities. This comprehensive analysis enables precise predictions, enhancing overall customer satisfaction by setting realistic expectations. Businesses can also benefit from improved planning and resource allocation as they gain insights into potential delays or optimal delivery windows. The continuous learning capabilities of generative AI ensure that its predictions adapt to changing conditions, making it a valuable tool for maintaining reliability and efficiency in the delivery process. Ultimately, this use case enhances customer experiences and operational efficiency in the supply chain.
Shipment tracking
Generative AI significantly enhances supply chain and logistics shipment tracking by predicting and optimizing the entire tracking process. By analyzing historical shipment data, real-time location information, and various contextual factors, generative AI can accurately forecast delivery times and potential delays. The technology enables the creation of dynamic tracking systems that provide real-time updates to customers, offering transparency and improving their overall experience. Additionally, generative AI can identify patterns in shipping routes, allowing for predicting potential issues and enabling proactive problem-solving. The continuous learning capabilities of the AI ensure that the tracking system adapts to evolving conditions, optimizing efficiency and minimizing uncertainties in the shipment process. Generative AI transforms shipment tracking, making it more accurate, responsive, and customer-centric in the complex supply chain and logistics landscape.
Streamlining Supply Chain Workflow with GenAI
Generative AI is transforming the supply chain, optimizing every stage from planning to delivery. From intelligent demand forecasting to AI-powered logistics optimization, GenAI is transforming how companies manage and execute their supply chain operations, leading to increased efficiency, resilience, and agility. Here’s a breakdown of how GenAI is making a significant impact across the supply chain lifecycle:
- Planning and design: Demand forecasting
Steps involved | Sub-steps | Role of GenAI |
Capture data |
|
|
Generate forecasts | Apply demand models | Suggest the best performing forecasting models (ARIMA, LSTM, Prophet) for different scenarios. |
Validate forecasts | N/A | Enables backtesting and simulation techniques to validate forecast accuracy and identify biases. |
Planning |
|
|
Procurement execution |
|
|
Evaluation |
|
|
Conclusion |
|
|
- Sourcing and procurement: Supplier management
Steps involved | Sub-steps | Role of GenAI |
Capture information |
|
|
Onboard supplier |
|
|
Evaluate performance |
|
|
Manage contracts |
|
|
Resolve issues |
|
|
- Shipment
Steps involved | Sub-steps | Role of GenAI |
Capture details |
|
|
Order processing |
|
|
Shipment transit |
|
|
Delivery management |
|
|
Return and exchange management |
|
|
Shipment completion |
|
|
Generative AI is transforming the supply chain management lifecycle from planning to delivery. By optimizing forecasting, automating tasks, and providing data-driven insights, GenAI is transforming how companies design, manage, and execute their supply chain operations.
Optimize Your Operations With AI Agents
Optimize your workflows with ZBrain AI agents that automate tasks and empower smarter, data-driven decisions.
How does GenAI in the supply chain work?
Integrating GenAI into supply chain management involves various components to automate data analysis, extract actionable insights, and enable informed decision-making. It surpasses conventional methods by integrating robust Large Language Models (LLMs) with the organization’s unique knowledge base, enhancing the analysis of supply chain operations. This approach empowers organizations to find valuable insights and make informed decisions at every stage of the supply chain lifecycle, thereby maximizing efficiency and effectiveness.
The architecture integrates multiple components to streamline and optimize the supply chain process. Here’s a step-by-step breakdown of how it works:
- Data sources: The initial step involves collecting data pertinent to the supply chain process from diverse sources. This data can include:
- Enterprise Resource Planning (ERP) systems: Data related to various aspects of the supply chain, including inventory levels, procurement, production schedules, and order fulfillment.
- Supplier databases: Information from suppliers such as lead times, pricing, quality metrics, delivery schedules and performance evaluations.
- Inventory management systems: Data on inventory levels, turnover rates, stockouts and storage costs.
- Transportation management systems (TMS): TMS data includes shipping schedules, carrier performance, and transportation costs.
- Warehouse management systems (WMS): WMS data tracks warehouse activities such as receiving, picking, packing, and shipping.
- Point of Sale (POS) systems: POS data provides insights into consumer demand patterns, helping forecast demand and replenish inventory.
