Generative AI for enterprises: Outlook, use cases, benefits, solutions, implementations and future trends
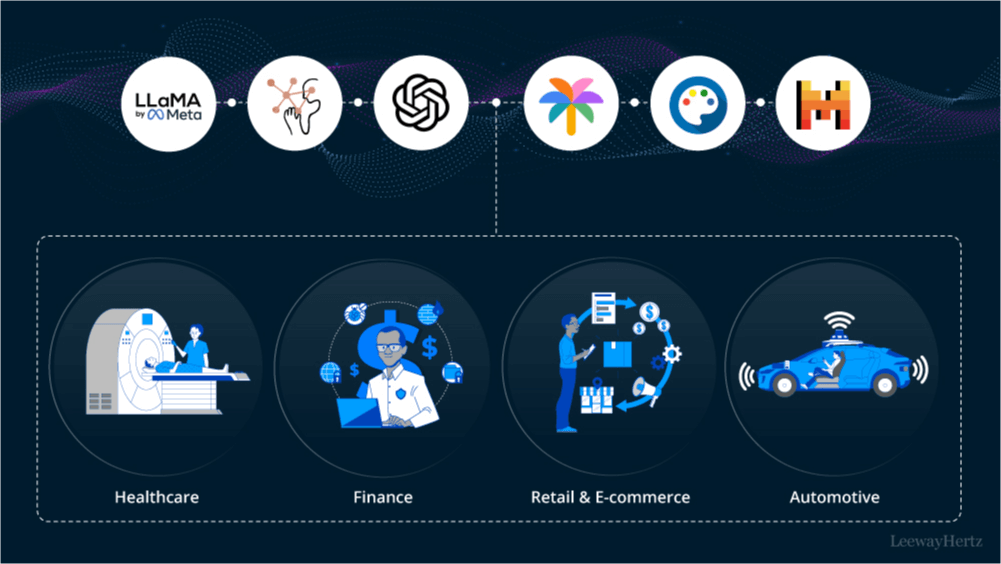
Generative AI (GenAI) is transforming how enterprises operate, innovate, and engage with customers. This technology, which includes sophisticated models capable of producing original content, solving complex problems, and automating tasks, is rapidly becoming a cornerstone of digital transformation strategies. For businesses, the integration of GenAI offers unparalleled opportunities for creativity, efficiency, and personalization. By leveraging models like GPT (Generative Pre-trained Transformer) for text generation or DALL·E for image creation, companies can enhance content production, streamline workflows, and deliver personalized experiences at scale.
The strategic deployment of GenAI within enterprises goes beyond mere automation. It involves the use of AI to generate insights, drive decision-making, and foster innovation across various domains, including marketing, customer service, product development, and beyond. The customization and fine-tuning of these models to suit specific enterprise needs ensures that the generated outputs are highly relevant and valuable. Furthermore, as these AI technologies advance, they become more accessible and integrated into enterprise ecosystems, enabling even small and medium-sized businesses to leverage their capabilities.
However, harnessing the full potential of GenAI requires careful consideration of ethical implications, data privacy, and security. Enterprises must navigate these challenges with robust governance frameworks and ethical AI practices to ensure responsible use. As GenAI continues to evolve, its role in shaping the future of enterprise operations and strategy is unmistakably significant, promising a new era of innovation and competitive advantage.
This article provides an in-depth exploration of generative AI (GenAI) within the enterprise context, starting from its foundational technologies and potential to transform business operations. It outlines the various challenges businesses face today and how GenAI offers innovative solutions, emphasizing the benefits and wide-ranging use cases across different enterprise functions. Additionally, the article offers strategic insights on implementing GenAI, addressing ethical considerations, governance, best practices, and future prospects, guiding enterprises through the journey of adopting and maximizing the value of Gen AI technologies.
- Generative AI for enterprises: Outlook
- The foundation of generative AI in enterprises
- Challenges in enterprises: How generative AI provides solutions
- Generative AI use cases for enterprises
- Benefits of generative AI for enterprises
- Generative AI implementation in enterprises
- How can LeewayHertz help your enterprise implement generative AI?
- Ethical and governance strategies for generative AI deployment in enterprises
- Enterprise deployment of generative AI: A comprehensive strategy
- Future of GenAI in Enterprises
Generative AI for enterprises: Outlook
In the rapidly evolving landscape of modern business, generative artificial intelligence (GenAI) emerges as a transformative force, driving innovation and efficiency across a myriad of industries. At its core, GenAI refers to the subset of AI technologies capable of generating new content, ideas, or data patterns based on the training received from existing datasets. Unlike traditional AI, which focuses on analyzing data and making predictions, GenAI can create, innovate, and ideate, pushing the boundaries of what machines can achieve.
A survey by McKinsey underscores the significance of GenAI in the corporate world, revealing that approximately one-third of companies globally are now leveraging GenAI within at least one business segment. Moreover, 40% of respondents intend to augment their AI investments, motivated by recent technological breakthroughs. These figures highlight the growing recognition of GenAI’s potential to deliver substantial business value.
Generative AI is increasingly prevalent in enterprise scenarios, including content generation, product development, and tackling complex challenges where traditional analytical methods are inadequate. Its capacity to assimilate information from data and spontaneously produce new, unprogrammed outputs positions it as a vital asset for companies aiming to maintain a competitive edge in the digital era. For instance, the same McKinsey survey projects that three-quarters of industry leaders anticipate GenAI to precipitate significant, potentially disruptive changes in market dynamics in the forthcoming years. This landscape signifies a promising yet challenging frontier for businesses. Embracing GenAI not only demands robust computational resources and a sophisticated data infrastructure but also a strategic approach to managing high-quality, diverse training datasets.
Evolution and current state of Gen AI
The journey of GenAI from a theoretical concept to enterprise reality is marked by significant milestones. Initially rooted in academic research and experimental projects, the technology gained momentum with the development of neural networks and deep learning algorithms, which provided the computational power and sophistication required to process and learn from large datasets.
