Generative AI in healthcare: Applications, architecture, implementation, and benefits
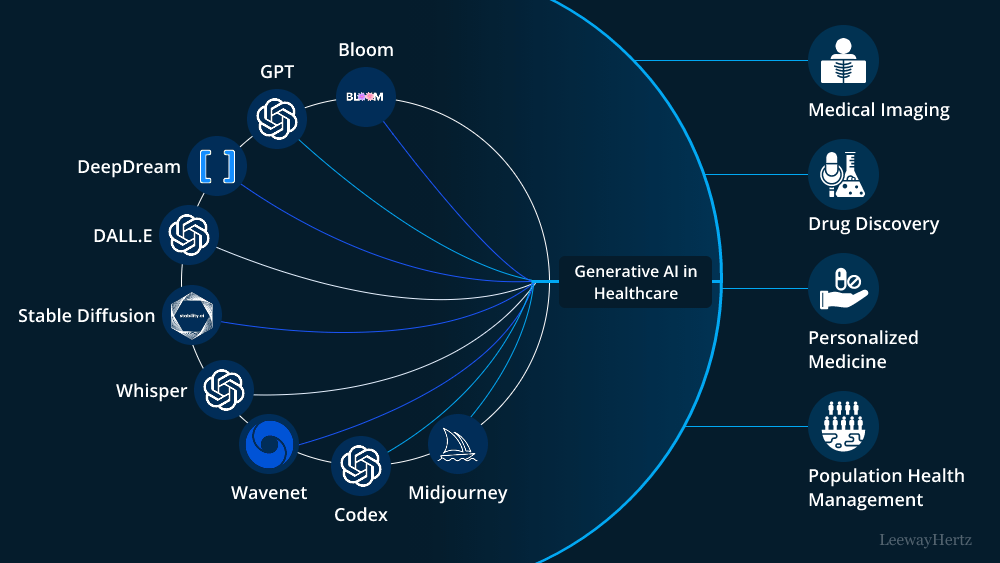
Listen to the article
Like many other industries embracing technological advancements, the healthcare landscape is on the cusp of transformative progress driven by the emergence of generative AI. As advanced machine learning algorithms continue to evolve, they are reshaping multiple aspects of the healthcare industry, transcending the boundaries of traditional approaches. From diagnosis and treatment to drug discovery and personalized medicine, generative AI is poised to transform how healthcare professionals approach complex medical challenges.
By harnessing the capabilities of generative AI, the healthcare industry is poised to witness remarkable advancements that have the capability to enhance patient outcomes, improve medical research, and reshape the entire healthcare landscape. This article will dive deep into the profound impact of generative AI in healthcare and delve into its applications, benefits and other key areas.
- Generative AI in healthcare
- Applications of generative AI in healthcare
- Streamlining healthcare processes with GenAI
- How does LeewayHertz’s generative AI platform transform healthcare workflows?
- How does generative AI in healthcare work?
- How to implement generative AI in healthcare businesses?
- LeewayHertz’s AI development services for healthcare
- AI in action: LeewayHertz’s custom medical assistant solution
- Benefits of generative AI in healthcare
Generative AI in healthcare
Generative AI in healthcare refers to the application of generative artificial intelligence techniques and models in various aspects of the healthcare industry. It involves using machine learning algorithms to generate new and original content that is relevant to healthcare, such as medical images, personalized treatment plans, and more.
Generative AI is ushering in a profound transformation within the healthcare industry, reshaping the way care is delivered and managed on a macro scale. By harnessing the power of artificial intelligence, genAI has unlocked capabilities that were previously inconceivable, fundamentally altering the landscape.
In the past, treatments were often administered based on broad population data, with limited consideration for individual variations. GenAI, however, has made it possible to delve deep into patients’ genetic profiles, medical histories, and real-time health data. This means that healthcare can now be tailored to the unique needs and genetic makeup of each patient. The result: a more precise, effective, and patient-centric approach to medical care that improves outcomes and reduces the occurrence of adverse effects significantly.
Furthermore, genAI is streamlining drug discovery and development processes. Historically, the path from drug discovery to market availability has been arduous and time-consuming. By accelerating the identification of potential drug candidates, optimizing molecular structures, and even predicting side effects and drug interactions, the speed and efficiency enabled by genAI holds the promise of bringing novel and safer medications to patients.
Moreover, the healthcare industry is becoming increasingly data-driven thanks to genAI. The technology’s capacity to analyze vast datasets, detect trends, and make predictions is invaluable for proactive disease management, efficient resource allocation, and evidence-based decision-making.
