Enterprise AI solutions for healthcare: Applications, benefits, technologies and development
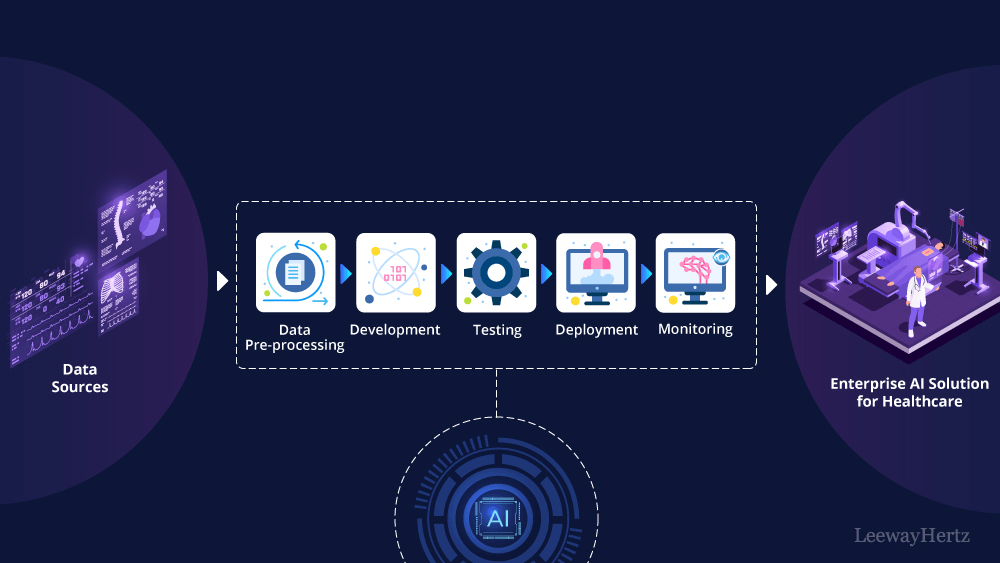
In an era defined by technological advancements, the integration of Artificial Intelligence (AI) has emerged as a crucial asset for healthcare organizations striving to navigate the complexities of modern medical practice. The healthcare sector, with its vast pools of data and intricate workflows, stands to gain immensely from the implementation of enterprise AI solutions. From optimizing operational processes to enhancing patient care delivery, AI presents a myriad of opportunities for innovation and efficiency.
At the core of its significance lies the ability of AI to analyze vast amounts of healthcare data swiftly and accurately. This capability empowers healthcare providers to make data-driven decisions, leading to improved diagnosis, personalized treatment plans, and, ultimately, better patient outcomes. Furthermore, AI-driven automation streamlines administrative tasks, liberating healthcare professionals to focus on delivering high-quality care.
This article explores the multifaceted benefits of integrating AI solutions into the framework of healthcare organizations. It delves into the pivotal role of AI in enhancing operational processes and elevating the customer experience within healthcare settings. Moreover, it outlines a strategic approach for building enterprise AI solutions tailored specifically for healthcare, emphasizing the importance of a well-defined strategy in maximizing the potential of AI integration.
With a comprehensive understanding of the significance of AI in healthcare established, the article outlines the steps to craft and implement enterprise AI solutions in healthcare systems. From conceptualization to execution, each phase of the development process is dissected, offering valuable insights and practical guidance for healthcare stakeholders embarking on this transformative journey.
- Understanding the significance of AI in healthcare
- Role of AI in enhancing operational processes and elevating the customer experience in healthcare
- Exploring AI models for building enterprise healthcare solutions
- How to build an enterprise AI solution for healthcare?
- LeewayHertz’s AI development services for healthcare
- Benefits of AI integration in healthcare workflows
- Best practices for developing enterprise AI solutions in healthcare
- How can LeewayHertz aid in the development of enterprise AI solutions for healthcare?
Understanding the significance of AI in healthcare
Artificial intelligence profoundly reshapes the landscape of healthcare delivery and management. As healthcare organizations increasingly embrace digital transformation, AI emerges as a powerful tool capable of redefining the industry in myriad ways. From diagnostic assistance to personalized treatment recommendations, AI offers unprecedented opportunities to enhance patient outcomes, enhance operational efficiency, and drive innovation across the healthcare ecosystem.
Traditionally, healthcare delivery has relied on human expertise and manual processes, leading to inefficiencies, errors, and disparities in care. AI, however, holds the promise of augmenting human capabilities and revolutionizing every aspect of healthcare delivery. By leveraging advanced algorithms, machine learning techniques, and big data analytics, AI enables healthcare providers to extract actionable insights from vast amounts of clinical data, facilitating faster and more accurate decision-making.
AI’s transformative potential in healthcare is evident across various domains, including diagnostic support, predictive analytics, precision medicine, and healthcare operations. The integration of AI into healthcare ecosystems presents a multitude of benefits and opportunities for healthcare businesses, including enhanced patient care, improved efficiency and productivity, cost savings, and innovation-driven competitive advantage.
Role of AI in enhancing operational processes and elevating the customer experience in healthcare
Operations lifecycle
AI offers a range of applications that can enhance various phases of the operations lifecycle in healthcare, leading to improved efficiency, accuracy, and patient outcomes. Here’s how AI can help in each phase:
1. Patient registration and scheduling:
- AI-powered chatbots can assist patients in filling out registration forms and scheduling appointments, improving efficiency and reducing administrative burden.
