Generative AI in insurance: Use cases, solutions, development and implementation
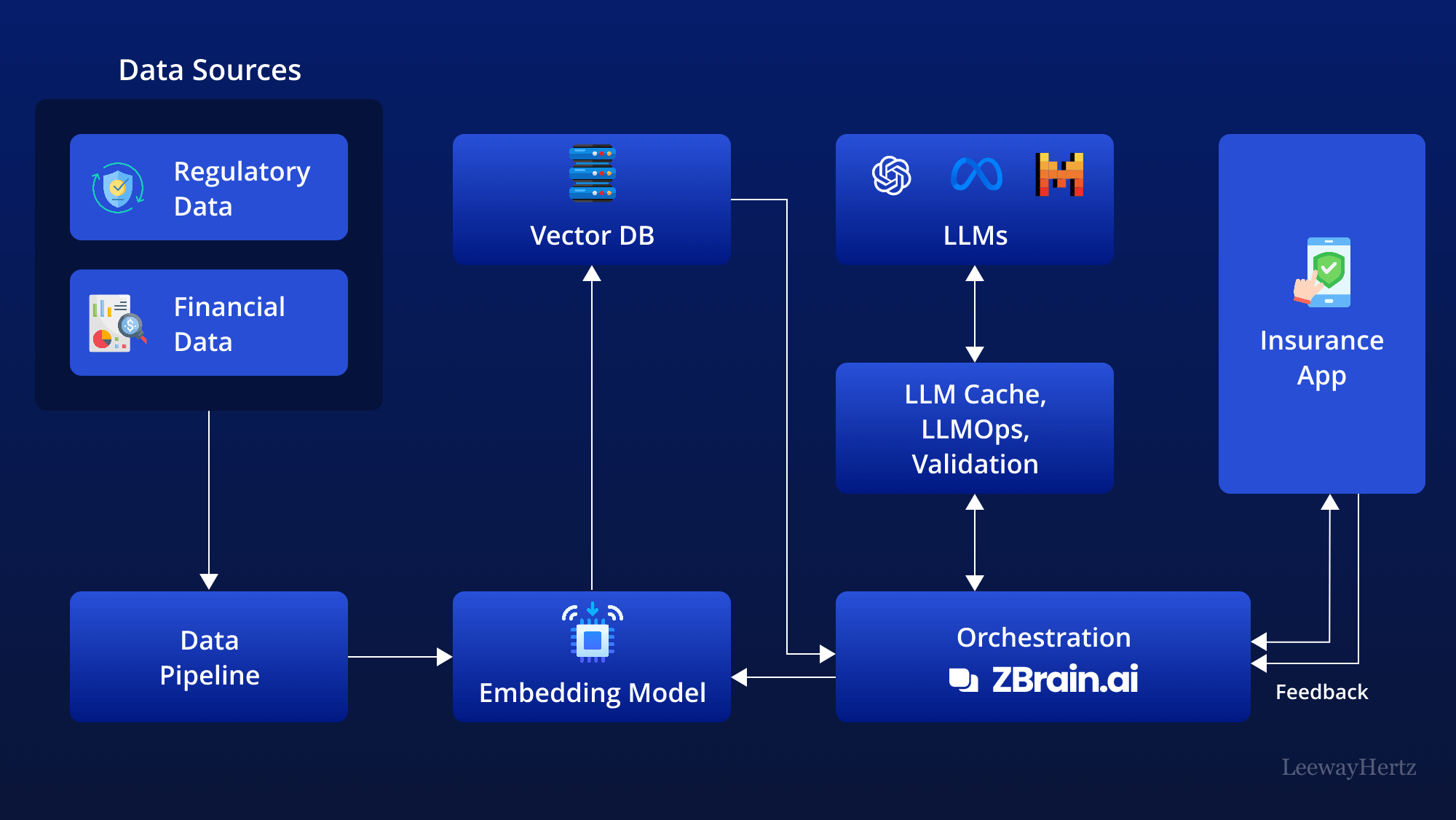
Listen to the article
In the rapidly evolving landscape of the insurance industry, where data-driven decisions and personalized experiences reign supreme, generative AI, a subset of Artificial Intelligence (AI), emerges as a formidable catalyst for innovation. Given this dynamic setting, insurance providers must devise innovative solutions to fulfill customer demands and enhance operational efficiency. Generative AI, with its distinct capabilities, is actively influencing the insurance sector, reshaping traditional practices and redefining how insurers conduct their operations.
Generative AI empowers insurers to harness the power of advanced ML models, facilitating the creation of personalized recommendations and customized products for customers as well as the precise determination of individualized pricing while maintaining high levels of customer satisfaction. This data-driven approach not only enhances insurers’ decision-making capabilities but also paves the way for a faster and more seamless digital buying experience for policyholders.
Further, the success of an insurance business heavily relies on its operational efficiency, and generative AI plays a central role in helping insurers achieve this goal. Through AI-enabled task automation, they can achieve significant improvements in their operational efficiency, enable insurers to respond faster, reduce manual interventions, and deliver superior customer experiences.
Generative AI’s ability to generate fresh and synthetic data is another game-changer. This unique capability empowers insurers to make faster and more informed decisions, leading to better risk assessments, more accurate underwriting, and streamlined claims processing. With generative AI, insurers can stay ahead of the curve, adapting rapidly to the ever-evolving insurance landscape.
This article delves into the realm of generative AI within the insurance industry. It provides an insightful overview of the distinctions between traditional and generative AI in insurance operations, highlighting their unique contributions. The narrative extends to explore various use cases, benefits, and key steps in implementing generative AI, emphasizing the role of LeewayHertz’s platform in elevating insurance operations. Additionally, the article sheds light on the types of generative AI models applied in the insurance sector and concludes with a glimpse into the future trends shaping the landscape of generative AI in insurance.
- Generative AI in insurance: An overview
- Comparing traditional and generative AI in insurance operations: What sets them apart?
- How does generative AI in insurance work?