The breakthrough came with the advent of Generative Adversarial Networks (GANs) and other similar technologies, which demonstrated GenAI’s potential to produce high-quality, original content. Today, the state of GenAI is characterized by rapid advancements and increasing accessibility, with tools and platforms making it easier for enterprises to implement and benefit from this technology.
The current state of GenAI showcases its versatility across different domains, such as creating realistic images and videos, generating human-like text, and designing innovative products. These capabilities are not just novelties but have practical applications in marketing, R&D, customer service, and more, signifying GenAI’s transition from experimental to essential in enterprise environments.
Strategic importance in the digital era
The strategic importance of GenAI for enterprises in the digital era cannot be overstated. In a landscape dominated by digital transformation, GenAI provides businesses with a competitive edge through several key advantages:
- Innovation acceleration: GenAI fosters creativity, enabling companies to explore new product designs, content strategies, and business models at an unprecedented pace.
- Efficiency and productivity: By automating creative and analytical processes, GenAI allows teams to focus on strategic tasks, reducing time-to-market and operational costs.
- Personalization at scale: GenAI’s ability to generate customized content and solutions meets the growing demand for personalization, enhancing customer engagement and satisfaction.
- Data-driven decision making: Beyond generating content, GenAI can uncover insights and patterns within large datasets, supporting more informed and strategic business decisions.
- Adaptability and resilience: In an era marked by rapid change, GenAI equips businesses with the tools to adapt quickly, leveraging the technology to respond to new challenges and opportunities as they arise.
As we move forward, the integration of GenAI into enterprise strategies is not just an option but a necessity for those aiming to thrive in the digital era. Its potential to transform operations, drive innovation, and create value makes it a critical component of the digital transformation journey for businesses across the globe. The evolution of GenAI is a testament to the remarkable potential of artificial intelligence to not just augment human capabilities but to pave new paths to the future of business and society.
The foundation of generative AI in enterprises
Core technologies behind generative AI
1. Machine learning and deep learning
Machine Learning (ML) and Deep Learning (DL) serve as the backbone for Generative AI, enabling computers to learn from data, identify patterns, and make decisions with minimal human intervention. Deep Learning, a subset of ML, utilizes neural networks with many layers (hence “deep”) to process data in complex ways. This foundation allows Generative AI to produce new content by learning from vast datasets, whether it’s text, images, or another form of media.
2. Natural language processing and generation
Natural Language Processing (NLP) is a pivotal technology that enables computers to understand, interpret, and generate human language. Within Generative AI, NLP is used to create text-based content that mimics human writing styles, from news articles to poetry. Advanced models like GPT (Generative Pre-trained Transformer) showcase how Generative AI can produce coherent and contextually relevant text on a wide array of topics.
3. Image and video generation technologies
Generative AI also excels in creating visual content, thanks to technologies like Generative Adversarial Networks (GANs). These networks consist of two models: one that generates images and another that evaluates them. Through their interaction, GANs can produce highly realistic images and videos. This capability is transforming fields such as digital art, game development, and virtual reality, enabling the generation of detailed and diverse visual assets at scale.
Generative AI tech stack
The generative AI tech stack consists of three core layers:
1. Application layer: The application layer is the front-facing component of the generative AI tech stack, facilitating collaboration between humans and machines in novel and innovative ways. These powerful applications act as essential workflow tools, making AI models accessible and easy to use for businesses and consumers alike. Whether you’re aiming to enhance your business’s productivity or exploring innovative entertainment avenues, the application layer of the generative AI tech stack is pivotal for unlocking the full potential of this cutting-edge technology.
This layer can be divided into two broad types:
- End-to-end apps using proprietary models: These apps incorporate generative AI models into user-facing products, handling all aspects of the generative AI pipeline, from data collection to model training, inference, and deployment. Developed and owned by companies or organizations, these proprietary generative AI models are not publicly available but are made accessible to customers as part of a software product or service. Examples include OpenAI’s DALL-E and GPT.
- Apps without proprietary models: These apps utilize generative AI models but do not depend on proprietary models. Typically built using open-source generative AI frameworks or libraries like TensorFlow, PyTorch, or Keras, they allow developers to build custom generative AI models for specific use cases. Examples include RunwayML, StyleGAN, and NeuralStyler.
2. Model layer: At the core of the generative AI tech stack lies a trifecta of layers. This unique combination provides maximum flexibility, catering to specific market needs and nuances. The layers of AI engines below serve as the foundation for creating remarkable generative tech outputs.
- General AI models: These models, including GPT-3 for text, DALL-E-2 for images, Whisper for voice, and Stable Diffusion for various applications, form the foundational breakthrough of the generative tech revolution. Unlike narrow AI models designed for specific tasks, general AI models replicate human-like thinking and decision-making processes, handling a wide range of tasks and learning from experience. User-friendly and open-source, these models represent a powerful starting point for advancements in the generative tech stack.
- Specific AI models: Also known as domain-specific models, these are designed to excel in specific tasks such as generating ad copy, tweets, song lyrics, e-commerce photos, or 3D interior design images. Trained on highly specific and relevant data, they outperform general AI models in their domain by leveraging specialized knowledge and data.
- Hyperlocal AI models: With hyperlocal and often proprietary data, these models achieve unparalleled levels of accuracy and specificity in their outputs. From writing scientific articles to creating e-commerce photos aligned with a company’s branding, hyperlocal AI models produce highly customized and accurate outputs tailored to specific needs.
3. Infrastructure layer: The infrastructure layer is a critical component of the generative AI tech stack, comprising hardware and software necessary for creating and training AI models. Hardware components may include specialized processors like GPUs or TPUs, while software components provide developers with the necessary tools to build and train AI models. Additionally, cloud computing services offer organizations instant access to extensive computing resources and storage capacity, reducing the cost and complexity of developing and deploying AI models while enabling scalable AI capabilities.
