Generative AI in asset management: Use cases, benefits, architecture, solution and development
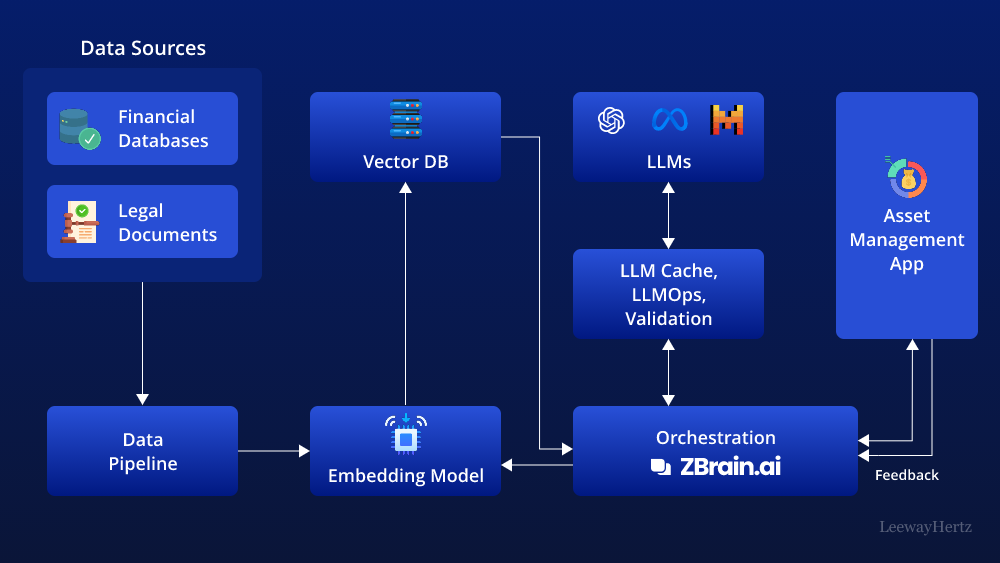
In the dynamic world of finance, where every decision can be the difference between profit and loss, Generative Artificial Intelligence (AI) is not just a tool but a game-changer. Picture this: a technology that can sift through mountains of financial data, predict market trends with uncanny accuracy, and even simulate hypothetical scenarios to guide asset managers in making optimal decisions. That is the power of generative AI in asset management – a force redefining the very essence of financial decision-making.
According to a recent market analysis, the generative AI in the asset management market is poised for exponential growth. Its size is expected to soar from a modest USD 312 million in 2022 to an astounding USD 1,701 million by 2032. This significant expansion is anticipated to take place at an astounding Compound Annual Growth Rate of 19% throughout the forecast period spanning from 2022 to 2032, highlighting the industry’s acknowledgment of its disruptive potential. Traditional methods of asset management, while effective to a degree, often grapple with the sheer complexity and volatility of today’s markets. The finance industry has long relied on historical data and human expertise to steer investments, but this approach has its limitations. It is in this context that generative AI emerges as a transformative ally, blending the best of human insight with the computational prowess of machine learning.
Imagine a technology that does not just analyze past financial data but creates entirely new insights and predictive models. Generative AI accomplishes this by leveraging advanced techniques like Generative Adversarial Networks (GANs) and Reinforcement Learning to simulate market conditions and optimize portfolios. This means that asset managers can not only respond to market changes but anticipate and harness them, all with unprecedented precision.
But that’s not all. Generative AI does not just stop at structured financial data; it embraces the unstructured as well. It scours news articles, gauges social media sentiment, painting a holistic picture that traditional models often miss. This comprehensive data analysis equips asset managers to make smarter decisions, adapt to ever-changing market dynamics, and capitalize on emerging trends in real-time.
In this rapidly evolving landscape, generative AI is not replacing human expertise; it’s empowering it. It is automating mundane tasks, allowing asset managers to focus on strategic planning, ethical considerations, and high-level decision-making. It’s forging a partnership where human judgment and AI-driven insights combine, creating a potent force that is redefining the future of asset management.
This article delves into the transformative realm of generative AI in asset management. It explores the benefits, challenges, real-world applications, and emerging trends of generative AI, shedding light on how this technology is reshaping financial decision-making and portfolio optimization.
- An overview of asset management
- How does generative AI in asset management work?
- Generative AI use cases in asset management
- Portfolio optimization and generation
- Predictive analytics
- Scenario analysis
- Asset allocation optimization
- Economic forecasting
- Customized investment strategies
- Fraud detection
- Operational efficiency
- Volatility modeling
- Client communication
- Performance monitoring
- Market research and analysis
- ESG monitoring
- Automated customer support
- Risk management
- Decision support
- Knowledge management
- How does LeewayHertz’s generative AI platform optimize asset management workflows?
- LeewayHertz’s AI development services for asset management
- What are the challenges faced in the asset management workflow, and how does AI address those challenges?
- Enterprise requirements for integrating generative AI in asset management
- How to implement generative AI in asset management?
- Benefits of generative AI in asset management
- Generative AI models used in asset management
- Emerging trends in generative AI for asset management
An overview of asset management
Asset management is a critical financial discipline that involves the strategic management and monitoring of various types of assets owned by individuals, organizations, or institutions. These assets can encompass a wide range of investments, including stocks, bonds, real estate, commodities, and more. The fundamental aim of asset management is to optimize the performance of these assets while minimizing risk, in line with the investor’s financial objectives and risk tolerance.
Key elements of asset management include diversification, risk management, portfolio allocation, research and analysis, active vs. passive management, a long-term perspective, a client-centric approach, technology integration, and compliance with regulatory frameworks to ensure transparency and fairness. This personalized approach helps tailor investment strategies to individual needs, with technology integration playing a pivotal role in modern asset management.
Key drivers for the adoption of generative AI in asset management
Generative AI is gaining traction in asset management due to several compelling drivers that make it a valuable tool for improving decision-making, optimizing portfolios, and enhancing operational efficiency within the financial industry. These drivers include:
- Data abundance: The financial industry generates vast amounts of data daily, from market data to news articles and social media sentiment. Generative AI can process and analyze this data at scale, extracting valuable insights and patterns that human analysts might miss.
- Complexity of financial markets: Financial markets are becoming increasingly complex, with numerous asset classes, and global economic factors at play. Generative AI can navigate this complexity by simulating various scenarios, optimizing portfolios, and identifying investment opportunities.
- Real-time decision-making: In today’s fast-paced markets, real-time decision-making is critical. Generative AI can process and analyze data in real-time, allowing asset managers to respond swiftly to market developments and execute trades with precision.
- Alternative data sources: Generative AI has the capacity to analyze diverse data sources like social media sentiment, satellite imagery, and geolocation data. This equips asset managers with distinctive insights into investment opportunities and potential risks.
- Risk management: Risk is inherent in asset management, and generative AI can help identify, assess, and mitigate risks more effectively. It can model various risk scenarios, stress test portfolios, and provide early warnings of potential market downturns.