- IoT Sensors and Devices: IoT devices collect real-time data on factors like temperature, humidity, and location, enabling better visibility and monitoring of goods throughout the supply chain.
- Customer Relationship Management (CRM) systems: CRM data includes customer orders, preferences, and feedback.
- Data pipeline: The data gathered from the previous sources is subsequently channeled through data pipelines. These pipelines handle tasks such as data ingestion, cleaning, processing (including data transformations like filtering, masking, and aggregations), and structuring, preparing it for subsequent analysis.
- Embedding model: The processed data is segmented into chunks and fed into an embedding model. This model converts textual data into numerical representations called vectors, enabling AI models to comprehend it effectively. Well-known models for this purpose are developed by OpenAI, Google, and Cohere.
- Vector database: The resulting vectors are stored in a vector database, facilitating streamlined querying and retrieval processes. This database efficiently manages the storage, comparison, and retrieval of potentially billions of embeddings (i.e., vectors). Prominent examples of such vector databases include Pinecone, Weaviate, and PGvector.
- APIs and plugins: APIs and plugins such as Serp, Zapier, and Wolfram serve a critical function by linking various components and facilitating additional functionalities, such as accessing supplementary data or executing specific tasks seamlessly.
- Orchestration layer: The orchestrating layer plays a pivotal role in managing the workflow. An example of this layer is ZBrain. ZBrain streamlines tasks like prompt chaining, handling interactions with external APIs to determine when API calls are necessary, fetching contextual data from vector databases, and preserving memory across multiple LLM calls. This layer generates a prompt or series of prompts for submission to a language model for processing. Overall, its responsibility lies in orchestrating data flow and tasks, ensuring smooth coordination among all other components in this architecture.
- Query execution: The data retrieval and generation process begins when the user submits a query to the supply chain management app. This query can pertain to any aspect relevant to the supply chain, covering factors such as inventory levels, supplier performance, demand forecasts and logistics requirements.
- LLM processing: Upon receiving the query, the application forwards it to the orchestration layer. This layer then retrieves pertinent data from the vector database and LLM cache and sends it to the suitable LLM for processing, with the selection of the LLM dependent upon the query’s nature.
- Output: The LLM generates an output based on the query and the data it receives. This output can take various forms relevant to supply chain management, such as supplier performance reports, optimization recommendations for inventory levels, risk assessments related to logistics operations, or summaries of demand forecasts and procurement requirements.
- Supply chain management app: The verified output is then presented to the user via the supply chain management app. This central application consolidates all data, analysis, and insights, presenting findings intuitively. Decision-makers can review and act upon the information provided, facilitating efficient supply chain operations and strategic decision-making.
- Feedback loop: User feedback on the LLM’s output is a crucial component of this architecture. This feedback is utilized to continuously enhance the accuracy and relevance of the LLM’s results, ensuring better performance over time.
- Agent: AI agents play a vital role in this process by solving complex problems, interacting with the external environment, and enhancing learning through post-deployment experiences. They accomplish this by employing advanced reasoning and planning, strategically utilizing tools, memory, recursion, and self-reflection.
- LLM cache: To expedite the AI system’s response time, frequently accessed information is cached using tools like Redis, SQLite, or GPTCache.
- Logging/LLMOps: During this process, LLMOps tools such as Weights & Biases, MLflow, Helicone, and Prompt Layer aid in logging actions and monitoring performance. This ensures optimal functionality of the LLMs and facilitates continuous improvement through feedback loops.
- Validation: A validation layer is utilized to authenticate the LLM’s output, accomplished through tools such as Guardrails, Rebuff, Guidance, and LMQL. This ensures the precision and dependability of the information shared.
- LLM APIs and hosting: LLM APIs and hosting platforms are integral for executing supply chain tasks and hosting the application. Depending on project requirements, developers can choose from LLM APIs offered by companies like OpenAI and Anthropic or explore open-source models. Similarly, they have various hosting platform options, including cloud providers such as AWS, GCP, Azure, and Coreweave, or opinionated clouds like Databricks, Mosaic, and Anyscale. The selection of LLM APIs and cloud hosting platforms depends on the unique needs of the project.
This process offers a comprehensive insight into how GenAI optimizes supply chain management, leveraging diverse data sources and technological tools to generate accurate and actionable insights. Ultimately, by automating various supply chain tasks, generative AI enhances efficiency, mitigates risks, and improves the overall quality of operational decisions, leading to smoother logistics, better inventory management, and enhanced customer satisfaction.