Evolution of generative AI in the business context
The landscape of Generative AI in business has transitioned significantly, moving from basic automation to undertaking roles that require creativity and deep analytical capabilities. Initially, the focus of AI was to streamline routine tasks, leveraging basic algorithms and computational resources. However, as these technologies advanced in sophistication, their application broadened. Generative AI started to play pivotal roles in creating dynamic marketing content, innovating product designs, and deriving insightful analyses from complex datasets. This progression has unlocked new opportunities for businesses to enhance innovation, operational efficiency, and personalization within their processes. It underscores a trend toward making Generative AI an integral element of corporate strategies, illustrating its growing importance and potential to reshape the future of enterprise operations.
Key factors driving the adoption of generative AI in enterprises
Several factors are propelling the adoption of generative AI in enterprises:
- Data availability and quality: The explosion of data in the digital age provides the raw material for generative AI to learn and create, making AI more powerful and accurate.
- Computational power: Advances in hardware, such as GPUs and cloud computing, have made it feasible to train large, complex AI models, making generative AI more accessible to businesses.
- Business efficiency and innovation: Companies are under constant pressure to innovate and stay ahead of the competition. Generative AI offers a way to enhance creativity, streamline operations, and personalize customer experiences at scale.
- Customer expectations: With the rise of digital services, customers expect highly personalized and engaging experiences. Generative AI enables businesses to meet these expectations by generating tailored content, recommendations, and interactions.
- Cost reduction: Generative AI can automate content creation, data analysis, and other tasks, significantly reducing operational costs and freeing up human resources for more strategic initiatives.
The integration of generative AI into enterprises marks a significant shift towards more intelligent, automated, and personalized business processes. As these technologies continue to evolve, their potential to transform industries and redefine the way we work becomes even more apparent.
Optimize Your Operations With AI Agents
Optimize your workflows with ZBrain AI agents that automate tasks and empower smarter, data-driven decisions.
Challenges in enterprises: How generative AI provides solutions
Enterprises today are facing a multitude of challenges driven by rapidly evolving market demands, technological advancements, and the imperative for sustainable operations. Generative AI emerges as a pivotal technology capable of addressing these challenges by leveraging its unique capabilities to innovate, automate, and analyze at an unprecedented scale.
Addressing market demands for personalization at the scale
Challenge: In the digital age, customers expect products, services, and experiences that cater specifically to their preferences and needs. Meeting these expectations requires businesses to personalize at scale, a daunting task given the vast amount of data and the nuanced understanding needed.
GenAI solution: Generative AI can analyze customer data to identify patterns, preferences, and behaviors, enabling the creation of personalized content, recommendations, and products. By automating the personalization process, enterprises can cater to individual customer needs without compromising on scale or efficiency.
Overcoming resource constraints and enhancing productivity
Challenge: Businesses often face the dilemma of improving productivity and output while operating within limited resource constraints. Traditional methods of scaling up involve significant investments in manpower and capital.
GenAI solution: Generative AI technologies automate complex and time-consuming tasks, from content creation to data analysis, reducing the need for additional human resources and enabling existing teams to focus on higher-value activities. This shift not only enhances productivity but also optimizes resource allocation.
Breaking through creative limitations in product development
Challenge: Innovation in product development is key to staying ahead of the competition but is often hampered by creative limitations and the risk of investing in unproven ideas.
GenAI solution: By leveraging GenAI, enterprises can explore a wider range of creative solutions and prototypes without the traditional constraints. GenAI can generate innovative design options, simulate outcomes, and predict market responses, thereby reducing the risk associated with new product development.
Solving complex problems with enhanced data analysis
Challenge: The increasing complexity of business environments and the sheer volume of data generated make it difficult to derive actionable insights and solve complex problems efficiently.
GenAI Solution: Generative AI excels in sifting through and analyzing vast datasets, identifying trends, and predicting outcomes that might not be apparent to human analysts. This capability enables businesses to address complex challenges with data-driven strategies and solutions.
Automating routine tasks to focus on strategic activities
Challenge: Valuable human resources are often bogged down by routine tasks, limiting their capacity to contribute to strategic initiatives.
GenAI solution: Generative AI can take over repetitive tasks such as data entry, report generation, and even routine customer service inquiries, freeing up human employees to focus on strategic thinking, creativity, and decision-making processes.
Navigating rapid technological changes and staying competitive
Challenge: The fast pace of technological advancement requires businesses to continually adapt and innovate to remain competitive. However, the speed at which new technologies emerge can be overwhelming.
GenAI solution: Generative AI itself is at the forefront of technological innovation, offering businesses tools to quickly generate new ideas, adapt products and services, and stay ahead of trends. By integrating GenAI into their operations, companies can become more agile and responsive to technological shifts.
Implementing sustainable practices through efficient resource use
Challenge: There is increasing pressure on enterprises to adopt sustainable practices, requiring them to find ways to reduce waste and improve efficiency across their operations.
GenAI solution: Generative AI can optimize resource allocation, predict maintenance needs to prevent wastage, and even aid in designing more efficient products and processes. This not only helps businesses reduce their environmental footprint but also leads to significant cost savings.
Innovation pressure
Challenge: The constant need to innovate to stay competitive and meet evolving customer expectations.
GenAI solution: Generative AI can analyze market trends, customer feedback, and competitor strategies to identify innovation opportunities. It can generate new product ideas, design prototypes, and simulate different scenarios to test feasibility, reducing the time and cost associated with traditional R&D processes.
Operational efficiency
Challenge: Streamlining operations to maximize productivity and minimize costs while maintaining high quality.
GenAI solution: Generative AI can automate repetitive tasks, optimize workflows, and predict maintenance needs. It can analyze operational data to identify bottlenecks and recommend improvements, leading to more efficient resource utilization and reduced operational costs.
Content creation and management
Challenge: Producing and managing large volumes of high-quality content for marketing, communication, and documentation purposes.
GenAI solution: Generative AI can create content such as articles, reports, marketing copy, and social media posts quickly and consistently. It can also assist in managing and organizing content by tagging, categorizing, and summarizing documents, ensuring that information is easily accessible and up-to-date.
Quality control and assurance
Challenge: Ensuring products and services meet high standards of quality and consistency.
GenAI solution: Generative AI can monitor production processes in real-time, detecting anomalies and potential defects before they impact the final product. It can analyze data from various stages of production to identify patterns that may indicate quality issues, enabling proactive measures to maintain high standards.