- Alpha generation: Asset managers are constantly seeking alpha or excess returns above a benchmark index. Generative AI can identify non-obvious alpha-generating opportunities by uncovering hidden patterns and correlations in data.
- Portfolio optimization: Generative AI can optimize portfolios by considering multiple factors, including risk tolerance, investment objectives, and market conditions. This results in well-balanced portfolios that maximize returns while minimizing risk.
- Cost efficiency: By automating routine tasks like data analysis, research, and report generation, generative AI can reduce operational costs for asset management firms. This allows human analysts to focus on higher-level strategic tasks.
- Personalization: Generative AI can provide personalized investment recommendations and strategies based on individual investor profiles and preferences, enhancing the client experience and retention.
- Competitive advantage: As generative AI adoption grows, asset managers who leverage this technology gain a competitive advantage. They can provide superior investment strategies, generate higher returns, and attract more clients.
- Regulatory compliance: Generative AI can assist asset managers in meeting regulatory compliance requirements by providing accurate and auditable records of investment decisions and risk assessments.
- Scalability: Generative AI solutions can scale to accommodate growing volumes of data and expand portfolios, ensuring asset managers can handle increased workloads efficiently.
Asset management combines financial expertise, strategic planning, and a deep understanding of market dynamics with technology integration, particularly generative AI, to make informed decisions that align with an investor’s unique objectives. This integration of technology is driven by the growing complexities of financial markets and the need for data-driven decision-making, positioning asset management as an evolving and dynamic field within the financial industry.
How does generative AI in asset management work?
Integrating generative AI into asset management processes enhances data analysis, derives actionable insights, and facilitates informed decision-making. This transcends conventional methods by leveraging sophisticated AI models, particularly large language models (LLMs), and integrating them with the organization’s proprietary knowledge base. This method opens new possibilities for generating insights and enables businesses to make real-time data-driven decisions confidently.
This architecture leverages various components to streamline the asset management process. Here’s a step-by-step breakdown of how it works:
1 . Data sources: The process begins by gathering data from various sources relevant to the asset management process. This data can include:
- Financial databases: Accessing company financial data from sources like corporate filings, public records, and financial databases to analyze the financial health and performance of assets.
- Market data providers: These companies utilize market data services to gather information on asset prices, market trends, which is essential for making informed investment decisions.
- Legal documents: This includes legal documents such as asset purchase agreements, investment contracts, and regulatory filings to assess legal risks and compliance obligations associated with asset management activities.
- Operational data: Collecting operational data, including asset utilization, maintenance records, and performance metrics, to optimize asset performance and efficiency.
2. Data pipelines: The data gathered from the above sources undergoes processing through data pipelines. These pipelines handle data ingestion, cleaning, and structuring tasks, ensuring the data is prepared for subsequent analysis.
3. Embedding model: Following preparation, the data is passed through an embedding model. This model converts textual data into numerical representations called vectors that can be understood by AI models. Widely used models include those developed by OpenAI, Google, and Cohere.
4. Vector database: The resulting vectors are stored in a vector database, facilitating efficient querying and retrieval. Prominent examples of vector databases include Pinecone, Weaviate, and PGvector.
5. APIs and plugins: APIs and plugins such as Serp, Zapier, and Wolfram are instrumental in linking various architecture components and offering additional functionalities. They enable tasks such as accessing additional data or seamlessly performing specific actions.
6. Orchestration layer: The orchestrating layer plays a pivotal role in handling the workflow. ZBrain is an example of this layer, simplifying prompt chaining, handling interactions with external APIs by identifying when API calls are necessary, retrieving contextual data from vector databases, and maintaining memory across multiple LLM calls. Ultimately, this layer crafts a prompt or series of prompts for submission to a language model for processing. Its function is to orchestrate the movement of data and tasks, ensuring smooth coordination across all components of the architecture.
7. Query execution: The data retrieval and generation process commences when the user submits a query to the due diligence app. This query can encompass various aspects relevant to the target company, from financial well-being to legal compliance and operational risks.
8. LLM processing: Upon receiving the query, the app forwards it to the orchestration layer. This layer then retrieves pertinent data from the vector database and LLM cache and directs it to the suitable LLM for processing. The selection of the LLM hinges on the query’s characteristics and requirements.
9. Output: The LLM produces an output based on the query and the data it receives. This output can manifest in diverse forms, including factual information summaries, identification of potential risks, or even drafting reports.
10. Asset management app: The validated output is then presented to the user through the asset management app. This core application is the central hub where all data, analysis, and insights converge. It presents the findings in a user-friendly format, making it easy for decision-makers to understand.
11. Feedback loop: User feedback on the LLM’s output is another vital aspect of this architecture. This feedback loop is instrumental in progressively refining the accuracy and relevance of the LLM’s output over time.
12. Agent: AI agents are crucial in tackling complex problems, engaging with the external environment, and enhancing learning through post-deployment experiences. They achieve this by utilizing advanced reasoning and planning, strategic tool utilization, and leveraging memory, recursion, and self-reflection.
13. LLM cache: Tools such as Redis, SQLite, or GPTCache store frequently accessed information, thereby accelerating the AI system’s response time.
14. Logging/LLMOps: Throughout this process, LLM operations (LLMOps) tools like Weights & Biases, MLflow, Helicone, and Prompt Layer assist in logging actions and monitoring performance. This ensures that LLMs operate at their best and continually enhance through feedback loops.
15 . Validation: A validation layer is integrated to verify the accuracy and reliability of the LLM’s output. Tools such as Guardrails, Rebuff, Guidance, and LMQL ensure that the information provided meets the required standards.
16 . LLM APIs and hosting: LLM APIs and hosting platforms are crucial in executing asset management tasks and hosting the application. Developers have various options, including LLM APIs provided by OpenAI and Anthropic or open-source models. Similarly, they can opt for hosting platforms offered by cloud providers like AWS, GCP, Azure, and Coreweave or prefer opinionated clouds like Databricks, Mosaic, and Anyscale. The selection of LLM APIs and cloud hosting platforms depends on the needs and preferences of the project.
This structured flow offers a comprehensive overview of how AI enhances asset management. It harnesses diverse data sources and technological tools to produce accurate and actionable insights. By automating tasks, AI boosts efficiency and enables a thorough analysis of assets, ultimately streamlining the asset management process.
Optimize Your Operations With AI Agents
Optimize your workflows with ZBrain AI agents that automate tasks and empower smarter, data-driven decisions.
Generative AI use cases in asset management
Generative AI has found a variety of applications across different industries, and asset management is no exception. In asset management, generative AI techniques can be used to enhance decision-making processes, optimize portfolio management, predict market trends, and automate various tasks. Here are some generative AI use cases for asset management:
Portfolio optimization and generation
Generative AI holds significant potential in both the optimization and generation of investment portfolios, taking into account a multitude of factors, including risk tolerance, investment objectives, and ever-evolving market conditions. In contrast to traditional portfolio optimization techniques that primarily rely on historical data and underlying assumptions, generative AI stands out by offering a dynamic approach to crafting investment portfolios.