How does LeewayHertz’s generative AI platform optimize logistics operations?
LeewayHertz’s generative AI platform, ZBrain, plays a transformative role in streamlining logistics processes by significantly enhancing supply chain management, operational efficiency, inventory workflows, and overall logistics optimization. As a comprehensive, enterprise-ready platform, ZBrain empowers businesses to design and implement applications tailored to their specific operational requirements. The platform uses clients’ data, whether in the form of text, images, or documents, to train advanced LLMs like GPT-4, Vicuna, Llama 2, or GPT-NeoX for developing contextually aware applications capable of performing diverse tasks.
Enterprises contend with the complexities of navigating logistics hurdles, including inventory management, supply chain visibility, escalating transportation costs, demand forecasting intricacies, technology integration complexities, last-mile delivery challenges, regulatory compliance intricacies, and data accuracy. ZBrain effectively addresses these challenges through its distinctive feature called “Flow,” which provides an intuitive interface that allows users to create intricate business logic for their apps without the need for coding. Flow’s easy-to-use drag-and-drop interface enables the seamless integration of large language models, prompt templates, and other generative AI models into your app’s logic for its easy conceptualization, creation, or modification.
To comprehensively understand how ZBrain Flow works, explore this resource that outlines a range of industry-specific Flow processes. This compilation highlights ZBrain’s adaptability and resilience, showcasing how the platform effectively meets the diverse needs of various industries, ensuring enterprises stay ahead in today’s rapidly evolving business landscape.
ZBrain’s potent applications elevate logistics operations by transforming complex data into actionable insights, resulting in heightened operational efficiency, advanced predictive analytics, and optimized inventory management. ZBrain’s holistic solutions adeptly address key challenges within logistics processes. Here are some noteworthy examples:
AI-driven demand forecasting
ZBrain employs an automated approach to forecasting demand by extracting pertinent data, including sales history, current inventory levels, market trends, and external factors, such as promotions. This comprehensive dataset serves as the basis for precise forecasting. ZBrain conducts Exploratory Data Analysis (EDA) to unveil insights within the data, identifying missing values, outliers, correlations, and patterns influencing demand. Then, ZBrain transforms textual data into numerical representations using advanced embedding techniques, enhancing forecasting accuracy. When a user queries the demand forecasting report, ZBrain fetches relevant data based on query requirements and processes it using the chosen Large Language Model (LLM) for dynamic report generation. The report, affluent in insights, outlines projected demand scenarios, trends, and influencing factors. ZBrain then expertly extracts essential information, presenting the parsed data in a structured, concise, and accurate format for informed decision-making.
ZBrain applications markedly cut down on time and costs, ensuring precision and enabling superior resource allocation, helping logistics managers optimize warehouse operations. Explore this detailed Flow to understand how ZBrain streamlines demand forecasting and warehouse planning, unlocking unparalleled operational efficiency.
AI-driven fleet management
ZBrain streamlines fleet management and performance monitoring through an automated process. It begins by automatically extracting relevant data, including fleet details, operational data, GPS and telematics data, and maintenance records from diverse sources, ensuring a comprehensive overview of fleet management. Following data collection, ZBrain employs an automated Exploratory Data Analysis (EDA) to find insightful correlations, trends, and outliers within the datasets, providing a deeper understanding of informed fleet management decisions. Utilizing advanced embedding techniques, ZBrain transforms textual data into numerical representations, enhancing analysis efficiency and delivering precise insights for fleet management. When a user queries the fleet management report, ZBrain fetches relevant data based on query requirements and utilizes the chosen Large Language Model (LLM) to generate a comprehensive report. ZBrain parses the report post-generation, extracting critical details. This structured data ensures a coherent and valuable fleet management insight, facilitating strategic and well-informed decisions.
ZBrain applications deliver significant time and cost savings, optimizing fleet management for improved resource allocation and enhanced service levels. Dive into this comprehensive Flow to grasp how ZBrain facilitates seamless fleet management and performance monitoring, ultimately optimizing operational outcomes.