These data-driven insights are reshaping public health strategies, optimizing hospital operations, and enhancing care delivery at large, ultimately leading to improved patient care and increased sustainability of healthcare systems. In this macro lens view, genAI is paving the way for a more responsive, patient-focused, and data-enhanced healthcare ecosystem that was simply beyond reach in the pre-AI era.
Applications of generative AI in healthcare
Generative AI has shown significant potential in various applications within the healthcare industry. Let us explore each of these applications in detail:
Medical imaging
Advanced AI models, especially those designed for medical analysis, prominently feature advanced techniques like convolutional neural networks (CNNs) and various deep learning frameworks. Here are some key aspects of the impact of generative AI in medical imaging:
- Image synthesis: Generative models synthesize organ or tissue images, serving educational purposes like training medical professionals and simplifying medical condition explanations to patients through visually comprehensible representations.
- Automated segmentation: Generative AI automates the segmentation of organs or abnormalities in medical images, efficiently saving time for healthcare professionals and streamlining the image analysis process.
- Pathology prediction: Analyzing patterns in medical images, generative AI aids in predicting or identifying pathological conditions, facilitating early detection and intervention for improved patient outcomes.
Drug discovery and development
Generative AI techniques can potentially reinvent drug discovery and development, leading to faster and more efficient drug development pipelines. Here is how generative AI is applied:
- Compound generation: Researchers can use generative AI models to expedite drug discovery by efficiently exploring a diverse chemical space. These models propose novel compounds tailored to desired properties, optimizing the search for potential drug candidates and accelerating the identification of promising molecules for further development.
- Predicting drug-drug interactions: Generative AI is crucial in forecasting potential drug interactions, assisting researchers in evaluating the safety and effectiveness of combining medications. This capability is vital for the strategic design of combination therapies, ensuring optimal treatment outcomes while minimizing associated risks in pharmaceutical interventions.
- Biomarker discovery: Generative AI plays a pivotal role in pinpointing disease-related biomarkers, enabling the stratification of patients based on individual characteristics. This process enhances the development of targeted therapies, leading to more precise and effective medical interventions tailored to specific patient needs.
- Clinical trial design: AI models leverage historical clinical trial data to refine the trial design, pinpoint appropriate patient cohorts, and anticipate potential challenges. This optimization significantly boosts drug development efficiency by enhancing the strategic planning of clinical trials.
- Predicting drug adverse effects: AI models play a crucial role in anticipating and mitigating potential adverse effects of drugs, actively contributing to the safety evaluation of drug candidates in their developmental stages. This proactive approach enables the early identification and resolution of safety concerns, enhancing the overall risk management process in drug development.
- Repurposing existing drugs: Generative AI plays a pivotal role in drug repurposing by identifying novel applications for existing medications and predicting their efficacy against different diseases. This opens up promising avenues for accelerated development of treatments across a spectrum of medical conditions, leveraging the established safety profiles of repurposed drugs to address diverse health challenges.
Personalized medicine
Adopting generative AI for medicine is crucial in developing personalized treatment plans based on individual patient data. Here is how generative AI is utilized:
- Tailored treatment plans: Generative models can analyze patient data, including genetic information, medical history, and clinical data, to generate personalized treatment plans. This can aid in selecting the most effective therapies and predicting individual patient responses.
- Predictive analytics for disease progression and treatment response: Generative AI can generate predictive models that estimate disease progression and treatment outcomes by analyzing large datasets and integrating various patient factors. This helps healthcare professionals make informed decisions regarding treatment strategies and optimize patient care.
- Real-time clinical decision support: Gen AI provides clinicians with real-time, evidence-based recommendations for personalized treatment options based on a patient’s genetic profile. This accelerates decision-making by swiftly considering vast amounts of data with precision.
- Ethical and legal compliance assistance: Gen AI aids in navigating ethical and legal considerations related to personalized medicine, ensuring adherence to privacy regulations and ethical standards. This builds patient trust and maintains compliance with healthcare laws.
- Resource optimization in genetic testing: Gen AI contributes to resource optimization by streamlining workflows, automating routine tasks, and enhancing the efficiency of genetic testing processes. This is essential for overcoming resource limitations and making personalized medicine more accessible.
- Pharmacogenomic optimization: One of the applications of AI in medicine is pharmacogenomic optimization. GenAI analyzes pharmacogenomic data to predict individual medication responses, enabling tailored drug prescriptions based on genetic factors. This optimizes treatment outcomes and minimizes adverse effects.
