Generative AI in finance and banking: The current state and future implications
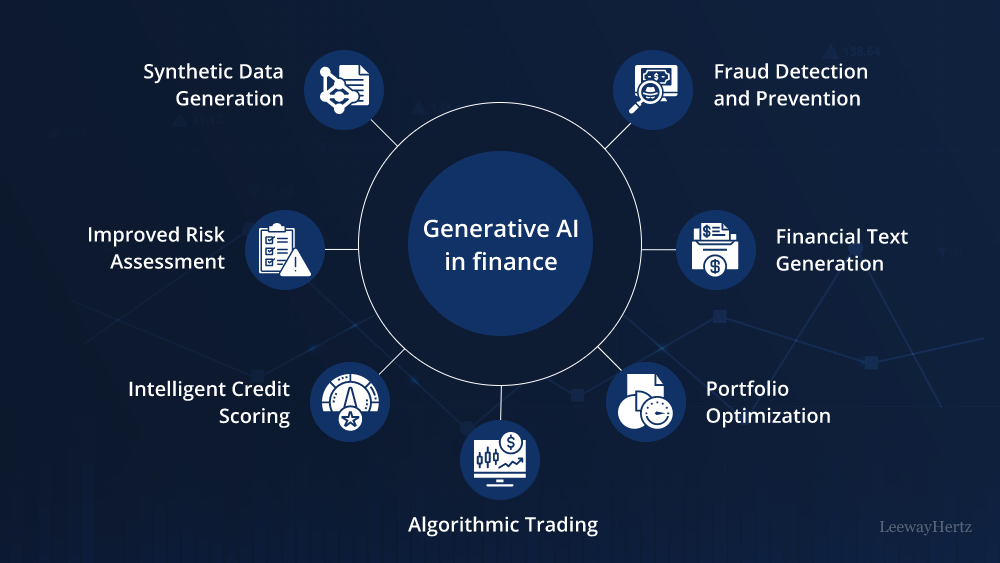
For over a decade, Machine Learning (ML) and Artificial Intelligence (AI) have been instrumental in propelling the financial services industry forward, enabling notable advancements such as better underwriting and improved foundational fraud scores.
While AI has proven beneficial to finance businesses in diverse ways, the finance industry has embraced generative AI and is extensively harnessing its power as an invaluable tool for its operations. While traditional AI/ML is focused on making predictions or classifications based on existing data, generative AI creates novel content by analyzing patterns in existing data. This versatile technology can generate content in a wide range of modalities, including text, images, code, and music, making it ideal for a range of use cases. Its potential to enhance accuracy and efficiency has made it increasingly popular in the finance and banking industries.
Recent statistics highlight the growing adoption of generative AI in finance and banking. According to a report by MarketResearch.biz, the global market size for generative AI in financial services is projected to reach approximately USD 9,475.2 million by 2032, marking a significant growth from USD 847.2 million in 2022. The market is expected to experience a Compound Annual Growth Rate (CAGR) of 28.1% during the forecast period spanning from 2023 to 2032. Financial institutions are recognizing the disruptive potential of generative AI and are actively integrating it into their operations to gain a competitive edge and drive innovation.
This insight offers an overview of generative AI in the finance industry, exploring the specific models leveraged in this field, delving into the applications of generative AI in finance, discussing the ethical considerations and challenges associated with generative AI in the finance industry, and more.
- An overview of generative AI in finance
- Generative AI use cases in banking/financial services
- Fraud detection and prevention
- Personalized customer experience
- Risk assessment and credit scoring
- Investment management
- Customer Service
- Compliance and regulatory reporting
- Cybersecurity and risk management
- Loan underwriting and mortgage approval
- Financial document search and synthesis
- Regulatory code change consultant
- Debt collection
- Automation of back-office operations
- Financial product innovation and design
- Marketing and lead generation
- Customer sentiment analysis
- Treasury operations
- Corporate governance
- Customer onboarding
- Deposits and withdrawals
- Payments and transactions
- Audit and internal controls
- Corporate finance
- How LeewayHertz’s generative AI platform enhances financial operations
- How does generative AI in finance work?
- How to implement AI in banking and finance operations?
- Generative AI models that find application in the finance industry
- Benefits of generative AI in finance and banking
- Ethical considerations and challenges of generative AI in the finance industry
- Future implications and opportunities of generative AI in the finance industry
An overview of generative AI in finance
Generative AI is an advanced type of AI that has the capability to learn from extensive datasets and generate responses based on queries. It possesses the ability to analyze large amounts of existing data, allowing it to identify patterns and trends, which in turn enables it to make informed decisions
Generative AI is fast gaining momentum in the finance industry. It entails using machine learning algorithms to generate new data and valuable insights that can assist in making informed financial decisions. The generative AI application in finance holds the potential to redefine traditional approaches by generating realistic and informative financial scenarios, enhancing portfolio optimization strategies, enabling sophisticated risk simulations and fraud detection and more.
GenAI in the finance industry: Driving factors
Here, we explore some factors responsible for the growing use of generative AI within the finance industry:
Machine learning algorithms advancements: The development of advanced ML algorithms, such as deep learning and reinforcement learning, has led to notable progress in the financial industry. These algorithms allow models to be trained on massive datasets, enabling the generation of highly accurate predictions. As a result, financial institutions are now able to harness the power of generative AI for various applications, such as portfolio optimization and fraud detection.
The growing volume of data: The finance sector produces a substantial volume of data, making it challenging to analyze it using traditional methods. However, generative AI offers a solution for financial institutions to make the most of this data; by employing generative AI techniques, new insights and predictions can be generated, providing valuable information to guide decision-making in the finance industry.
Reducing costs in the financial sector: By automating previously performed manual processes, such as data analysis and fraud detection, financial institutions can enhance their efficiency and lower operational expenses. GenAI in financial services facilitates automation, allowing for streamlined operations and more effective resource allocation, resulting in significant cost savings for financial institutions.
Significance of generative AI in finance
Integrating generative AI in the financial sector brings a range of benefits and opportunities that can reshape various aspects of financial operations. Firstly, generative AI enables the creation of synthetic data that closely resembles real-world financial data. This synthetic data is then utilized to train machine learning models, improving their capability to identify patterns, detect trends, and provide precise predictions. By overcoming limitations associated with real-world data, such as missing data or biased samples, generative AI facilitates more robust and accurate analysis.
Furthermore, generative AI offers automation capabilities that can completely reshape financial processes. It can automate tasks that were previously performed manually, such as data analysis and fraud detection. By automating these processes, financial institutions can enhance operational efficiency, reduce human errors, and significantly lower costs.
Generative AI also empowers financial institutions to analyze large volumes of financial data. It provides valuable insights that can inform investment decisions, risk management strategies, and fraud detection methods. By leveraging generative AI, financial services can gain a competitive edge by making data-driven decisions and staying ahead in the rapidly evolving financial landscape.
The significance of generative AI in financial services lies in its ability to generate synthetic data, automate processes, and provide valuable insights for decision-making. Overall, implementing AI for finance professionals can help reduce their workload, improve efficiency, mitigate risks, and achieve better outcomes.
