Decision Transformer Model: Architecture, Use Cases, Applications and Advancements
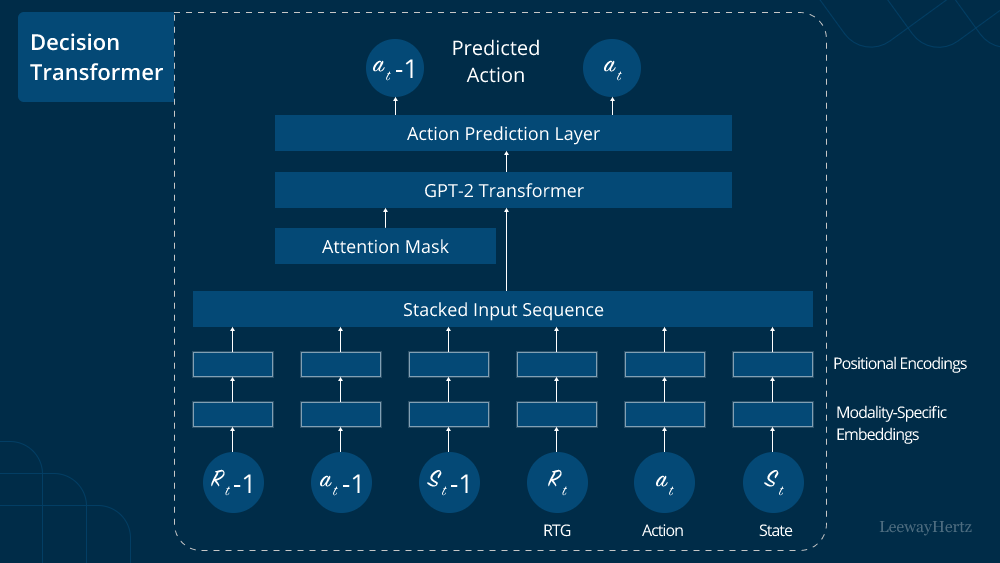
The realm of Reinforcement Learning (RL) has experienced a surge of innovations in recent years, propelling artificial intelligence towards its maximum potential. One such advancement is the Decision Transformer, a model that melds the power of Transformer architectures with the adaptability of reinforcement learning. The emergence of Decision Transformers marks a crucial milestone in the evolution of machine learning, showcasing their remarkable potential in transforming how RL-based agents interact with their environments.
Decision Transformers harness the strengths of Transformer models, known for their prowess in handling sequential data such as natural language processing, and couple them with the dynamic learning capabilities of reinforcement learning. This convergence allows for a more efficient and effective way to train intelligent agents, overcoming traditional RL methods’ limitations.
By leveraging the power of transformers, Decision Transformers enable offline reinforcement learning, reducing the need for resource-intensive online training, enabling agents to learn from existing datasets. This accelerates learning and mitigates risks associated with training agents in real-world environments or flawed simulators.
Furthermore, Decision Transformers address long-term dependencies, a persistent challenge in RL, by handling complex sequential data and generating future action sequences to optimize reward outcomes. This innovative approach has far-reaching implications for a wide range of applications, from robotics and autonomous vehicles to strategic gameplay and personalized user experiences.
By embracing the synergy between Transformer architectures and reinforcement learning, Decision Transformers pave the way for a new era of AI-powered solutions and advancements.
This article delves into the evolution of the Transformer model and highlights the challenges in reinforcement learning. It introduces offline reinforcement learning and explains sequence modeling via RL. The Decision Transformer model is presented with a detailed overview of its architecture and its key components. The article delves into the workings of the Decision Transformer, exploring its functionality, diverse applications, and recent advancements. It also provides practical guidance on how to use a Decision Transformer in a Transformer model and offers tips on effective training. This comprehensive guide is invaluable for anyone keen to understand and leverage this cutting-edge technology.
- What is a transformer model?
- Evolution of Transformer-based models
- What is decision transformer modeling?
- What is offline reinforcement learning?
- What is reinforcement learning via sequence modeling?
- The key components of a Decision Transformer
- The architecture of the Decision Transformer model
- How does the Decision Transformer work?
- Applications and use cases of the Decision Transformer
- The role of transformer in Decision Transformer: Essential or optional for continuous control tasks?
- How to use the Decision Transformer in a Transformer?
- How to train a Decision Transformer model?
- Advancements in Decision Transformers
What is a transformer model?