- AI algorithms can analyze historical data to predict appointment no-shows and optimize scheduling to minimize gaps in provider schedules. Appointment no-shows refer to instances where patients fail to appear for their scheduled appointments without prior notice or cancellation.
2. Patient triage:
- AI-based triage systems can help prioritize patients by analyzing symptoms and vital signs and providing recommendations for urgency based on severity.
- Natural Language Processing (NLP) can assist healthcare providers in extracting relevant information from patient descriptions, aiding in the triage process.
3. Clinical assessment and diagnosis:
- AI algorithms can assist healthcare providers in interpreting medical images, such as X-rays, MRIs, and CT scans, to aid in diagnosis and treatment planning.
- Machine learning models trained on electronic health record (EHR) data can help identify patterns and predict potential diagnoses, assisting clinicians in decision-making.
4. Treatment planning:
- AI systems can analyze patient data and medical literature to recommend personalized treatment plans based on individual characteristics and medical history.
- Decision support systems powered by AI can provide clinicians with evidence-based guidelines and recommendations for treatment options.
5. Treatment delivery:
- Robotics and automation guided by AI can assist surgeons during procedures, improving precision and reducing the risk of human error.
- AI-powered devices can monitor patients’ vital signs and alert healthcare providers to changes in real time, facilitating timely interventions.
6. Patient monitoring and follow-up:
- AI-driven predictive analytics can identify patients at high risk of complications or readmission, enabling proactive interventions and personalized follow-up care.
- Remote monitoring systems using wearable devices and AI algorithms can track patients’ health status outside of healthcare facilities, enabling early detection of problems.
7. Documentation and record-keeping:
- Natural Language Processing (NLP) can automate clinical documentation by extracting relevant information from physician notes and transcribing them into structured data fields.
- AI-powered tools can assist in coding and billing processes by automatically assigning appropriate codes based on clinical documentation, reducing errors and streamlining reimbursement.
8. Billing and coding:
- AI algorithms can analyze billing data to identify potential coding errors or fraud, helping to ensure compliance with regulations and maximize revenue integrity.
- Predictive analytics can forecast revenue trends and optimize billing processes to improve financial performance.
9. Quality assurance and improvement:
- AI-driven analytics can analyze clinical outcomes and patient satisfaction data to identify areas for improvement and implement targeted quality improvement initiatives.
- Machine learning models can analyze healthcare processes to identify inefficiencies and recommend workflow optimizations.
10. Resource management:
- AI-based forecasting models can predict patient demand for services and optimize resource allocation, ensuring sufficient staffing levels and inventory management.
- AI-powered predictive maintenance can monitor equipment performance and schedule maintenance proactively, minimizing downtime and ensuring availability.
11. Regulatory compliance:
- AI-powered compliance monitoring tools can analyze healthcare processes and documentation to ensure adherence to regulatory requirements and accreditation standards.
- Natural Language Processing (NLP) can assist in compliance reporting by extracting relevant information from regulatory documents and facilitating audit processes.
12. Patient discharge and transition of care:
- AI-driven healthcare platforms can facilitate communication among healthcare providers and ensure seamless transitions of care for patients moving between different care settings.
- Predictive analytics can identify patients at high risk of readmission or adverse outcomes post-discharge, enabling targeted interventions to prevent complications.
By leveraging AI across the operations lifecycle, healthcare organizations can unlock new opportunities for efficiency, quality, and innovation, ultimately improving patient care and organizational performance.
Customer lifecycle
AI can play a significant role in enhancing various processes within the customer lifecycle in healthcare. Here’s how AI can help in each of the mentioned processes:
1. Patient acquisition:
- AI-powered analytics can analyze patient demographics, behaviors, and preferences to target marketing efforts more effectively, reaching potential patients who are more likely to engage with the healthcare facility.
- NLP can be used to analyze patient inquiries and interactions on digital platforms, enabling personalized responses and tailored communication strategies.
2. Appointment scheduling:
- AI-powered chatbots can assist patients in scheduling appointments by analyzing availability, patient preferences, and urgency, offering convenient and efficient scheduling options.
- Predictive analytics can forecast demand for appointment slots and optimize scheduling to minimize wait times and maximize resource utilization.
3. Patient registration and check-in:
- AI-driven automation tools can streamline the registration process by extracting relevant information from patient documents and forms, reducing manual data entry errors and speeding up check-in procedures.
- Biometric authentication powered by AI can enhance security and privacy during patient registration by verifying patient identities using unique biological characteristics.
4. Clinical consultation:
- AI-based decision support systems can assist healthcare providers during consultations by providing access to relevant medical literature, clinical guidelines, and patient-specific data, aiding in diagnosis and treatment decisions.
- Virtual health assistants powered by AI can conduct preliminary assessments and gather patient history before consultations, enabling more productive interactions with healthcare providers.
5. Diagnosis and treatment:
- AI algorithms can analyze medical imaging and diagnostic test results to assist healthcare providers in interpreting findings accurately and efficiently, aiding in diagnosis and treatment planning.