Optimize Your Operations With AI Agents
Optimize your workflows with ZBrain AI agents that automate tasks and empower smarter, data-driven decisions.
Generative AI use cases in banking/financial services
Generative AI use cases in banking and other financial services are reshaping the landscape of these industries, offering unprecedented advancements. From personalized customer experiences to risk assessment and fraud detection, generative AI is transforming how financial institutions operate and serve their customers. Below are a few significant generative AI use cases in banking and other financial services:
Fraud detection and prevention
Generative AI significantly enhances fraud detection and prevention capabilities in the banking and financial services sector. By analyzing vast amounts of transaction data, customer behavior patterns, and historical fraud cases, generative AI solutions can identify subtle anomalies and fraud trends that might escape traditional rule-based systems. It can flag deviations in real-time, allowing for rapid intervention. Generative AI can also adapt to new fraud tactics by continually learning from incoming data, and staying ahead of evolving threats. Additionally, it can generate realistic fraud scenarios to stress-test existing security measures and help financial institutions proactively strengthen their defenses. This technology’s ability to process and interpret complex, multidimensional data enables highly accurate risk assessments and reduces false positives, improving the overall efficiency of fraud prevention efforts while minimizing disruptions to legitimate customer activities.
Personalized customer experience
Generative AI analyzes vast amounts of customer data, including transaction history, browsing behavior, and demographic information, to create detailed customer profiles. It then uses these insights to generate customized product recommendations, personalized financial advice, and targeted marketing messages. This technology powers intelligent chatbots and virtual assistants that provide 24/7 customer support by answering queries, assisting with transactions, and even offering financial guidance. Additionally, generative AI can create personalized financial reports and forecasts based on historical data, helping customers better understand and manage their finances.
Risk assessment and credit scoring
Generative AI solutions can transform risk assessment and credit scoring in the financial sector by providing highly nuanced and accurate evaluations of creditworthiness. It can analyze a broader range of data points beyond traditional financial metrics, including social media activity, spending patterns, and even the language used in loan applications. This comprehensive approach allows for a more holistic view of an individual’s or business’s financial health and reliability. Generative AI solutions can identify complex relationships between various factors that human analysts might overlook, leading to more precise risk profiles. This technology enables financial institutions to offer more tailored products and interest rates, potentially expanding access to credit while maintaining robust risk management practices. Moreover, generative AI solutions can continuously update assessments based on real-time data, providing dynamic credit scores that reflect current circumstances more accurately than static, periodically updated scores.
Investment management
Generative AI can significantly enhance investment management by automating research and analysis, thereby processing vast amounts of financial data, news articles, and reports to identify trends and provide actionable insights. By identifying intricate patterns and correlations across global markets, generative AI can help fund managers optimize asset allocation and diversification strategies. It can simulate market scenarios, allowing for more robust stress-testing of investment portfolios and better risk management. Generative AI also enables the creation of highly personalized investment strategies tailored to individual client goals, risk tolerances, and preferences. Overall, generative AI in investment banking enables the creation of complex financial models and simulations, improving strategic planning.
Customer Service
Generative AI streamlines customer service by boosting the capabilities of chatbots and virtual assistants in the banking and financial services sector. These AI-powered tools can now engage in natural, context-aware conversations with customers, providing personalized financial advice and support around the clock. They can interpret complex queries, understand nuanced financial terminology, and offer detailed explanations of banking products and services. Generative AI enables virtual assistants to analyze a customer’s financial history and current situation to provide tailored recommendations for savings, investments, or debt management. They can guide users through complex processes such as loan applications or account openings, simplifying these tasks and reducing the need for human intervention. By handling routine inquiries and transactions efficiently, they free up human staff to focus on more complex customer needs, ultimately enhancing overall service quality and operational efficiency.
Compliance and regulatory reporting
In the finance and banking sector, generative AI is transforming compliance and regulatory reporting. This technology analyzes complex regulatory texts, interprets new rules, and generates accurate, compliant reports. It monitors regulatory changes across jurisdictions, alerts compliance teams to updates, and suggests policy adjustments. GenAI automates the creation of compliance documentation and regulatory reports, collating data from various sources and identifying relevant information. It also conducts risk assessments, identifies potential compliance issues, and assists in responding to regulatory inquiries, all while adhering to the strict regulatory requirements of the financial industry.
Cybersecurity and risk management
Generative AI is significantly enhancing cybersecurity and risk management in the financial and banking sectors. By analyzing vast amounts of network traffic, transaction data, and user behavior patterns, generative AI can detect anomalies and potential security threats in real time. It can predict and simulate various cyber attack scenarios, allowing banks to proactively strengthen their defenses against emerging threats. In risk management, generative AI helps financial institutions model complex risk scenarios, considering a wide range of factors, including market volatility, geopolitical events, and regulatory changes. It can generate detailed risk reports and forecasts, enabling more informed decision-making at both operational and strategic levels. Additionally, generative AI enhances fraud detection by creating sophisticated models of normal financial activity and flagging suspicious deviations. It can also assist in regulatory compliance by continuously monitoring transactions for potential violations and generating comprehensive audit trails.
Loan underwriting and mortgage approval
Generative AI streamlines and enhances the traditionally time-consuming and complex task of assessing loan applications. By analyzing a wide array of data points, including financial history, employment records, market trends, and property valuations, generative AI can quickly produce comprehensive risk assessments for potential borrowers.
In mortgage approval, generative AI helps lenders evaluate property values more accurately by considering numerous factors such as location, market conditions, and comparable sales. It can also predict future property value trends, allowing for more informed lending decisions. The technology excels at identifying patterns and relationships in applicant data that human underwriters might miss, potentially uncovering both risks and opportunities.
Generative AI can also automate much of the document processing involved in loan applications, extracting relevant information from various forms and verifying its consistency and accuracy. This significantly reduces processing time and minimizes human error. Moreover, the technology can generate personalized loan terms based on an applicant’s unique financial situation, optimizing factors like interest rates, loan duration, and repayment schedules to best suit both the lender’s risk profile and the borrower’s needs. By automating and enhancing these processes, generative AI not only increases efficiency but also improves the consistency and objectivity of lending decisions, potentially reducing bias in loan approvals.
Financial document search and synthesis
Generative AI significantly enhances the ability to process, analyze, and extract valuable insights from vast repositories of financial documents. In document search, generative AI goes beyond simple keyword matching. It understands context and semantics, allowing users to find relevant information even when their search terms don’t exactly match the document’s text. This semantic search capability is particularly useful when dealing with complex financial terminology and concepts.
For document synthesis, generative AI can quickly summarize lengthy financial reports, contracts, or regulatory filings, distilling key points and presenting them in a concise, easily digestible format. It can also collate information from multiple documents, creating comprehensive overviews of specific financial topics or trends. The technology excels at extracting structured data from unstructured text, such as pulling financial figures from annual reports or identifying key clauses in contracts. This automated extraction saves countless hours of manual data entry and reduces errors.