By leveraging generative AI, asset managers gain the ability to simulate a wide spectrum of potential market scenarios, encompassing various states of uncertainty and adapting to the dynamic nature of financial markets. This versatility enables the generation of portfolios that are finely attuned to prevailing market conditions, ultimately enhancing their performance and resilience.
Generative AI’s capacity to analyze and adapt to real-time data provides a distinct advantage in portfolio management. It offers asset managers the flexibility to consider a broader range of variables and make data-driven decisions that align portfolios with contemporary market dynamics. As a result, portfolios crafted with the assistance of generative AI not only respond to evolving conditions but also exhibit a greater propensity to excel in complex and uncertain environments.
Predictive analytics
Predictive analytics plays a crucial role in asset management, and generative AI empowers asset managers in this domain by enhancing foresight and personalization. Traditional investor communication often needs more depth and personalization than today’s investors demand. Generative AI addresses this challenge by utilizing predictive capabilities to analyze an investor’s portfolio, deciphering its performance and risk factors. It then crafts compelling, personalized narratives outlining past performance and providing tailored insights into the portfolio’s trajectory. This approach fosters stronger client relationships and builds confidence in asset management services.
Furthermore, generative AI’s predictive analytics capabilities extend to delivering unique market insights. In today’s rapidly evolving financial landscape, asset managers must stay ahead of market developments, and generative AI helps by offering customized, real-time insights on market dynamics. It can monitor and identify market disruptors as they unfold, assess the implications of shock events, and provide a granular understanding of the forces influencing asset values. This real-time, customized analysis allows asset managers to make proactive, well-informed decisions, adjusting their strategies in response to market disruptors and capitalizing on emerging drivers. By harnessing generative AI’s predictive analytics, asset managers navigate the dynamic financial landscape with enhanced confidence and precision.
Scenario analysis
Among the myriad applications of generative AI in asset management, one stands out for its sheer importance—Scenario Analysis. In an era defined by market volatility, economic uncertainties, and unforeseen events, the ability to gauge how investment portfolios would fare under diverse, often extreme circumstances is paramount. Scenario analysis is the safeguard against the unknown, offering asset managers a crucial edge. It allows them to meticulously simulate a multitude of potential market scenarios, spanning from economic downturns to global crises and beyond. Unlike traditional methods, generative AI excels in creating these comprehensive simulations, providing asset managers with a deep understanding of their portfolios’ resilience.
The significance of scenario analysis lies in its ability to uncover hidden risks and its capacity to inform proactive decision-making. By identifying vulnerabilities and potential losses, asset managers can swiftly adjust their strategies and portfolio compositions, bolstering their resilience in the face of unpredictability. In this rapidly evolving financial landscape, generative AI-driven scenario analysis emerges as a distinctive and indispensable tool, ensuring that asset management remains adaptive, resilient, and future-proof.
Asset allocation optimization
Generative AI plays a crucial role in optimizing asset allocation strategies by continuously analyzing real-time market data and making dynamic adjustments to portfolio allocations. This dynamic approach allows asset managers to seize investment opportunities as they arise and reduce exposure to potential risks swiftly. The ultimate goal is to maximize portfolio returns while effectively managing risk, ensuring that the investment portfolio remains aligned with the ever-changing financial landscape. Generative AI enables asset managers to enhance their decision-making by relying on data-driven insights, ultimately aiming to deliver improved investment results for their clients.
Economic forecasting
Generative AI contributes significantly to economic forecasting by harnessing historical economic data and external variables to develop advanced predictive models. These models, driven by data analysis and pattern recognition, enable the anticipation of forthcoming economic trends with potential implications for investment choices. By assessing relationships among external factors, generative AI forecasts metrics such as GDP growth, inflation rates, and exchange rates. These forecasts, invaluable to asset managers, guide strategic investment decisions. For instance, predictions of high inflation might prompt adjustments to portfolios, including assets historically resilient to inflation. Moreover, economic forecasts enhance risk management by aiding preparations for potential economic downturns or shocks. In essence, generative AI empowers asset managers with data-driven foresight, facilitating informed investment strategies aligned with evolving economic conditions.
Customized investment strategies
Generative AI empowers asset managers to craft personalized investment strategies for high-net-worth clients, ushering in a new era of client-centric wealth management. This process begins with comprehensive client profiling, gathering specific details about financial objectives, risk tolerance, and ethical preferences. Leveraging the analytical prowess of generative AI, these profiles are then matched with a diverse array of financial data, including historical market trends and real-time conditions. Using algorithmic precision, the AI formulates tailored investment strategies, aligning with each client’s unique goals while factoring in their ethical criteria, if applicable. Crucially, these strategies remain adaptable, continuously adjusting to evolving market dynamics and changes in the client’s financial circumstances or values. The outcome is a deeply personalized, ethically aligned approach to asset management that not only optimizes financial success but also nurtures enduring client relationships grounded in trust and satisfaction.
Fraud detection
Fraud detection, particularly through anomaly detection, leverages generative models to identify unusual patterns or behaviors within financial transactions, significantly enhancing the ability to detect potential fraudulent activities. By employing advanced algorithms, these generative models learn the normal patterns of legitimate transactions and can effectively flag deviations from the established norms. This capability proves crucial in identifying irregularities, such as unusual spending patterns, unrecognized account access, or anomalous transaction frequencies. The use of generative AI in fraud detection not only provides a proactive approach to identifying potential threats but also allows for continuous learning and adaptation to emerging fraudulent tactics. This use case plays a vital role in bolstering the security of financial systems and mitigating risks associated with fraudulent activities.
Operational efficiency
Operational efficiency in asset management is significantly enhanced through the application of generative AI, specifically in process automation. By leveraging generative models, routine tasks, data analysis, and reporting processes can be automated, leading to substantial improvements in overall operational efficiency. These AI systems streamline repetitive tasks, allowing for quicker and more accurate data processing, reducing manual labor, and minimizing the risk of errors. The automation of routine operations not only saves time and resources but also enables asset management firms to allocate human resources more strategically, focusing on higher-value tasks such as strategic planning, decision-making, and client engagement. The use of generative AI in operational processes marks a transformative shift toward more streamlined and agile asset management operations.
Volatility modeling
Volatility modeling is a crucial application of AI in asset management, where generative models play a pivotal role in modeling and predicting market volatility. By leveraging advanced algorithms, these models analyze historical data and real-time market conditions to provide insights into potential fluctuations in asset prices. This capability enables more accurate risk assessment, empowering asset managers to make informed decisions regarding portfolio management and allocation. Additionally, the predictions derived from volatility modeling contribute to the development of effective hedging strategies, allowing firms to manage and mitigate potential risks associated with market volatility proactively. This use case not only enhances risk management practices but also equips asset managers with valuable tools to navigate dynamic market conditions and optimize investment strategies.