Cybersecurity threats
Challenge: Protecting sensitive data and systems from increasingly sophisticated cyber threats.
GenAI solution: Generative AI can enhance cybersecurity by analyzing network traffic and identifying unusual patterns that may indicate a threat. It can generate predictive models to anticipate potential attacks and recommend preventive measures. Additionally, it can automate responses to security incidents, reducing the time and effort required to mitigate risks.
Regulatory compliance
Challenge: Navigating complex and constantly changing regulations across different regions and industries.
GenAI solution: Generative AI can continuously monitor regulatory updates and ensure that enterprise policies and procedures remain compliant. It can generate compliance reports, identify areas of non-compliance, and recommend corrective actions. This reduces the risk of penalties and helps maintain good standing with regulatory bodies.
Market adaptation
Challenge: Quickly adapting to market changes and consumer behavior shifts.
GenAI solution: Generative AI can analyze vast amounts of market data to identify emerging trends and shifts in consumer behavior. It can model various scenarios to predict market changes and recommend strategies for adaptation, ensuring that enterprises remain agile and responsive.
Decision making
Challenge: Making informed and timely decisions based on vast and complex data sets.
GenAI solution: Generative AI can synthesize large datasets and provide actionable insights through advanced analytics. It can generate predictive models to forecast outcomes and support strategic decision-making, helping leaders make informed choices quickly.
Customer insights
Challenge: Gaining deep and actionable insights into customer preferences and behavior.
GenAI solution: Generative AI can analyze customer data from multiple sources to generate detailed profiles and segmentations. It can predict future behavior and preferences, allowing for more personalized marketing and customer engagement strategies.
Talent retention
Challenge: Retaining top talent in a competitive job market.
Solution: Generative AI can analyze employee engagement and performance data to identify factors that contribute to job satisfaction and retention. It can generate personalized career development plans and recommend initiatives to enhance employee satisfaction and reduce turnover.
Generative AI presents a versatile and powerful solution to the myriad challenges faced by enterprises today. Its ability to personalize, innovate, automate, and analyze holds the promise of transforming businesses, making them more efficient, competitive, and aligned with the demands of the digital era.
Generative AI use cases for enterprises
Generative AI has transformative potential across various industries, leveraging its ability to create content, designs, and solutions autonomously. Here are some notable use cases across different sectors:
Healthcare
- Drug discovery and development:
- Generative AI designs new molecules by predicting their chemical properties and interactions, speeding up the drug discovery process and reducing costs.
- AI models can simulate the interactions between potential drug compounds and biological targets, identifying promising candidates for further testing.
- Medical imaging:
- Enhances MRI and CT images by filling in missing details and reducing noise, aiding in diagnosing diseases with higher accuracy.
- Generates high-resolution images from low-resolution inputs, improving the clarity of medical scans and enabling better diagnosis.
- Personalized medicine:
- Analyzes genetic data to create patient-specific treatment plans, predicting individual responses to treatments and optimizing healthcare outcomes.
- Generates models that consider patient demographics, medical history, and genetic information to recommend personalized treatment options, improving patient care.
Finance
- Fraud detection:
- Generates models that detect fraudulent activities by analyzing transaction patterns, user behavior, and historical data, reducing financial losses and mitigating risks.
- Uses anomaly detection algorithms to identify unusual patterns and behaviors, flagging potentially fraudulent transactions for further investigation.
- Customer service:
- Utilizes natural language generation (NLG) to provide personalized financial advice and automated customer support, improving customer satisfaction and loyalty.
- Generates personalized investment recommendations and financial plans based on individual goals, risk tolerance, and financial situation.
Manufacturing
- Product design:
- Generates innovative product designs based on specified criteria, leveraging generative design algorithms to explore a wide range of design possibilities and optimize performance.
- Uses AI to create parametric designs that can be easily modified and customized to meet specific requirements, reducing design time and costs.
- Predictive maintenance:
- Creates models that predict equipment failures before they occur by analyzing sensor data, equipment performance history, and environmental factors, optimizing maintenance schedules and reducing downtime.
- Generates real-time alerts and maintenance recommendations based on predictive analytics, enabling proactive maintenance to prevent costly breakdowns.
- Supply chain optimization:
- Generates optimized logistics and inventory management plans by analyzing historical data, demand forecasts, and supply chain constraints, improving efficiency and reducing costs.
- Uses AI to optimize production schedules, inventory levels, and distribution routes, minimizing lead times and improving supply chain resilience.
Retail
- Personalized marketing:
- Generates personalized product recommendations and marketing content based on customer preferences, purchase history, and browsing behavior, improving customer engagement and sales.
- Utilizes AI to analyze customer data and identify patterns and trends, enabling targeted marketing campaigns and promotions.
- Inventory management:
- Predicts demand and generates optimal inventory levels by analyzing historical sales data, market trends, and seasonal fluctuations, reducing stockouts and overstock situations.
- Uses AI-driven demand forecasting models to optimize inventory replenishment, minimize carrying costs, and maximize inventory turnover.
- Visual merchandising:
- Creates virtual store layouts and visual displays using augmented reality (AR) and computer vision technology, enabling retailers to design and visualize store layouts before implementation.
- Generates virtual fitting rooms and personalized product recommendations based on customer preferences and body measurements, enhancing the shopping experience and increasing sales.
Entertainment and Media
- Content creation:
- Generates scripts, music, artwork, and video content using generative AI algorithms, aiding in the creative process and reducing production time.
- Uses AI to analyze audience preferences and trends to generate personalized content recommendations and tailor content to specific audiences.
- Personalized recommendations:
- Suggests movies, music, and articles tailored to individual preferences by analyzing user behavior, demographics, and content consumption history, enhancing user experience on digital platforms.
- Utilizes collaborative filtering and content-based recommendation algorithms to generate personalized recommendations and increase user engagement and retention.
- Game development:
- Creates realistic characters, dialogues, and game environments using generative AI algorithms, speeding up the development process and enhancing game quality.