Generative AI can also assist in creating dynamic, interactive dashboards that update in real time as new documents are added to the system. This allows financial professionals to maintain an up-to-date overview of relevant financial information. Moreover, the technology can identify patterns and trends across large sets of documents, potentially uncovering insights that might be missed by human analysts. For instance, it could track changes in financial reporting language over time, potentially flagging early warning signs of financial distress.
Regulatory code change consultant
Staying compliant with global regulations and adapting to frequent code changes are imperative in the financial services industry. Generative AI steps into the role of a regulatory code change consultant, significantly easing the burden on developers and ensuring swift adaptation to new requirements. By providing summarized answers with links to specific locations containing relevant information, generative AI offers developers valuable context about underlying regulatory or business changes. This facilitates a quicker understanding of the framework modifications necessary for code changes, especially in scenarios like Basel III international banking regulations involving extensive documentation. Moreover, generative AI assists in automating coding changes, ensuring accuracy through human oversight and cross-checking against code repositories. This transformative technology streamlines compliance efforts and enhances documentation processes, offering a proactive approach to regulatory challenges in the financial services sector.
Debt collection
Generative AI redefines debt collection processes by enhancing communication strategies and optimizing customer interactions. Through natural language processing, AI algorithms generate personalized and empathetic messages tailored to individual debtor circumstances. This improves the overall customer experience and increases the likelihood of successful debt resolution. Additionally, AI analyzes vast datasets to identify patterns and predict debtor behavior, enabling proactive and targeted interventions. By automating routine tasks and communication workflows, generative AI allows debt collection agencies to allocate resources more efficiently, reduce operational costs, and streamline the debt recovery process. Furthermore, the technology continuously learns and adapts based on evolving debtor responses, ensuring a dynamic and adaptive approach to debt collection strategies.
Automation of back-office operations
Generative AI is significantly enhancing the automation of back-office operations in the banking and financial services sector. This technology streamlines numerous processes, reducing manual workload and improving efficiency across various departments. In data entry and processing, generative AI can extract information from diverse document types, including handwritten forms, scanned images, and digital files. It accurately populates databases and systems with this information, minimizing human error and speeding up data processing.
For reconciliation tasks, the technology can automatically match and verify transactions across multiple systems, flagging discrepancies for human review. This dramatically reduces the time spent on daily reconciliations and improves accuracy. In reporting, generative AI can compile data from various sources, generate comprehensive reports, and even produce narrative summaries explaining key trends or anomalies. This capability is particularly useful for regulatory reporting and internal performance analysis.
GenAI also enhances customer service operations by automating responses to routine inquiries, processing service requests, and routing complex issues to appropriate human staff. It can analyze customer communication patterns to predict common issues and proactively suggest solutions.
In compliance and audit processes, generative AI can continuously monitor transactions and activities for potential violations, generating alerts and audit trails. It can also assist in preparing documentation for audits, saving significant time and resources. For HR and payroll functions, the technology can automate various tasks such as timesheet processing, leave management, and benefits administration. It can also assist in analyzing employee data to identify trends and support strategic workforce planning.
By automating these back-office operations, generative AI allows financial institutions to reallocate human resources to more complex, value-added tasks, ultimately improving overall operational efficiency and service quality.
Financial product innovation and design
Generative AI stands at the forefront of redefining product innovation and design enhancements within the finance and banking sectors. Leveraging advanced algorithms, financial institutions employ generative design to create innovative products by exploring many possibilities and optimizing for specific criteria. The automation of product ideation and prototyping processes streamlines development cycles, enabling rapid design iterations. Furthermore, generative AI simulates market demand, effectively predicting customer preferences to tailor offerings. In customer-centric approaches, sentiment analysis tools analyze feedback, social media posts, and reviews, providing valuable insights for improving banking services and products. The technology extends beyond practical applications, empowering artists to explore new concepts and generate visual elements. Additionally, through image synthesis, generative AI produces realistic visuals, while text generation models facilitate tasks like article writing, code generation, and conversational agent creation. This comprehensive integration of generative AI fosters innovation, efficiency, and enhanced customer engagement in the dynamic landscape of finance and banking.
Marketing and lead generation
Marketing and lead generation in banking see a transformative boost with the integration of AI, specifically leveraging generative AI. In the fiercely competitive financial landscape, targeted marketing is crucial for attracting new customers, yet the traditional process can be resource-intensive. Here, AI steps in to streamline marketing endeavors by swiftly analyzing customer preferences and online behavior, effectively segmenting leads into distinct groups. Generative AI becomes a valuable ally in this process, contributing to the creation of personalized marketing materials tailored to specific customer segments. Moreover, it plays a crucial role in tracking conversion rates and customer satisfaction, providing insights for continuous improvement. Through A/B testing, banks can evaluate the effectiveness of various strategies, enabling ongoing refinement of marketing approaches. This iterative approach improves the precision of marketing campaigns and fosters a more streamlined and cost-effective lead-generation strategy, ultimately enhancing the return on investment for marketing initiatives over time.
Customer sentiment analysis
Generative AI plays a pivotal role in customer sentiment analysis within the finance sector by scrutinizing social media, news articles, and online content to assess public sentiment concerning particular financial products, services, or market trends. This analytical capability provides valuable insights for making informed investment decisions and refining marketing strategies. By gauging the overall sentiment, financial institutions can swiftly adapt to changing public perceptions, anticipate market shifts, and tailor their approaches to align with customer sentiments. This proactive use of generative AI ensures a more responsive and customer-centric approach, ultimately contributing to more effective decision-making and strategic planning in the dynamic finance landscape.
Treasury operations
Generative AI transforms treasury operations within the financial sector by introducing advanced analytics and automation to optimize cash management, liquidity, and risk. Through the analysis of extensive datasets, generative AI models can forecast cash flows, predict market trends, and identify potential risks, empowering treasury departments to make more informed and strategic decisions. Automation capabilities streamline routine tasks such as transaction processing, reconciliation, and reporting, enhancing operational efficiency. Additionally, generative AI aids in scenario analysis and stress testing, allowing treasury teams to assess the impact of various economic conditions on their portfolios. The technology’s integration into treasury operations improves decision-making processes and contributes to financial institutions’ overall agility and resilience in managing their assets and liabilities effectively.
Corporate governance
Generative AI significantly influences corporate governance within the financial sector by enhancing transparency, accountability, and decision-making processes. Harnessing sophisticated algorithms, generative AI assists in the automated monitoring of compliance, guaranteeing conformity to regulatory norms and minimizing the risks linked to governance lapses. The technology facilitates the analysis of diverse data sources, enabling real-time monitoring of corporate activities and identifying potential areas of improvement. Through automated reporting and analysis, generative AI contributes to more effective board oversight and strategic planning. Moreover, the ability to simulate and predict various governance scenarios enhances risk management, allowing financial institutions to address governance challenges proactively. Generative AI emerges as a transformative force in promoting a culture of ethical conduct, regulatory compliance, and responsible business practices, ultimately reinforcing corporate governance frameworks in the financial industry.