- Generative AI use cases in the insurance industry
- Personalized insurance policies
- Automated underwriting
- Claims processing automation
- Fraud detection and prevention
- Virtual assistants and customer support
- Risk modeling and predictive analytics
- Product development and innovation
- Anomaly detection
- Image and video analysis
- Customer profiling
- Redefining product innovation
- Cyber insurance
- Reinsurance optimization
- Customer behavior analysis
- Regulatory compliance
- AI-powered solutions: Transforming the insurance workflow by addressing key challenges at every stage
- Streamlining insurance workflow with generative AI
- How LeewayHertz’s generative AI platform enhances insurance operations
- LeewayHertz’s AI development services for insurance
- Implementing generative AI in insurance: Key steps
- The benefits of generative AI in insurance
- Types of generative AI models used in the insurance sector
- The future of generative AI in insurance
Generative AI in insurance: An overview
Generative AI is the subset of AI technology that enables machines to generate new content, data, or information similar to that produced by humans. Unlike traditional AI systems that rely on pre-defined rules and patterns, generative AI leverages advanced algorithms and deep learning models to create original and dynamic outputs. In the insurance industry context, generative AI plays a crucial role in redefining various aspects, from customer interactions to risk assessment and fraud detection. Generative AI introduces a new paradigm in the insurance landscape, offering unparalleled opportunities for innovation and growth. The ability of generative AI to create original content and derive insights from data opens doors to novel applications pertinent to this industry. It facilitates predictive modeling, enabling the creation of risk scenarios that empower insurers to formulate preemptive strategies for proactive risk management. Additionally, generative AI’s capability to create personalized content enables insurers to offer tailor-made insurance policies and experiences, fostering stronger relationships with customers.
Growth projections for generative AI in insurance
The global market size for generative AI in the insurance sector is set for remarkable expansion, with projections showing growth from USD 346.3 million in 2022 to a substantial USD 5,543.1 million by 2032. This substantial increase reflects a robust growth rate of 32.9% from 2023 to 2032, as reported by Market.Biz.
The factors driving this growth are:
- Automation and efficiency: Generative AI’s ability to automate insurance tasks leads to heightened operational efficiency. It streamlines operations and enhances overall effectiveness by accelerating processes and reducing human errors.
- Rising complexity and data volume: The insurance industry deals with an escalating volume of data. Generative AI helps handle this surge and manage complex datasets, extracting valuable insights. This capacity to utilize vast data sets is a major driving force behind generative AI’s growth in the insurance market.
Comparing traditional and generative AI in insurance operations: What sets them apart?
Generative AI and traditional AI are distinct approaches to artificial intelligence, each with unique capabilities and applications in the insurance sector. Understanding how generative AI differs from traditional AI is essential for insurers to harness the full potential of these technologies and make informed decisions about their implementation.
Traditional AI, also known as rule-based AI or narrow AI, relies on predefined rules and patterns to perform specific tasks. It follows a deterministic approach, where the output is directly derived from the input and predefined algorithms. In contrast, generative AI operates through deep learning models and advanced algorithms, allowing it to generate new content and data. Unlike traditional AI, generative AI is not bound by fixed rules and can create original and dynamic outputs.
When it comes to data and training, traditional AI algorithms require labeled data for training and rely heavily on human-crafted features. The performance of traditional AI models is limited to the quality and quantity of the labeled data available during training. On the other hand, generative AI models, such as Generative Adversarial Networks (GANs) and Variational Autoencoders (VAEs), can generate new data without direct supervision. They learn from unlabelled data and can produce meaningful outputs that go beyond the training data.
Traditional AI is widely used in the insurance sector for specific tasks like data analysis, risk scoring, and fraud detection. It can provide valuable insights and automate routine processes, improving operational efficiency. On the other hand, generative AI opens up new possibilities. It can create synthetic data for training, augmenting limited datasets, and enhancing the performance of AI models. Generative AI can also generate personalized insurance policies, simulate risk scenarios, and assist in predictive modeling.
Traditional AI models excel at analyzing structured data and detecting known patterns of fraudulent activities based on predefined rules regarding risk assessment and fraud detection. In contrast, generative AI can enhance risk assessment by generating diverse risk scenarios and detecting novel patterns of fraud that may not be explicitly defined in traditional rule-based systems. Furthermore, generative AI enables insurers to offer truly personalized insurance policies, customizing coverage, pricing, and terms based on individual customer profiles and preferences. While traditional AI can support personalized recommendations based on historical data, it may be limited in creating highly individualized content.
However, generative AI, being more complex and capable of generating new content, raises challenges related to ethical use, fairness, and bias, requiring greater attention to ensure responsible implementation. Traditional AI systems are more transparent and easier to explain, which can be crucial for regulatory compliance and ethical considerations.
How does generative AI in insurance work?
Integrating generative AI into insurance processes entails leveraging multiple components to streamline data analysis, derive insights, and facilitate decision-making. This transcends conventional methods by harnessing robust Large Language Models (LLMs) and integrating them with the insurance company’s distinct knowledge repository. This architecture opens up a new frontier of insight generation, empowering insurance enterprises to make real-time, data-informed decisions.
The architecture leverages various components to streamline the insurance process. Here’s a step-by-step breakdown of how it works:
- Data sources: The process begins by gathering data from various sources relevant to the insurance process. This data can include:
- Policyholder information: Data collected from policyholders, including personal details, coverage history, claims history, and demographic information.
- Claims data: Information related to insurance claims, including claim type, date of claim, claim amount, claimant information, and claim status.
- Underwriting data: Data used to assess risk during the underwriting process, such as medical history, occupation, lifestyle factors, and property details.
- Financial data: Financial information related to policy premiums, investment portfolios, claim payouts, loan underwriting statistics, and customer payment history
- Regulatory data: Data related to regulatory compliance, including legal requirements, licensing information, and industry regulations.
- Data pipelines: Data from the sources above undergo processing via data pipelines. These pipelines handle data ingestion, cleaning, and structuring tasks, preparing it for subsequent analysis.
- Embedding model: The prepared data is subsequently fed into an embedding model, which converts textual data into numerical representations called vectors comprehensible to AI models. Prominent models include those developed by OpenAI, Google, and Cohere.
- Vector database: The resulting vectors are stored in a vector database, facilitating efficient querying and retrieval. Prominent examples of vector databases include Pinecone, Weaviate, and PGvector.
- APIs and plugins: APIs and plugins such as Serp, Zapier, and Wolfram are pivotal in bridging various components and facilitating additional functionalities. These tools enable seamless access to extra data and streamline specific tasks, enhancing overall efficiency.
