A complete knowledge guide on Digital Twins
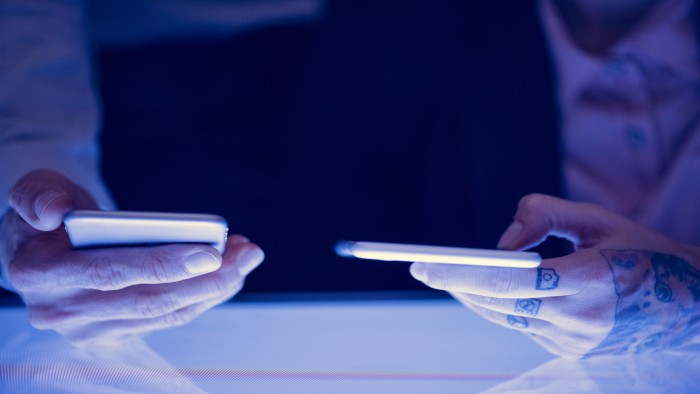
The successful IoT deployments have enabled many paradigms associated with digital transformation to drive concrete business outcomes in the industry. An idea among this rapidly gaining popularity is the “digital twin.” Gartner mentioned digital twin as one of the top 10 strategic technology trends for 2017, 2018 and 2019. It says within three to five years, billions of things will be represented by digital twins.
According to marketsandmarkets, the global market size of the digital twin in 2020 was 3.1 billion USD and is expected to reach 48.2 billion USD by 2026 at a CAGR of 58%.
Digital twins provide a glimpse of what’s happening with physical assets now or what can happen in the future. Let’s begin with understanding the basics of the digital twin.
- What is a digital twin?
- How does digital twin work?
- How to create a digital twin?
- A five-level digital twin taxonomy
- What are the applications of digital twins?
What is a digital twin?
In most simple terms, a digital twin is defined as a virtual representation of a physical thing. A thing could be a jet engine, a car, a building or a bridge. The sensors connected to these physical assets collect data that is mapped onto the virtual model. Digital twin enables people to see vital information about how the physical thing operates in the real world.
Technologies including Artificial Intelligence, Machine Learning, Deep Learning, IoT, sensor, simulation, connectivity and big data have led to sophisticated digital twins.
The main characteristics of digital twin are:
- Connectivity: A digital twin is based on connectivity. It enables connection between the physical element and its digital counterpart. The sensors create the connectivity of physical products that obtain, integrate and communicate data using various integration technologies.
- Homogenization: Digital twins are both the consequence and enabler of homogenization of data. It allows the decoupling of information from its physical form.
- Reprogrammable and Smart: Digital twins automatically enable re-programmability through sensors, artificial intelligence techniques and predictive analysis.
- Digital traces: Digital twin technologies leave digital traces. The trails are helpful to diagnose the source of the problem that occurred in case of machine malfunctions.
- Modularity: Modularity is referred to the design and customization of products and production modules. The addition of modularity to functional models helps manufacturers gain the ability to tweak machines and models.
How does digital twin work?
A digital twin comprises three main elements:
Past data: It includes historical performance data of machines, specific systems and overall processes.
Present data: Real-time data comprises sensor data, outputs from manufacturing platforms and systems throughout the distribution chain.
Future data: It is the predicted data and insights from Machine learning algorithms and inputs from engineers.
Digital twin performs the following three operational steps:
Step 1: Data collection and simulation
The data is collected and simulated in a virtual copy of assets. The collected information is either stored locally or on a decentralized cloud.
Step 2: Applying cognitive insights
After receiving data from the simulation, parameters are applied to tangible assets and integrated for precise virtual representation. Operation is done on the data to understand its variances and tolerances. Techniques are used for machine-to-machine communication, natural language processing, acoustical video analytics and more to help understand the dynamics of the information that is being presented.
Step 3: Dynamic recalibration
The collected information and cognition are used to dynamically recalibrate the environment affecting the design, the build, and the operations phases of everything done around that particular device. The integration of data in real and virtual representations helps in optimizing the performance of tangible assets.
How to create a digital twin?
AWS IoT Core and Microsoft Azure IoT are the next-generation IoT platforms that model the real world into the digital world. Azure Digital Twins is an IoT platform that helps create a digital representation of real-world objects, business processes, places, etc. It also helps to gain insights to drive products better, optimize operations and costs and provide enhanced customer experience. The execution process of Azure IoT digital twin involves:
- Open modeling language for creating custom domain models of any connected environment leveraging Digital Twins Definition Language.
- It provides a live execution environment that brings digital twins to life through a live graph representation.
- It uses IoT to connect the assets, including IoT devices using Login Apps, Azure IoT Hub and REST APIs.
- It provides output to Time Series Insights, analytics and storage using event routes to downstream services like Azure Synapse Analytics.
The creation of a digital twin is carried out under the following three stages:
Design
The design of a digital twin consists of two main elements:
- Select the enabling technology to integrate the physical asset with its digital twin to enable the real-time flow of operational and transactional information. It is also essential to be clear about the type of device required. Modeling software is needed to design a 3D representation of the asset. Moreover, it is crucial to know who will access the information through digital twin and control the physical asset.
- Understand the type of information required across the asset’s life cycle, where this information is stored and how to access and use it. Structure the information in a reusable way that is quickly and efficiently exchanged between the systems.
Operation
Determine the purpose and functions of the digital twin. Will it perform asset monitoring, control and asset alteration, perform advanced analytics for predictive maintenance or
perform simulations? The answer to this question will identify the digital twin’s scope and functionality and help determine the type of devices required to attach to the asset.