Client communication
The integration of generative AI, specifically Natural Language Generation (NLG), introduces a transformative approach to report generation and client interactions within asset management. By employing advanced algorithms, generative models can automatically process complex financial data and translate it into coherent, human-readable narratives. This automation not only streamlines the report generation process but also ensures consistency and precision in financial communications. Through NLG, asset managers can produce customized reports, summaries, and client communications tailored to individual investor needs swiftly and efficiently. This not only enhances transparency in conveying investment strategies and performance but also fosters more effective and personalized communication, ultimately strengthening the relationship between asset managers and investors. The use of generative AI in client communication marks a significant advancement in providing clear and insightful information to clients, contributing to improved satisfaction and trust.
Performance monitoring
One key benefit of generative AI in asset management is the ability to continuously monitor the performance of portfolios, individual assets. By analyzing historical market data, portfolio compositions, it identifies patterns and trends affecting asset performance. It can detect correlations between specific market events and asset performance, enabling asset managers to adjust strategies accordingly. GenAI’s predictive capabilities allow proactive decision-making, helping asset managers mitigate losses and maximize returns by identifying potential downturns or underperforming assets ahead of time.
Market research and analysis
Generative AI helps conduct comprehensive market research by analyzing vast financial data, market news, and analyst reports. It identifies emerging trends, assesses market sentiment, and predicts movements with greater accuracy. Asset managers can leverage these insights to identify investment opportunities, optimize portfolio allocations, and manage risks associated with market volatility. Moreover, generative AI provides deeper insights into specific sectors or industries, aiding asset managers in making more informed investment decisions aligned with client objectives.
ESG monitoring
Asset managers increasingly integrate Environmental, Social, and Governance (ESG) factors into investment decisions. Generative AI analyzes unstructured ESG data from various sources, evaluating implications for investments. It identifies companies with strong ESG practices, assesses associated risks and opportunities, and ensures alignment with sustainability goals and regulatory requirements. This capability allows asset managers to construct portfolios that reflect clients’ ESG preferences and values while maximizing financial performance.
Automated customer support
Generative AI-powered chatbots and virtual assistants enhance customer support services in asset management by providing automated assistance. These systems handle inquiries related to account management, investment performance, and fund information, offering quick and accurate responses. Leveraging natural language processing and machine learning, they understand investor queries and provide personalized recommendations. This improves customer satisfaction, frees human resources for complex tasks, and strengthens client relationships, enhancing overall service quality.
Risk management
Generative AI plays a critical role in risk management within asset management firms. It analyzes historical market data, financial statements to identify potential risks such as market volatility, credit defaults, and liquidity issues. By simulating various scenarios and stress-testing portfolios, it helps assess the impact of risks on investment performance. Asset managers use these insights to develop strategies that mitigate risks, ensuring portfolio stability and resilience even in uncertain market conditions.
Decision support
Generative AI provides decision support for asset managers by analyzing complex data and generating actionable insights. It recommends investment strategies, asset allocations, and risk mitigation measures based on historical performance, market trends, and client preferences. Additionally, it optimizes portfolio construction, considering factors like diversification and risk-adjusted returns. By augmenting human decision-making with advanced analytics, generative AI helps asset managers make informed, strategic investment decisions that align with client objectives and drive long-term value.
Knowledge management
Effective knowledge sharing across organizations has long been a challenge for asset managers, hindering the utilization of collective wisdom and insights. For decades, organizations have grappled with siloed structures, inefficient document management, and barriers to seamless collaboration. However, the emergence of GenAI promises to change this landscape by transforming knowledge retrieval and synthesis. GenAI addresses the hurdles faced by asset managers by simplifying processes and enhancing user experience. By leveraging natural language processing, it enables intuitive interactions and eliminates the need for manual tagging and uploading of documents. GenAI’s ability to comprehend the intent behind queries allows for more nuanced information retrieval, including documents, images, and insights. Consider a scenario where an asset manager seeks insights into the feasibility of AI capabilities through the lens of cost-effectiveness. With GenAI, they can articulate their query in natural language, such as: “Explore our firm’s analysis on the cost implications of adopting AI capabilities versus developing them in-house.” This level of specificity was previously challenging to achieve. However, with GenAI this is possible, showcasing its transformative impact on knowledge management. By leveraging GenAI, organizations diminish reliance on individual expertise, mitigating key person risk while fostering a culture of collective intelligence. Furthermore, improved access to crucial documents and policies streamlines decision-making processes, significantly reducing the time and resources needed for recreating past analyses.
Generative AI use cases for asset management can lead to more data-driven, adaptable, and efficient investment strategies. However, it’s important to note that while these techniques offer significant potential, they also require careful validation, continuous monitoring, and human expertise to ensure that the generated solutions align with the objectives of asset managers and their clients.
Optimize Your Operations With AI Agents
Optimize your workflows with ZBrain AI agents that automate tasks and empower smarter, data-driven decisions.
How does LeewayHertz’s generative AI platform optimize asset management workflows?
LeewayHertz’s generative AI platform, ZBrain, plays a transformative role in enhancing asset management processes, providing a systematic approach that empowers institutions to achieve their financial objectives efficiently. As a comprehensive, enterprise-ready platform, ZBrain empowers businesses to design and implement applications tailored to their specific operational requirements. The platform uses clients’ data, whether in the form of text, images, or documents, to train advanced LLMs like GPT-4, Vicuna, Llama 2, or GPT-NeoX for developing contextually aware applications capable of performing diverse tasks.
Businesses grapple with challenges in asset management, ranging from ensuring data accuracy to handling the overwhelming volume and speed of financial market data, integrating diverse data sources, addressing legacy system constraints, and navigating complex regulatory compliance issues. ZBrain effectively addresses these challenges through its distinctive feature called “Flow,” which provides an intuitive interface that allows users to create intricate business logic for their apps without the need for coding. Flow’s easy-to-use drag-and-drop interface enables the seamless integration of large language models, prompt templates, and other genAI models into your app’s logic for its easy conceptualization, creation, or modification.
To comprehensively understand how ZBrain Flow works, explore this resource that outlines a range of industry-specific Flow processes. This compilation highlights ZBrain’s adaptability and resilience, showcasing how the platform effectively meets the diverse needs of various industries, ensuring enterprises stay ahead in today’s rapidly evolving business landscape.
Leveraging ZBrain’s LLM-based applications, asset management processes experience a transformative enhancement as intricate data is converted into actionable insights. This results in efficient data analysis, enhanced decision-making, real-time market trend identification, and optimized asset allocation within the asset management domain. ZBrain’s comprehensive solutions effectively address critical challenges, bringing efficiency and strategic value to asset management practices. Delve into the subsequent section to understand how ZBrain elevates asset management within the finance and banking sectors.
AI-driven asset management in finance and banking
With ZBrain’s automated asset management process, financial organizations can remarkably reduce the time and effort required for managing assets. It initiates a robust data-gathering process, including financial statements, customer portfolios, regulatory reports, and market data, ensuring a solid foundation for the knowledge base. Through meticulous collection and preprocessing, ZBrain conducts automated exploratory data analysis involving data structuring, pattern identification, and insight extraction. In the next phase, advanced embedding techniques convert textual data into numerical representations, streamlining analysis for efficient information retrieval and serving as the cornerstone for precise insights, ultimately enhancing decision-making.