- Machine learning models can analyze patient data to predict disease progression and treatment outcomes, helping healthcare providers tailor treatment plans to individual patient needs.
6. Patient education:
- AI-powered chatbots and virtual assistants can deliver personalized health education materials and resources to patients based on their specific medical conditions, preferences, and learning styles.
- Natural Language Processing (NLP) can be used to create interactive educational content and answer patient questions in real-time, improving health literacy and patient engagement.
7. Treatment delivery:
- Robotics and automation guided by AI can assist healthcare providers during surgical procedures, improving precision, reducing surgical time, and minimizing the risk of complications.
- AI-driven monitoring systems can continuously monitor patients during treatments, detecting early signs of deterioration and alerting healthcare providers to intervene promptly.
8. Follow-up care:
- AI-powered predictive analytics can identify patients at high risk of readmission or complications, enabling proactive interventions and personalized follow-up care plans.
- Virtual follow-up consultations facilitated by AI-driven telemedicine platforms can provide convenient access to healthcare providers for monitoring patients’ progress and addressing any concerns remotely.
9. Billing and payment:
- AI algorithms can automate billing processes by analyzing healthcare services rendered, insurance coverage, and reimbursement rules to generate accurate invoices and claims.
- Predictive analytics can forecast revenue trends and optimize billing strategies to improve financial performance and minimize revenue cycle management inefficiencies.
10. Patient satisfaction and feedback:
- AI-driven sentiment analysis can analyze patient feedback and online reviews to identify trends, sentiments, and areas for improvement in healthcare services and patient experiences.
- Natural Language Processing (NLP) can categorize and prioritize patient concerns and complaints, enabling healthcare providers to address them promptly and effectively.
11. Patient retention:
- AI-powered personalized engagement platforms can tailor communications and outreach efforts to individual patient preferences, fostering stronger connections and loyalty to the healthcare facility.
- Predictive analytics can identify patients at risk of disengagement or attrition, enabling proactive retention strategies and targeted interventions to improve patient retention rates.
12. Referral management:
- AI-driven referral management systems can analyze patient needs, provider specialties, and network availability to match patients with the most appropriate healthcare providers for specialized care or additional services.
- Automation tools powered by AI can streamline the referral process, reducing administrative burden and ensuring timely coordination of care between different healthcare providers.
Overall, AI technologies hold great promise in transforming both the customer experience and operational efficiency in healthcare. However, it’s crucial to prioritize ethical considerations, validate AI solutions for accuracy and effectiveness, and seamlessly integrate them into existing workflows to ensure optimal benefits for both patients and healthcare providers.
Optimize Your Operations With AI Agents
Optimize your workflows with ZBrain AI agents that automate tasks and empower smarter, data-driven decisions.
Exploring AI models for building enterprise healthcare solutions
Several enterprise AI models are commonly employed in healthcare to develop comprehensive solutions tailored to various challenges and tasks. Here are some types of AI models used in building enterprise AI solutions for healthcare:
Diagnostic imaging models
In healthcare, diagnostic imaging plays a significant role in disease detection and treatment planning. Among the various AI models employed in this domain, Convolutional Neural Networks (CNNs) stand out for their widespread use in medical image analysis tasks. These include identifying abnormalities in X-rays, MRIs, CT scans, and pathology slides. Additionally, Generative Adversarial Networks (GANs) are utilized to generate synthetic medical images, augmenting limited datasets and enhancing model performance. Transfer learning further refines diagnostic accuracy by fine-tuning pre-trained models on medical imaging datasets, reducing the need for extensive labeled data.
Clinical decision support models
Clinical decision-making often relies on complex assessments of patient data. AI models, such as Rule-based Systems, provide explicit guidelines and decision rules to aid healthcare providers in making informed choices regarding medication dosing or treatment recommendations. Bayesian Networks leverage probabilistic relationships between clinical variables to support diagnostic reasoning and treatment planning. Expert Systems incorporate domain-specific knowledge to offer recommendations in intricate medical scenarios, thereby enriching the decision-making process for clinicians.
Natural Language Processing (NLP) models
With the abundance of unstructured clinical text data, NLP models play a vital role in extracting meaningful insights. Named Entity Recognition (NER) identifies and extracts entities such as medical conditions, treatments, and patient demographics from clinical notes. Clinical Text Classification Models categorize clinical literature for tasks like disease classification or severity assessment. Question Answering Systems provide accurate responses to clinical queries posed in natural language, enabling healthcare professionals to access relevant information efficiently.
Patient outcome prediction models
Predicting patient outcomes is critical for personalized treatment planning. Logistic Regression Models assess the likelihood of adverse medical events based on patient demographics and clinical variables. Random Forests and Gradient Boosting Models integrate diverse clinical features and temporal data to forecast patient outcomes. Survival Analysis Models evaluate the probability of survival or time-to-event outcomes for patients with chronic conditions, aiding in prognosis estimation and treatment planning.
Population health management models
Population health management requires a comprehensive understanding of community health dynamics. Clustering Algorithms group patients with similar characteristics to identify high-risk cohorts for targeted interventions. Predictive Analytics Models forecast disease prevalence and healthcare resource utilization to inform public health strategies. Social Determinants of Health (SDOH) Models integrate social and environmental data with clinical information to address underlying factors influencing health outcomes within communities.