Medical research and data analysis
Generative AI techniques have immense potential in medical research and data analysis. Here are how generative AI aids in medical research and data analysis:
- Data processing: Generative AI swiftly analyzes extensive medical data, automating data extraction and document reviews. This streamlines administrative processes, allowing researchers to focus more on critical aspects of their work.
- Medical document summarization: Generative AI excels at summarizing lengthy medical documents, offering concise overviews for researchers. This accelerates comprehension and decision-making, especially when navigating extensive medical literature.
- Trend identification and analysis: Processing large datasets, Generative AI identifies patterns and analyzes trends in medical research. This keeps researchers informed about the latest developments, fostering a proactive and informed approach in the field.
- Optimizing resource utilization: Generative AI addresses resource constraints in medical research by automating tasks and optimizing available resources. This particularly benefits projects with limited funding or access to high-performance computing resources.
- Predictive analytics insights: Leveraging historical medical data, Generative AI provides insights into potential outcomes, aiding researchers in making informed decisions and creating strategies for their medical research projects.
Administrative task
- Efficient appointment management: Gen AI optimizes appointment scheduling by automating booking and rescheduling, ensuring convenient time slots for patients and enhancing overall scheduling efficiency.
- Automated documentation and record-keeping: Generative AI automates documentation tasks, like transcribing medical notes and updating patient records, relieving healthcare professionals of administrative burdens and fostering streamlined record-keeping.
- Streamlined billing and claims processing: AI automates billing and claims processes, improving accuracy, reducing errors, and expediting reimbursement cycles for healthcare providers, ultimately enhancing financial workflows.
- Data entry and extraction automation: Gen AI automates tasks, extracting pertinent information from diverse sources to minimize manual data input and elevate the accuracy of healthcare databases.
- Effective communication management: AI-powered chatbots handle routine inquiries, appointment reminders, and follow-ups, allowing healthcare staff to focus on more complex tasks and improving communication efficiency.
- Automated regulatory compliance checks: Generative AI automates regulatory compliance checks, ensuring that administrative processes align with healthcare regulations and standards, promoting adherence to legal requirements.
- Workflow efficiency optimization: By analyzing administrative workflows, AI identifies inefficiencies and recommends optimizations, leading to more streamlined and effective processes, ultimately enhancing overall workflow efficiency.
Risk prediction of pandemic preparedness
- Early detection and surveillance: Gen AI processes real-time data from diverse sources, such as social media, health reports, and environmental data, to identify early signs of emerging infectious diseases. Recognizing unusual patterns or clusters contributes to the development of early warning systems.
- Predictive analytics for disease outbreaks: Leveraging historical data and machine learning, Gen AI predicts potential hotspots for disease outbreaks by analyzing population density, travel patterns, and environmental conditions. This provides insights into areas at higher risk.
- Optimizing vaccine development: Gen AI accelerates drug discovery by analyzing genetic data and predicting potential vaccine candidates. This expedites development and testing, contributing to a quicker response to novel pathogens.
- Enhancing supply chain resilience: Gen AI improves supply chain management by predicting and mitigating disruptions. It analyzes global logistics, monitors inventory levels, and forecasts demand, ensuring a more robust and responsive supply chain for medical equipment and pharmaceuticals.
- Optimizing resource allocation: During a pandemic, Gen AI analyzes data on healthcare facility capacities, equipment availability, and workforce distribution to optimize resource allocation based on predicted outbreak patterns, ensuring efficient utilization.
- Scenario planning and preparedness: Gen AI simulates various scenarios based on historical data and current conditions, helping authorities and healthcare organizations plan and prepare for different potential outcomes. This enables a proactive and adaptive approach to pandemic response.
These applications of generative AI in healthcare demonstrate its potential to improve diagnostics, drug development, personalized medicine, and medical research. By leveraging generative AI techniques, healthcare professionals can enhance decision-making, optimize treatment strategies, and improve patient outcomes.
Clinical decision-making
Generative AI is proving to be a powerful tool in assisting healthcare providers with clinical decision-making. By analyzing vast amounts of patient data, including electronic health records, medical images, and genomic information, these models can generate valuable insights to support diagnosis, treatment planning, and risk assessment. Key applications include:
- Predictive modeling: AI can identify patterns in patient data to predict disease progression, potential complications, and treatment responses, enabling proactive care and early interventions.