The transformer model, introduced in 2017, is a prominent artificial neural network renowned for its aptitude in processing sequential data, particularly text. Transformer models excel in tasks like text classification and machine translation. The transformer architecture enables machine learning models to analyze text bi-directionally, extracting insights from various parts of a sentence—both preceding and following a word’s occurrence. Self-attention mechanisms empower the model to spotlight pertinent segments within the input sequence, capturing connections between different words and phrases across the entire context. Consequently, the model comprehends word context and significance by considering the comprehensive semantic and syntactic framework of the text, surpassing isolated words or phrases evaluation.
Evolution of Transformer-based models
This section will briefly discuss the original Transformer architecture and four significant Transformer architectures that emerged shortly after its introduction.
Before diving in, let’s understand the kind of tasks various models perform.
- Encoder-only models: Suitable for tasks requiring input comprehension, such as sentiment analysis.
- Decoder-only models: Designed for tasks that involve text generation, like story-writing.
- Encoder-decoder models or sequence-to-sequence models: Used for tasks that require generating text from input, like summarization.
Transformer by Google
In 2017, Google researchers introduced a network architecture called Transformer in their paper “Attention is all you need.” Designed primarily for natural language processing tasks, Transformers changed the field by addressing the limitations of Recurrent Neural Networks (RNNs).
Unlike RNNs, which handle one word at a time, Transformers can process complete sentences at once. This makes Transformers more efficient and better at understanding context. The attention mechanism helps Transformers access past information more accurately than RNNs, which have difficulty keeping context beyond the previous state. Transformers also use positional embeddings to track word positions within sentences. This advantage has led Transformers to outperform RNNs in almost all language tasks. Undoubtedly, learning about Transformers’ innovative design and incredible growth in recent years is essential.
This groundbreaking architecture led to the emergence of four significant Transformer-based models: OpenAI’s GPT, Google’s BERT, OpenAI’s GPT-2, and Facebook’s RoBERTa.
GPT by OpenAI
The evolution of GPT in recent years is nothing short of remarkable, making it an excellent starting point for discussing Transformers. GPT, short for Generative Pre-training, pioneered the concept of unsupervised learning for pre-training, followed by supervised learning for fine-tuning—a method now widely adopted by many Transformers.
GPT was initially trained on a unique dataset comprising 7,000 unpublished books, showcasing its prowess in understanding complex language patterns. Its architecture, composed of 12 stacked decoders, distinguishes it as a decoder-only model. Despite having 117 million parameters, GPT-1’s capacity pales compared to the more advanced Transformers developed today. GPT’s unidirectional nature, which involves masking tokens to the right of the current token, allows it to generate one token at a time and use that output for the subsequent timestep.
GPT marked the dawn of a new era for Transformers, paving the way for even more astonishing innovations that would soon follow.
The evolution of GPT models is a testament to the incredible advancements in natural language processing. Spanning several iterations, GPT models have consistently pushed the boundaries of what AI language models can achieve.
- GPT (GPT-1): The first iteration, GPT, or Generative Pre-trained Transformer, introduced the concept of unsupervised learning for pre-training and supervised fine-tuning. It utilized 12 decoders in its architecture and was trained on a dataset of 7,000 unpublished books. With 117 million parameters, GPT-1 was a groundbreaking model for its time, though its successors have since eclipsed it.
- GPT-2: Building upon the foundation of GPT-1, GPT-2 featured an impressive 1.5 billion parameters, significantly increasing the model’s capabilities. It utilized 48 decoders in its architecture and was trained on a much larger dataset of 8 million web pages. Despite concerns about potential misuse, GPT-2 demonstrated an extraordinary ability to generate coherent and contextually relevant text.
- GPT-3: The latest powerful iteration, GPT-3, boasts 175 billion parameters. This model has shown remarkable performance across a wide range of tasks, including translation, summarization, and even programming assistance. Its advanced architecture and unparalleled capacity for contextual understanding have positioned GPT-3 as a leader in the field of natural language processing.