Upon receiving a user’s asset management request, ZBrain integrates data into the chosen Large Language Model (LLM) for a thorough risk assessment. Utilizing advanced analytics and pattern recognition, ZBrain optimizes asset allocation and investment strategies for maximizing returns while managing risk. LLM generates a detailed report, providing insights into asset performance and regulatory compliance. Post LLM’s report generation, ZBrain employs parsing to enhance report quality and extract actionable insights, ensuring data-driven, user-friendly reports precisely adhere to the desired layout and guidelines. This meticulous approach ensures a thorough analysis, empowering businesses with actionable insights for informed decision-making.
ZBrain applications empower financial institutions in asset management, streamlining decision-making processes and markedly reducing the time and effort traditionally needed for manual assessments. This results in more effective asset management procedures, decreased risk exposure, optimized asset distribution, personalized strategies, and elevated decision-making capabilities, all while upholding strict data privacy standards. Examine this comprehensive flow to grasp how ZBrain transforms financial asset management, providing a precise and streamlined approach to data analysis, investment strategy formulation, and performance evaluation, thereby redefining the asset management process for enhanced efficiency and strategic value.
LeewayHertz’s AI development services for asset management
At LeewayHertz, we design customized AI solutions that address the unique demands of asset management firms. We offer strategic AI/ML consulting to help asset managers harness AI for improved decision-making, enhanced client engagement, and optimized investment strategies.
Our proficiency in developing Proof of Concepts (PoCs) and Minimum Viable Products (MVPs) allows firms to evaluate the real-world impact of AI tools, ensuring solutions are both effective and tailored to the asset management sector’s specific needs.
Our expertise in generative AI transforms routine tasks like report generation and data management, automating these processes to free up asset managers for more strategic roles.
By fine-tuning large language models to the specific terminology and intricacies of financial assets and client interactions, LeewayHertz enhances the accuracy and relevance of AI-driven communications and analyses.
Additionally, we ensure these AI systems integrate seamlessly with existing technological infrastructures, enhancing operational efficiency and decision-making in asset management firms.
Our AI solutions development expertise
AI solutions development for asset management typically involves creating systems that enhance decision-making, automate routine tasks, and personalize client services. These solutions integrate key components such as data aggregation technologies, which compile and analyze financial information from diverse sources. This comprehensive data foundation supports predictive analytics capabilities, allowing for the forecasting of market trends that inform strategic decisions. Additionally, machine learning algorithms are employed to tailor investment strategies to individual client profiles, ensuring that each client’s unique financial goals and risk tolerance are considered. These solutions often cover areas like portfolio management, risk assessment, regulatory compliance, and client relationship management.
Overall, AI solutions in asset management aim to optimize financial outcomes, improve efficiency, and elevate the client experience.
AI agent/copilot development for asset management
LeewayHertz builds custom AI agents and copilots that enhance various asset management operations, enabling companies to save time and resources while facilitating faster decision-making. Here is how they help:
Portfolio management and optimization:
- Performing in-depth financial data analysis and generating comprehensive investment reports to support portfolio construction and management.
- Identifying potential investment opportunities based on predefined criteria or rules and aligning those opportunities with client goals and risk profiles.
- Analyzing market trends by processing historical and real-time data and predicting future market movements to guide portfolio adjustments.
Client engagement:
- Analyzing client data and past interactions to provide personalized advice and recommendations.
- Automating routine communication tasks like appointment reminders and portfolio updates.
- Offering 24/7 virtual assistance to answer client queries and provide basic information.
Compliance and risk monitoring:
- Automating regulatory document analysis, ensuring firms stay compliant with ever-evolving regulations.
- Monitoring portfolios for compliance with predefined rules and policies.
- Automating documentation and reporting processes.
- Flagging any potential compliance violations or discrepancies.
Process automation:
- Automating repetitive tasks such as data entry and report generation.
- Automating data validation and verification tasks.
- Streamlining client onboarding and KYC processes.
Financial planning:
- Gathering and analyzing data from diverse sources, providing asset managers with a holistic view of their clients’ financial situations.
- Customizing financial plans based on individual client goals, risk tolerance, and financial situations, ensuring personalized and relevant advice.
- Providing asset managers with real-time insights into market conditions and asset performance, supporting timely and informed decision-making.
Asset allocation and rebalancing:
- Recommending asset allocation strategies based on predefined models or rules.
- Identifying imbalances in portfolios and suggesting rebalancing actions within defined thresholds.
Fraud detection:
- Monitoring financial transactions for predefined patterns or rules associated with potential fraud.
- Flagging suspicious activities based on predefined criteria or models.
Marketing and content generation:
- Generating personalized marketing communications or financial education materials based on templates or structured data inputs.
- Assisting with content creation for websites, social media, and marketing campaigns within defined parameters.
Customer segmentation and targeting:
- Analyzing client data to segment customers based on predefined criteria (e.g., demographics, risk profiles, investment preferences).
- Identifying potential cross-selling or upselling opportunities based on customer segments.
AI agents/copilots do not just increase the efficiency of operational processes but also significantly enhance the quality of customer service and strategic decision-making. By integrating these advanced AI solutions into their existing infrastructure, asset management firms can achieve a significant competitive advantage, navigating the complex financial landscape with innovative, efficient, and reliable AI-driven tools and strategies.
What are the challenges faced in the asset management workflow, and how does AI address those challenges?
Asset managers face several challenges throughout the asset management workflow, and AI can address many of these issues by providing efficient solutions. Here are some common challenges and how AI can help:
- Data overload and complexity:
- Challenge: Managing large volumes of data related to asset details, performance, and maintenance can be overwhelming and prone to errors.
- Solution: AI enables automated data processing and analysis, handling complex datasets efficiently. Machine learning algorithms can uncover patterns, anomalies, and insights from the data, reducing the burden on asset managers.
- Risk assessment and compliance:
- Challenge: Assessing and managing risks associated with assets while ensuring compliance with regulations is a complex task.
- Solution: AI-powered risk assessment models can analyze historical data, prevailing market trends, and regulatory changes to provide more accurate risk assessments. Natural Language Processing (NLP) can help in monitoring and staying updated on compliance requirements.
- Predictive maintenance:
- Challenge: Traditional maintenance practices may lead to unnecessary downtime or reactive maintenance, impacting asset performance.
- Solution: AI-driven predictive maintenance uses machine learning algorithms to analyze historical performance data, predicting when an asset is likely to fail. This helps in scheduling proactive maintenance, reducing downtime, and extending asset lifespan.
- Optimization of asset portfolios:
- Challenge: Balancing and optimizing asset portfolios to align with organizational goals can be challenging without real-time insights.
- Solution: AI analytics can optimize asset portfolios by assessing various factors such as risk, return, and strategic importance. Machine learning algorithms can continuously analyze data to recommend adjustments for optimal portfolio performance.