AI-driven route optimization
ZBrain efficiently streamlines delivery routes through an automated process. It commences by automatically gathering essential data for route optimization, including delivery points, distances, traffic conditions, time windows, vehicle capacities, and road restrictions. This comprehensive dataset forms the base for refining delivery routes. Post-data acquisition, ZBrain engages in Exploratory Data Analysis (EDA) to reveal useful insights, identifying patterns and correlations that enhance route efficiency. Advanced embedding techniques are then applied to transform textual data into numerical representations, capturing semantic relationships for efficient analysis and precise route optimization. When a user queries route optimization, ZBrain fetches relevant data and query details, forwarding them to the chosen Large Language Model (LLM) for dynamic report generation. The extracted information undergoes meticulous structuring to ensure precision. ZBrain then compiles all insights into a cohesive final report, showcasing optimized delivery routes, distance comparisons, time reductions, and potential benefits. This consolidated report empowers businesses to implement highly effective route planning strategies.
ZBrain applications elevate delivery efficiency, reducing time and driving cost reductions while enhancing service quality. Explore this detailed Flow to comprehend how ZBrain optimizes route planning, contributing to improving logistics operations.
Optimize Your Operations With AI Agents
Optimize your workflows with ZBrain AI agents that automate tasks and empower smarter, data-driven decisions.
LeewayHertz’s AI development services for supply chain
At LeewayHertz, we develop tailored AI solutions that cater to the unique requirements of supply chain management. We provide strategic AI/ML consulting that enables companies to harness AI for enhanced decision-making, improved supplier relationships, and optimized inventory management strategies.
Our expertise in developing Proof of Concepts (PoCs) and Minimum Viable Products (MVPs) allows companies to preview the potential impacts of AI tools in real scenarios, ensuring that the solutions are effective and tailored to the supply chain sector’s specific needs.
Our work in generative AI also transforms routine tasks like demand forecasting, report generation and data management, automating these processes to free supply chain professionals for more strategic roles.
By fine-tuning large language models to the nuances of supply chain terminology and vendor interactions, LeewayHertz enhances the accuracy and relevance of AI-driven communications and analyses.
Additionally, we ensure these AI systems integrate seamlessly with existing technological infrastructures, enhancing operational efficiency and decision-making in supply chain management.
Our AI solutions development expertise
AI solutions development for supply chain management typically involves creating systems that enhance decision-making, automate routine tasks, and optimize logistics processes. These solutions integrate key components such as data aggregation technologies, which compile and analyze supply chain information from diverse sources. This comprehensive data foundation supports predictive analytics capabilities, allowing for forecasting demand trends and supply chain disruptions that inform strategic decisions. Additionally, machine learning algorithms are employed to optimize inventory levels, transportation routes, and supplier selection, ensuring that each company’s unique operational goals and risk tolerance are considered. These solutions often cover areas like demand planning, warehouse management, transportation optimization, and supplier relationship management.
Overall, AI solutions in supply chain management aim to optimize operational efficiency, improve resilience, and elevate the customer experience by ensuring timely and cost-effective delivery of goods.
AI agent/copilot development for supply chain
LeewayHertz builds custom AI agents and copilots that enhance various supply chain management operations, enabling companies to save time and resources while facilitating faster decision-making. Here is how they help:
- Demand forecasting & planning:
- Analyze historical data and market trends to predict future demand accurately. This enables better inventory planning and reduces stockouts and overstocking.
- Adjust prices in real-time based on demand fluctuations, competitor pricing, and other factors, optimizing revenue and maximizing profit.
- Inventory management & optimization:
- Analyze data on inventory levels, demand, and lead times to identify optimal inventory levels and reorder points. This minimizes holding costs and stockouts.
- Transportation & logistics:
- Calculate the most efficient routes for trucks, minimizing delivery time and fuel consumption.
- Provide real-time visibility of shipments, enabling better route adjustments and proactive issue resolution.
- Predict potential equipment failures based on sensor data, allowing for preventive maintenance and reducing downtime.
- Adjust delivery routes in real-time based on unforeseen events like accidents or road closures, ensuring timely deliveries.
- Assign deliveries to available vehicles based on capacity, location, and delivery time windows, improving efficiency and minimizing delays.
- Supply chain risk management:
- Monitor data streams for potential disruptions (natural disasters, political instability, etc.) and issue early warnings to mitigate risks.
- Identify alternative suppliers and routes, enabling businesses to adapt to unforeseen disruptions and maintain operations.