- Orchestration layer: The orchestrating layer is crucial in handling the workflow. An example of this layer is ZBrain, which streamlines prompt chaining, coordinates interactions with external APIs by determining when API calls are necessary, retrieves contextual data from vector databases, and preserves memory across multiple LLM calls. Ultimately, this layer crafts a prompt or series of prompts for submission to a language model for processing. Its primary function is orchestrating data flow and tasks, ensuring smooth coordination among all components of the AI-driven insurance architecture.
- Query execution: The data retrieval and generation process commences when the user submits a query to the insurance app. This query may pertain to various aspects, such as policyholder trends and risk assessments, information about potential fraud or claim anomalies, and details of policy performance and underwriting results.
- LLM processing: The insurance app forwards the query to the orchestration layer upon receipt. This layer then retrieves pertinent data from the vector database and LLM cache, directing it to the suitable LLM for processing based on the query’s nature.
- Output: The LLM generates output based on the query and received data. This output can take various forms, including summaries of policyholder trends and risk assessments, identification of potential fraud or claim anomalies, or draft reports on policy performance and underwriting results.
- Insurance app: The validated output is subsequently showcased to the user via the insurance app. This is the central application where all data, analyses, and insights converge. It presents the findings in a user-friendly format tailored for decision-makers.
- Feedback loop: User feedback on the LLM’s output constitutes another vital aspect of this architecture. This feedback loop is instrumental in enhancing the accuracy and relevance of the LLM’s output progressively over time.
- Agent: AI agents are critical in tackling complex problems, engaging with the external environment, and enhancing learning through post-deployment experiences. They achieve this through advanced reasoning and planning, strategic tool utilization, and leveraging memory, recursion, and self-reflection mechanisms.
- LLM cache: Tools such as Redis, SQLite, or GPTCache are utilized to cache frequently accessed information, thereby accelerating the response time of the AI system.
- Logging/LLMOps: Throughout this process, LLMOps tools like Weights & Biases, MLflow, Helicone, and Prompt Layer aid in logging actions and monitoring performance. This guarantees that the LLMs function optimally and continuously improve through feedback loops.
- Validation: A validation layer is incorporated to validate the LLM’s output. This is achieved through tools such as Guardrails, Rebuff, Guidance, and LMQL, ensuring the accuracy and reliability of the information provided by the LLM.
- LLM APIs and hosting: LLM APIs and hosting platforms are vital for executing insurance tasks and hosting applications. Developers can choose from LLM APIs, such as those offered by OpenAI and Anthropic, or opt for open-source models based on their requirements. Similarly, they can select hosting platforms from cloud providers like AWS, GCP, Azure, and Coreweave or opt for opinionated clouds like Databricks, Mosaic, and Anyscale. The choice of LLM APIs and cloud hosting platforms depends on the project’s specific needs and preferences.
This structured flow offers a comprehensive overview of how AI facilitates insurance processes, utilizing diverse data sources and technological tools to generate precise and actionable insights.
Optimize Your Operations With AI Agents
Optimize your workflows with ZBrain AI agents that automate tasks and empower smarter, data-driven decisions.
Generative AI use cases in the insurance industry
Generative AI has a wide range of use cases in the insurance industry, offering innovative solutions to longstanding challenges and driving disruptive changes. Here are some notable generative AI use cases in the insurance industry:
Personalized insurance policies
Generative AI enables insurers to create personalized insurance policies tailored to individual customers’ needs and risk profiles. By analyzing vast datasets and customer information, AI algorithms generate customized coverage options, pricing, and terms, enhancing the overall customer experience and satisfaction. For instance, an auto insurer can utilize generative AI to analyze a customer’s driving history, vehicle details, and personal characteristics to offer a customized car insurance policy that aligns with the individual’s specific requirements.
Automated underwriting
Generative AI streamlines the underwriting process by automating risk assessment and decision-making. AI models can analyze historical data, identify patterns, and predict risks, enabling insurers to make more accurate and efficient underwriting decisions. For instance, a life insurance company can use generative AI algorithms to analyze medical records, lifestyle data, and family history to make efficient underwriting decisions, enabling faster policy approvals and enhancing customer experiences.
Claims processing automation
Generative AI automates claims processing by extracting and validating data from claim documents, reducing manual efforts and processing time. Automated claims processing ensures faster and more accurate claim settlements, improving customer satisfaction and operational efficiency. For example, property insurers can utilize generative AI to automatically process claims for damages caused by natural disasters, automating the assessment and settlement for affected policyholders.
Fraud detection and prevention
Generative AI helps combat insurance fraud by analyzing vast amounts of data and detecting patterns indicative of fraudulent behavior. AI-powered algorithms can identify suspicious claims in real-time, enabling insurers to take proactive measures to prevent fraud and reduce financial losses. For instance, health insurers can identify anomalies in medical billing data, uncovering potential fraudulent claims and saving costs.
Virtual assistants and customer support
Generative AI-powered virtual assistants provide real-time support to customers, addressing policy inquiries, claims status updates, and general insurance-related questions. Virtual assistants enhance customer interactions and reduce the burden on customer support teams. For example, a property insurance company can implement a virtual assistant on their website, guiding customers through the claims process and providing helpful information.
Risk modeling and predictive analytics
Generative AI models can simulate various risk scenarios and predict potential future risks, helping insurers optimize risk management strategies and make informed decisions. Predictive analytics powered by generative AI provides valuable insights into emerging risks and market trends. For instance, a property and casualty insurer can use generative AI to forecast weather-related risks in different regions, enabling proactive measures to minimize losses.
Product development and innovation
Generative AI facilitates product development and innovation by generating new ideas and identifying gaps in the insurance market. AI-driven insights help insurers design new insurance products that cater to changing customer requirements and preferences. For example, a travel insurance company can utilize generative AI to analyze travel trends and customer preferences, leading to the creation of tailored insurance plans for specific travel destinations.
Anomaly detection
Generative AI’s anomaly detection capabilities allow insurers to identify irregular patterns in data, such as unusual customer behavior or suspicious claims. Early detection of anomalies helps mitigate risks and ensures more accurate decision-making. For example, an auto insurer can use generative AI to detect unusual claims patterns, such as a sudden surge in accident claims in a specific region, leading to the identification of potential fraud or emerging risks.