By leveraging these diverse AI models, healthcare organizations can develop innovative solutions to enhance patient care, improve clinical decision-making, and optimize healthcare delivery processes effectively.
How to build an enterprise AI solution for healthcare?
Building an enterprise AI solution in healthcare involves harnessing advanced technologies to enhance patient care, streamline operations, and improve outcomes within healthcare organizations. These solutions can encompass areas such as medical imaging analysis, patient diagnosis, treatment recommendations, and healthcare resource allocation. Let’s break down the process of building such AI solutions for healthcare businesses:
- The process starts with gathering data from diverse sources such as electronic health records (EHRs), medical devices, wearables, and research databases. This data includes patient demographics, medical history, diagnostic tests, treatment outcomes, and more.
- Once collected, the data undergoes preprocessing to clean, normalize, and standardize it for analysis. This step may also involve anonymizing patient information to comply with privacy regulations like HIPAA (Health Insurance Portability and Accountability Act) in the United States.
- Following data preprocessing, machine learning models are developed utilizing this refined data to tackle specific healthcare challenges. These models may encompass various techniques, including supervised learning for predictive analytics (e.g., disease diagnosis, patient prognosis), unsupervised learning for clustering and anomaly detection, and natural language processing (NLP) for extracting insights from clinical notes or medical literature.
- Once the models are developed, they undergo rigorous training and validation using historical healthcare data. This process involves splitting the data into training, validation, and testing sets, employing techniques such as cross-validation and hyperparameter tuning to optimize model performance. Validation ensures that the models can generalize well to unseen data and are robust across different patient demographics and clinical contexts.
- Next, the models are deployed into the existing healthcare infrastructure, seamlessly integrating with EHR systems, clinical decision support tools, telemedicine platforms, and other healthcare IT systems. This deployment phase involves careful consideration of interoperability standards, data privacy requirements, and regulatory compliance to ensure smooth integration and adherence to industry standards.
- Post-deployment, continuous monitoring and maintenance of the AI solution are imperative to uphold its efficacy and reliability. This includes real-time monitoring of model performance in clinical settings, detecting any drift or degradation in performance, and promptly addressing any issues or errors that arise. Regular updates and improvements to the models are made based on feedback from healthcare providers and evolving patient needs, ensuring that the AI solution remains effective and aligned with the latest advancements in healthcare.
While the process outlined above applies broadly to building enterprise AI solutions for healthcare businesses, it can be customized to specific use cases. For example, let’s consider the process in the context of developing an AI-powered system for optimizing patient scheduling and resource allocation.
Problem formulation:
Consider a scenario where a healthcare organization seeks to streamline its operations by developing an AI-powered system for optimizing patient scheduling and resource allocation. The primary objective is to enhance operational efficiency, minimize appointment wait times, and improve patient satisfaction by leveraging data-driven insights to optimize the scheduling of appointments and allocation of resources within the healthcare facility.
Data sources:
There can be many data sources used for data ingestion. A few of them include the following:
Electronic Health Records (EHR): EHR systems store comprehensive patient records encompassing appointment histories, medical backgrounds, and demographic details. These records provide valuable insights into patients’ health statuses, treatment histories, and healthcare interactions, serving as a foundational resource for informed decision-making and personalized care delivery within healthcare organizations.
Appointment booking system: Appointment booking systems capture crucial data regarding appointment schedules, healthcare provider availability, and facility resources. These systems facilitate efficient scheduling, resource utilization, and patient access by maintaining real-time records of appointment slots, provider availability, and resource allocations, contributing to streamlined operations and improved patient experiences.
Patient feedback surveys: Patient feedback surveys collect valuable insights into patients’ appointment experiences, waiting times, and satisfaction levels. By soliciting feedback directly from patients, healthcare organizations can gauge patient perceptions, identify areas for improvement, and implement targeted interventions to enhance service quality and patient satisfaction. Patient feedback surveys serve as a valuable tool for continuous quality improvement and patient-centered care delivery.
Healthcare provider availability: Data on the availability and scheduling preferences of healthcare providers is essential for optimizing appointment scheduling and resource allocation. By capturing information on providers’ availability, shift schedules, and preferences, healthcare organizations can ensure equitable distribution of workload, minimize appointment wait times, and maximize provider productivity. This data enables efficient workforce management and enhances operational efficiency within healthcare delivery settings.
External data APIs: In addition to internal data sources, healthcare organizations can leverage external data APIs to access supplementary information relevant to patient care. These APIs enable the integration of external data sources such as insurance claims data, public health databases, and pharmaceutical records.
Data ingestion and pre-processing:
There are several steps to ingest and pre-process data after data collection, which include:
Data cleaning: This step involves identifying and eliminating duplicates, errors, and inconsistencies from appointment and patient records. By ensuring data cleanliness, healthcare organizations can maintain the integrity and accuracy of their datasets, thereby improving the reliability of subsequent analyses and model development.
Data integration: Integrating data from various sources, such as Electronic Health Records (EHR) systems, appointment booking systems, and patient feedback surveys, is essential for creating a comprehensive and unified dataset. By combining data from disparate sources, healthcare organizations can gain a holistic view of patient interactions and experiences, enabling more informed decision-making and resource allocation.