- Analyze images and videos of products to identify defects, ensuring quality control and reducing product recalls.
- Monitor transactions and data patterns to identify potential fraud or anomalies, mitigating financial risks and protecting the supply chain.
- Customer service & communication:
- Answer customer inquiries about orders, delivery, and other supply chain aspects, providing 24/7 support.
- Analyze customer data and preferences to personalize communication and provide relevant information.
- Send automated notifications, updates, and alerts to stakeholders, improving communication and transparency within the supply chain.
- Procurement & sourcing:
- Analyze supplier data (reliability, pricing, quality) to identify the most suitable suppliers for specific needs.
- Assist in automating contract negotiation, ensuring optimal terms and conditions.
- Identify and procure materials and services based on pre-defined criteria, automating the sourcing process and reducing procurement costs.
- Data analysis & decision support:
- Analyze vast amounts of data and generate insightful reports, clearly understanding supply chain performance and identifying areas for improvement.
- Analyze sensor data from machinery and equipment to predict potential failures, allowing for preventive maintenance and minimizing downtime.
- Simulate different scenarios and predict potential outcomes, enabling better decision-making and preparation for uncertainties.
AI agents and copilots increase operational process efficiency and significantly enhance customer service and strategic decision-making. By integrating LeewayHertz’s advanced AI agents into their existing infrastructure, supply chain management firms can achieve a significant competitive advantage, navigating the complex logistical landscape with innovative, efficient, and reliable AI-driven tools and strategies.
How to implement generative AI solutions in logistics and supply chain operations?
Implementing generative AI solutions in logistics and supply chain operations involves a strategic and systematic approach. Here are key steps to consider:
- Identify use cases: Clearly define the use cases where generative AI can be valuable, such as demand forecasting, route optimization, or automated document generation. Focus on areas that align with business goals and operational challenges.
- Data collection and preparation: Gather relevant and diverse datasets for training the generative AI model. This should include historical data, real-time information, and other pertinent data sources. Ensure data quality, cleanliness, and proper anonymization if necessary.
- Choose the right generative AI model: Choose the suitable generative AI model based on the identified use cases. Options such as GPT-3, GPT-4, or other specialized models should be considered, taking into account the complexity and specific requirements of the tasks at hand.
- Train the model: Train the chosen generative AI model using the prepared datasets. Fine-tune the model to ensure it understands the specific language, patterns, and contexts relevant to the logistics and supply chain domain.
- Pilot testing: Conduct pilot tests of the generative AI solution in a controlled environment. Collect input from users and stakeholders to pinpoint areas for enhancement, validating the model’s accuracy and efficacy. This iterative feedback process ensures continuous improvement and aligns the model with evolving requirements and expectations.
- Scale and monitor: Once the pilot phase proves successful, scale the generative AI solution across broader operations. Implement robust monitoring systems to track the model’s performance, promptly addressing issues and ensuring ongoing optimization.
- Integration with existing systems: Integrate the generative AI solution with logistics and supply chain systems. Ensure compatibility and seamless communication with other software and data sources to facilitate real-time decision-making.
- Security and compliance: Address security concerns related to sensitive supply chain data. Incorporate security measures, including encryption and access controls, to safeguard against potential threats. These protective measures are essential to fortify the system and ensure the confidentiality and integrity of sensitive data. Ensure compliance with relevant regulations, especially in industries with strict data privacy requirements.
- Continuous Improvement: Foster a culture of continuous improvement by regularly updating and retraining the generative AI model. Stay attuned to changes in the supply chain landscape, technology advancements, and evolving business requirements.
LeewayHertz, a pioneering generative AI development company, excels in crafting intelligent solutions for logistics, transforming supply chain operations with cutting-edge technology. Leveraging expertise in models like GPT, LeewayHertz delivers innovative, tailored solutions for businesses seeking to optimize processes, enhance decision-making, and transform their approach to supply chain management.
Benefits of generative AI in logistics and supply chain
- Improved efficiency: Generative AI streamlines logistics operations by automating repetitive tasks, reducing manual effort, and enhancing overall process efficiency. It optimizes routes, minimizes delays, and accelerates the movement of goods, contributing to a more agile and cost-effective supply chain.