Image and video analysis
Generative AI can analyze images and videos to assess damages in insurance claims, such as vehicle accidents or property damage. This visual analysis aids in faster claims processing and accurate assessment of losses. For example, a car insurance company can use image analysis to estimate repair costs after a car accident, facilitating quicker and more accurate claims settlements for policyholders.
Customer profiling
Generative AI plays a crucial role in the realm of insurance by facilitating the creation of synthetic customer profiles. This innovative approach proves instrumental in refining models dedicated to customer segmentation, predicting behavior, and implementing personalized marketing strategies. The use of generative AI in this context prioritizes privacy norms, allowing organizations to bolster their analytical capabilities while safeguarding individual customer data confidentiality. This ensures a harmonious equilibrium between technological innovation and adherence to stringent privacy compliance, enabling insurers to derive actionable insights and enhance customer engagement without compromising sensitive information.
Redefining product innovation
Generative AI emerges as a transformative force, particularly in automated product design within the insurance industry. By meticulously analyzing market trends, customer preferences, and regulatory requirements, this technology facilitates the efficient and informed generation of novel insurance products. Furthermore, generative AI empowers insurers to go beyond conventional offerings by creating highly customized policies. This tailored approach ensures that insurance products align seamlessly with individual customer needs and preferences, marking a significant leap forward in the industry’s ability to meet diverse and evolving consumer demands.
Cyber insurance
Generative models play a pivotal role in improving risk management strategies. Employing threat simulation capabilities, these models enable insurers to simulate various cyber threats and vulnerabilities. This simulation serves as a valuable tool for understanding and assessing the complex landscape of cybersecurity risks, allowing insurers to make informed underwriting decisions. Furthermore, generative AI contributes to policy customization by tailoring cybersecurity insurance offerings to address the unique risks faced by individual clients. This personalized approach ensures that insurance coverage aligns closely with the specific cybersecurity challenges and needs of each client, ultimately bolstering the effectiveness of cybersecurity insurance in an increasingly digital and interconnected world.
Reinsurance optimization
Generative models serve as instrumental tools for refining risk management approaches. These models specialize in conducting thorough risk portfolio analyses, providing insurers with valuable insights into the intricacies of their portfolios. By leveraging generative AI, insurers can optimize their reinsurance strategies by modeling and understanding complex risk scenarios. This analytical prowess enables the identification of potential gaps and areas for improvement. It empowers insurers to make informed decisions, enhancing the overall efficiency and effectiveness of their reinsurance strategies. Generative models, through their sophisticated risk portfolio analyses, contribute significantly to the continuous improvement and optimization of reinsurance practices in the ever-evolving landscape of the insurance industry.
Customer behavior analysis
Generative models emerge as indispensable tools for deciphering intricate patterns and preferences. Through advanced analytics, these models facilitate customer segmentation, providing insurers with a nuanced understanding of individual behaviors. This insight, in turn, becomes the foundation for crafting targeted marketing and retention strategies, ensuring a personalized and engaging experience for each customer. Furthermore, generative AI extends its impact to cross-selling and upselling initiatives. By leveraging the wealth of information gleaned from customer profiles and preferences, insurers can strategically recommend additional insurance products. This personalized strategy not only enhances the overall customer experience but also proactively addresses evolving needs. In essence, generative models in customer behavior analysis contribute to the creation of dynamic and customer-centric strategies, fostering stronger relationships and driving business growth within the insurance industry.
Regulatory compliance
In the landscape of regulatory compliance, generative AI emerges as a crucial ally, offering streamlined solutions for navigating the complexities of ever-changing regulations. Through its capabilities, generative models facilitate automated compliance checks, providing insurers with a dynamic and efficient mechanism to ensure adherence to the latest regulatory requirements. By automating the validation and updating of policies in response to evolving regulations, this technology not only enhances the accuracy of compliance but also significantly reduces the manual burden on regulatory teams. In doing so, generative AI plays a pivotal role in helping insurance companies maintain a proactive and responsive approach to compliance, fostering a culture of adaptability and adherence in the face of regulatory evolution.
AI-powered solutions: Transforming the insurance workflow by addressing key challenges at every stage
The insurance workflow encompasses several stages, ranging from the initial application and underwriting process to policy issuance, premium payments, claims processing, and policy renewal. Although the specific stages may vary slightly depending on the type of insurance (e.g., life insurance, health insurance, property and casualty insurance), the general workflow consistently includes the key stages mentioned here. Below, we delve into the challenges encountered at each stage, presenting innovative AI-powered solutions aimed at enhancing efficiency and effectiveness within the insurance industry.
Stage 1: Application and quoting
Challenge: Manual assessment of applicant data is time-consuming and may lead to inaccuracies.
Solution: Implement AI-driven underwriting tools that analyze diverse data sources quickly, providing more accurate risk assessments and generating precise premium quotes.
Stage 2: Underwriting
Challenge: Underwriters may struggle with the vast amount of data needed for a comprehensive risk assessment.
Solution: Utilize AI for data analytics to process and interpret large datasets, assisting underwriters in making well-informed decisions based on a thorough understanding of the applicant’s risk profile.
Stage 3: Policy issuance
Challenge: Manual creation of policy documents is prone to errors and inefficiencies.
Solution: Implement AI-driven document automation systems to streamline policy issuance, ensuring accuracy and reducing processing time.
Stage 4: Premium payment
Challenge: Manual handling of premium payments can result in delays and errors.
Solution: Implement automated billing and payment systems powered by AI, reducing administrative burden and enhancing the efficiency of financial transactions.
Stage 5: Policyholder services
Challenge: Handling routine inquiries manually can be resource-intensive.
Solution: Deploy AI-driven chatbots and virtual assistants to handle routine policyholder services, allowing human agents to focus on more complex issues and improving overall customer service efficiency.
Stage 6: Claims processing
Challenge: Manual claims triage can lead to delays and inefficiencies.
Solution: Implement AI algorithms for claims triage, quickly assessing claim validity and severity to prioritize and allocate resources effectively.
Stage 7: Fraud mitigation and claims payout optimization
Challenge: Fraudulent claims may go undetected without advanced analytics.