- GPT-4: It is the most recent breakthrough in OpenAI’s continuous endeavor to scale up deep learning. As a multimodal model, GPT-4 is designed to accept both image and text inputs while generating text outputs. This innovative approach allows the model to demonstrate human-level performance across numerous professional and academic benchmarks. GPT-4 builds on the success of its predecessors, incorporating the advancements made in earlier iterations while pushing the boundaries of AI capabilities. Its ability to process and understand both visual and textual data enables GPT-4 to tackle a wider range of tasks and adapt to diverse real-world applications more effectively. While GPT-4 may not yet surpass human performance in every scenario, it represents a significant step forward in the field of artificial intelligence. GPT-4 demonstrates its potential to revolutionize industries and reshape our understanding of AI’s role in modern society by excelling in various benchmarks. With the release of GPT-4, OpenAI reaffirms its commitment to advancing deep learning and creating models that improve upon existing technology and pave the way for future breakthroughs. As the AI community continues to explore the possibilities offered by GPT-4, we can expect further innovations and exciting developments in the realm of artificial intelligence.
Google’s BERT
BERT, the Bidirectional Encoder Representation from Transformers, is a game-changing model that redefines how we approach natural language processing. BERT’s bidirectional nature sets it apart from its counterparts, as its attention mechanism can effectively capture the context from both the left and right directions of the current token. With a powerful architecture comprising 12 stacked encoders, BERT serves as an encoder-only model that can process entire sentences as input, allowing it to reference any word in the sentence for unparalleled performance.
Boasting 110 million parameters, BERT shares the flexibility of GPT, as it can be trained on specific tasks and fine-tuned for a variety of applications. Its unique pre-training method, which essentially involves a fill-in-the-blank task, demonstrates the exceptional capabilities of this highly efficient model.
Launch your project with LeewayHertz
Unlock the full potential of AI with our Decision Transformer model-powered solutions
Facebook’s RoBERTa
Facebook’s RoBERTa, Robustly Optimized BERT Pre-training Approach, takes the power of BERT several notches higher. While RoBERTa shares the same architecture as BERT, the significant optimization it has undergone sets it apart. These enhancements have led to state-of-the-art results on various NLP benchmarks, including MNLI, QNLI, RTE, STS-B, GLUE, and RACE tasks.
By eliminating BERT’s next-sentence pretraining objective, training with larger mini-batches and altering the masking pattern, RoBERTa has surpassed its predecessor in performance. Furthermore, RoBERTa has been trained on a more extensive dataset, incorporating existing unannotated NLP datasets and CC-News, a novel collection of public news articles.
The rapid evolution of Transformer-based models has significantly impacted the field of natural language processing. Researchers have created new models that perform specific NLP tasks more effectively than the original Transformer by separating and stacking encoder and decoder architectures. The widespread adoption of unsupervised learning for pre-training and supervised learning for fine-tuning has furthered the development of these models.
What is decision transformer modeling?
The Decision Transformer model, introduced by Chen L. et al. in “Decision Transformer: Reinforcement Learning via Sequence Modeling,” transforms the reinforcement learning (RL) landscape by treating RL as a conditional sequence modeling problem. Instead of relying on traditional RL methods, like fitting a value function to guide action selection and maximize returns, the Decision Transformer utilizes a sequence modeling algorithm (i.e., the Transformer) to generate future actions that achieve a specified desired return. In simple terms, decision transformers represent a specialized kind of transformer model crafted for tasks involving step-by-step decision-making. These models excel at taking in sequences of information and producing sequences of actions, which empowers them to make well-informed decisions in a structured and sequential manner.
This innovative approach hinges on an autoregressive model conditioned on the desired return, past states, and actions. By using generative trajectory modeling, that predicts the combined patterns of situations, actions, and rewards, the method simplifies and makes it easier for people to understand the reinforcement learning process, the Decision Transformer sidesteps the conventional RL process of return maximization and directly generates a series of future actions that fulfill the desired return.
The process is as follows:
- The Decision Transformer takes information from the most recent events (the last K timesteps) and uses three pieces of data for each event: the expected future rewards (return-to-go), the current situation (state), and the action taken. Using this information, the model can learn to generate actions that help achieve a desired outcome.
- Tokens are embedded using a linear layer for vector states or a CNN encoder for frame-based states. This means that the information (tokens) about the current situation is transformed into a more useful format for the model. If the information is a simple list of numbers (vector state), a basic method (linear layer) is used. If the information is a series of images (frame-based states), a more complex method (CNN encoder) designed for image processing is utilized.
- These inputs are then processed by a GPT-2 model, which predicts future actions through autoregressive modeling, employing a causal self-attention mask.