Augmentation
The implementation of digital twins usually starts small such as monitoring a component of an asset but expands over time. The expansion is carried out in two ways:
- Organizations bring numerous more petite digital twins to provide a complete picture of the entire asset.
- Organizations add more sophisticated capabilities like simulations into the existing digital twin.
In either case, organizations want to layer up functionality within digital twin to meet the evolving requirements. The need is to securely add those functionalities to scale the digital twin while maintaining performance.
A five-level digital twin taxonomy
The proposed five-level taxonomy accounts for various manifestations of digital twins. Different kinds of analysis are associated with each classification level. The description of the fundamental data model is done at five levels:
Level 1: The digital representation, when augmented with physical simulation insights from CAE (Computer-Aided Engineering) or controlled lab experiments, leads to level 2 digital twin.
Level 2: Level 2 digital twin is obtained from CAE-based predictions, controlled data-based lab predictions and time-series analysis.
Level 3: The digital representation that couples sensor data with a data model is level 3 digital twin.
Level 4: Level 4 digital twin is the one that uses design insights, asset physics, sensor data and human knowledge encoded into the digital twin.
Level 5: It integrates level 4 digital twin with enterprise systems such as Enterprise Resource Planning (ERP), Manufacturing Execution System (MES) and Product Lifecycle Management (PLM). It is the most sophisticated digital twin because it leverages information from multiple enterprise sources to provide a comprehensive view of all aspects of the asset.
Analysis
Data at every level allows engineers to perform analysis corresponding to each digital twin representation.
Levels 1 and 2, comprising CAD, CAM and CAE, are already being used by design and engineering teams at major Original Equipment Manufacturers (OEMs).
At level 3, the sensor data entails anomaly detection, regression and other data-driven studies. The studies are mainly statistical, with seldom inputs from the design of the asset.
Level 4 representation enables engineers to perform various insightful analyses as this representation is augmented with physics-based models and knowledge from expert systems. It is beneficial in failure analysis and decision trees to track down issues with assets. For instance, a pump service engineer may have observed that leveling the pump or tightening a few bolts addresses overheating issues over time. Such human knowledge is valuable to encode in a digital twin. This representation helps perform system-level performance simulations, system-level optimization and analyzing what-if scenarios.
The ideal target state of a complete digital twin is at level 5. It is used to understand the impact of asset behavior and performance on supply chain and procurement.
Visualization techniques
Information obtained from each digital twin representation is visualized in various ways. It is important to note that the essence of visualization techniques is understanding the data and generating actionable insights. Multiple visualization techniques are implemented to glean insights from the gathered data using modalities such as 1d, 2d or 3d plots, scatter plots, heat maps, histograms and animations.
Business outcomes
A manufacturer or an asset operator manages business outcomes associated with each representation.
Digital twins at level 1 are used to design assets that can easily be assembled, manufactured and serviced.
Level 2 digital twins predict asset performance and failures in the field.
At level 3, digital twins receive sensor data and other parameters from the field, which provide condition monitoring, planned maintenance and real-time asset health as the potential outcomes.
Business outcomes for levels 4 and 5 are similar; the only difference is that level 5 provides an improved ability over level 4 results because of additional knowledge sources.
The asset operator and the manufacturer can:
- enhance predictive and prescriptive maintenance
- design product leveraging data from the field
- using design insights perform system optimization
- create asset-as-a-service offering
- provide real-time insights for improved decision making
What are the applications of digital twins?
The application of digital twins has brought a breakthrough in multiple industries:
Manufacturing
In the manufacturing industry, digital twins are used for facilitating product development, design customization, shop floor performance improvement and predictive maintenance.
- Product development: Engineers gain benefits from digital twins as it helps to test the feasibility of upcoming products before launching.
- Design customization: Digital twins help businesses design multiple permutations of the product and offer personalized products and services to their clients.
- Shop floor performance improvement: Digital twins are helpful in monitoring and analyzing the end products. It helps engineers to spot the defective and low-performing products in the lot.
- Predictive maintenance: Digital twins help manufacturers predict potential downtimes of machines to improve their overall productivity by minimizing non-value-adding maintenance activities.
Retail
In the retail sector, digital twins are used for modeling and augment customer experience at prominent shopping centers and individual stores.
Automotive
Digital twins are highly used for creating virtual models of connected vehicles. Automotive companies simulate and analyze the production phase to identify the potential problems during production or when the car hits the roads.
Healthcare
Digital twins virtualize healthcare services and help healthcare providers to optimize patient care, cost and performance. It aims to improve the operational efficiency of healthcare processes and enhance personalized care.
Smart cities
The digital twin can help cities to become more economically, socially and environmentally sustainable.
Conclusion
Digital twin applied across any industry makes a positive impact on product quality and accelerates service delivery speed. It implements real-time simulation and helps businesses leverage the advantage of predictive maintenance.
Product and business owners can realize the benefits of creating the virtual replica of their assets. A digital twin reduces cost and tends to increase market offers of high-quality products that benefit customers.
If you are looking for an IoT development company to develop a digital twin for your business, we are here to help you. Schedule a consultation with our technical experts and discuss your requirements.
Start a conversation by filling the form
All information will be kept confidential.
Insights
Generative AI for Regulatory Compliance: Benefits, integration approaches, use cases, best practices, and future trends
Generative AI is reshaping the field of regulatory compliance by enhancing risk management, boosting operational efficiency, and improving compliance monitoring.
Generative AI for marketing: Overview, use cases, integration strategies, and future outlook
Generative AI is transforming the marketing landscape by enhancing content creation, customer interaction, and data analysis.
Generative AI in due diligence: Integration approaches, use cases, challenges and future outlook
Generative AI is reshaping the due diligence landscape, establishing new data analysis and processing benchmarks.