Feature engineering: Extracting relevant features from the integrated dataset, such as appointment time, duration, patient demographics, and provider availability, allows for the creation of informative variables that can drive scheduling decisions. By identifying and engineering meaningful features, healthcare organizations can capture important patterns and relationships in the data, facilitating more accurate predictions and optimizations in scheduling and resource allocation processes.
Data pipeline:
ETL processes: Extracting, transforming, and loading data into a centralized data repository involves retrieving data from various sources, transforming it into a standardized format, and loading it into a centralized location for analysis and modeling. This ETL (Extract, Transform, Load) process ensures data consistency and accessibility, laying the foundation for subsequent analyses and insights.
Data quality assurance: Conducting quality checks on the ingested data is essential for ensuring its accuracy, completeness, and consistency. By implementing data quality assurance measures, healthcare organizations can identify and rectify errors or discrepancies in the data, mitigating the risk of misleading or biased analyses. This ensures that subsequent analyses and model development efforts are based on reliable and high-quality data, ultimately leading to more robust and trustworthy insights.
Model development:
Algorithm selection: Carefully selecting algorithms suitable for predicting optimal appointment schedules and resource allocations involves considering factors such as the complexity of the data, the desired level of accuracy, and computational efficiency. By choosing the right algorithms, healthcare organizations can ensure that their AI solution effectively addresses their specific scheduling and resource allocation challenges.
Data splitting/segregation: Divide the historical data into two or more sets. Most of the data is used for training the model (training set), and a smaller portion is set aside to evaluate the model’s performance (validation or test set).
Model training: Training the selected algorithms involves feeding them with historical appointment data to identify patterns and trends in patient scheduling and resource usage. Through iterative learning processes, the algorithms adjust their internal parameters to minimize errors and improve predictive accuracy, enabling them to make informed recommendations for appointment scheduling and resource allocation decisions.
Hyperparameter tuning: Optimizing the model’s hyperparameters is crucial for enhancing its performance and effectiveness in resource allocation. Hyperparameters are configurable settings that influence the behavior and performance of the model, such as learning rate, regularization strength, and tree depth. Healthcare organizations can fine-tune their AI model to achieve optimal performance and better meet their operational objectives by systematically tuning these hyperparameters using techniques like grid search or random search.
Model evaluation:
Cross-validation: Assessing the model’s performance through techniques like cross-validation ensures its robustness and reliability across different datasets. Cross-validation involves partitioning the data into multiple subsets, training the model on different combinations of these subsets, and evaluating its performance on the remaining data. By validating the model’s performance on independent data subsets, healthcare organizations can gain confidence in its generalization ability and mitigate overfitting or underfitting issues.
Performance metrics: Evaluating the model’s performance using metrics such as patient wait times, appointment utilization rates, and provider efficiency offers valuable insights into its effectiveness in addressing scheduling and resource allocation challenges. These performance metrics serve as tangible measures of the model’s impact on operational efficiency and patient experiences, allowing healthcare organizations to identify areas for improvement and optimize their AI solution for maximum effectiveness.
User Interface (UI) development:
- Initial UI design: Concurrently with model development, an initial user interface is crafted. This interface caters to end-users, such as healthcare providers and administrators, facilitating their interaction with the patient scheduling and resource allocation system.
- Displaying results: The UI encompasses features like showcasing scheduling recommendations, resource allocation decisions, and real-time updates on patient flow. Additionally, visualizations of key metrics influencing scheduling and resource allocation may be included to enhance decision-making transparency.
Integration with workflow:
- Connecting models to UI: Trained models are seamlessly integrated into the UI to ensure smooth data flow between the interface and the models. The UI acts as a front-end for healthcare professionals to interact with the scheduling and resource allocation system, enabling them to input data, receive recommendations, and make informed decisions.
- Decision outputs: The results generated by the models, such as optimized appointment schedules and resource allocation plans, are conveyed through the UI. This may involve presenting explanations of the factors influencing scheduling decisions and any additional information necessary for compliance or user understanding.
The “development” stage is typically iterative, with continuous refinement based on feedback from model performance, user interactions, and evolving business requirements. Adjustments to both the models and the user interface are made iteratively to ensure alignment with organizational goals and user needs.
Deployment:
Containerization: This involves packaging the AI models and their associated code, along with any dependencies and runtime environments, into containers. Containers are lightweight, portable units that encapsulate everything needed to run an application, ensuring consistency across different environments. By containerizing the AI models, healthcare organizations can deploy them consistently across various healthcare environments, making deployment easier and more reliable.
Kubernetes deployment: Kubernetes is a powerful tool for orchestrating and managing containerized applications. It automates the deployment, scaling, and management of containers, ensuring optimal resource utilization and fault tolerance. By utilizing Kubernetes for deploying AI models, healthcare organizations can efficiently manage their resources, scale applications based on demand, and ensure high availability and reliability.
Microservices architecture: Implementing the scheduling and resource allocation models as microservices involves breaking down the AI solution into smaller, independently deployable services that perform specific functions. Microservices architecture enables greater flexibility, agility, and scalability in integrating AI solutions with existing systems and workflows. It also facilitates easier maintenance, updates, and enhancements, as each microservice can be developed, deployed, and scaled independently.