The Decision Transformer model paves the way for a new era of advanced and efficient AI systems by reimagining the RL paradigm and leveraging the power of Transformers. This groundbreaking approach can potentially reshape reinforcement learning and unlock exciting new applications across diverse domains.
What is offline reinforcement learning?
Offline reinforcement learning, also known as batch reinforcement learning or data-driven reinforcement learning, is a method of training decision-making agents without direct interaction with the environment. Instead, agents learn from pre-collected data, which can originate from other agents or human demonstrations. This approach offers an alternative to online reinforcement learning, where agents gather data and learn from it through direct interaction with the environment or a simulator.
Offline reinforcement learning involves the following process:
- Create a dataset using one or more policies and/or human interactions.
- Run offline RL on this dataset to learn a policy.
This method is particularly beneficial when building a complex, expensive, or insecure simulator. However, offline reinforcement learning has a major drawback known as the counterfactual queries problem. This issue arises when an agent decides to perform an action for which no data is available in the pre-collected dataset. For example, suppose the agent wants to turn right at an intersection but the dataset does not contain information about this trajectory. In that case, the agent may struggle to make an informed decision.
Despite this limitation, offline reinforcement learning offers a valuable alternative for training deep reinforcement learning agents, especially in situations where real-world interactions or simulator construction is challenging or impractical.
What is reinforcement learning via sequence modeling?
Reinforcement learning via sequence modeling is a method that combines reinforcement learning with sequence modeling techniques to address problems where the agent’s decision-making process involves a sequence of actions or states with temporal dependencies. This approach is necessary when the agent needs to learn optimal strategies in complex environments where the relationships between actions and states evolve over time.
Sequence modeling is a technique in machine learning that focuses on analyzing and predicting sequences of data points. It deals with problems where the order and relationships between data points are significant, such as time series forecasting, natural language processing, and protein sequence analysis. Common algorithms used in sequence modeling include recurrent neural networks (RNNs), long short-term memory (LSTM) networks, and Transformers, which can capture complex patterns and dependencies within the data sequences. By understanding these patterns, sequence modeling techniques can generate predictions or generate entirely new sequences based on the learned context. These techniques allow the agent to learn from the history of states, actions, and rewards, which in turn helps it generate an optimal sequence of actions that maximize the cumulative reward over time.
The necessity of reinforcement learning via sequence modeling arises in various applications, including:
- Natural language understanding: In tasks like dialogue systems or machine translation, agents must generate appropriate responses based on the context provided by the input text, which often requires understanding the relationships between words and phrases over time.
- Time-series prediction: When predicting future values in time-series data, such as stock prices or weather patterns, it is crucial to learn the data’s temporal dependencies and underlying patterns, which can be addressed using sequence modeling techniques combined with reinforcement learning.
- Robot control: In robotics, agents often need to learn the optimal sequence of actions to complete specific tasks, such as object manipulation or navigation, based on the history of states and rewards. Reinforcement learning via sequence modeling provides a suitable framework to tackle such problems.
Launch your project with LeewayHertz
Unlock the full potential of AI with our Decision Transformer model-powered solutions
The key components of a Decision Transformer
The key components of a Decision Transformer include:
- Transformer architecture: The Decision Transformer utilizes the Transformer architecture, which is known for effectively processing sequential data. Transformers consist of multi-headed self-attention mechanisms and feed-forward neural networks, which enable the model to capture complex dependencies within the input data.
- State-Action-Reward sequences: The Decision Transformer learns from a dataset of state-action-reward (SAR) sequences, where each sequence represents the agent’s interaction with the environment. These sequences are used to train the Transformer in an offline reinforcement learning setting.
- Reward modeling: The Decision Transformer uses the reward information embedded within the SAR sequences to predict the optimal actions that lead to the highest cumulative rewards. This reward-driven approach ensures that the generated action sequences align with the agent’s goal of maximizing its rewards in the environment.
- Context conditioning: To predict the optimal action, the Decision Transformer conditions its predictions on the current state and the history of past states, actions, and rewards. This context conditioning allows the model to learn the relationships between past experiences and future actions, thus guiding the agent towards better decisions.
- Offline Reinforcement Learning: The Decision Transformer operates in an offline reinforcement learning setting, where the agent learns from a pre-collected dataset of SAR sequences without actively exploring the environment. This approach reduces computational requirements and enables learning from diverse sources, such as human demonstrations or other agents’ experiences.