- Uses procedural content generation (PCG) techniques to generate game levels, terrain, and environments dynamically, providing infinite replayability and reducing development costs.
Education
- Personalized learning:
- Generates customized learning plans and materials based on individual student performance and needs, enabling personalized and adaptive learning experiences.
- Uses AI-driven tutoring systems to provide real-time feedback and assistance, adapting the learning pace and content to the student’s progress and learning style.
- Content creation:
- Develops educational content, including quizzes, interactive exercises, and study materials, using generative AI algorithms to supplement traditional teaching methods.
- Generates interactive simulations and virtual labs to facilitate hands-on learning and experimentation, enhancing student engagement and understanding.
- Tutoring systems:
- Creates intelligent tutoring systems that can interact with students in real-time, providing personalized assistance and feedback across various subjects and skill levels.
- Utilizes natural language processing (NLP) to analyze student responses and provide targeted feedback, helping students master complex concepts and skills more effectively.
Automotive
- Autonomous vehicles:
- Generates algorithms for self-driving cars by analyzing sensor data, traffic conditions, and environmental factors, enhancing navigation and safety systems.
- Uses AI to simulate driving scenarios and predict potential hazards, enabling autonomous vehicles to make real-time decisions and navigate complex environments.
- Design and prototyping:
- Creates innovative vehicle designs and prototypes using generative design algorithms, optimizing performance, efficiency, and safety.
- Utilizes 3D printing and additive manufacturing technologies to produce prototype parts and components quickly and cost-effectively, accelerating the vehicle development process.
- Predictive maintenance:
- Predicts vehicle maintenance needs by analyzing sensor data, vehicle performance history, and environmental conditions, improving reliability and customer satisfaction.
- Generates real-time alerts and maintenance recommendations based on predictive analytics, enabling proactive maintenance to prevent costly breakdowns and minimize downtime.
Insurance
- Risk assessment:
- Generates predictive models to assess and quantify insurance risks by analyzing historical data, market trends, and risk factors, improving underwriting accuracy and profitability.
- Utilizes machine learning algorithms to analyze customer data and predict insurance claims, enabling insurers to price policies more accurately and mitigate risk.
Logistics
- Route optimization:
- Generates optimized delivery routes by analyzing real-time traffic data and delivery schedules, reducing transportation costs and improving delivery efficiency.
- Uses AI-driven predictive analytics to forecast demand and plan inventory replenishment, optimizing warehouse operations and reducing stockouts.
- Inventory management:
- Predicts demand and generates optimal inventory levels by analyzing historical sales data, market trends, and seasonal fluctuations, reducing carrying costs and minimizing stockouts.
- Utilizes AI-driven demand forecasting models to optimize inventory replenishment and distribution, improving supply chain efficiency and customer satisfaction.
- Fleet management:
- Generates predictive maintenance schedules for vehicles and equipment by analyzing sensor data, maintenance records, and environmental conditions, reducing downtime and maintenance costs.
- Uses AI to optimize fleet routes and schedules, minimize fuel consumption, and reduce carbon emissions, improving sustainability and operational efficiency.
Private equity and principal investment
- Investment analysis:
- Generates investment models to predict returns and risks by analyzing financial data and market trends, aiding investors in decision-making and portfolio management.
- Utilizes machine learning algorithms to identify investment opportunities and optimize portfolio allocation, maximizing returns and minimizing risk.
- Due diligence:
- Automates the due diligence process by analyzing company financials, market trends, and industry benchmarks, identifying potential risks and opportunities for investment.
- Uses natural language processing (NLP) to analyze legal documents, contracts, and regulatory filings, identifying key terms and clauses that may impact investment decisions.
- Portfolio management:
- Generates portfolio optimization strategies by analyzing asset performance, market trends, and investor preferences, maximizing returns and minimizing risk.
- Utilizes AI-driven predictive analytics to monitor portfolio performance, identify emerging trends, and rebalance asset allocations, ensuring investment objectives are met.
Generative AI continues to unfold a world of possibilities across industries, promising to redefine traditional practices with its dynamic and innovative capabilities.
Optimize Your Operations With AI Agents
Optimize your workflows with ZBrain AI agents that automate tasks and empower smarter, data-driven decisions.
Benefits of generative AI for enterprises
Generative AI holds significant promise for enterprises across various industries, offering several tangible benefits:
- Enhanced creativity and innovation: Generative AI enables enterprises to automate and streamline the creative process by generating novel ideas, designs, and solutions. This can lead to breakthrough innovations and competitive advantages in product development, marketing campaigns, and more.
- Time and cost efficiency: By automating repetitive tasks such as content creation, design iterations, and prototyping, generative AI helps enterprises save time and reduce operational costs. This allows teams to focus on higher-value activities, accelerating project timelines and improving overall productivity.
- Personalization at scale: With the ability to analyze vast amounts of data and generate personalized content or recommendations, enterprise generative AI empowers businesses to deliver tailored experiences to their customers at scale. This leads to higher customer engagement, satisfaction, and, ultimately, increased revenue.
- Optimized decision-making: Generative AI algorithms can analyze complex datasets and simulate various scenarios to provide valuable insights for decision-making. Whether it’s optimizing supply chain logistics, predicting market trends, or identifying potential risks, this technology equips enterprises with data-driven intelligence to make informed decisions.
- Improved customer service: Through natural language processing and dialogue generation capabilities, generative AI enables enterprises to deploy virtual assistants and chatbots that can interact with customers in real-time. These AI-powered agents can efficiently handle customer inquiries, provide support, and personalize interactions, leading to enhanced customer satisfaction and loyalty.
- Augmented creativity and collaboration: Enterprise generative AI tools facilitate collaboration among teams by generating diverse ideas, designs, or concepts that can spark creativity and inspire collaboration. By leveraging the collective intelligence of employees and AI systems, enterprises can foster a culture of innovation and drive continuous improvement.
- Risk mitigation and compliance: Generative AI can help enterprises identify potential risks, detect anomalies, and ensure regulatory compliance across various processes and operations. By proactively addressing compliance issues and mitigating risks, businesses can safeguard their reputation, minimize legal liabilities, and maintain trust with stakeholders.