Customer onboarding
Generative AI redefines customer onboarding in the financial sector by introducing efficiency, personalization, and enhanced security to the process. Leveraging advanced algorithms, generative AI automates and accelerates customer identity verification, documentation checks, and compliance procedures, ensuring a seamless and rapid onboarding experience. The technology’s ability to analyze diverse datasets enables the creation of personalized customer profiles, allowing financial institutions to tailor their services and offerings based on individual preferences and needs. Additionally, generative AI enhances security measures through advanced biometric authentication and fraud detection, bolstering the overall integrity of the onboarding process. By optimizing and expediting customer onboarding, generative AI improves operational efficiency for financial institutions and enhances the overall customer experience, fostering trust and satisfaction from the outset of the client relationship.
Deposits and withdrawals
Generative AI significantly transforms deposit and withdrawal services in banking by introducing efficiency and personalized experiences. In deposit services, generative AI automates account opening procedures, expediting the Know Your Customer (KYC) process and ensuring compliance. By employing sophisticated fraud detection algorithms that scrutinize transaction patterns, it reinforces security measures, promptly identifying and preventing unauthorized activities to safeguard deposited funds. For withdrawal services, generative AI streamlines transaction processing by automating routine tasks and tailoring withdrawal recommendations based on individual customer behavior. Furthermore, AI-powered customer support, including chatbots, facilitates seamless navigation of withdrawal channels such as ATMs, branches, and online banking, offering real-time assistance and improving overall customer satisfaction. By leveraging generative AI, financial institutions optimize their operational processes and elevate the security and personalization aspects of depositing and withdrawing funds.
Payments and transactions
Generative AI plays a pivotal role in redefining payments and transactions within the financial landscape. In payment services, generative AI enhances the user experience by facilitating seamless electronic and traditional payment methods, such as wire transfers, online payments, and mobile payments. It employs advanced algorithms for fraud detection, ensuring secure transactions, and safeguarding sensitive financial information. In transaction processing, generative AI optimizes the clearing and settlement of financial transactions by automating and streamlining these processes, thereby improving efficiency and reducing processing times. Through its ability to analyze vast datasets rapidly, generative AI contributes to more accurate and secure financial transactions, fostering a dynamic and technologically advanced ecosystem for payment services.
Audit and internal controls
Generative AI is reshaping audit and internal control processes in the banking and financial services sector, enhancing efficiency, accuracy, and risk management capabilities.
In audit planning, generative AI can analyze historical data, risk assessments, and industry trends to identify high-risk areas that require closer examination. This allows for more targeted and effective audit strategies, optimizing resource allocation. During the audit execution phase, the technology can process vast amounts of financial data, transaction records, and documentation. It can quickly identify anomalies, inconsistencies, or patterns that may indicate errors, fraud, or non-compliance. This capability significantly reduces the time required for data analysis and increases the likelihood of detecting issues that human auditors might miss. Generative AI enhances sampling techniques by creating more representative and risk-focused samples. It can also perform continuous auditing, constantly monitoring transactions and activities in real time, flagging potential issues for immediate review.
In internal controls, GenAI can assess the design and effectiveness of control systems by simulating various scenarios and identifying potential weaknesses. It can generate recommendations for improving control mechanisms based on best practices and regulatory requirements. GenAI assists in creating comprehensive audit reports by summarizing findings, generating visualizations, and even drafting narrative explanations. This streamlines the reporting process and ensures consistency across audit engagements. Generative AI also supports audit follow-ups by tracking the implementation of recommendations and assessing their effectiveness over time. It can generate alerts for overdue actions and provide insights into the progress of remediation efforts.
By automating many aspects of the audit and internal control processes, generative AI allows human auditors to focus on more complex, judgment-based tasks, ultimately improving the overall quality and depth of audit engagements.
Corporate finance
Generative AI in corporate finance is transforming the way organizations manage their financial operations and strategies. By leveraging advanced AI algorithms, companies can automate and optimize complex financial tasks such as forecasting, budgeting, and risk management. AI can rapidly process vast amounts of financial data, identifying trends, anomalies, and potential risks. Furthermore, generative AI can assist in generating financial reports and investor presentations and even draft complex financial documents like merger and acquisition proposals. By automating routine tasks, financial analysts can focus on higher-value strategic initiatives. The potential of generative AI in corporate finance is immense, promising to enhance decision-making, improve efficiency, and unlock new growth opportunities.
Optimize Your Operations With AI Agents
Optimize your workflows with ZBrain AI agents that automate tasks and empower smarter, data-driven decisions.
How LeewayHertz’s generative AI platform enhances financial operations
LeewayHertz’s proprietary generative AI platform, ZBrain, offers significant advantages for the finance and banking sectors. You can leverage it to develop custom applications using advanced Large Language Models (LLMs) and a business’s proprietary data. ZBrain adeptly sources data in diverse forms, including texts, images, and documents, and connects them with LLMs like GPT-4, Llama 3, Gemma, Gemini, and Mistral to create powerful apps that help you refine decision-making, deepen analytical insights, and enhance productivity, all while upholding stringent data privacy standards. It’s an ideal tool for transforming finance and banking operations into smarter, data-driven systems.
One of the main challenges faced by GenAI models is hallucinations, where the model generates incorrect or nonsensical information that seems plausible but is not based on input data or factual reality. ZBrain addresses this issue through its RAG-based architecture, implementation of guardrails, feedback loop, and validation layer. Issues such as complex risk assessment, slow customer service, and inefficient data processing are prevalent in the financial and banking sectors. ZBrain adeptly tackles these challenges with its specialized “Flow” feature, which enables straightforward, no-code development of business logic for apps through its easy-to-use interface. It offers various large language model options, programming logic, helper methods and other third-party tools and platforms to choose from, streamlining the creation and customization of intelligent applications.
This comprehensive approach effectively solves critical challenges faced in the finance and banking industry, ensuring better risk management, faster customer response, and more efficient data handling. Here are a few examples of how ZBrain helps streamline and simplify tasks-
AI-powered financial risk management
ZBrain effectively addresses risk management and analysis challenges in the financial sector. By enabling users to build LLM-based applications, the GenAI platform facilitates risk assessment with accurate prediction and analysis of potential financial risks. This advanced approach leads to highly effective risk management strategies, reducing uncertainties and optimizing decision-making processes. To understand how ZBrain transforms risk management and analysis, explore the detailed Flow process here.
AI-powered operational efficiency analysis
ZBrain adeptly tackles operational efficiency challenges in the financial sector. Robust LLM-based applications built on ZBrain facilitate thorough analyses of operational processes and the identification of areas that need improvement. The apps’ advanced capabilities enhance process optimization, resulting in significant operational cost savings, reduced inefficiencies, and increased overall productivity. To understand how ZBrain transforms operational efficiency through AI-driven analysis and offers tangible benefits to businesses, you can delve into the specific Flow detailed on this page.
AI-driven contract analysis
ZBrain has effectively transformed contract analysis through its GenAI applications. ZBrain’s LLM-based apps streamline the process of scrutinizing and understanding complex contractual documents. This innovation results in considerable time savings, reduces the potential for human error, and enhances the accuracy of contract interpretations. By implementing ZBrain, businesses benefit from more efficient and accurate contract analysis, leading to improved compliance, risk management, and decision-making. For a detailed insight into how ZBrain transforms contract analysis with its GenAI apps, you can explore the specific Flow described on this page.