Solution: Integrate AI-powered fraud detection systems to analyze claims data for patterns and anomalies, ensuring accurate payouts and preventing fraudulent activities.
Stage 8: Risk management and loss prevention
Challenge: Identifying and mitigating risks manually can be challenging.
Solution: Implement AI-driven predictive analytics to analyze historical data, identify patterns, and proactively manage and mitigate risks.
Stage 9: Renewal
Challenge: Lack of personalized renewal offers may lead to customer dissatisfaction.
Solution: Use predictive modeling powered by AI to analyze customer behavior and preferences, enabling insurers to tailor renewal offers based on individual policyholder needs and risk profiles.
Stage 10: Cancellation or termination
Challenge: Manual processes for cancellations may lead to delays and compliance issues.
Solution: Implement automated workflows with AI to streamline policy cancellations or terminations, ensuring compliance with regulatory requirements.
Stage 11: Regulatory compliance
Challenge: Keeping up with evolving regulations manually can be time-consuming.
Solution: Utilize AI tools for compliance monitoring to stay informed about regulatory changes and ensure insurance processes and documentation remain in compliance with evolving standards.
By addressing these challenges with AI-driven solutions, insurers can significantly enhance the efficiency, accuracy, and overall effectiveness of their insurance workflow.
Streamlining insurance workflow with generative AI
Generative AI is making significant inroads into the insurance industry, enhancing processes across various areas, including underwriting, claims processing, customer service, fraud detection, and risk management. With its ability to analyze vast datasets and generate insights, generative AI is reshaping how insurers operate and interact with customers.
Key personas involved in the insurance process
- Claims manager: Uses GenAI to automate claims processing, enhancing decision-making efficiency and accuracy.
- Policy administrator: Utilizes GenAI to streamline policy management tasks with automated document generation tools.
- Underwriters: Leverages GenAI to analyze data and assess risk for determining appropriate premiums.
- Compliance officers: Employs GenAI to monitor regulatory changes and ensure adherence through automated alerts.
- Customer service representative: Utilizes GenAI-powered chatbots for efficiently handling customer inquiries and support.
- Actuarial analyst: Uses GenAI for predictive modeling to enhance risk assessment and forecasting accuracy.
Here’s a breakdown of the key stages in insurance workflow and how generative AI is making a significant impact at each step:
Application and underwriting
Steps involved | Sub-steps | Role of generative AI |
---|---|---|
Application submission |
|
|
Data validation and enrichment |
|
|
Risk assessment |
|
|
Premium calculation |
|
|
Application approval |
|
|
Policy issuance and payment
Steps involved | Sub-steps | Role of generative AI |
---|---|---|
Policy issuance |
|
|
Policy document generation |
|
|
Policy delivery |
|
|
Payment reminders |
|
|
Customer support and inquiries |
|
|
Claims reporting and processing
Steps involved | Sub-steps | Role of generative AI |
---|---|---|
Claims reporting |
|
|
Claims assessment |
|
|
Investigation |
|
|
Decision making |
|
|
Claims payment |
|
|
Policy renewal and modification
Steps involved | Sub-steps | Role of generative AI |
---|---|---|
Renewal initiation |
|
|
Risk reassessment |
|
|
Premium recalculation |
|
|
Policy modification |
|
|
Customer approval |
|
|
Policy cancellation
Steps involved | Sub-steps | Role of generative AI |
---|---|---|
Cancellation request |
|
|
Cancellation processing |
|
|
Cancellation documentation |
|
|
The integration of generative AI throughout the insurance workflow lifecycle significantly enhances operational efficiency and customer experience by automating routine tasks and providing data-driven insights. However, while GenAI streamlines processes, human judgment remains essential in making final decisions, ensuring that the unique needs and nuances of each customer are effectively addressed.
Optimize Your Operations With AI Agents
Optimize your workflows with ZBrain AI agents that automate tasks and empower smarter, data-driven decisions.
How LeewayHertz’s generative AI platform enhances insurance operations
LeewayHertz’s generative AI platform, ZBrain, serves as an indispensable tool for optimizing and streamlining various facets of insurance processes within the industry. By crafting tailored LLM-based applications that cater to clients’ proprietary insurance data, ZBrain enhances operational workflows, ensuring efficiency and elevating overall service quality. The platform adeptly uses diverse insurance data types, including policy details and claims documents, to train advanced LLMs like GPT-4, Vicuna, Llama 2, or GPT-NeoX. This enables the creation of context-aware applications that enhance decision-making, provide deeper insights, and boost overall productivity. All these advancements are achieved while upholding stringent data privacy standards, making ZBrain an essential asset for modern insurance operations.
Challenges such as intricate procedural workflows, interoperability issues across insurance systems, and the need to adapt to rapid advancements in insurance technology are prevalent in the insurance domain. ZBrain addresses these challenges with sophisticated LLM-based applications, which can be conceptualized and created using ZBrain’s “Flow” feature. Flow offers an intuitive interface, allowing users to effortlessly design intricate business logic for their apps without requiring coding skills. With Flow, users can seamlessly integrate large language models, prompt templates, and other generative AI models into their app’s logic, using a user-friendly drag-and-drop interface for the easy conceptualization, creation, and modification of intelligent applications.
To comprehensively understand how ZBrain Flow works, explore this resource that outlines a range of industry-specific Flow processes. This compilation highlights ZBrain’s adaptability and resilience, showcasing how the platform effectively meets the diverse needs of various industries, ensuring enterprises stay ahead in today’s rapidly evolving business landscape.
With robust apps built on ZBrain, insurance professionals can transform complex data into actionable insights, ensuring heightened operational efficiency, minimized error rates, and elevated overall quality in insurance processes. ZBrain stands out as a versatile solution, offering comprehensive answers to some of the most intricate challenges in the insurance industry.
LeewayHertz’s AI development services for insurance
At LeewayHertz, we craft tailored AI solutions that cater to the unique requirements of insurance companies. We provide strategic AI/ML consulting that enables insurers to harness AI for enhanced risk assessment, improved customer engagement, and optimized policy management.
Our expertise in developing Proof of Concepts (PoCs) and Minimum Viable Products (MVPs) allows insurance firms to preview the potential impacts of AI tools in real scenarios, ensuring that the solutions are both effective and tailored to the insurance sector’s specific needs.