- Treatment optimization: By analyzing patient-specific factors and treatment outcomes, generative AI can suggest tailored treatment plans, optimizing efficacy and minimizing adverse effects.
- Diagnosis support: AI algorithms can analyze medical images, such as X-rays and MRIs, to assist in detecting abnormalities and suggesting potential diagnoses, enhancing diagnostic accuracy and efficiency.
These applications of generative AI in healthcare demonstrate its potential to improve diagnostics, drug development, personalized medicine, and medical research. By leveraging generative AI techniques, healthcare professionals can enhance decision-making, optimize treatment strategies, and improve patient outcomes.
Optimize Your Operations With AI Agents
Optimize your workflows with ZBrain AI agents that automate tasks and empower smarter, data-driven decisions.
Streamlining healthcare processes with GenAI
Generative AI is reshaping healthcare by streamlining processes and enhancing efficiency. From automating administrative tasks to enhancing patient care and optimizing data management, GenAI is revamping healthcare operations. This section explores how generative AI is transforming healthcare operations for improved outcomes and reduced operational costs.
Patient admission and registration
Steps Involved | Sub-Steps | Role of Generative AI |
---|---|---|
Appointment Scheduling |
|
|
Patient Arrival and Check-In |
|
|
Patient Data Collection and Assessment |
|
|
Care Team Assignment and Consent |
|
|
Administrative Setup |
|
|
Diagnosis and assessment
Steps Involved | Sub-Steps | Role of Generative AI |
---|---|---|
Evaluate Eligibility |
|
|
Obtain Approval |
|
|
Conduct Health Status Evaluation |
|
|
Coordinate Care |
|
|
Conduct and Interpret Imaging |
|
|
Treatment and medication management
Steps Involved | Sub-Steps | Role of Generative AI |
---|---|---|
Treatment Planning and Management |
|
|
Patient Information Management |
|
|
Prescription Processing |
|
|
Medication Monitoring and Adjustment |
|
|
Monitoring, follow-up, and post-treatment care
Steps Involved | Sub-Steps | Role of Generative AI |
---|---|---|
Monitoring Health Status |
|
|
Follow-up Scheduling |
|
|
Post-Treatment Evaluation |
|
|
Patient Support and Education |
|
|
Documentation and Reporting |
|
|
Patient discharge and health education
Steps Involved | Sub-Steps | Role of Generative AI |
---|---|---|
Discharge Preparation |
|
|
Patient Education |
|
|
Care Transition |
|
|
Post-Discharge Support |
|
|
Feedback and Improvement |
|
|
Medical billing and quality improvement
Steps Involved | Sub-Steps | Role of Generative AI |
---|---|---|
Claim Preparation |
|
|
Claim Submission |
|
|
Payment Processing |
|
|
Quality Improvement |
|
|
Compliance and Auditing |
|
|
Optimize Your Operations With AI Agents
Optimize your workflows with ZBrain AI agents that automate tasks and empower smarter, data-driven decisions.
How does LeewayHertz’s generative AI platform transform healthcare workflows?
LeewayHertz’s generative AI platform, ZBrain, is a transformative solution for healthcare organizations seeking to optimize processes, improve patient care, and drive innovation. With its ability to create highly customized LLM-based applications trained on client’s proprietary data, ZBrain presents a unique opportunity to enhance healthcare workflows to elevate operational efficiency and the overall healthcare experience. Leveraging leading language models such as GPT-4, Gemma, Llama 3, and Gemini, ZBrain becomes a versatile tool for interpreting diverse healthcare formats, including medical texts, images, and documents, while ensuring utmost data privacy.
ZBrain’s Flow feature allows the development of complex apps with sophisticated workflows. This low-code interface, integrated within the ZBrain platform, empowers businesses to create and manage business workflows with ease. Its intuitive visual interface allows users to develop complex business logic by seamlessly connecting multiple components, such as AI models, knowledge bases, programming logic, and helper methods. Additionally, ZBrain Flow supports integration with a variety of third-party tools and platforms, including Salesforce, Microsoft and Google software and services, facilitating the creation of diverse generative AI applications. This powerful feature enables hospitals and other healthcare institutions to enhance their cumbersome administrative tasks, improve complex diagnostic procedures, and deliver personalized patient care.
To comprehensively understand how ZBrain Flow works, explore this resource that outlines a range of industry-specific Flow processes. This compilation highlights ZBrain’s adaptability and resilience, showcasing how the platform effectively meets the diverse needs of various industries, ensuring enterprises stay ahead in today’s rapidly evolving business landscape.