AI-driven customer churn analysis
ZBrain effectively addresses customer churn analysis challenges in finance. With its LLM-based apps, ZBrain provides in-depth insights into customer behavior and churn patterns. This enables businesses to identify and address factors that lead to customer attrition. The benefits of implementing ZBrain include improved customer retention strategies, enhanced understanding of customer needs, and, ultimately, increased customer loyalty and satisfaction. For a closer look at how ZBrain empowers businesses with advanced churn analysis and helps maintain a robust customer base, you can check out the detailed Flow on this page.
AI-powered competitor analysis
ZBrain tackles the challenge of competitor analysis for businesses in the finance and banking sectors. It enables you to create custom LLM-based applications that facilitate comprehensive and insightful analysis of competitors. This gives companies a strategic advantage with detailed insights into market trends, competitor strategies, and performance metrics. The integration of ZBrain apps into workflows leads to enhanced market understanding, better strategic planning, and improved competitive positioning. For an in-depth view of how ZBrain streamlines competitor analysis, offering significant benefits in understanding and responding to market dynamics, you can explore the specific Flow process on this page.
AI-enhanced budget analysis
ZBrain has innovatively addressed budget analysis challenges across financial sectors. With its LLM-based apps, ZBrain enhances the accuracy and efficiency of budget analysis. The apps aid businesses in optimizing their budget allocation, identifying cost-saving opportunities, and making data-driven financial decisions. The implementation of ZBrain apps into workflows results in improved financial planning, reduced expenditures, and enhanced overall fiscal management. To gain a comprehensive understanding of how ZBrain transforms budget analysis and contributes to effective financial strategies, you can go through the detailed Flow available on this page.
ZBrain’s contract analysis and optimization tool: A real-world example of AI in contract analysis
LeewayHertz’s AI-powered contract analysis tool, built on ZBrain, equips your negotiation teams with rapid contract analysis capabilities.
The tool enables users to quickly analyze and compare contracts, identifying shortcomings and opportunities for strategic modifications aligned with organizational objectives.
Key features of the contract analysis tool
Advanced contract analysis: The tool offers advanced contract analysis capabilities, allowing users to easily examine different versions of contracts, detect edits made to clauses, and evaluate how these changes might affect the negotiation process.
Issue identification: The solution automatically creates a list of issues within contracts for review. This feature allows users to address potential problems and make updates to clauses in a proactive manner. ZBrain ensures that a human reviewer is involved in the process before sending the contract back to the opposing counsel. This helps streamline negotiation processes, making them more efficient and effective.
Customize any contract: ZBrain’s solution is highly adaptable and can be configured to accommodate various contract types. Its flexible architecture ensures proper clause segmentation and tailored application to meet the unique needs of your organization.
The benefits of ZBrain’s contract analysis solution
- Swift contract analysis: The solution enables rapid analysis of contracts, saving time and effort for negotiation teams.
- Enhanced efficiency: The solution streamlines the contract review process with automated issue list generation, facilitating proactive mitigation and clause updates.
- Improved decision-making: By identifying shortcomings and opportunities within contracts, the tool helps negotiation teams make informed decisions that align with organizational objectives.
- Customization: The tool adapts to various contract types, ensuring proper clause segmentation and tailored application to meet the unique needs of each organization.
- Efficient negotiation processes: ZBrain promotes efficient negotiation processes by ensuring human-in-the-loop review before sending contracts back to opposing counsel.
- Cost reduction: By automating certain aspects of contract analysis, the tool helps reduce manual effort and associated costs, leading to overall savings for the organization.
How does generative AI in finance work?
Incorporating generative AI into financial processes involves multiple components to streamline data analysis, generate insights, and support decision-making. It enhances traditional financial operations by integrating powerful LLMs with an organization’s unique knowledge base. This architecture generates valuable insights, enabling financial institutions and individuals to make informed, real-time decisions.
Here’s a detailed breakdown of how generative AI works in finance:
Data sources: The process starts with gathering data from various relevant sources, including:
- Market data: Information on stock prices, indices, commodities, and market trends from financial markets and stock exchanges.
- Company financials: Financial statements, earnings reports, and forecasts from public companies.
- Customer data: Information about customer transactions, account balances, credit histories, and spending patterns from banks and financial institutions.
- News and social media: Real-time news, social media trends, and sentiment analysis from platforms like Bloomberg, Reuters, and Twitter.
Data pipelines: The gathered data is routed through data pipelines responsible for ingestion, cleaning, and structuring the data, making it ready for further analysis.
Embedding model: The prepared data is then processed by an embedding model, which transforms textual data into numerical representations (vectors) that AI models can understand. Popular models include those from OpenAI, Google, and Cohere.
Vector database: The generated vectors are stored in a vector database, allowing for efficient querying and retrieval. Examples of vector databases include Pinecone, Weaviate, and PGvector.
APIs and plugins: APIs and plugins like Serp, Zapier, and Wolfram connect different components and enable additional functionalities, such as accessing extra data or performing specific tasks with ease.
Orchestration layer: The orchestration layer manages the workflow. Tools like ZBrain are examples of this layer, which simplifies prompt chaining, manages interactions with external APIs, retrieves contextual data from vector databases, and maintains memory across multiple LLM calls. This layer generates prompts or a series of prompts for processing by a language model. It ensures seamless coordination across all components within the architecture.
Query execution: The data retrieval and generation process is triggered when the user submits a query to the financial app. This query can be about anything relevant to finance and banking, such as stock price fluctuations or evaluating investment options.
LLM processing: The app transmits the query to the orchestration layer, which retrieves relevant data from the vector database and LLM Cache and sends it to the appropriate LLM. The choice of LLM depends on the nature of the query.
Output: The LLM generates an output based on the query and the received data. This output can take various forms, such as a summary of stock price fluctuations, investment recommendations, or financial insights.
Financial app: The validated output is then presented to the user through the financial app. This core application consolidates all the data, analysis, and insights, presenting them in a user-friendly format for financial professionals and individual investors.
Feedback loop: User feedback on the LLM’s output helps improve the accuracy and relevance of future outputs, forming a crucial part of the architecture.
AI agents: AI agents handle complex problems, interact with the external environment, and enhance learning through post-deployment experiences. They achieve this purpose by using advanced reasoning/planning, strategic tool utilization and leveraging memory, recursion, and self-reflection.
LLM cache: Tools like Redis, SQLite, or GPTCache are employed to cache frequently accessed information, speeding up the AI system’s response time.
Logging/LLMOps: LLMOps tools like Weights & Biases, MLflow, Helicone, and Prompt Layer log actions and monitor performance, ensuring LLMs function optimally and improve performance through feedback loops.
Validation: A validation layer is incorporated to ensure the LLM’s output is accurate and reliable, using tools like Guardrails, Rebuff, Guidance, and LMQL.