API exposures: Exposing well-defined APIs allows seamless integration of AI solutions with other healthcare systems and applications. APIs serve as standardized interfaces that enable communication and interaction between different software components. By offering accessible APIs for the AI models, healthcare organizations can easily integrate them into existing systems such as appointment booking platforms, electronic health record (EHR) systems, and other healthcare IT infrastructure components.
Consumption layer: Building interfaces to convey scheduling recommendations and resource allocation decisions to healthcare providers and administrators is crucial for optimizing AI solution utilization. The consumption layer comprises user interfaces, APIs, and process interfaces that enable healthcare providers and administrators to interact with and consume the outputs of the AI models. These interfaces provide actionable insights and recommendations, facilitating informed decision-making and improving operational efficiency within healthcare organizations.
Monitoring and maintenance:
Model performance metrics: Implementing robust monitoring mechanisms within healthcare AI systems enables the tracking of key performance metrics such as accuracy, sensitivity, specificity, and AUC (Area Under the Curve). By continuously assessing these metrics, healthcare organizations can gauge the effectiveness and reliability of their AI models in clinical settings, ensuring high-quality patient care and safety.
Data drift detection: Monitoring changes in appointment patterns and patient preferences over time is essential for maintaining the relevance and effectiveness of the model. Detecting shifts in data distributions enables timely updates to the model, ensuring its predictions remain accurate and aligned with evolving patient needs and preferences. This proactive approach helps healthcare organizations stay ahead of changing trends and maintain the quality of their scheduling and resource allocation processes.
Error logging: Logging errors and exceptions in healthcare AI systems facilitate prompt identification and resolution of issues. By recording discrepancies between expected and actual model outputs, healthcare organizations can swiftly address errors or anomalies, minimizing potential risks to patient safety and data integrity.
Logging and auditing:
Audit trails: Maintaining comprehensive audit trails for healthcare AI models is critical for compliance and retrospective analysis. These audit trails document all model decisions, timestamps, and input data, providing transparency and accountability in clinical decision-making processes.
Logging changes: Logging changes to the model, code, or configurations ensures traceability and transparency in healthcare AI development. This practice enables healthcare organizations to understand the context of model behavior and facilitates troubleshooting and debugging efforts in case of issues or discrepancies.
Alerting and notifications:
Alerts for anomalies: Implementing alerting mechanisms to notify relevant stakeholders of anomalies or issues in healthcare AI systems is essential for proactive risk management. By promptly identifying deviations from expected behavior, healthcare organizations can take corrective actions to mitigate potential impacts on patient care and safety.
Threshold monitoring: Setting thresholds for key performance indicators and monitoring them enables timely detection of deviations in model performance. For instance, in a diagnostic imaging model, a threshold could be set for the sensitivity and specificity rates. If the sensitivity drops below 90% or the specificity falls below 85%, it could trigger an alert for immediate investigation. Triggering alerts when thresholds are exceeded prompts immediate investigation and intervention, ensuring the continued reliability and effectiveness of healthcare AI systems in clinical practice.
Security and compliance: Upholding security and compliance standards is paramount in healthcare AI solutions to safeguard patient data and maintain trust. By implementing robust security measures and adhering to industry regulations, such as HIPAA, in the United States, healthcare organizations ensure the confidentiality, integrity, and availability of sensitive information. Compliance with data privacy and security standards not only protects patient privacy but also mitigates legal and reputational risks for the organization.
Developing an enterprise AI solution for streamlining healthcare operations involves leveraging AI technologies to optimize patient scheduling, resource allocation, and operational efficiency, ultimately improving the quality of care and patient experiences.
Optimize Your Operations With AI Agents
Optimize your workflows with ZBrain AI agents that automate tasks and empower smarter, data-driven decisions.
LeewayHertz’s AI development services for healthcare
At LeewayHertz, we develop customized AI solutions tailored to the dynamic needs of the healthcare industry. Our strategic AI/ML consulting enables healthcare organizations to leverage artificial intelligence for enhanced diagnostic accuracy, improved patient care, and optimized operational efficiency.
Our proficiency in developing Proof of Concepts (PoCs) and Minimum Viable Products (MVPs) allows healthcare providers to test the real-world impact of AI tools, ensuring that solutions are both effective and specifically designed to meet the sector’s unique challenges.
Our expertise in generative AI transforms routine tasks such as patient data management, appointment scheduling, and reporting processes, automating these tasks to free up medical professionals for more patient-centric roles.
By fine-tuning large language models to the specific terminology and intricacies of medical diagnostics and patient interactions, LeewayHertz enhances the accuracy and relevance of AI-driven communications and analyses.
Additionally, we ensure that these AI systems integrate seamlessly with existing healthcare infrastructures, enhancing data interoperability and decision-making processes in healthcare settings.
Our AI solutions development expertise
AI solutions development for healthcare typically involves creating systems that enhance diagnostic precision, automate routine administrative tasks, and personalize patient care. These solutions integrate key components such as data aggregation technologies, which compile and analyze health data from diverse sources, including electronic health records (EHRs), wearable devices, and genomic databases. This comprehensive data foundation supports predictive analytics capabilities, allowing for the anticipation of patient health trends and personalized treatment plans. Additionally, machine learning algorithms help tailor healthcare interventions to individual patient needs, ensuring optimal outcomes. These solutions cover patient risk assessment, treatment prediction, resource allocation, and chronic disease management.