Our work in generative AI also transforms routine tasks like claim processing and documentation, automating these processes to free up underwriters and claims adjusters for more strategic roles.
By fine-tuning large language models to the nuances of insurance terminology and customer interactions, LeewayHertz enhances the accuracy and relevance of AI-driven communications and analyses.
Additionally, we ensure these AI systems integrate seamlessly with existing technological infrastructures, enhancing operational efficiency and decision-making in insurance companies.
Our AI solutions development expertise
AI solutions development for the insurance industry typically involves creating systems that enhance decision-making, automate routine tasks, and personalize customer interactions. These solutions integrate key components such as data aggregation technologies, which compile and analyze information from diverse sources. This comprehensive data foundation supports predictive analytics capabilities, allowing for the forecasting of risks and claims trends that inform strategic decisions.
Generative AI, specifically, plays a pivotal role in transforming tasks like claim processing, policy documentation, and customer service interactions. Machine learning algorithms are employed to tailor insurance policies to individual client profiles, ensuring that each client’s unique needs and risk factors are considered. These solutions often cover areas like underwriting, fraud detection, risk assessment, regulatory compliance, and customer relationship management.
Overall, AI solutions in insurance aim to optimize operational efficiency, improve accuracy in risk assessments, and elevate the customer experience by providing timely and personalized services.
AI agent/copilot development for insurance
LeewayHertz builds custom AI agents and copilots that enhance various insurance operations, enabling companies to save time and resources while facilitating faster decision-making. Here is how they help:
Claims processing:
- Automating the analysis and verification of claims documents.
- Generating detailed claims reports and processing claims efficiently.
- Identifying potential fraudulent claims based on predefined patterns.
Customer engagement:
- Analyzing customer data and past interactions to provide personalized advice and recommendations.
- Automating routine communication tasks like policy updates and claim status notifications.
- Offering 24/7 virtual assistance to answer customer queries and provide basic information.
Underwriting and risk assessment:
- Automating the evaluation of underwriting documents to streamline the process.
- Assessing risk profiles and generating risk assessment reports.
- Monitoring policies for compliance with predefined rules and regulations.
Regulatory compliance:
- Automating the analysis of regulatory documents to ensure compliance with evolving regulations.
- Generating compliance reports and flagging potential compliance violations.
- Monitoring transactions for adherence to predefined compliance standards.
Policy management:
- Automating the generation and updating of policy documents.
- Managing policy renewals and customer notifications.
- Customizing policies based on individual client profiles and risk factors.
Fraud detection:
- Monitoring transactions and claims for patterns associated with potential fraud.
- Flagging suspicious activities based on predefined criteria or models.
- Generating reports on detected fraud instances for further investigation.
Marketing and content generation:
- Generating personalized marketing communications or insurance education materials based on templates or structured data inputs.
- Assisting with content creation for websites, social media, and marketing campaigns within defined parameters.
Customer segmentation and targeting:
- Analyzing customer data to segment clients based on predefined criteria (e.g., demographics, risk profiles, policy preferences).
- Identifying potential cross-selling or upselling opportunities based on customer segments.
AI agents/copilots don’t just increase the efficiency of operational processes but also significantly enhance the efficiency of the insurance sector’s operations. By integrating these advanced AI solutions into their existing infrastructure, insurance companies can achieve a significant competitive advantage, navigating the complex insurance landscape with innovative, efficient, and reliable AI-driven tools and strategies.
Implementing generative AI in insurance: Key steps
Implementing generative AI in the insurance industry involves a strategic and phased approach. Here are key steps for incorporating generative AI:
Define objectives: Identify specific use cases and objectives for implementing generative AI, such as risk assessment, customer profiling, fraud detection, or automated underwriting.
Data preparation: Ensure high-quality and diverse datasets are available. Generative AI models require robust training data to effectively learn and generate meaningful outputs.
Select appropriate models: Choose generative AI models based on the use case. Generative Adversarial Networks (GANs), Variational Autoencoders (VAEs), or other relevant models may be suitable for different applications.
Training and validation: Train the chosen model using historical data. Validate the model’s performance to ensure it accurately captures patterns and relationships within the data.
Integration with existing systems: Integrate generative AI seamlessly into existing insurance systems and workflows. This may involve collaboration with IT teams to ensure compatibility and minimal disruption.
Security and privacy considerations: Establish strong security protocols to protect sensitive data effectively. Ensure compliance with data privacy regulations and utilize techniques such as differential privacy when handling personal information.
Continuous monitoring and improvement: Implement mechanisms for ongoing monitoring of generative AI models. Regularly update and retrain models to adapt to changing data patterns and improve accuracy.
Collaboration with domain experts: Work closely with insurance domain experts to interpret model outputs and validate the relevance of generated content. Domain expertise is crucial for refining and optimizing model performance.
User training and acceptance: Train end-users, such as underwriters or claims processors, to leverage generative AI outputs effectively. Foster acceptance and understanding of the technology among stakeholders.
Scale gradually: Begin with pilot projects or specific use cases to assess the impact of generative AI. Gradually scale implementation based on successful outcomes and feedback.
Feedback loop: Establish a feedback loop to facilitate ongoing enhancements and improvements. Collect input from users and stakeholders to pinpoint areas for improvement, refining the implementation of generative AI over time.
If you are in search of a tech partner for transforming your insurance operations through innovative technology, look no further than LeewayHertz. Our team specializes in offering extensive generative AI consulting and development services uniquely crafted to propel your insurance business into the digital age. Backed by a proven track record, LeewayHertz brings a wealth of expertise in implementing diverse advanced generative AI models and solutions, empowering you to kickstart or enhance your AI-driven initiatives within the insurance industry.
The benefits of generative AI in insurance
Generative AI is significantly impacting the insurance industry, offering numerous advantages that redefine how insurers operate, interact with customers, and manage risks. Here are some key benefits of implementing generative AI in the insurance industry:
Enhanced customer experience
Generative AI enables insurers to offer personalized experiences to their customers. By processing extensive volumes of customer data, AI algorithms have the capability to tailor insurance products to meet individual needs and preferences. Virtual assistants powered by generative AI engage in real-time interactions, guiding customers through policy inquiries and claims processing, leading to higher satisfaction and increased customer loyalty.