LLM APIs and hosting: LLM APIs and hosting platforms execute financial tasks and host applications. Depending on requirements, developers can select from LLM APIs offered by companies like OpenAI and Anthropic or use open-source models. Hosting platforms include AWS, GCP, Azure, and Coreweave, or opinionated clouds like Databricks, Mosaic, and Anyscale.
This structured flow demonstrates how generative AI in finance leverages various data sources and technological tools to generate accurate and actionable insights, automate financial tasks, and provide a personalized and optimized financial experience.
Explore ZBrain’s Finance and Banking use cases
ZBrain finds widespread applicability in Finance and Banking, performing diverse critical functions. The following highlights key use cases of this GenAI platform within the Finance and Banking industry.
How to implement AI in banking and finance operations?
Implementing AI in banking and finance operations can significantly improve efficiency, accuracy, and customer satisfaction. Here is a guide on how to implement AI in the banking and finance sector:
Define objectives and use cases:
- Clearly identify the business objectives and challenges that AI can address.
- Identify specific use cases where AI can provide the most value, such as fraud detection, customer service automation, risk management, and personalized financial advice.
Data quality and integration:
- Ensure that your data is of high quality, well-organized, and accessible. AI algorithms heavily rely on data and the success of your implementation hinges on the quality of the input.
- Integrate data from various sources within the organization, such as transaction records, customer profiles, and external data sources.
Machine learning models:
- Choose appropriate machine learning models for your use cases. For fraud detection, anomaly detection algorithms can be useful. Natural language processing models can enhance chatbots and virtual assistants for customer service.
- Train your models using historical data and continuously update them to adapt to changing patterns and trends.
Robotic Process Automation (RPA):
- Implement RPA to automate repetitive and rule-based tasks, such as data entry, document processing, and compliance checks.
- Streamline workflows to reduce operational costs and enhance efficiency.
Chatbots and virtual assistants:
- Deploy AI-powered chatbots to handle customer queries and provide real-time assistance. This can improve customer service by providing instant responses and freeing up human agents for more complex tasks.
- Utilize machine learning to train chatbots, elevating their capacity to understand and address customer inquiries effectively.
Fraud detection and security:
- Utilize AI algorithms to detect unusual patterns and anomalies in transaction data, helping identify potential fraudulent activities.
- Implement biometric authentication methods, like fingerprint or facial recognition, to enhance security for customer transactions.
Regulatory compliance:
- Use AI to streamline regulatory compliance processes. AI systems can help monitor and ensure adherence to changing regulations, reducing the risk of non-compliance.
- Implement algorithms that can automatically analyze and interpret regulatory texts to keep your organization updated on compliance requirements.
Customer insights and personalization:
- Leverage the power of AI to analyze customer behavior and preferences. Utilize this information to offer personalized product recommendations, deploy targeted marketing campaigns, and provide tailored services.
- Implement machine learning models for customer segmentation and predictive analytics to anticipate customer needs.
Continuous monitoring and improvement:
- Evaluate the performance of your AI systems and routinely gather feedback from users. This feedback loop is crucial for identifying areas of improvement and ensuring that the AI solutions align with business goals.
- Stay abreast of the latest advancements in AI technology and continuously update your systems to leverage the latest capabilities.
Collaboration and training:
- Encourage a collaborative environment among data scientists, domain experts, and IT professionals to guarantee a comprehensive approach to implementing AI.
- Provide training programs for staff to enhance their understanding of AI technologies and foster a culture of innovation and continuous learning.
If you are looking for a tech partner, LeewayHertz is your trusted ally, offering generative AI consulting and development services to propel your finance business into the digital forefront. With a proven track record in deploying diverse advanced LLM models and solutions, LeewayHertz helps you kickstart or further your AI journey.
Generative AI models that find application in the finance industry
There are several generative AI models that are commonly used in the finance sector. Some of the prominent ones include:
Variational Autoencoders (VAEs)
Variational Autoencoders (VAEs) are generative AI models that are widely used in the finance sector. VAEs are designed to learn the underlying structure of the input data and generate new samples that closely resemble the original data distribution. In the context of finance, VAEs work by encoding the input financial data into a lower-dimensional latent space representation. This latent representation captures the essential features and patterns of the data. The encoded data is then decoded back into the original data space, reconstructing the input data.
The training process of VAEs involves two main components: the encoder and the decoder. The encoder maps the input financial data to a latent space, typically using probabilistic techniques. The encoder learns to generate a mean and variance for each dimension of the latent space, which represents the probability distribution of the latent variables given the input data. The decoder takes samples from the latent space and reconstructs them back into the original data space. It learns to generate outputs that resemble the input data as closely as possible. The reconstruction process allows VAEs to generate new samples that resemble the original data distribution while introducing variations.
The training of VAEs involves optimizing two objectives: reconstruction loss and the Kullback-Leibler (KL) divergence. The reconstruction loss measures the difference between the input data and the reconstructed data, encouraging the model to generate accurate representations. The KL divergence helps regularize the latent space by encouraging it to follow a prior distribution, typically a standard normal distribution. This regularization promotes the generation of diverse and meaningful samples.
In finance, VAEs find applications in various areas, including:
- Portfolio optimization: VAEs can learn the underlying structure of historical market data and generate new investment portfolios.
- Anomaly detection: VAEs can identify abnormal patterns in financial transactions or market behavior.
- Risk modeling: VAEs can be utilized to model and assess risks in financial systems.
- Fraud detection: VAEs can help detect fraudulent activities in financial transactions.
- Synthetic data generation: VAEs can generate synthetic financial data to overcome limitations in real-world datasets.
By leveraging the capabilities of VAEs, financial institutions can gain insights, generate new data samples, and improve decision-making processes based on the learned representations and generated outputs.
Generative Adversarial Networks (GANs)
GANs are used in finance for tasks like synthetic data generation, market simulation, and improving risk modeling. Generative Adversarial Networks (GANs) are a type of generative AI model that consists of two components: a generator and a discriminator. GANs have gained significant popularity and application in the finance sector due to their ability to generate synthetic data and improve various financial tasks.
The generator in a GAN learns to create new samples that resemble real financial data, such as stock prices, transaction records, or market indicators. It takes random noise as input and tries to generate data that is indistinguishable from real financial data. The discriminator, on the other hand, is trained to differentiate between real and generated data. It learns to identify the distinguishing characteristics of real financial data and aims to classify the generated samples as fake.
During training, the generator and discriminator are trained in an adversarial manner. The generator’s objective is to fool the discriminator by producing samples that are increasingly similar to real data, while the discriminator’s objective is to become more accurate in distinguishing real from generated data. As the training progresses, the generator improves in generating more realistic financial data, and the discriminator becomes more adept at differentiating real from fake samples.
Applications of GANs in finance include:
- Financial fraud detection: GANs can help distinguish between legitimate and fraudulent transactions, enhancing fraud detection in the financial sector.
- Market simulation and scenario analysis: GANs can generate artificial market data, assisting in understanding market dynamics, predicting price movements, and evaluating the impact of different factors on financial markets.
- Anomaly detection: GANs can identify unusual patterns or outliers in financial data.