- Budgeting and planning:
- Challenge: Developing accurate budgets and plans for asset acquisition, maintenance, and disposal can be complex without a clear understanding of future asset performance.
- Solution: AI-based forecasting models can predict future asset-related costs based on historical data and performance trends. This helps in developing more accurate budgets and long-term plans.
- Disposal and replacement strategies:
- Challenge: Deciding when to dispose of or replace assets can be subjective and may lead to suboptimal decisions.
- Solution: AI algorithms can analyze factors such as asset age, performance, and market trends to recommend optimal disposal or replacement strategies. This ensures that decisions are data-driven and aligned with organizational goals.
- Reporting and analytics:
- Challenge: Generating meaningful reports and extracting valuable insights from vast datasets can be time-consuming and challenging.
- Solution: AI analytics tools can automate the generation of reports and provide real-time insights. Natural Language Processing can assist in extracting valuable information from unstructured data, making reporting more efficient and informative.
- Continuous improvement:
- Challenge: Implementing effective continuous improvement processes requires ongoing analysis and adaptation.
- Solution: AI enables continuous learning and adaptation by analyzing new data and adjusting models accordingly. This ensures that asset management strategies are continuously refined based on changing conditions and evolving business needs.
By leveraging AI technologies, asset managers can overcome these challenges, streamline their workflows, and make more informed decisions, ultimately improving the efficiency and effectiveness of their asset management processes.
Enterprise requirements for integrating generative AI in asset management
To successfully integrate generative AI into asset management, organizations must carefully consider various aspects of their operations. From aligning AI strategies to enhancing data governance, each step plays a crucial role in maximizing the benefits of this advanced technology.
Business enablement and Ops strategy
- Align the enterprise’s existing AI strategy to include GenAI.
- Collaborate across business units to define and prioritize use cases, establish KPIs, and quantify ROI.
- Develop an operating model, frameworks, and playbooks to manage use case progression from ideation to enterprise deployments.
Data
- Redefine current data lake architectures to enhance data risk controls (copyright, accuracy, privacy).
- Scale design for processed data (e.g., tokens, vectors) and metadata required for GenAI models.
- Dedicate time for data cleansing and governance, aligning on the right definitions of key data features.
- Establish data standards for protected PII use and new IP data creation while adhering to existing AI data governance requirements.
Technology
- Invest in infrastructure and technology patterns to scale capabilities, from development to production, with connectivity to the enterprise data lake for accessing structured/unstructured data.
- Establish an adaptable architecture to accommodate fit-for-purpose LLM models.
- Enhance the AI ModelOps process and frameworks for deploying/monitoring LLMs for the enterprise.
Center of Excellence (COE)/AI lab
- Expand the scope of COEs to include research for GenAI, conducting experiments on closed/open source LLM models for performance and field of use.
- Experiment with prompt engineering, fine-tuning techniques, and orchestration frameworks.
- Evaluate existing vendor portfolios for LLM solution offerings and their impact on current and future deployments.
Risk and governance
- Establish and update firmwide AI-focused policies, disclosures, and risk governance frameworks to address heightened risks posed by GenAI.
- Reevaluate contracts, legal and compliance policies for protected IP usage, copyright infringements, and model bias/discrimination amplification (AI ethics).
- Develop robust testing and monitoring frameworks to measure model risks and performance.
People and training
- Train employees on leading practices and business and security risks associated with LLM usage.
- Upskill business users with focused training on using generative AI enterprise applications.
- Develop talent with technical experience (e.g., fine-tuning, chunking, prompt chaining/classification) to develop enterprise GenAI applications.
By addressing these enterprise requirements, organizations can unlock the full potential of generative AI in asset management, driving innovation, efficiency, and competitive advantage in an increasingly data-driven industry landscape.
How to implement generative AI in asset management?
Implementing generative AI in asset management involves utilizing algorithms and models that can generate novel data points or simulate scenarios to improve decision-making processes. Here’s a general guide on how you might approach this:
- Define objectives and use cases: Identify specific tasks or challenges within asset management where generative AI can add value. This could include generating synthetic data for training models, simulating market conditions, or optimizing portfolio allocations.
- Data collection and preprocessing: Gather relevant historical data for training your generative AI model. This could include financial market data, and other relevant information. Preprocess the data to ensure it is in a suitable and proper format for training and analysis. Clean and normalize the data to remove noise and inconsistencies.
- Choose a generative model: Select a generative AI model that fits your specific use case. Popular choices include Variational Autoencoders (VAEs), Generative Adversarial Networks (GANs), and autoregressive models like LSTMs or transformers.
- Train the generative model: Train the selected model using your historical data. This involves feeding the model with the data and fine-tuning its parameters to minimize the difference between the generated output and the actual data. Validate the model using a separate dataset to ensure it generalizes well.
- Synthetic data generation: Use the trained generative model to generate synthetic data. This can be beneficial for augmenting your dataset, especially in scenarios where obtaining a large amount of real-world data is challenging.
- Scenario simulation: Implement the generative model to simulate different market scenarios. This allows you to analyze how your assets might perform under various conditions, helping with risk management and decision-making.
- Portfolio optimization: Apply generative AI to optimize portfolio allocations by generating and evaluating different portfolio compositions. This can help in creating more robust and adaptive investment strategies.
- Risk management: Use generative models to simulate potential risks and market fluctuations. This can assist in stress-testing portfolios and identifying vulnerabilities.
- Continuous monitoring and iteration: Regularly monitor the performance of your generative AI model and update it as needed. Financial markets are dynamic, and models may need to be retrained to adapt to changing conditions.
- Compliance and ethical considerations: Ensure that your generative AI implementation complies with relevant regulations and ethical guidelines in the asset management industry.
- Collaboration with domain experts: Work closely with domain experts in finance and asset management to validate the outputs of your generative model and incorporate their insights into the decision-making process.
Remember that the successful implementation of generative AI in asset management requires a combination of technical expertise, domain knowledge, and ongoing refinement of models based on real-world feedback and performance monitoring.
If you are in search of a tech partner, LeewayHertz is your trusted ally, offering generative AI consulting and development services tailored to elevate your asset management strategies. With a proven track record in deploying diverse advanced generative AI models and solutions, LeewayHertz helps you kickstart or further your AI journey in asset management.
Optimize Your Operations With AI Agents
Optimize your workflows with ZBrain AI agents that automate tasks and empower smarter, data-driven decisions.
Benefits of generative AI in asset management
GenAI has emerged as a game-changing technology with the potential to redefine various industries, including asset management. By harnessing the power of generative AI, asset management firms can unlock several significant benefits that can enhance decision-making processes, optimize portfolio management strategies, and offer a competitive edge in a rapidly evolving financial landscape.
- Enhanced decision-making: Generative AI brings data-driven insights to the forefront of decision-making. It generates alternative scenarios, allowing asset managers to explore a range of possibilities and outcomes. By simulating various market conditions and risk scenarios, asset managers gain a clearer understanding of potential outcomes, aiding in more informed investment choices. These insights can reduce reliance on gut feelings and emotions, leading to more rational and well-founded decisions.