- Sequence generation: Leveraging the Transformer’s ability to generate future sequences, the Decision Transformer predicts the sequence of actions that will maximize the agent’s cumulative reward. This sequence generation capability is key to the model’s success in reinforcement learning tasks.
Combining these components, the Decision Transformer creates a powerful model bridging the gap between Transformers and reinforcement learning, enabling more efficient and versatile learning in various domains.
The architecture of the Decision Transformer model
The architecture of a Decision Transformer is based on the original Transformer architecture, with modifications to adapt it for reinforcement learning tasks. The key components of the Decision Transformer architecture are:
- Input representation: The input for the Decision Transformer consists of state-action-reward (SAR) tuples from the agent’s interaction with the environment. These tuples are represented as a sequence of tokens, which are then embedded into continuous vectors using an embedding layer.
- Positional encoding: Positional encodings are added to the embedded input vectors to capture the relative position of the input tokens. This allows the model to recognize the order of the input tokens and understand the temporal relationships between them.
- Multi-head self-attention: The core of the Transformer architecture is the multi-head self-attention mechanism. This component computes attention scores for each input token, capturing the dependencies between different tokens in the input sequence. The self-attention mechanism is applied multiple times in parallel (multi-head) to learn different aspects of the input data.
- Feed-forward neural network: A feed-forward neural network is applied to each position independently after the multi-head self-attention layer. This component consists of two linear layers with an activation function (usually ReLU) in between, which helps the model learn more complex patterns within the input data.
- Residual connections and layer normalization: In each layer of the Transformer, residual connections combine the outputs of the self-attention and feed-forward layers with their inputs. Layer normalization is then applied to stabilize the training process and improve the model’s generalization capability.
- Stacking layers: The Decision Transformer’s architecture comprises multiple layers of self-attention and feed-forward components stacked on top of each other. This deep structure enables the model to learn complex hierarchical relationships within the input data.
- Output layer: The final layer of the Decision Transformer is a linear layer that maps the continuous output vectors back to the action space, generating the predicted action sequence.
- Training objective: The Decision Transformer is trained to predict the action sequence that maximizes the agent’s cumulative reward. This is achieved by minimizing the cross-entropy loss between the predicted and ground truth action sequences derived from the input SAR tuples.
In the Decision Transformer architecture, reinforcement learning is integrated implicitly through the way the input data is represented and the training objective is defined. The reinforcement learning aspects can be found in the following components:
- Input representation: The input consists of state-action-reward (SAR) tuples collected from the agent’s interaction with the environment. This data directly links the architecture to the reinforcement learning problem, as it captures the agent’s experience and the corresponding rewards.
- Training objective: The training objective in the Decision Transformer is designed to predict the action sequence that maximizes the agent’s cumulative reward. By minimizing the cross-entropy loss between the predicted action sequence and the ground truth action sequence, the model learns to make decisions that result in higher rewards.
While the architecture itself is built on the Transformer structure, the reinforcement learning aspects are incorporated through the input representation and training objective. The Decision Transformer does not explicitly include traditional reinforcement learning components such as value functions or policy gradients. Instead, it leverages the power of the Transformer architecture to learn an effective policy for the given task by predicting future action sequences that maximize cumulative rewards.
How does the Decision Transformer work?
The Decision Transformer model works by leveraging the Transformer architecture to learn a policy for reinforcement learning tasks from collected trajectory data. Here’s a detailed explanation of its learning process:
Learning from collected trajectory data
The Decision Transformer uses offline reinforcement learning, meaning it works by learning from previously collected data, which includes sequences of states, actions, and rewards (called trajectories). These trajectories come from past experiences of other agents or even human demonstrations.
To understand how the Decision Transformer works, imagine you have a dataset of these trajectories representing different experiences and their corresponding rewards. The model takes in the current situation and information about past experiences and tries to predict the best sequence of actions that would lead to the highest total reward.
In simpler terms, the Decision Transformer looks at what has been done before in similar situations and uses this knowledge to decide what actions to take in the current situation to achieve the best outcome.
Handling long-term dependencies
One of the key challenges in reinforcement learning is handling long-term dependencies, where the agent must make decisions based on information that may not be immediately available or only becomes relevant after a series of steps. Transformers, by design, are well-suited to address this issue due to their ability to capture contextual information and dependencies across long sequences.