- New revenue streams: By leveraging generative AI to create unique digital assets, such as virtual characters, artwork, or music, enterprises can explore new revenue streams and business opportunities. Whether through licensing, merchandising, or content monetization, generative AI opens doors to innovative ways of monetizing intellectual property.
In summary, the adoption of generative AI offers enterprises a multitude of benefits, including enhanced creativity, efficiency, personalization, decision-making, customer service, collaboration, risk mitigation, and revenue generation. By harnessing the power of AI-driven creativity and innovation, businesses can stay ahead of the competition and thrive in today’s rapidly evolving digital landscape.
Generative AI implementation in enterprises
Generative AI implementation in enterprises marks a significant advancement in how businesses leverage technology for innovation, efficiency, and competitive advantage. This process involves integrating AI models capable of generating novel content, insights, and solutions into various facets of business operations. The journey from conceptualization to integration of generative AI in enterprises encompasses several crucial steps, tailored strategies, and considerations that ensure its success and alignment with business objectives.
Planning and strategy development
The first step towards successful generative AI implementation involves thorough planning and strategic development. Enterprises must identify specific business goals, challenges, and opportunities where generative AI can provide substantial value. This stage requires a deep understanding of generative AI capabilities and limitations, enabling businesses to set realistic expectations and measurable objectives.
Selection of generative AI models and technologies
Choosing the right generative AI models and technologies is critical. Enterprises need to evaluate various models, such as GPT for text generation or DALL·E for image creation, based on their specific use cases. This selection process also involves considering factors like model scalability, customization needs, and integration capabilities with existing enterprise systems.
Data management and model training
Effective data management is the cornerstone of generative AI implementation. Enterprises must collect, clean, and structure high-quality data for model training. The process may involve fine-tuning pre-trained models with enterprise-specific data to enhance relevance and accuracy, ensuring the AI outputs are aligned with business needs and industry standards.
Ethical considerations and compliance
Implementing generative AI necessitates addressing ethical considerations and regulatory compliance. Enterprises must establish frameworks to mitigate biases, ensure data privacy, and adhere to ethical AI use principles. This includes compliance with data protection regulations like GDPR, ensuring the responsible use of AI technologies.
Integration and deployment
Integrating generative AI into existing enterprise ecosystems requires careful planning and execution. This step involves developing or updating IT infrastructure, setting up deployment pipelines, and ensuring seamless integration with existing business processes and systems. Successful integration ensures that generative AI tools enhance operational efficiency without disrupting existing workflows.
Monitoring, evaluation, and continuous improvement
After deployment, continuous monitoring and evaluation are essential to measure the impact of generative AI against predefined KPIs and business objectives. Feedback loops should be established to gather insights and identify areas for improvement. Regular updates and refinements to AI models and applications are necessary to adapt to changing business needs and technological advancements.
Future-proofing and scalability
Looking ahead, enterprises must consider the scalability of their generative AI solutions and their ability to adapt to future technological developments. This involves staying informed about advancements in AI, preparing for the integration of next-generation models, and ensuring the AI infrastructure can scale with business growth.
The implementation of generative AI in enterprises is a complex but rewarding endeavor that requires strategic planning, careful execution, and ongoing management. By adhering to best practices and ethical standards, enterprises can leverage generative AI to drive innovation, enhance operational efficiency, and maintain a competitive edge in their respective industries.
How can LeewayHertz help your enterprise implement generative AI?
LeewayHertz offers a comprehensive suite of services to support enterprises implementing generative AI, aligning with their operational enhancement, innovation, and growth objectives. Their expertise in generative AI development encompasses a wide range of capabilities, from consulting and data analysis to the development of domain-specific models and solutions. Here’s how LeewayHertz can assist enterprises in embracing generative AI:
Tailored generative AI solutions
LeewayHertz leverages advanced technologies, including machine learning, natural language processing, and data engineering, to develop custom solutions powered by large language models (LLMs) like GPT-4, Llama, and PaLM 2. These solutions are designed to address specific business needs, facilitating streamlined operations and rapid advancements.
Integration services
LeewayHertz doesn’t just stop at building generative AI solutions; they also specialize in integrating these solutions into existing workflows. This approach ensures a seamless transition, minimizing disruption to ongoing operations and enabling organizations to leverage the transformative potential of generative AI effectively.
Engagement models
LeewayHertz offers flexible engagement models to meet diverse client needs, including dedicated development teams, team extension, and project-based collaboration. This flexibility allows for a tailored approach to each project, ensuring that the specific objectives and requirements of the client are met.
Ethical AI practices
The company places a strong emphasis on ethical AI practices, focusing on transparency, security, and privacy. This commitment ensures that the AI solutions developed and implemented are responsible and sustainable, aligning with the broader goals of trust and reliability in AI applications.
Experience and expertise
With a history of collaboration with Fortune 500 companies and a diverse portfolio of AI-driven projects, LeewayHertz has demonstrated its capability to deliver high-quality, impactful solutions across various sectors. Their team of AI experts works closely with clients to build future-ready GenAI solutions that fully realize the potential of AI technology.
Support and maintenance
LeewayHertz provides ongoing support and maintenance services post-deployment, ensuring that the generative AI solutions continue to perform optimally. This includes regular updates and technical support to address any issues or enhancements needed over time.
In conclusion, LeewayHertz, a prominent generative AI development company, offers a blend of technological expertise, flexible engagement models, and a commitment to ethical AI, making them a strong partner for enterprises looking to harness the power of generative AI. Their approach of customizing solutions to meet specific business needs, combined with their integration and support services, positions them well to help enterprises navigate the complexities of implementing generative AI technologies.
Ethical and governance strategies for generative AI deployment in enterprises
- Data privacy and security: Enterprises must prioritize data privacy and security when collecting, storing, and processing data for generative AI algorithms. Implementing robust encryption, access controls, and data anonymization techniques can help safeguard sensitive information and prevent unauthorized access or breaches.