- Enhanced decision-making: Generative AI empowers businesses by analyzing extensive datasets, facilitating data-driven decision-making in real time. This capability allows organizations to derive valuable insights from diverse sources, enabling timely and informed strategic choices. It provides valuable insights into inventory levels, market trends, and demand fluctuations, allowing for more accurate and strategic decision-making in supply chain management.
- Real-time insights: Generative AI offers real-time monitoring and analysis of key performance indicators, enabling quick responses to change conditions. This leads to improved adaptability, reduced lead times, and a more responsive supply chain that can swiftly address fluctuations in demand or unexpected disruptions.
- Cost reduction: Through automation of various logistics processes, predictive maintenance to minimize downtime, and optimized resource allocation, generative AI contributes to cost reduction in inventory management, transportation, and overall logistics operations, thereby improving the bottom line.
- Optimized resource allocation: Generative AI analyzes data on inventory levels, order patterns, and transportation routes to optimize resource allocation. This ensures that resources such as workforce, warehouse space, and transportation capacity are utilized efficiently, reducing unnecessary costs and improving overall resource management.
- Enhanced customer satisfaction: Generative AI facilitates accurate delivery time predictions, real-time tracking, and improved communication throughout the shipping process. This increases customer satisfaction as consumers receive timely and transparent information about their orders, fostering trust and loyalty.
- Customized strategies: Generative AI tailors supply chain strategies based on regional preferences, cultural factors, and market dynamics. This customization ensures businesses can adapt their approaches to different markets, fostering more effective and targeted operations.
- Dynamic adaptability: Generative AI’s ability to continuously learn and adapt to new data ensures that supply chain strategies remain relevant and effective. This dynamic adaptability allows businesses to stay ahead of changing market conditions, regulations, and customer preferences, maintaining a competitive edge.
- Streamlined communication: AI-powered chatbots facilitate seamless communication within the supply chain. They enhance customer service by providing quick responses, real-time updates, and efficient query resolution, contributing to improved communication efficiency and a more responsive overall supply chain.
Difference between traditional and generative AI in the supply chain
Aspect | Traditional Supply Chain Operations | Generative AI in Supply Chain |
Demand forecasting |
Relies on historical data and basic models for forecasting. |
Utilizes advanced algorithms and real-time data analysis for accurate demand predictions. |
Inventory management |
Often relies on manual processes and periodic reviews. |
Automates inventory tracking, optimizes levels based on predictive analytics, and minimizes stockouts or overstock. |
Route optimization |
Routes are planned based on general knowledge or data collected by designated authority. |
Dynamically optimizes routes in real-time, considering current conditions like traffic and unexpected disruptions. |
Document generation |
Manual creation of logistics and customs documents, prone to errors. |
Automates document generation through Natural Language Generation (NLG), ensuring accuracy and compliance. |
Decision-making |
Primarily human-driven decision-making based on experience and intuition. |
Augments human decision-making with data-driven insights, enabling more informed and strategic choices. |
Customer communication |
Relies on traditional communication channels with limited real-time updates. |
Utilizes AI-powered chatbots for instant and personalized communication, offering real-time tracking and updates to customers. |
Risk management |
Reactive approach to handling risks, often relying on crisis management. |
Proactively identifies and assesses risks through continuous analysis, enabling strategic and timely risk mitigation. |
Resource allocation |
Manual resource allocation based on historical patterns and periodic assessments. |
Optimizes resource allocation dynamically, considering real-time data, improving efficiency, and reducing unnecessary costs. |
Adaptability to change |
Limited adaptability to rapid changes in market conditions or disruptions. |
Highly adaptable to dynamic market conditions, continuously learning and adjusting strategies for better responsiveness. |
Efficiency |
May suffer from inefficiencies due to manual processes and lack of real-time insights. |
Improves overall efficiency through automation, optimization, and data-driven decision-making, leading to streamlined operations. |
Endnote
Incorporating generative AI promises to be a game-changer for supply chain management, propelling it into an era of unprecedented innovation. By harnessing the power of generative models, businesses can evaluate various scenarios, model diverse strategies, and fine-tune their decision-making mechanisms. For instance, generative AI could be the key to architecting highly streamlined warehouse layouts, fine-tuning production lines, or formulating creative packaging approaches. Through constant trial and innovation, generative AI equips businesses with the tools to discover novel efficiencies, pinpoint opportunities, and promote ongoing refinement within their supply chains.