GANs have emerged as a powerful tool for credit card fraud detection, particularly in handling imbalanced class problems. Compared to other machine learning approaches, GANs offer better performance and robustness due to their ability to understand hidden data structures. Ngwenduna and Mbuvha conducted an empirical study highlighting the effectiveness of GANs and their superiority over other sampling models. They also compared GANs with resampling methods like SMOTE, showing GANs’ superior performance.
Additionally, Kim et al. utilized CTAB-GAN, a conditional GAN-based tabular data generator, to generate synthetic data for credit card transactions, outperforming previous approaches. Saqlain et al. employed a Generative Adversarial Fusion Network (IGAFN) to detect fraud in imbalanced credit card transactions. IGAFN integrated heterogeneous credit data, addressing the data imbalance issue and outperforming other methods in credit scoring. These studies demonstrate GANs’ efficacy in credit card fraud detection and their potential for enhancing risk assessment in the financial sector.
Autoregressive Models
Autoregressive models are a class of time series models commonly used in finance for analysis and forecasting. These models capture the temporal dependencies and patterns in sequential data. Autoregressive models work on the principle that the value of a variable at a certain time is dependent on its previous values.
Autoregressive models, including autoregressive moving average (ARMA) and autoregressive integrated moving average (ARIMA), work by considering the relationship between an observation and a lagged set of observations. The core concept is that the value of a variable at a particular time can be predicted using a linear combination of its past values and possibly some noise term.
In an autoregressive model, the “autoregressive” part refers to the dependence on lagged values of the variable itself. The model assigns weights to these lagged values based on their importance in predicting the current value. The “moving average” part, in the case of ARMA models, refers to the dependence on past forecast errors or residuals.
Autoregressive models are typically estimated using historical data to minimize the difference between the actual observations and the predicted values.
Applications of autoregressive models in finance:
- Time series forecasting: Autoregressive models can predict future values of financial variables based on their past values. They are used for predicting stock prices, interest rates, exchange rates, and other financial indicators.
- Risk management and portfolio optimization: Autoregressive models help model the volatility and correlations of asset returns, aiding in risk assessment and portfolio optimization.
One advantage of autoregressive models is their interpretability, as the model coefficients provide insights into the historical relationships between variables. However, autoregressive models assume stationarity, meaning that the statistical properties of the data remain constant over time. Therefore, it is important to assess the stationarity of the data and possibly apply transformations or consider more sophisticated models, such as ARIMA, which incorporates differencing to address non-stationarity.
Transformer Models
A transformer is a specific type of neural network architecture that has gained popularity for its ability to process sequential data, like text, more efficiently. They are known for their capability to capture long-range dependencies and effectively process sequential data. In the context of finance, transformer models have been applied to tasks such as sentiment analysis, document classification, and financial text generation.
Unlike traditional Recurrent Neural Networks (RNNs), transformers use self-attention mechanisms to capture dependencies between different words in a sentence, allowing them to understand contextual relationships more effectively. This architecture has proven highly effective in various natural language processing tasks, enabling improved machine translation, language generation, and other text-based applications.
The core component of a transformer model is the attention mechanism. Attention allows the model to assign different weights or importance to different parts of the input sequence when generating representations. It enables the model to focus on relevant information and effectively capture dependencies between elements.
Transformer models consist of an encoder and a decoder. The encoder processes the input sequence, such as financial text data, and generates contextualized representations for each element. The decoder takes these representations and produces output sequences, often used in tasks like language translation or text generation.
Applications of transformer models in finance:
- Sentiment analysis: Transformer models can understand the sentiment or opinion expressed in financial news, social media posts, and other textual data. They capture context and word dependencies to provide insights into market sentiment, supporting investment decisions.
- Document classification: Transformer models are used to classify financial reports, research papers, and other textual documents into predefined categories. This helps in organizing and filtering large amounts of financial information.
- Financial text generation: Transformer models can generate synthetic financial reports, market commentaries, and other relevant text. They learn to generate text based on patterns and structures observed in financial data, offering opportunities for automated report generation and content creation.
Optimize Your Operations With AI Agents
Optimize your workflows with ZBrain AI agents that automate tasks and empower smarter, data-driven decisions.
Benefits of generative AI in finance and banking
Generative AI is rapidly transforming the financial landscape, offering a range of benefits that enhance efficiency, accuracy, and innovation across various financial domains.
- Automation and efficiency
Generative AI streamlines processes by automating repetitive tasks, freeing up human resources for more strategic initiatives. For instance, AI can automatically generate financial reports, contracts, and other documents, ensuring consistency and accuracy while reducing manual effort and errors. This automation extends to tasks like data entry and compliance checks, improving operational efficiency and reducing costs.
- Enhanced data insights and analytics
Generative AI excels at analyzing vast datasets, uncovering hidden patterns and trends that might escape human analysts. This ability leads to more accurate financial forecasting, risk assessment, and market analysis. AI algorithms can also identify unusual patterns in transactions, facilitating early fraud detection and prevention.
- Improved customer experience
Generative AI empowers financial institutions to provide a more personalized and engaging customer experience. AI-powered chatbots can offer 24/7 support, answering customer queries and providing tailored financial advice based on individual needs.
- Innovation and new opportunities
Generative AI opens doors to novel financial applications and opportunities. For example, developers can create sophisticated AI algorithms for portfolio management, leveraging real-time market data to identify profitable opportunities. It can also be used to develop advanced risk management models, enabling institutions to better assess and mitigate financial risks. Furthermore, AI can analyze market trends and customer needs to develop innovative financial products and services.
- Cost savings and increased profitability
The efficiency gains and improved decision-making facilitated by generative AI lead to significant cost savings. Automation reduces labor costs, while improved data insights enable better resource allocation and risk management. These benefits ultimately contribute to increased profitability. By improving customer experiences and developing innovative products, generative AI can also drive customer acquisition and retention, further boosting revenue.
Ethical considerations and challenges of generative AI in the finance industry
The rapid advancements in generative AI raise important questions about how we can best leverage this technology in an ethical manner. In various sectors like the financial services industry, it’s no longer just about what we can do with generative AI; it’s also about what we should do and when.
1. Ethical considerations: Generative AI models could generate outputs that are biased or discriminatory due to biases existing in their training data, making their decision-making processes unfair. It is crucial for financial institutions to prioritize ethical considerations and take necessary measures to ensure that generative AI models make decisions that are fair, transparent, and unbiased.
2. Data privacy and security: Financial institutions handle sensitive and confidential data, such as personal identification details, account balances, and transaction history. Safeguarding the privacy and security of this information is of utmost importance. However, training a generative AI model using such data carries the risk of unintentional disclosure or misuse of sensitive information.
3. Model output accuracy: Given the impact an answer to a financial question can have on individuals, companies, and society, these new AI models need to be as accurate as possible. They can’t hallucinate or make up wrong but confident-sounding answers to critical questions about one’s taxes or financial health, and they need to be far more accurate than the approximate answers for popular culture queries or generic high school essays. It’s best to have a human in the loop for the final verification of an AI-generated answer.