Improved risk assessment and underwriting
Generative AI models can assess risks and underwrite policies more accurately and efficiently. Through the analysis of historical data and pattern recognition, AI algorithms can predict potential risks with greater precision. This enables insurers to optimize underwriting decisions, offer tailored coverage options, and reduce the risk of adverse selection.
Streamlined claims processing
Generative AI automates claims processing, extracting and validating data from claim documents. This streamlines the entire claims settlement process, reducing turnaround time and minimizing errors. Faster and more accurate claims settlements lead to higher customer satisfaction and improved operational efficiency for insurers.
Advanced fraud detection and prevention
Advanced generative AI algorithms can detect patterns and anomalies associated with fraudulent behavior, enabling early detection and prevention of fraudulent claims. By analyzing vast datasets, generative AI enhances fraud detection capabilities, safeguarding insurers from potential financial losses and maintaining their credibility.
Proactive risk management
Generative AI’s predictive modeling capabilities allow insurers to simulate and forecast various risk scenarios. By identifying potential risks in advance, insurers can develop proactive risk management strategies, mitigate losses, and optimize their risk portfolios effectively.
Data-driven insights
Generative AI-driven customer analytics provides valuable insights into customer behavior, market trends, and emerging risks. This data-driven approach empowers insurers to develop innovative services and products that cater to changing customer needs and preferences, leading to a competitive advantage.
Cost savings and operational efficiency
By automating various processes, generative AI reduces the need for manual intervention, leading to cost savings and improved operational efficiency for insurers. Automated claims processing, underwriting, and customer interactions free up resources and enable insurers to focus on higher-value tasks.
Regulatory compliance and transparency
Generative AI can incorporate explainable AI (XAI) techniques, ensuring transparency and regulatory compliance. Insurers can understand the reasoning behind AI-generated decisions, facilitating compliance with regulatory standards and building customer trust in AI-driven processes.
Put data control back in the consumer’s hands
Generative AI makes it efficient for insurers to digitally activate a zero-party data strategy—a data-gathering approach proving successful for many other industries. The zero-party advantage leverages responses that consumers willingly provide an insurer to a set of simple, personalized questions posed to them, helping sales and marketing agents collect response data in a noninvasive and transparent way. Insurers receive actionable data insights from consumers, while consumers receive more customized insurance that better protects them.
Generative AI holds immense potential to reshape the insurance industry. By enhancing customer experiences, improving risk assessment and underwriting, streamlining claims processing, detecting and preventing fraud, enabling proactive risk management, providing data-driven insights, optimizing operations, fostering innovation, and ensuring regulatory compliance, generative AI empowers insurers to thrive in an ever-changing market.
Types of generative AI models used in the insurance sector
Generative models in AI are designed to learn and replicate patterns and structures found within their training data, allowing them to generate new samples that resemble the original data. In the context of generative AI in insurance, three prominent types of generative models stand out: Generative Adversarial Networks (GANs), Variational Autoencoders (VAEs), and autoregressive models.
Generative Adversarial Networks (GANs)
GANs are a class of generative models introduced by Ian Goodfellow and his colleagues in 2014. They consist of two neural networks, the generator and the discriminator, engaged in a competitive game. The generator’s role is to generate fake data samples, while the discriminator’s task is to distinguish between real and fake samples. During training, the generator learns to generate data that is increasingly difficult for the discriminator to differentiate from real data. This back-and-forth training process makes the generator proficient at generating highly realistic and coherent data samples.
In the context of insurance, GANs can be employed to generate synthetic but realistic insurance-related data, such as policyholder demographics, claims records, or risk assessment data. These generated samples can augment the existing data for training and improve the performance of various AI models used in insurance applications. For instance, insurers have used GANs to generate synthetic insurance data, which helps in training AI models for fraud detection, customer segmentation, and personalized pricing. By generating realistic synthetic data, GANs not only enhance data quality but also enable insurers to develop more accurate and reliable predictive models, ultimately improving insurance operations’ overall efficiency and accuracy.
Variational Autoencoders (VAEs)
VAEs are another type of generative model that combines elements of both generative and inference models. VAEs consist of two main components: a decoder and an encoder. The encoder is responsible for transforming the input data into a latent space representation, while the decoder reconstructs the data from the latent space back into the original data space.
VAEs differ from GANs in that they use probabilistic methods to generate new samples. By sampling from the learned latent space, VAEs generate data with inherent uncertainty, allowing for more diverse samples compared to GANs. In insurance, VAEs can be utilized to generate novel and diverse risk scenarios, which can be valuable for risk assessment, portfolio optimization, and developing innovative insurance products.
Autoregressive models
Autoregressive models are generative models known for their sequential data generation process, one element at a time, based on the probability distribution of each element given the previous elements. In other words, an autoregressive model predicts each data point based on the values of the previous data points. These models are often used for generating sequences or time-series data.
In insurance, autoregressive models can be applied to generate sequential data, such as time-series data on insurance premiums, claims, or customer interactions. These models can help insurers predict future trends, identify anomalies within the data, and make data-driven decisions for business strategies. For example, autoregressive models can predict future claim frequencies and severities, allowing insurers to allocate resources and proactively prepare for potential claim surges. Additionally, these models can be used for anomaly detection, flagging unusual patterns in claims data that may indicate fraudulent activities. By leveraging autoregressive models, insurers can gain valuable insights from sequential data, optimize operations, and enhance risk management strategies.
All three types of generative models, GANs, VAEs, and autoregressive models, offer unique capabilities for generating new data in the insurance industry. GANs excel at producing highly realistic samples, VAEs provide diverse and probabilistic samples, while autoregressive models are well-suited for generating sequential data. By leveraging these powerful generative models, insurers can enhance their data analysis, risk assessment, and product development, ultimately redefining how the insurance industry operates.
The future of generative AI in insurance
As the insurance industry continues to evolve, generative AI has already showcased its potential to redefine various processes by seamlessly integrating itself into these processes. Generative AI has left a significant mark on the industry, from risk assessment and fraud detection to customer service and product development. However, the future of generative AI in insurance promises to be even more dynamic and disruptive, ushering in new advancements and opportunities.