Overall, AI solutions in healthcare aim to optimize health outcomes, improve the efficiency of healthcare delivery, and enhance the patient experience.
AI agent/copilot development for healthcare
LeewayHertz builds custom AI agents and copilots that enhance various healthcare operations, enabling institutions to save time and resources while facilitating faster and more informed decision-making. Here is how they help:
Patient diagnosis and treatment:
- Analyze medical imaging and diagnostic data, improving the accuracy and speed of diagnoses.
- Automate the generation of treatment plans based on patient data and prevailing medical guidelines, ensuring personalized care.
- Provide real-time decision support for healthcare professionals during patient interactions, enhancing treatment effectiveness.
Operational efficiency and management:
- Optimize hospital workflow management, from patient intake and scheduling to resource allocation and discharge planning.
- Streamline and automate inventory management, ensuring that medical supplies and equipment are efficiently stocked and replenished.
- Forecast patient admissions and manage staffing levels effectively, improving service delivery.
Patient engagement and monitoring:
- Develop personalized patient engagement strategies to analyze behavior patterns and preferences, improving health outcomes through tailored communication.
- Implement remote monitoring solutions to track patient health data in real time, enabling proactive care and early intervention for at-risk patients.
- Enhance patient education and compliance with treatment protocols.
Data management and security:
- Ensure the security and privacy of sensitive patient data.
- Enhance data interoperability across healthcare systems to improve collaboration and information sharing among providers.
- Automate the extraction and analysis of health data, enabling more accurate and efficient research and reporting.
Regulatory compliance and quality assurance:
- Monitor and ensure compliance with healthcare regulations and standards, reducing the risk of non-compliance and associated penalties.
- Implement quality assurance protocols to continuously evaluate and improve healthcare services and patient safety.
- Automate the documentation process to meet legal and regulatory requirements, ensuring thorough and accurate medical records.
LeewayHertz’s AI agents and copilots streamline healthcare processes and significantly enhance healthcare providers’ capabilities. By integrating these advanced AI solutions into existing healthcare infrastructures, organizations can navigate the complex landscape of healthcare delivery with greater precision, efficiency, and care, achieving a substantial competitive advantage in the healthcare industry.
Benefits of AI integration in healthcare workflows
Integrating AI into healthcare workflows offers numerous benefits that can significantly improve patient outcomes, streamline processes, and enhance overall efficiency. Some of the key benefits include:
- Enhanced diagnostic accuracy: AI algorithms exhibit high precision in analyzing medical images, including X-rays, MRIs, and CT scans, thereby assisting healthcare providers in early and accurate disease diagnosis.
- Personalized treatment plans: Using AI-driven predictive analytics, patient data can be analyzed to customize treatment plans according to individual characteristics, medical history, genetic makeup, and lifestyle factors. This approach results in more effective and personalized care for each patient.
- Improved operational efficiency: AI-powered tools automate administrative tasks, such as scheduling appointments, managing medical records, and processing insurance claims, allowing healthcare professionals to focus more on patient care.
- Early disease detection and prevention: By analyzing extensive patient data, AI algorithms can identify patterns and risk factors linked to different diseases, enabling early detection and proactive interventions to halt disease progression.
- Optimized resource allocation: AI-driven predictive modeling can forecast patient admission rates, identify high-risk patients, and optimize resource allocation within healthcare facilities, ensuring that the right resources are available at the right time and place.
- Drug discovery and development: AI algorithms can analyze molecular data and predict the efficacy and safety of potential drug candidates, accelerating the drug discovery and development process and potentially leading to the discovery of novel treatments for diseases.
- Remote monitoring and telemedicine: AI-powered wearable devices and remote monitoring systems facilitate continuous monitoring of patients’ health metrics, enabling timely interventions and decreasing the necessity for in-person visits, which is particularly beneficial for patients with chronic conditions or those residing in remote areas.
- Reduced medication errors: AI systems can flag potential medication errors, alert healthcare providers to adverse drug interactions, and offer decision support tools to reduce diagnostic errors, ultimately improving patient safety.
- Cost reduction: By automating repetitive tasks, optimizing workflows, and preventing costly medical errors, AI integration can assist healthcare organizations in reducing operational costs and improving the overall efficiency of healthcare delivery.
- Continuous learning and improvement: AI systems can continuously learn from new data and experiences, improving their accuracy and effectiveness over time and facilitating ongoing advancements in healthcare practices and treatments.
Overall, integrating AI into healthcare workflows can redefine healthcare service delivery, improve patient outcomes, and drive efficiencies across the healthcare ecosystem.
Best practices for developing enterprise AI solutions in healthcare
Building an enterprise AI solution for a healthcare business involves several critical considerations and best practices to ensure effectiveness, compliance, and ethical use. Here are some tips and considerations:
- Data privacy and security: Healthcare data is highly sensitive and subject to strict privacy regulations (e.g., HIPAA in the United States). Ensure that the AI solution complies with all relevant data protection laws and industry standards. Implement robust security measures to safeguard patient information from unauthorized access or breaches.
- Ethical use of AI: Develop the AI solution with ethical principles in mind, ensuring fairness, transparency, and accountability. Avoid bias in data and algorithms, and regularly assess the model’s performance to mitigate any unintended consequences.