- Bias and fairness: Enterprises need to address bias and fairness concerns inherent in generative AI systems, which may perpetuate or amplify existing biases present in training data. Employing techniques such as bias detection, fairness testing, and diverse dataset curation can help mitigate biases and ensure equitable outcomes across diverse user groups.
- Transparency and explainability: Enterprises should strive for transparency and explainability in their generative AI systems to foster trust and accountability. Providing clear explanations of how AI-generated outputs are generated and enabling users to understand the underlying processes can help mitigate concerns related to opacity and algorithmic accountability.
- Accountability and oversight: Establishing clear lines of accountability and oversight mechanisms is essential for ensuring responsible deployment and governance of generative AI in enterprises. This may involve defining roles and responsibilities for AI development, deployment, and monitoring, as well as implementing mechanisms for auditing and accountability.
- Ethical use cases and impact assessment: Enterprises should conduct thorough ethical assessments of potential use cases for generative AI deployment, considering the potential impacts on stakeholders, society, and the environment. Evaluating the ethical implications of AI-generated content, such as misinformation, deepfakes, or offensive material, is essential to mitigate risks and ensure responsible use.
- Regulatory compliance: Enterprises must ensure compliance with relevant laws, regulations, and industry standards governing the use of AI technologies, including data protection, consumer privacy, intellectual property rights, and anti-discrimination laws. Proactively staying abreast of evolving regulatory landscape and incorporating compliance requirements into AI development processes is critical for mitigating legal and reputational risks.
- Human oversight and control: Maintaining human oversight and control over generative AI systems is essential to prevent unintended consequences and ensure ethical decision-making. Enterprises should establish mechanisms for human intervention, review, and override when necessary, particularly in high-stakes applications such as autonomous vehicles, healthcare, or financial services.
- Continuous monitoring and evaluation: Enterprises should implement robust monitoring and evaluation frameworks to assess the performance, fairness, and ethical implications of generative AI systems over time. Regular audits, impact assessments, and feedback mechanisms can help identify and address emerging ethical concerns and ensure ongoing compliance with ethical and governance standards.
Ethical and governance considerations in enterprise generative AI deployment involve ensuring privacy, fairness, transparency, accountability, compliance, human oversight, and continuous evaluation. By prioritizing ethical considerations and governance principles, enterprises can harness the transformative potential of generative AI while mitigating risks and upholding ethical standards.
Enterprise deployment of generative AI: A comprehensive strategy
Generative AI is rapidly transforming industries, and enterprises are keen to harness its potential. However, effective deployment of this technology within an enterprise requires a comprehensive strategy that addresses various technical, operational, and ethical considerations. Here’s a structured approach to help enterprises successfully integrate generative AI solutions.
Customize and fine-tune for optimized performance
Enterprises must extensively customize and fine-tune foundational models to meet their unique requirements effectively. This process is critical because base models, trained on general data, do not inherently understand domain-specific or proprietary data. Fine-tuning makes models specialists in enterprise-specific tasks, leading to better performance and accuracy.
For instance, Google’s Med-PaLM 2, fine-tuned on medical data, outperforms GPT-4 in medical examinations despite being significantly smaller. Similarly, models like Vicuna and GOAT, fine-tuned on specialized datasets, achieve superior performance in their respective domains compared to larger generalist models.
Enhance security and safety
Custom-built applications allow enterprises to keep sensitive data within their secure environments, avoiding the security risks associated with sending data to external providers. This approach ensures that data handling complies with an enterprise’s security policies and regulatory requirements.
Furthermore, establishing robust monitoring and evaluation mechanisms for generative AI applications is crucial for maintaining credibility and safety, especially in customer-facing roles or sensitive operational areas. These mechanisms help mitigate risks such as inaccuracies or inappropriate outputs.
Consider the build vs. buy decision
When deploying generative AI, enterprises must evaluate each layer of the stack and make informed build versus buy decisions based on their unique requirements:
- Applications: Custom applications are preferred when specific performance is dependent on proprietary data and sensitivity. Off-the-shelf applications may suffice for less critical or generic tasks.
- Development platform: Building an internal development platform is generally reserved for entities aiming to commercialize the platform or have unique requirements. Purchasing a development platform can save resources and time, allowing focus on core business functions.
- Data engine: While building a data engine offers customization and data privacy, purchasing one can significantly speed up deployment and access high-quality tooling and expertise.
- Base foundation models: The choice between building a proprietary base model or purchasing access to commercial models depends on the specific needs and capabilities of the enterprise.
Address ethical implications and bias mitigation
Addressing the ethical implications of deploying generative AI is critical. Enterprises should implement frameworks to ensure ethical usage, including measures to detect and mitigate biases in AI models. Regular audits of AI applications for fairness and transparency are necessary to uphold ethical standards and promote trust among users and stakeholders.
Ensure scalability, flexibility, and user experience
As enterprises grow, their generative AI needs will evolve. Building scalable and flexible AI systems that can easily integrate with new technologies and data sources is crucial. This includes leveraging cloud-based solutions for elasticity and adopting modular architectures that allow for the easy addition of new features or integration with different systems.
Improving the user experience (UX) for both developers creating AI applications and the end-users interacting with them is essential. Enterprises should focus on creating intuitive interfaces and accessible applications, ensuring that generative AI technologies enhance user interactions without introducing unnecessary complexity.
Enable continuous learning and adaptation
Generative AI models can become outdated as new data emerges and business needs change. Establishing processes for continuous learning and model updating can help enterprises maintain the relevance and accuracy of their AI applications. This might involve periodic retraining of models with new data or the adoption of techniques like few-shot learning to quickly adapt models to new tasks.
Foster collaboration and cross-functional teams
The deployment of generative AI often requires collaboration across different departments within an enterprise, including IT, data science, legal, and business units. Forming cross-functional teams can facilitate the successful integration of AI by ensuring all potential impacts are considered, from technical feasibility to legal compliance and business value.
Maintain regulatory compliance and data governance
Staying compliant with regional and global regulations (e.g., GDPR, CCPA) is paramount, especially when dealing with consumer data. Enterprises should establish robust data governance policies and practices to manage data ethically and legally, including data collection, storage, processing, and sharing procedures.