The Decision Transformer benefits from the self-attention mechanism in Transformers, which allows the model to focus selectively on different parts of the input sequence and learn long-range dependencies between the states, actions, and rewards. As a result, the model can capture the relationships between past experiences and future decisions, effectively learning a policy that accounts for both immediate and long-term rewards.
By combining the powerful representation learning capabilities of Transformers with trajectory data from reinforcement learning tasks, the Decision Transformer is able to learn effective policies that consider both short-term and long-term dependencies. This results in an agent capable of making decisions that maximize the cumulative reward over time, addressing one of the major challenges in reinforcement learning.
The role of transformer in Decision Transformer: Essential or optional for continuous control tasks?
Decision Transformer (DT) is a novel architecture for Reinforcement Learning (RL) that redefines decision-making as an auto-regressive sequence modeling problem, using a transformer model to predict the next action in a sequence of states, actions, and rewards. This innovative approach takes inspiration from the successes of Transformers in Natural Language Processing (NLP) and Computer Vision, aiming to leverage their sequential modeling capabilities for RL tasks.
Transformers have demonstrated impressive results in various domains, primarily due to their ability to model sequences effectively. Unlike Recurrent Neural Networks (RNNs) and Long Short-Term Memory (LSTM) networks, Transformers do not rely on sequential processing of input elements. Instead, they use attention mechanisms, which allow for parallelization and handling of long-range dependencies in the data. This parallelization capability is particularly advantageous for tasks with short input sequences but high-dimensional latent representations, which are common in NLP.
In the context of a Decision Transformer, the core idea is to predict the next action in a trajectory of states, actions, and rewards. The DT architecture employs the GPT model, a type of transformer, to auto-regressively model these trajectories. The GPT architecture’s ability to condition outputs on past sequences without requiring recurrent processing makes it a strong candidate for this task. However, an intriguing question arises: Is the transformer an indispensable component of the Decision Transformer architecture, particularly for continuous control tasks?
Continuous control tasks, such as pendulum swing-up and stabilization, present unique challenges for RL algorithms. These tasks require fine-grained stabilization and real-time control, which are critical for applications in robotics and dynamic system management. The Decision Transformer aims to address these challenges by framing the RL problem as a sequence modeling task, predicting the next action based on the sequence of past states, actions, and rewards.
To evaluate the transformer’s importance in DT, a comparative analysis is conducted using an alternative architecture, Decision LSTM (DLSTM). This model retains the overall framework of DT but replaces the Transformer with an LSTM network. LSTMs, known for their ability to handle sequences of varying lengths and maintain information over time, provide a useful comparison to understand the transformer’s role in DT.
The experimental results reveal interesting insights. While Decision Transformers perform well in various RL tasks, they encounter difficulties in continuous control problems, such as inverted pendulum and Furuta pendulum stabilization. In contrast, the Decision LSTM model demonstrates superior performance in these tasks, achieving expert-level control and successfully learning swing-up controllers on real systems. These findings suggest that the strength of the Decision Transformer may lie more in the sequence modeling approach rather than the specific use of the Transformer architecture.
The comparison highlights the computational differences between Transformers and LSTMs. Transformers scale linearly with input dimensionality and quadratically with input length, making them efficient for short sequences with high-dimensional inputs. However, for long sequences, this can become computationally intensive. LSTMs, on the other hand, scale linearly with input length and quadratically with input dimensionality, offering computational advantages for long sequences with smaller input dimensions. This makes LSTMs potentially more suitable for certain RL tasks where input sequences are lengthy.
In summary, the crucial aspect of the Decision Transformer appears to be the framing of RL as a sequence modeling problem. While the Transformer brings certain advantages, particularly in parallelization and handling high-dimensional inputs, the LSTM network’s performance in the DLSTM variant indicates that other sequence modeling architectures can also excel in this framework. Therefore, the overall sequence modeling approach is paramount, with the choice of specific architectures like Transformers or LSTMs depending on the particular requirements and constraints of the task at hand.
Applications and use cases of the Decision Transformer
The Decision Transformer has a wide range of applications and use cases due to its ability to learn from trajectory data and handle long-term dependencies. Some possible applications and use cases include:
- Robotics: Decision Transformers can be used to train robotic agents to perform complex tasks, such as navigation, manipulation, and interaction with their environment, by learning from collected demonstrations or past experiences.
- Autonomous vehicles: In the context of self-driving cars, Decision Transformers can learn optimal decision-making policies from collected driving data, enabling safer and more efficient navigation in various traffic conditions.