- Interoperability: Ensure that the AI solution can integrate seamlessly with existing healthcare systems, such as electronic health records (EHRs), medical imaging platforms, and other clinical applications. Interoperability facilitates data exchange and improves workflow efficiency.
- Clinical validation: Thoroughly validate the AI solution in clinical settings to ensure its accuracy, reliability, and safety. Collaborate with healthcare professionals to assess the solution’s clinical utility and its potential impact on patient outcomes.
- Scalability and flexibility: Design the AI solution to scale effectively to accommodate growing data volumes and user demands. Ensure flexibility to adapt to evolving healthcare requirements and technological advancements.
- Regulatory compliance: Stay informed about regulatory requirements specific to healthcare AI solutions, such as FDA clearance (for medical devices) or CE marking (for European markets). Comply with all applicable regulations and standards to ensure compliance and lawful entry into a market.
- Human oversight and interpretability: Incorporate mechanisms for human oversight, allowing healthcare professionals to interpret AI-generated insights and make informed decisions. Ensure that the AI model’s outputs are explainable and understandable to users, promoting trust and acceptance.
- Continuous monitoring and improvement: Implement mechanisms for continuous monitoring of the AI solution’s performance in real-world settings. Gather feedback from users and stakeholders to identify areas for improvement and iterate accordingly.
- Patient-centric design: Prioritize patient needs and experiences throughout the development process. Design the AI solution to enhance patient outcomes, improve access to care, and empower individuals to actively participate in their healthcare journey.
- Collaboration and knowledge sharing: Foster collaboration among multidisciplinary teams, including data scientists, clinicians, IT professionals, and healthcare administrators. Encourage knowledge sharing and cross-functional communication to leverage diverse expertise and perspectives.
- Cost-effectiveness: Consider the cost implications of developing and implementing the AI solution, including initial development costs, ongoing maintenance expenses, and potential return on investment (ROI). Strive to deliver value to the healthcare organization while optimizing resource utilization.
By adhering to these tips and considerations, healthcare businesses can build AI solutions that deliver meaningful benefits to patients, providers, and the broader healthcare ecosystem while ensuring compliance, ethics, and sustainability.
How can LeewayHertz aid in the development of enterprise AI solutions for healthcare?
LeewayHertz offers comprehensive expertise and support in developing enterprise AI solutions tailored specifically for the healthcare industry. Here’s how we can aid in the development of AI solutions for healthcare businesses:
Industry experience: Benefit from our deep understanding of the healthcare sector and its unique challenges. Our team has extensive experience working with healthcare organizations, allowing us to develop AI solutions that address industry-specific needs effectively.
Customized solutions: Collaborate with our team to design and develop custom AI solutions that meet your organization’s specific requirements. Whether it’s improving patient outcomes, optimizing workflows, or enhancing diagnostic accuracy, we tailor our solutions to align with your goals and objectives.
Advanced technologies: Leverage the latest advancements in AI technologies, including Machine Learning(ML), Natural Language Processing (NLP), computer vision, and predictive analytics. Our expertise in these areas enables us to build sophisticated AI-driven applications that deliver tangible benefits to healthcare providers and patients alike.
End-to-end support: We offer extensive support spanning the entire development lifecycle, from conceptualization to deployment and ongoing maintenance. Our team of skilled AI developers, data scientists, and project managers ensures seamless execution and timely delivery of your AI solution.
Data security and compliance: Rest assured that your healthcare data is in safe hands. We adhere to stringent security measures and regulatory compliance standards to protect sensitive patient information and ensure data privacy at every stage of the development process.
Continuous improvement: Our dedication to excellence goes beyond the initial implementation of your AI solution. We continuously monitor and optimize performance, incorporating feedback and making enhancements as needed to ensure the ongoing success of your healthcare AI solution.
By partnering with LeewayHertz, healthcare organizations can harness the power of AI to drive innovation, improve patient care, and achieve their strategic objectives in an ever-evolving healthcare landscape.
Endnote
Designing and implementing AI solutions for healthcare businesses requires a strategic approach that considers the unique challenges and opportunities within the industry. By understanding the significance of AI in healthcare and recognizing its transformative potential, organizations can harness the power of AI to enhance patient care, improve operational efficiency, and drive innovation. The process of building enterprise AI solutions involves leveraging advanced technologies, such as machine learning, natural language processing, and predictive analytics, to analyze vast amounts of healthcare data and extract actionable insights. AI solutions for healthcare businesses empower organizations to make informed decisions, optimize workflows, and improve patient care delivery.
Additionally, it requires collaboration among multidisciplinary teams, including clinicians, data scientists, and IT professionals, to ensure the successful implementation and integration of AI into existing workflows. Moreover, organizations must prioritize data privacy, security, and regulatory compliance throughout the development process to safeguard patient information and maintain trust in AI-driven solutions. By adopting a strategic and holistic approach to building enterprise AI solutions, healthcare organizations can unlock new possibilities for delivering high-quality, personalized care and driving positive outcomes for patients and stakeholders alike.
Ready to build your enterprise AI solution for streamlining your healthcare business operations? Partner with LeewayHertz for expert AI consulting and development services tailored to your specific needs.
Start a conversation by filling the form
All information will be kept confidential.