- Improved risk management: Risk assessment is a critical component of asset management. Generative AI assists in this area by creating diverse and realistic market scenarios. This capability enables asset managers to assess portfolio vulnerabilities under different economic and geopolitical circumstances. By identifying potential risks and vulnerabilities, asset managers can develop more robust risk mitigation strategies, enhancing the resilience of portfolios.
- Personalized client solutions: Clients’ financial goals and risk tolerances vary widely. Generative AI enables asset managers to customize investment strategies based on individual preferences and objectives. By analyzing client data and generating tailored portfolio recommendations, asset managers can offer more personalized solutions that align with client’s specific needs, ultimately building stronger client relationships.A recent study reveals that the use of generative AI in client service has reduced AUM attrition by 20% through proactive engagements.
- Increased efficiency: The integration of generative AI streamlines operational processes and increases efficiency. Automating data processing, analysis, and reporting allows asset managers to concentrate on higher-level tasks, such as strategic decision-making and client interaction. This efficiency boost reduces human errors and saves a substantial amount of time, approximately 50-80%, enabling asset managers to focus on value-added activities.
- Enhanced portfolio optimization: Generative AI can contribute to portfolio optimization by analyzing vast datasets and identifying optimal asset allocations. By considering historical performance, market trends, and risk factors, asset managers can fine-tune portfolios for maximum risk-adjusted returns. This optimization process can be carried out more comprehensively and accurately through AI-powered algorithms.
- Addressing cognitive biases: Cognitive biases, often unconscious, can impact investment decisions. Generative AI offers an objective analysis of data, serving as a counterbalance to emotional biases. By relying on data-driven insights, asset managers can mitigate the effects of biases like fear, greed, and overconfidence, leading to more objective and rational investment choices.
- Innovative investment strategies: Generative AI opens the door to innovative investment strategies that leverage sophisticated data analysis. Asset managers can uncover hidden patterns and correlations that traditional methods might miss by creating synthetic data or simulating scenarios. These insights can lead to the development of unique strategies that offer a competitive advantage.
- Adaptation to market dynamics: Financial markets are dynamic and subject to rapid changes. Generative AI equips asset managers with the ability to adapt quickly to shifting market conditions. By generating real-time insights and adapting strategies accordingly, asset managers can make timely adjustments that align with evolving market trends.
- Strengthened compliance and reporting: Generative AI aids in compliance by automating data validation and ensuring adherence to regulations. AI-driven reporting processes can provide accurate and timely insights for regulatory reporting requirements. This reduces the risk of non-compliance and enhances transparency in client reporting.
Generative AI has the potential to reshape asset management by providing data-driven insights, optimizing portfolio strategies, and addressing inherent challenges. The benefits outlined above underscore the transformative impact of generative AI on asset management practices, positioning firms to thrive in a data-driven and dynamic financial environment.
Generative AI models used in asset management
AI models and techniques have found applications in various fields, including asset management. These models can help generate valuable insights, forecasts, and decision-support tools for asset managers. Here are some generative AI models commonly used in asset management:
Variational Autoencoders (VAEs)
Variational Autoencoders (VAEs) play a vital role in asset management by enabling feature extraction, risk assessment, portfolio optimization, and market sentiment analysis. VAEs, often combined with algorithms like Reinforcement Learning, assist in constructing optimized portfolios, simulating market scenarios, and detecting anomalies in asset behavior. They enhance risk parity strategies, aid in time series forecasting, and identify different market regimes. However, it’s crucial to remember that VAEs are one tool among many, and their effectiveness depends on specific applications and data quality, often used alongside traditional financial models and domain expertise to make informed investment decisions in dynamic financial markets.
Auto-Regressive models
Auto-Regressive (AR) models are valuable in asset management for time series forecasting. They capture the temporal dependencies in historical asset price data, enabling the prediction of future price movements. AR models, often extended with components like GARCH for volatility modeling, assist in risk assessment and portfolio optimization. By forecasting asset returns and volatility, they help in managing and adjusting portfolios to respond to changing market conditions, ultimately aiding in the development of more informed investment strategies.
Transformer-based models
Transformer-based models, known for their effectiveness in sequence-to-sequence tasks, are valuable in asset management for their ability to capture complex relationships in financial data. They excel in modeling both short and long-term dependencies, making them suitable for predicting asset prices and portfolio optimization. Transformers can process large-scale financial data efficiently, extract meaningful features, and detect patterns, enhancing decision-making. They can also be applied to natural language processing tasks, facilitating sentiment analysis of news and social media data for market sentiment-based strategies. By leveraging these capabilities, Transformer-based models assist in improving asset management strategies through enhanced data-driven insights and decision-making processes.
It’s important to note that while generative AI models can be powerful tools in asset management, they also come with challenges related to data quality, model interpretability, and ethical considerations. Asset managers must carefully evaluate and validate the models they use, considering their industry’s requirements and regulatory constraints. Additionally, these models should be part of a broader decision-making process that combines human expertise with AI-driven insights.
Emerging trends in generative AI for asset management
The future prospects and trends of generative AI in asset management hold immense promise for transforming the industry. As technology continues to evolve, several key developments are anticipated:
Enhanced data generation: Generative AI models will become even more proficient at generating synthetic financial data that closely mimics real-world market conditions. This synthetic data will be invaluable for backtesting strategies, conducting stress tests, and training machine learning models, reducing the reliance on scarce historical data.
Interpretable AI: As the use of AI in asset management grows, there will be a growing emphasis on the interpretability and explainability of AI models. Developments in generative AI will strive to make the decision-making process more transparent, helping asset managers understand why a particular strategy or recommendation was generated.
Advanced risk management: Generative models will play a pivotal role in enhancing risk management practices. They will provide more accurate simulations of market scenarios, enabling asset managers to develop strategies that are resilient in the face of extreme events and black swan occurrences.
Personalized portfolio management: Generative AI will enable asset managers to offer highly personalized investment strategies to clients. These strategies will be tailored to individual risk tolerance, financial goals, and ethical preferences, thanks to the improved predictive capabilities of AI models.
Ethical investing and ESG integration: Generative AI is poised to enhance the seamless integration of Environmental, Social, and Governance (ESG) criteria into the process of making investment decisions. These models can be used to identify ESG-compliant investments and screen out non-compliant ones, aligning portfolios with ethical values.
AI-powered robo-advisors: The adoption of AI-powered robo-advisors will continue to grow. Generative AI will enhance the sophistication of these platforms, providing retail investors with increasingly intelligent and automated investment advice.
Market sentiment analysis: Natural Language Processing (NLP) techniques within generative AI will advance market sentiment analysis. AI models will be better equipped to process vast amounts of textual data from news, social media, and financial reports to gauge market sentiment accurately.
Regulatory compliance: Generative AI will play a pivotal role in ensuring compliance with ever-evolving financial regulations. These models will assist in automating compliance tasks, reducing the risk of human errors and costly regulatory breaches.