Through AI-driven automation and advanced data analysis, ZBrain’s workflows convert intricate healthcare data into actionable insights. This results in increased operational efficiency, lower error rates, and improved healthcare quality. ZBrain emerges as a comprehensive solution to address critical issues in the healthcare industry, making strides toward a more efficient and patient-centric healthcare ecosystem. Here are a few of them-
AI-driven fraud detection
ZBrain transforms the healthcare fraud detection process by significantly decreasing the time and effort needed to pinpoint fraudulent activities. Through ZBrain, healthcare providers and insurance companies gain an efficient tool for detecting and preventing fraud, safeguarding the integrity of the healthcare system and mitigating financial losses and risks. Embrace the capabilities of ZBrain to strengthen the security of your healthcare operations and fortify defenses against fraudulent activities. To understand how ZBrain transforms the fraud detection process, explore the detailed Flow process here.
AI-driven pharmaceutical pricing and promotion
Leveraging the insights and automation offered by ZBrain, pharmaceutical companies can swiftly establish competitive prices and create impactful promotions, accomplishing in a fraction of the traditional time. This increases patient satisfaction, market share, and profitability within the dynamic pharmaceutical sector. To understand how ZBrain helps in mastering pharmaceutical pricing and promotion, explore the detailed Flow process here.
How does generative AI in healthcare work?
Incorporating generative AI into healthcare processes involves various components to streamline data analysis, generate insights, and support decision-making. It goes beyond traditional healthcare methods by integrating powerful Large Language Models (LLMs) and connecting them with an organization’s unique knowledge base. This architecture helps generate new insights and empowers healthcare providers and patients to make data-driven decisions in real-time.
Here’s a step-by-step breakdown of how generative AI works in healthcare:
Data sources: The process starts with gathering data from various relevant sources, including:
- Patient records: Electronic health records (EHRs), patient history, and medical images from hospitals and clinics.
- Medical literature: Research papers, clinical trial results, and guidelines from medical journals and databases.
- Wearable devices: Health metrics such as heart rate, blood pressure, and activity levels from wearable devices and health apps.
- Genomic data: Genetic information from genomic sequencing databases.
- Pharmacy data: Prescription records and medication details from pharmacies.
- Public health data: Information on disease outbreaks, vaccination rates, and public health reports from governmental and health organizations.
Data pipelines: The gathered data is routed through data pipelines responsible for ingestion, cleaning, and structuring the data, making it ready for further analysis.
Embedding model: The prepared data is then processed by an embedding model, which transforms textual data into numerical representations (vectors) that AI models can understand. Popular models include those from OpenAI, Google, and Cohere.
Vector database: The generated vectors are stored in a vector database, allowing for efficient querying and retrieval. Examples of vector databases include Pinecone, Weaviate, and PGvector.
APIs and plugins: APIs and plugins like Serp, Zapier, and Wolfram connect different components and enable additional functionalities, such as accessing extra data or performing specific tasks with ease.
Orchestration layer: The orchestration layer manages the overall workflow. Tools like ZBrain are prominent orchestration platforms. They simplify prompt chaining, manage interactions with external APIs, retrieve contextual data from vector databases, and maintain memory across multiple LLM calls. This layer generates prompts or series of prompts for processing by a language model, ensuring seamless coordination across all components within the architecture.
Query execution: When a user submits a query to the healthcare app, the data retrieval and generation process gets triggered. This query can be about anything relevant to healthcare, such as diagnosing symptoms or suggesting treatment options.
LLM processing: The app transmits the query to the orchestration layer, which retrieves relevant data from the vector database and LLM Cache and sends it to the appropriate LLM. The choice of LLM depends on the nature of the query.
Output: The LLM generates an output based on the query and received data. This output can take various forms, such as diagnostic suggestions, treatment plans, or health advice.
Healthcare app: The validated output is then presented to the user through the healthcare app. This core application consolidates all the data, analysis, and insights, presenting them in a user-friendly format for healthcare providers and patients.
Feedback loop: User feedback on the LLM’s output helps improve the accuracy and relevance of future outputs. It is a crucial part of the architecture.
AI agents: AI agents handle complex problems, interact with the external environment, and enhance learning through post-deployment experiences. To achieve this, they use advanced reasoning/planning, strategic tool utilization and leverage memory, recursion, and self-reflection.
LLM cache: Tools like Redis, SQLite, or GPTCache are employed to cache frequently accessed information, speeding up the AI system’s response time.