Autonomous claims processing: The future of generative AI in insurance will see a significant shift toward autonomous claims processing. With the integration of advanced computer vision and natural language processing capabilities, AI-powered systems will efficiently process and validate claims without human intervention. Customers will benefit from quicker and more accurate settlements, reducing the time and effort involved in claim filing and processing.
Integration of IoT and generative AI: The Internet of Things (IoT) will intersect with generative AI to create a seamless ecosystem of interconnected devices and data. Insurers will leverage data from smart devices such as connected cars, wearables, and home sensors to assess risks accurately, tailor policies based on real-time data, and prevent losses by offering personalized safety recommendations.
Explainable AI (XAI) for transparency: As the adoption of generative AI in insurance grows, the demand for Explainable AI (XAI) will intensify. XAI techniques will be crucial in ensuring transparency, compliance, and trust in AI-generated decisions. Insurers will need to explain how AI algorithms arrive at specific recommendations or decisions, enabling better customer understanding and regulatory compliance.
Ethical AI and regulatory compliance: As generative AI becomes more pervasive in the insurance industry, ensuring ethical AI practices and adherence to regulatory guidelines will be paramount. Insurers will be required to adopt robust governance frameworks to address bias, fairness, and privacy concerns associated with AI algorithms.
Health and well-being convergence: The trend of health and well-being convergence complements the application of generative AI in promoting holistic and personalized care. Insurers can leverage AI-generated insights to offer customized health insurance plans that incentivize healthy lifestyles, reducing claims costs and improving customer retention. Generative AI’s ability to analyze health data and predict risk factors will enable insurers to design wellness programs tailored to individual policyholders’ needs.
Inclusive insurance: Generative AI in insurance is well-aligned with the trend of inclusive insurance. As AI models process vast amounts of data, insurers can identify underserved markets and demographics, including low-income households, migrants, and small businesses. By expanding into these underserved segments, insurers bridge the protection gap and contribute to the financial well-being and stability of the broader population.
Cybersecurity insurance solutions: With the growing importance of cybersecurity insurance, generative AI could play a significant role in simulating and predicting cyber threats. This could aid insurers in offering more robust and customized cybersecurity policies based on the evolving risk landscape.
Climate risk assessment: As attention to climate change grows, there is a rising interest in utilizing generative AI to evaluate and model risks associated with climate change in the insurance sector. Employing generative AI in this context could provide insurers with enhanced capabilities to comprehend and effectively address the risks linked to extreme weather events and other factors influenced by climate change.
It is crucial to acknowledge that the adoption of these trends will hinge on diverse factors, encompassing technological progress, regulatory assessments, and the specific requirements of individual industries. The insurance sector is likely to see continued evolution and innovation as generative AI technologies mature and their applications expand.
Final words
The emergence of generative AI has significantly impacted the insurance industry, delivering a multitude of advantages for insurers and customers alike. From automating business processes and enhancing operational efficiency to providing personalized customer experiences and improving risk assessment, generative AI has proven its potential to redefine the insurance landscape. As the technology continues to advance, insurers are poised to unlock new levels of innovation, offering tailored insurance solutions, proactive risk management, and improved fraud detection. However, the adoption of generative AI also demands attention to data privacy, regulatory compliance, and ethical considerations. With a balanced approach, the future of generative AI in insurance holds immense promise, ushering in a new era of efficiency, customer satisfaction, and profitability in the dynamic and ever-evolving insurance landscape.
Want to leverage the power of generative AI in your insurance operations? Connect with LeewayHertz’s team of AI experts to explore tailored solutions that enhance efficiency, streamline processes, and elevate customer experiences.
Listen to the article
Start a conversation by filling the form
All information will be kept confidential.
FAQs
What are the key applications of generative AI in the insurance sector?
Generative AI finds applications in insurance for personalized policy generation, fraud detection, risk modeling, customer communication and more. Its versatility allows insurance companies to streamline processes and enhance various aspects of their operations.
Are there specific generative AI models commonly used in the insurance industry?
Yes, several generative AI models, including Variational Autoencoders (VAEs), Generative Adversarial Networks (GANs), and Transformer Models, are commonly used in the insurance sector. Each model serves specific purposes, such as data generation and natural language processing.
Is it possible to integrate generative AI into existing systems of small and medium-sized insurance enterprises, and what benefits can be expected?
LeewayHertz ensures flexible integration of generative AI into businesses’ existing systems. The benefits include improved risk assessment accuracy, streamlined claims processing, and enhanced customer engagement, offering a seamless transition for small and medium-sized insurance enterprises.
How can LeewayHertz assist my insurance company in leveraging generative AI for innovation and improved customer experiences?
LeewayHertz specializes in tailoring generative AI solutions for insurance companies of all sizes. We focus on innovation, enhancing risk assessment, claims processing, and customer communication to provide a competitive edge and drive improved customer experiences.
Can LeewayHertz customize generative AI applications to address specific challenges faced by my insurance business?
Absolutely. LeewayHertz specializes in customizing generative AI applications to address specific challenges in the insurance sector, including fraud detection, personalized policy generation, and optimizing various processes to meet the distinctive needs of your business.
What ethical considerations does LeewayHertz prioritize when implementing generative AI in insurance applications?
LeewayHertz prioritizes ethical considerations related to data privacy, transparency, and bias mitigation when implementing generative AI in insurance applications. We adhere to industry best practices to ensure fair and responsible use of AI technologies.
How can LeewayHertz assist my insurance business in addressing challenges associated with generative AI adoption, such as data privacy concerns?
LeewayHertz addresses challenges associated with generative AI adoption by implementing robust data privacy measures, obtaining explicit customer consent, and ensuring compliance with regulatory frameworks. Transparent communication with customers about data usage is a key focus.
- Generative AI in insurance: An overview
- Comparing traditional and generative AI in insurance operations: What sets them apart?
- How does generative AI in insurance work?
- Generative AI use cases in the insurance industry
- AI-powered solutions: Transforming the insurance workflow by addressing key challenges at every stage
- Streamlining insurance workflow with generative AI
- How LeewayHertz's generative AI platform enhances insurance operations
- LeewayHertz’s AI development services for insurance
- Implementing generative AI in insurance: Key steps
- The benefits of generative AI in insurance
- Types of generative AI models used in the insurance sector
- The future of generative AI in insurance
- Contact us