Global expansion: The adoption of generative AI in asset management is not limited to a specific region. It is a global trend that will see wider adoption in different financial markets, creating a more level playing field for investors worldwide.
Generative AI is poised to redefine asset management by offering advanced tools for data generation, risk assessment, ethical investing, and personalized portfolio management. The industry’s future will likely see more intelligent, adaptive, and data-driven investment decision-making approaches, ultimately benefiting asset managers and their clients. However, managing the challenges of bias, ethics, and regulatory compliance is essential as AI continues to gain prominence in the field.
Endnote
Generative AI is poised to redefine the landscape of asset management, ushering in an era of data-driven, personalized, and resilient investment strategies. The dynamic and complex nature of financial markets demands innovative approaches, and generative AI emerges as a powerful ally that empowers asset managers. Generative AI’s ability to process vast amounts of structured and unstructured data, simulate market conditions, and anticipate trends in real-time positions it as a game-changer. It not only enhances decision-making but also offers tools for risk management, ethical investing, and tailored portfolio management. It assists in addressing cognitive biases, automates routine tasks, and fosters a partnership between human judgment and AI-driven insights.
However, as generative AI continues to evolve and integrate further into the asset management industry, it’s essential to address challenges related to data quality, model interpretability, and ethical considerations. Asset managers must remain vigilant and maintain a balance between harnessing the power of AI and ensuring transparency, accountability, and adherence to regulatory frameworks.
In this rapidly changing landscape, asset managers who embrace generative AI technology with a strategic, responsible, and ethical approach are likely to gain a competitive edge. While the financial industry evolves, one thing remains certain: Generative AI is reshaping asset management, making it more data-driven, agile, and client-centric than ever before. As this transformative journey continues, the partnership between human expertise and generative AI insights promises to redefine the future of asset management.
Maximize your investment potential by utilizing generative AI in asset management. Reach out to LeewayHertz AI experts and redefine your financial success.
Start a conversation by filling the form
All information will be kept confidential.
FAQs
What is generative AI, and how is it applied in asset management?
Generative AI refers to a subset of artificial intelligence that involves machines creating original content, such as images, text, or data, by learning patterns and generating novel outputs. In asset management, it simulates market conditions, optimizes portfolios, and develops sophisticated trading strategies. Going beyond the analysis of historical data, generative AI creates new insights and predictive models, empowering asset managers to make informed decisions with unprecedented precision.
What challenges do businesses involved in asset management face, and how can generative AI address these challenges?
Businesses in asset management grapple with challenges like data quality, market volatility, evolving regulations, performance consistency, managing investor expectations, and maintaining a delicate balance between customization and scalability. Generative AI offers solutions that enhance decision-making, improve risk management, provide personalized client solutions, increase operational efficiency, optimize portfolios, and enable adaptation to market dynamics.
What are the benefits of incorporating generative AI into asset management practices?
The benefits of generative AI in asset management include enhanced decision-making, improved risk management, personalized client solutions, increased efficiency, enhanced portfolio optimization, addressing cognitive biases, innovative investment strategies, adaptation to market dynamics, strengthened compliance and reporting, and overall reshaping of asset management practices.
What ethical considerations should be considered when leveraging generative AI in asset management?
Ethical considerations in leveraging generative AI in asset management should focus on transparency in decision-making, avoiding bias in data generation, ensuring fair and responsible use of AI-generated insights, and addressing potential societal impacts, emphasizing accountability and adherence to ethical standards in financial decision-making.
What are the best practices for employing generative AI in asset management?
Best practices for employing generative AI in asset management include thorough planning, employee training, a dedicated focus on data privacy, starting with small-scale projects, collaborating with experienced AI professionals, and iterating based on real-world feedback for optimal results.
What factors drive the adoption of generative AI in asset management?
The adoption of generative AI in asset management is driven by factors such as data abundance, the complexity of financial markets, the need for real-time decision-making, alternative data sources, risk management, alpha generation, cost efficiency, personalization, competitive advantage, regulatory compliance, and scalability.
What are the future trends of generative AI in asset management?
Emerging trends in generative AI for asset management include improved data generation, increased emphasis on interpretability, enhanced risk management, personalized portfolio management, seamless ESG integration, advanced robo-advisors, improved market sentiment analysis, integration into quantitative trading, growing significance in regulatory compliance, and global adoption.
What are some examples of generative AI models commonly used in asset management?
Common generative AI models in asset management include Generative Adversarial Networks (GANs) for synthetic data generation, Variational Autoencoders (VAEs) for feature extraction and risk assessment, Auto-Regressive (AR) models for time series forecasting, and Transformer-based models for capturing complex relationships in financial data.
How do real-world firms utilize generative AI in asset management?
Firms like BlackRock, Deutsche Bank AG, Bridgewater Associates, Vanguard Group, and Goldman Sachs are actively leveraging generative AI for tasks such as extracting insights from text sources, embedding AI into financial services, reshaping investment strategies, automating routine cognitive tasks, and optimizing portfolios.
How can asset managers balance the benefits of generative AI with challenges related to data quality, model interpretability, and ethical considerations?
Asset managers must carefully evaluate and validate the generative AI models they use, considering industry requirements and regulatory constraints. These models should be integrated into a broader decision-making process that combines human expertise with AI-driven insights. Transparency, accountability, and adherence to ethical standards are crucial aspects of responsibly integrating generative AI.
How is generative AI reshaping the future of asset management, and what is the outlook for the industry?
Generative AI is reshaping the future of asset management by enhancing data analysis, improving risk management, enabling personalized strategies, and contributing to ethical investing. The outlook for the industry is optimistic, with generative AI expected to drive innovation, improve decision-making processes, and foster a more dynamic and responsive landscape for asset managers.
In what ways does LeewayHertz assist businesses aiming to leverage generative AI for asset management?
LeewayHertz is a leading AI-focused software development company specializing in creating custom generative AI models and solutions for diverse use cases. When businesses engage LeewayHertz for generative AI solutions to refine and enhance their asset management processes, the company meticulously follows a rigorous process to guarantee optimal outcomes.
The journey begins with thoroughly assessing the specific problem areas within the client’s asset management processes. LeewayHertz diligently identifies opportunities for integration, seeking out strategic points where generative AI can provide valuable solutions. By understanding the unique challenges each client faces, LeewayHertz ensures that the generative AI solutions built are precisely tailored to address their distinct needs in the realm of asset management.
LeewayHertz goes beyond mere development by offering ongoing support and maintenance services for the generative AI solutions implemented to optimize performance, address any emerging issues, and adapt the generative AI solutions to evolving business requirements. This commitment to post-implementation assistance underscores LeewayHertz’s dedication to ensuring that clients continue to derive maximum benefit from their generative AI applications in asset management.
In summary, Leewayhertz not only excels in the creation of custom generative AI solutions for asset management but also goes the extra mile to integrate, support, and maintain these solutions, fostering a seamless and enduring partnership with businesses seeking to leverage generative AI in their asset management endeavors.