Logging/LLMOps: LLMOps tools like Weights & Biases, MLflow, Helicone, and Prompt Layer log actions and monitor performance, ensuring LLMs function optimally and improve through feedback loops.
Validation: A validation layer is utilized that leverages tools like Guardrails, Rebuff, Guidance, and LMQL to ensure the LLM’s output is accurate and reliable.
LLM APIs and hosting: LLM APIs and hosting platforms execute healthcare tasks and host applications. Depending on requirements, developers can select from LLM APIs offered by companies like OpenAI and Anthropic or use open-source models. Hosting platforms include AWS, GCP, Azure, and Coreweave, or opinionated clouds like Databricks, Mosaic, and Anyscale.
This structured flow demonstrates how generative AI in healthcare leverages various data sources and technological tools to generate accurate and actionable insights, automate healthcare tasks, and provide a personalized and optimized healthcare experience.
How to implement generative AI in healthcare businesses?
Integrating generative AI into the healthcare industry requires a methodical approach to guarantee effective implementation and utilization. Here is a step-by-step guide:
- Identify use cases: Clearly define the specific use cases where generative AI can add value in healthcare, such as medical image analysis, natural language processing for clinical documentation, drug discovery, predictive analytics, or personalized medicine.
- Data collection and preparation: Gather high-quality, relevant datasets for training generative AI models. Ensure the data is diverse, representative, and compliant with privacy and regulatory standards. Clean and preprocess the data to enhance model training accuracy.
- Select the appropriate generative model: Choose the right one based on the identified use cases. Common architectures include GANs (Generative Adversarial Networks), VAEs (Variational Autoencoders), and language models like GPT (Generative Pre-trained Transformer).
- Train the model: Use the prepared datasets to train the chosen generative model. Depending on the complexity of the task, this may require significant computational resources. Fine-tune the model to achieve optimal performance for the specific healthcare application.
- Validation and testing: Validate the generative model using separate datasets not used during training. Test the model’s performance, accuracy, and reliability to ensure it meets the desired standards for deployment in a healthcare setting.
- Integration with healthcare systems: Integrate the generative AI model with existing healthcare systems and workflows. Ensure compatibility with Electronic Health Records (EHR) and other relevant tools used in the healthcare industry.
- Address regulatory compliance: Comply with healthcare regulations, such as HIPAA (Health Insurance Portability and Accountability Act) in the United States or similar data protection laws in other regions. Enforce robust security protocols to safeguard patient data.
- Continuous monitoring and improvement: Implement mechanisms for continuously monitoring the generative AI system’s performance. Regularly update the model using new data to adapt to changing healthcare landscapes and improve accuracy.
- Ethical considerations and transparency: Address ethical considerations, such as bias in AI algorithms, and ensure transparency in how generative AI is used in healthcare. Maintain open communication with stakeholders and patients about the impact of AI on healthcare processes.
If you are looking for a technological collaborator, LeewayHertz stands as your reliable partner, providing generative AI consulting and development services tailored to elevate your healthcare operations to the forefront of digital innovation. With an established history in deploying a broad spectrum of sophisticated LLM models and solutions, LeewayHertz is poised to either initiate or advance your journey in AI, enhancing patient care, operational efficiency, and data-driven decision-making in your healthcare enterprise.
Optimize Your Operations With AI Agents
Optimize your workflows with ZBrain AI agents that automate tasks and empower smarter, data-driven decisions.
LeewayHertz’s AI development services for healthcare
At LeewayHertz, we offer advanced AI solutions specifically tailored to enhance various aspects of healthcare. Our AI-powered tools are designed to streamline complex data analysis, automate patient assessments, and improve the accuracy and speed of medical diagnostics. Our strategic AI/ML consulting and development services help healthcare organizations leverage AI to automate in-depth data analysis, streamline patient care processes, and make informed decisions quickly and accurately.
Our expertise in developing Proof of Concepts (PoCs) and Minimum Viable Products (MVPs) allows healthcare providers to test the practical impact of AI tools, ensuring solutions are effective, innovative, and tailored to meet specific medical and compliance challenges. This step is crucial for demonstrating AI’s potential to transform traditional healthcare processes into more efficient and comprehensive systems.
Our AI solutions are designed to automate and enhance healthcare processes. By utilizing advanced data aggregation and machine learning algorithms, we swiftly analyze vast amounts of medical data—from patient records and lab results to clinical trial data and beyond. This automation extends to routine tasks such as data entry and documentation, freeing up healthcare professionals to focus on higher-level analysis and patient care.