As technology continues to advance, the integration and adoption of generative AI will have a significant role in shaping the future of supply chain management, transforming how supply chains are managed, leading to improved competitiveness and sustained growth for businesses.
Unlock superior supply chain efficiency with generative AI solutions. Contact LeewayHertz’s AI experts to transform your operations and drive unparalleled business growth.
Listen to the article
Start a conversation by filling the form
All information will be kept confidential.
FAQs
How does generative AI contribute to supply chain optimization?
Generative AI enhances supply chain optimization by creating synthetic data for simulation, improving demand forecasting accuracy, and providing innovative solutions for complex logistics challenges.
What distinguishes generative AI from traditional AI in supply chain applications?
Generative AI stands out from traditional AI by its ability to create new content, including data and solutions, rather than relying solely on existing patterns. It introduces creativity and innovation into supply chain processes, offering unique solutions to complex challenges.
What are the key use cases of generative AI in supply chain management?
Generative AI in supply chain management offers a paradigm shift by introducing creativity and innovation into traditional processes. Its diverse applications include enhancing demand forecasting and strategic planning, optimizing inventory levels, facilitating supplier selection and relationship management, predicting equipment maintenance needs, optimizing logistics routes, contributing to fraud detection, managing risks, fostering product design innovation, focusing on sustainability and environmental considerations, streamlining reverse logistics and returns management, optimizing financial strategies, and automating the creation of logistics and customs paperwork.
Can generative AI be customized to meet the unique needs of different supply chain models?
Yes, generative AI is highly customizable and adaptable to diverse supply chain models. It can be tailored to address distinct challenges and requirements, ensuring a seamless integration into different supply chain frameworks.
How does generative AI contribute to the overall efficiency of supply chain operations?
Generative AI improves overall supply chain efficiency by optimizing processes, reducing risks, and fostering innovation. It enhances decision-making, minimizes disruptions, and contributes to a more agile and responsive supply chain.
Can generative AI be integrated into existing supply chain systems?
Yes, generative AI is designed for seamless integration into existing supply chain systems. It can work harmoniously with current technologies, enhancing capabilities without disrupting established workflows.
How does generative AI contribute to the overall efficiency of supply chain operations?
Generative AI improves overall supply chain efficiency by optimizing processes, reducing risks, and fostering innovation. It enhances decision-making, minimizes disruptions, and contributes to a more agile and responsive supply chain.
In which areas of the supply chain can LeewayHertz's generative AI solutions bring the most impact?
LeewayHertz’s generative AI solutions can bring significant impact in various supply chain areas, including demand forecasting, inventory management, risk assessment, and route optimization, contributing to enhanced efficiency and innovation.
How can LeewayHertz's generative AI solutions assist in mitigating risks within my supply chain operations?
LeewayHertz’s generative AI evaluates potential risks by creating simulated scenarios, allowing for proactive risk management strategies. Our solutions contribute to identifying vulnerabilities, optimizing contingency plans, and enhancing overall supply chain resilience.
How do generative AI solutions by LeewayHertz contribute to sustainable practices in the supply chain?
LeewayHertz’s generative AI solutions focus on optimizing route planning, reducing environmental impact, and promoting sustainable practices in the supply chain. We prioritize solutions that align with green and sustainable initiatives.
Can LeewayHertz's generative AI solutions be scaled to accommodate the growth of my business's supply chain operations?
Certainly, LeewayHertz designs scalable generative AI solutions, ensuring they evolve with the growth of your supply chain operations. Our solutions are adaptable and capable of handling increased workloads seamlessly.
How does LeewayHertz ensure the security of sensitive data within generative AI applications for supply chains?
Security is a top priority for LeewayHertz. We implement robust encryption access controls and adhere to data protection regulations to safeguard sensitive data within generative AI applications for supply chains.
- Generative AI in the supply chain: a brief overview
- Use cases of generative AI in supply chain
- Streamlining supply chain workflow with GenAI
- How does GenAI in the supply chain work?
- How does LeewayHertz’s generative AI platform optimize logistics operations?
- LeewayHertz's AI development services for supply chain
- How to implement generative AI solutions in logistics and supply chain operations?
- Benefits of generative AI in logistics and supply chain
- Difference between traditional and generative AI in the supply chain
- Contact us