4. Talent and expertise gap: Building and deploying generative AI models require specific expertise from both AI and finance fields, making recruitment of the required talent challenging for financial institutions. Bridging this talent gap by cultivating collaboration among data scientists, AI specialists, and finance professionals is paramount for generative AI’s successful implementation.
5. Scalability and integration: Integrating generative AI solutions across an entire financial institution can be challenging, while seamless scalability must not disrupt ongoing operations or compromise ongoing efforts to integrate systems. Careful planning must take place for seamless scalability without disrupting ongoing operations.
6. Regulatory compliance: Due to strict regulations and the need to safeguard sensitive customer information and maintain ethical standards (such as AML, GDPR, and KYC), AI systems in the financial services sector must comply with these rules and regulations. But generative AI models may not always meet these requirements, exposing companies to legal and compliance risks. Additionally, new regulations around Consumer Duty will increase the burden on financial services providers to show due care and prove they have acted in the best interest of their customers.
Despite being a relatively new technology with social and ethical challenges to address, generative AI has already made significant strides and gained a strong foothold in various industries.
Future implications and opportunities of generative AI in the finance industry
Future implications of generative AI in finance encompass a range of possibilities that can shape the industry in significant ways. Some potential implications include:
- Data augmentation and analysis: Generative AI will transform data augmentation and analysis in the future. By generating synthetic data and augmenting existing datasets, generative AI will overcome limitations such as data scarcity or bias. This will enable financial institutions to train more accurate and robust machine learning models, conduct comprehensive data analysis, and unlock valuable insights. With enhanced data capabilities, institutions will gain a deeper understanding of customer behavior, market dynamics, and risk factors, empowering them to make data-driven decisions with confidence.
- Explainable AI (XAI) in regulatory compliance: As regulatory requirements continue to increase, the demand for explainable AI models in regulatory compliance is expected to rise. Financial institutions will likely prioritize AI solutions that provide transparent insights into decision-making processes, ensuring accountability and compliance with regulatory standards.
- Blockchain and GenAI interplay: Together, these technologies promise enhanced security, transparency, and efficiency for finance businesses. While generative AI will streamline complex tasks like risk assessment and fraud detection, blockchain ensures secure, transparent, and decentralized transactions. This synergy will optimize processes, reduce costs, and enhance overall operational efficiency, ushering in a new era of trust and innovation in the financial landscape.
- AI-driven ESG (Environmental, Social, and Governance) investing: Generative AI models may play a role in analyzing and integrating ESG factors into investment strategies. This could involve assessing the environmental impact of investments, evaluating social responsibility, and considering governance practices in decision-making processes.
Final thoughts
It’s safe to say that where there’s innovation, there’s a flurry of activity in the bid to stay ahead and stand apart. Every day comes with new announcements, and going forward, we will definitely see more of such applications of generative AI in financial services and beyond.
Generative AI is greatly impacting the finance industry by generating synthetic data, automating processes, and providing valuable insights for decision-making. It overcomes the limitations of real-world data and enables personalized consumer experiences, improved risk assessment, fraud detection, and smarter investment management. Advancements in machine learning algorithms, the growing volume of data, and the need for cost savings are driving the widespread adoption of generative AI in finance and banking. Variational Autoencoders (VAEs), Autoregressive Models, Recurrent Neural Networks (RNNs), and Transformer models are some of the generative AI models used in finance/banking. These models are utilized for tasks like personalized consumer experiences, synthetic data generation, risk assessment, fraud detection, investment management, and portfolio optimization. Embracing generative AI empowers financial institutions to make data-driven decisions, enhance operational efficiency, and stay ahead in the dynamic financial landscape.
Ready to embrace the power of generative AI? Contact LeewayHertz, and our expert team will help you harness the power of Generative AI to improve your business processes.
Start a conversation by filling the form
All information will be kept confidential.
FAQs
How can generative AI benefit the finance and banking industry?
What are some important driving factors behind the increasing adoption of generative AI in the finance industry?
The adoption of generative AI in finance is driven by its potential to improve accuracy in tasks such as underwriting and fraud detection, provide a competitive edge, and drive innovation. The technology’s versatility in generating diverse content contributes to its growing significance.
What generative AI models are commonly used to enhance operations in finance businesses?
Several generative AI models find application in finance, including Variational Autoencoders (VAEs), Generative Adversarial Networks (GANs), Autoregressive Models, and Transformer Models. Each model has unique strengths that cater to specific use cases in the finance sector.
How does generative AI contribute to fraud detection in the financial sector?
Generative AI enhances fraud detection by analyzing patterns, anomalies, and historical data. It has the capability to detect uncommon transactions or behaviors, adding an extra layer of security to prevent and address fraudulent activities in real-time proactively.
What ethical considerations and challenges are associated with the adoption of generative AI in the finance industry?
The adoption of generative AI in finance raises ethical considerations related to data privacy, bias in generated content, and transparency in decision-making. Challenges include addressing these ethical concerns, ensuring model interpretability, and navigating regulatory frameworks in the finance sector.
How can LeewayHertz assist my financial business in leveraging generative AI for innovation and competitive advantage?
LeewayHertz specializes in tailoring generative AI solutions for financial companies of all sizes. We focus on innovation, providing personalized services, and enhancing competitive advantage through advanced risk assessment, fraud detection, and customer engagement applications.
Is it possible to integrate your generative AI solutions into the existing workflows of my financial business?
LeewayHertz ensures flexible integration of generative AI into clients’ existing systems. Our solutions exhibit adaptability and can be customized to meet the specific needs of financial enterprises. This tailored approach minimizes disruptions and maximizes Return on Investment (ROI).
Can LeewayHertz customize generative AI applications to address unique challenges my finance business faces?
Absolutely. LeewayHertz specializes in customizing generative AI applications to address the unique challenges faced by your finance business. Whether it’s risk management, customer retention, or other specific needs, our solutions are tailored to maximize efficiency and effectiveness.
How does LeewayHertz ensure the security of sensitive financial data when implementing generative AI applications for banking clients?
Security is a top priority at LeewayHertz. We employ strong encryption, implement access controls, and ensure compliance with data protection regulations to secure sensitive financial data in generative AI applications. This comprehensive approach safeguards the confidentiality and integrity of financial information.
Does LeewayHertz provide ongoing support after the implementation of generative AI applications?
LeewayHertz is committed to delivering comprehensive services, extending support well beyond the initial implementation phase for generative AI applications. With a dedicated focus on client success, LeewayHertz ensures the seamless integration and continuous functionality of generative AI solutions. Their post-implementation support encompasses ongoing assistance, updates, and troubleshooting to address any evolving needs or challenges that may arise. This commitment reflects LeewayHertz’s dedication to providing a holistic and enduring partnership with clients in harnessing the full potential of generative AI technologies.
- An overview of generative AI in finance
- Generative AI use cases in banking/financial services
- How LeewayHertz’s generative AI platform enhances financial operations
- How does generative AI in finance work?
- How to implement AI in banking and finance operations?
- Generative AI models that find application in the finance industry
- Benefits of generative AI in finance and banking
- Ethical considerations and challenges of generative AI in the finance industry
- Future implications and opportunities of generative AI in the finance industry
- Contact us