Additionally, we ensure these AI systems integrate seamlessly with existing healthcare infrastructures. This facilitates a smooth transition to more automated operations, reducing the risk of errors and improving the speed of diagnostics and patient assessments.
Our AI solutions development expertise
AI solutions for healthcare involve creating systems that autonomously analyze complex medical data. These solutions automate comprehensive data reviews, including checks against global regulatory requirements and standards. They generate detailed reports and insights, supporting critical healthcare decisions with accurate and up-to-date information. Additionally, they provide decision support for diagnosing diseases, managing patient care, and planning treatments, ensuring all healthcare requirements are met efficiently. These systems utilize advanced machine learning algorithms and data aggregation technologies to process and analyze extensive datasets, generating outputs that support thorough and precise medical assessments. Key applications include automated compliance verification, risk assessment for health irregularities, and predictive analytics for future health risks.
By integrating advanced AI solutions into their healthcare processes, organizations can navigate the complexities of medical compliance with greater agility and accuracy, ensuring comprehensive risk management and operational excellence.
AI Agent/Copilot development for healthcare
LeewayHertz builds custom AI agents and copilots that significantly enhance the capabilities of healthcare organizations. Here’s how they help:
Automated data processing and analysis:
-
Automatically process and analyze complex medical documentation, extracting critical information quickly.
-
Forecast potential health risks based on historical data and current health conditions.
-
Implement data normalization and synthesis to maintain data accuracy and consistency across multiple sources and systems.
Enhanced compliance and risk management:
-
Ensure thorough compliance with all applicable medical laws and regulations, automating the verification process.
-
Assess potential health risks, providing a quantifiable risk score and detailed insights.
-
Continuously monitor updates in regulatory requirements and adjust compliance strategies in real-time.
Document analysis and management:
-
Automatically scan and extract key information from numerous documents, reducing the time and effort required for manual data entry and analysis.
-
Ensure all medical processes comply with relevant laws and regulations by cross-referencing and verifying compliance across documents.
Operational efficiency:
-
Optimize the workflow of healthcare processes, ensuring that all steps are completed efficiently and in a timely manner.
-
Facilitate better collaboration among healthcare teams, regardless of their geographic locations, by providing synchronized access to findings and reports.
Real-time updates and monitoring:
-
Monitor developments and updates related to patient health, providing real-time alerts to any changes that could affect treatment outcomes.
-
Offer ongoing support and receive regular updates to adapt to new regulatory requirements and industry standards.
By integrating advanced AI solutions into their healthcare processes, organizations can navigate the complexities of medical compliance with greater agility and accuracy, ensuring comprehensive risk management and operational excellence.
AI in action: LeewayHertz’s custom medical assistant solution
LeewayHertz collaborated with a leading healthcare organization to develop an AI-driven medical assistant that optimizes disease diagnosis. By leveraging Natural Language Processing (NLP) and machine learning, the solution analyzes patient data to generate accurate and timely diagnoses.
The AI assistant streamlined the diagnostic workflow of the healthcare provider by generating structured reports that contained potential diagnoses, recommended tests, treatment plans, and patient education materials. This comprehensive solution enhanced diagnostic accuracy, improved patient care, and saved healthcare providers valuable time.
Key benefits of the solution include:
-
Enhanced diagnostic precision: Accurate and timely diagnoses through in-depth data analysis.
-
Structured reporting: Clear and actionable recommendations for healthcare providers.
-
Streamlined workflow: Efficient data input and streamlined diagnostic process.
-
Evidence-based decision making: Treatment recommendations based on latest medical research.
-
Improved patient care: Comprehensive care plans and patient education resources.
This AI-powered medical assistant demonstrates the potential of generative AI to transform healthcare by providing intelligent support for clinical decision-making.
Benefits of generative AI in healthcare
The benefits of generative AI in healthcare are numerous, some of which include:
- Automating repetitive tasks: Generative AI can automate routine and repetitive tasks in healthcare, such as data entry, administrative processes, and image analysis. This automation saves time for healthcare professionals, enabling them to give attention to more complex and critical aspects of patient care.
- Streamlined workflows and resource utilization: Generative AI can optimize healthcare workflows by allocating resources and prioritizing tasks intelligently. It can assist in scheduling appointments, managing patient flow, and coordinating care, leading to smoother operations and efficient resource utilization. This can result in cost reduction and improved patient satisfaction.