Encourage experimentation and prototyping
Before full-scale deployment, it’s beneficial to prototype and test generative AI applications in controlled environments. This allows enterprises to assess the impact, identify potential issues, and gather user feedback, which can inform adjustments and improvements. An agile approach to development, with iterative cycles of testing and learning, can enhance the effectiveness of AI solutions.
Integrate with digital ecosystems
Consider the interoperability of generative AI applications with existing digital ecosystems and third-party services. This includes APIs, data exchange formats, and protocols that enable seamless integration and data flow between systems, enhancing the overall value and utility of AI applications within the enterprise.
By following this comprehensive strategy, enterprises can effectively navigate the technical, operational, and ethical considerations involved in deploying generative AI solutions, unlocking the full potential of this transformative technology while maintaining a responsible and sustainable approach.
Future of GenAI in enterprises
The future of generative AI (GenAI) in enterprises looks incredibly promising, with its potential to transform various aspects of business operations, innovation, and customer engagement. As we look ahead, several key trends and developments are likely to shape the integration and evolution of generative AI within the enterprise landscape:
1. Ubiquitous integration across sectors
Generative AI is set to become a staple across diverse sectors, including finance, healthcare, education, and entertainment, among others. Its ability to generate novel content, automate complex processes, and enhance decision-making will drive widespread adoption. Businesses will increasingly leverage generative AI to create unique customer experiences, automate content creation, personalize marketing efforts, and streamline operations.
2. Advancements in personalization and customization
As generative AI technologies evolve, their ability to offer highly personalized and customized experiences will significantly improve. Enterprises will use GenAI to deliver tailor-made content, products, and services to their customers, enhancing satisfaction and engagement. This personalization will extend beyond marketing into product development, customer service, and even into personalized learning and development programs for employees.
3. Ethical AI and governance
With the growth of generative AI comes increased scrutiny regarding ethical considerations, data privacy, and regulatory compliance. Enterprises will prioritize the development of ethical AI frameworks to address bias, ensure transparency, and protect user privacy. Governance structures will be essential to oversee AI operations, ensuring they align with ethical standards and legal requirements, including evolving global regulations.
4. Collaboration and human-AI partnerships
The future will see a shift towards more collaborative models between humans and AI. Rather than viewing AI as a replacement for human workers, enterprises will focus on human-AI partnerships that leverage the strengths of both. Generative AI will assist in enhancing human creativity, solving complex problems, and freeing employees from repetitive tasks to focus on higher-value work.
5. Innovation in AI model training and efficiency
As enterprises demand more from generative AI, there will be significant innovation in training techniques, model efficiency, and sustainability. Techniques like transfer learning, few-shot learning, and model fine-tuning will become more sophisticated, allowing businesses to develop powerful AI applications with less data, lower costs, and reduced environmental impact.
6. Expansion of AI as a Service (AIaaS)
The growth of AI as a Service (AIaaS) will enable more businesses to leverage generative AI without the need for extensive in-house expertise or resources. This will democratize access to AI technologies, allowing small and medium-sized enterprises to compete with larger organizations by utilizing state-of-the-art AI tools and platforms.
7. Breakthroughs in multimodal AI
Generative AI will increasingly become multimodal, capable of understanding and generating content across text, images, audio, and video. This multimodal capability will enable more complex and sophisticated applications, such as creating entire virtual environments for training or entertainment, enhancing digital assistants, and automating content creation across different media.
8. Enhanced security and robustness
As reliance on generative AI grows, so does the importance of ensuring these systems are secure and robust against attacks. Future developments will focus on enhancing the security of AI models, including techniques to prevent data poisoning and model theft and ensuring AI-generated content can be trusted and is free from manipulation.
The future of generative AI in enterprises is not just about technological advancements but also about how these technologies are integrated, governed, and leveraged to create value. By focusing on ethical use, human-AI collaboration, and innovative applications, enterprises can harness the full potential of generative AI to drive growth, innovation, and competitive advantage in the years to come.
Endnote
The integration of generative AI into enterprise ecosystems represents a paradigm shift in how businesses innovate, streamline operations, and engage with customers. As these AI technologies evolve, they offer unprecedented opportunities for creativity, efficiency, and personalization, enabling enterprises to stay ahead in an increasingly competitive landscape. The adoption of generative AI is not without its challenges, including ethical concerns, data privacy, and the need for robust governance frameworks. However, with strategic implementation, these obstacles can be navigated successfully, unlocking the full potential of AI to drive business transformation.
Generative AI’s ability to automate content creation, generate insights, and enhance decision-making processes across various domains signifies its critical role in the future of business. Enterprises that harness these capabilities effectively can expect not only to optimize their operations but also to foster innovation and create more meaningful connections with their customers. The importance of ethical considerations and continuous learning in the deployment of generative AI cannot be overstated, ensuring that these technologies are used responsibly and continue to evolve in alignment with enterprise goals and societal values.
Looking ahead, the trajectory of generative AI in enterprises is poised for growth, with advancements in AI models and applications expected to open new avenues for innovation and efficiency. By embracing generative AI, enterprises can navigate the complexities of the digital age, leveraging these powerful tools to unlock new opportunities, drive growth, and secure a competitive advantage in their respective industries.
Ready to harness the potential of generative AI for your enterprise? Connect with LeewayHertz for generative AI consultation and development services tailored to your business needs.
Start a conversation by filling the form
All information will be kept confidential.
Insights
AI in trend analysis: Predicting the pulse of the market
In today’s fast-paced, data-driven world, deciphering patterns and trends is more crucial than ever, serving as a compass for organizations and individuals navigating the extensive seas of information.
AI use cases and applications in private equity & principal investment
Investors use AI to analyze vast amounts of financial and non-financial data, identify patterns, and generate predictive models to help them make better investment decisions.
AI in hospitality: Use cases, applications, solution and implementation
AI has been making significant advancements in the hospitality industry, reforming various aspects of guest experiences, operational efficiency, and overall management.