- Reduction of human error and biases: Human errors and biases are inherent in healthcare, and they can impact diagnoses, treatment decisions, and patient outcomes. Generative AI models, when properly trained and validated, can minimize these errors by providing objective and consistent analysis. They can mitigate the effects of human biases and ensure more accurate and unbiased predictions and diagnoses.
- More accurate predictions and diagnoses: Generative AI models can analyze vast patient data, including medical records, genetic information, and environmental factors. By integrating and analyzing these data points, AI models can identify patterns and relationships that may not be apparent to humans. This can lead to more accurate disease progression predictions, personalized treatment plans, and early detection of potential risks.
- Virtual simulations and training scenarios: Generative AI can create realistic virtual simulations and training scenarios for medical education. These simulations allow healthcare professionals to replicate a wide range of medical conditions and procedures, creating a safe and controlled environment for skill practice and refinement. It enables hands-on training without risking patient safety and provides opportunities for repeated practice and feedback.
- Access to diverse case studies and expert knowledge: Generative AI can generate synthetic medical data, including patient profiles and clinical scenarios. This synthetic data can be used for educational purposes, providing access to diverse case studies and rare conditions. It enables healthcare professionals to enhance their knowledge, learn from experts, and develop expertise in specialized areas that may be limited in real-world patient encounters.
Endnote
The advent of generative AI has brought forth transformative advancements and opportunities in the healthcare field. With its ability to generate novel data, improve diagnostics, optimize treatment strategies, and expedite drug discovery, generative AI is reshaping the healthcare landscape. From enhancing medical imaging and patient care to enabling personalized medicine and streamlining drug development, this powerful technology holds immense potential for improving healthcare outcomes. As we continue to harness the capabilities of generative AI and address the associated challenges, we are poised to witness a new era of innovation and breakthroughs in the realm of healthcare.
Partner with LeewayHertz to build robust generative AI solutions tailored to your business-specific use case in healthcare and stay at the forefront of technological advancements for improved healthcare delivery.
Listen to the article
Start a conversation by filling the form
All information will be kept confidential.
FAQs
What is generative AI in healthcare?
Generative AI in healthcare refers to the application of artificial intelligence (AI) techniques that can generate new and meaningful data, such as images, text, or structures, to assist in various aspects of healthcare, from drug discovery to patient care.
What is the role of generative AI in drug discovery?
Generative AI plays a crucial role in drug discovery by predicting molecular structures, simulating drug interactions, and proposing potential compounds. This accelerates the drug development process, making it more efficient and cost-effective.
What is the primary purpose of using generative AI in the field of healthcare?
The primary purpose of using generative AI in healthcare is to leverage advanced algorithms to generate valuable information, optimize decision-making processes, enhance diagnostics, and contribute to innovations in treatment and drug development.
What are the applications of generative AI in healthcare?
Generative AI finds applications in various healthcare domains, including drug discovery, medical image analysis, personalized medicine, predictive analytics, and genomics. It aids in generating data and insights that can be used to improve patient outcomes and streamline healthcare workflows.
How can generative AI be used in drug design and development?
Generative AI can be used in drug design by predicting molecular structures, optimizing drug properties, and generating novel compounds. It expedites the identification of potential drug candidates and facilitates the exploration of vast chemical space.
In what ways does generative AI contribute to personalized medicine?
Generative AI contributes to personalized medicine by analyzing individual patient data to generate tailored treatment plans, predicting patient responses to specific therapies, and optimizing treatment regimens based on genetic, environmental, and lifestyle factors.
Can generative AI assist in medical imaging analysis?
Yes, generative AI can assist in medical imaging analysis by generating high-quality images, enhancing image resolution, and aiding in the interpretation of medical scans. This helps in early and accurate diagnosis of diseases.
How does generative AI support predictive analytics in healthcare?
Generative AI supports predictive analytics by generating models that can predict disease outbreaks, patient readmissions, and treatment responses. This aids healthcare providers in proactive decision-making and resource allocation.
What role does generative AI play in genomics research?
Generative AI contributes to genomics research by analyzing vast genomic datasets, predicting genetic variations, and generating synthetic genomic data. This assists researchers in understanding complex genetic relationships and uncovering potential therapeutic targets.
How can LeewayHertz contribute to the development of innovative healthcare solutions using AI and technology?
LeewayHertz can aid in the development of cutting-edge healthcare solutions by leveraging its expertise in artificial intelligence, data analytics, and software development. Their capabilities extend to designing and implementing custom solutions, such as health monitoring applications and AI-driven diagnostic tools, to address the evolving needs of the healthcare industry.