- Games: Decision Transformers can be employed to train agents that excel in strategic games, like Go, chess, or poker, by learning from a dataset of expert gameplays and mastering long-term planning.
- Finance: Decision Transformers can be applied to optimize portfolio management, and risk assessment by learning from historical financial data and taking into account long-term dependencies.
- Healthcare: In medical decision-making, Decision Transformers can be used to optimize treatment plans, diagnose diseases, or predict patient outcomes by learning from historical patient data and understanding the long-term effects of different interventions.
- Natural Language Processing (NLP): Although not a direct application of reinforcement learning, Decision Transformers can be adapted to solve NLP tasks that involve making decisions or generating responses based on long-term dependencies in text data, such as machine translation, text summarization, or dialogue systems.
- Supply chain optimization: Decision Transformers can be used to optimize inventory management, demand forecasting, and routing decisions in complex supply chain networks by learning from historical data and considering long-term effects.
- Energy management: Decision Transformers can help optimize energy consumption, demand response, and grid stability in smart grid applications by learning from historical usage data and considering long-term dependencies.
How to use the Decision Transformer in a Transformer?
Scenario: Here, we are demonstrating a Decision Transformer model, created by Huggingface. This model is trained to become an ‘expert’ using offline reinforcement learning. It’s specifically designed to operate effectively in the Gym Walker2d environment.This model learns how to make optimal decisions for a two-legged walking agent without directly interacting with the environment, using past experiences and data collected from other sources. The model then applies its knowledge to help the agent navigate and perform tasks effectively in the Gym Walker2d setting.
Step 1. Installation and setup
First, ensure that you have the necessary library installed. The Decision Transformer is available through the Hugging Face Transformers library. Installation typically involves using a package manager like pip to install the library from its repository.
Step 2: Model initialization
Utilizing the Decision Transformer is relatively simple; however, its autoregressive nature requires careful organization of the model’s inputs at each time step.
To use the Decision Transformer, you need to load a pre-trained model. These models are trained on specific environments and can be fine-tuned for various tasks. Initialization involves specifying the model name and loading it into your environment. This pre-trained model has learned how to make optimal decisions based on historical data from a reinforcement learning environment.
Step 3: Environment configuration
The Decision Transformer operates within a defined environment, such as a simulated environment provided by Gym. Gym is an open-source Python library that standardizes the development and comparison of reinforcement learning algorithms by offering a consistent API for communication between learning algorithms and environments, along with a diverse set of compliant environments, like Hopper-v3 and Walker2D, for model training. This environment includes:
- State dimension: Represents the size of the state space (e.g., observations).
- Action dimension: Represents the size of the action space (e.g., possible actions the agent can take).
Understanding these dimensions helps configure the model to interact effectively with the environment.
Step 4: Autoregressive prediction mechanism
The Decision Transformer operates autoregressively, meaning it predicts future actions based on past data. At each time step, it takes as input a sequence of past states, actions, rewards, and returns-to-go. The model processes this historical data (up to a certain number of previous time steps) to generate predictions for the next action.
- Input preparation: Historical data (states, actions, rewards, returns-to-go, and timesteps) is organized and processed. This data is used to condition the model’s predictions.
- Prediction process: The model predicts actions for the current timestep based on the provided historical context. It does so by conditioning its output on the sequence of past observations and actions, which helps it make informed decisions.
Step 5: Model evaluation
Evaluating the Decision Transformer involves:
- Normalization: States are normalized using predefined mean and standard deviation values obtained from training data.
- Target return: A target return value is set to guide the model’s performance. This value reflects the desired outcome or goal for the agent’s performance in the environment.
- Interaction: The model interacts with the environment by selecting actions based on its predictions and adjusting its predictions based on the received rewards and updated states.
The evaluation process involves running the model within the environment, collecting feedback, and iteratively updating the model’s predictions. This helps assess the model’s effectiveness in achieving the target return and making optimal decisions.
How to train a Decision Transformer model?
Training a Decision Transformer model involves several crucial steps, beginning with preparing the dataset and culminating in model evaluation and fine-tuning. The process leverages Transformer architectures to learn from offline reinforcement learning datasets, aiming to generate sequences of actions that maximize rewards. Here’s a detailed guide on how to train a Decision Transformer model:
Step 1: Dataset preparation
To train a Decision Transformer model, you first need an offline reinforcement learning (RL) dataset, which consists of data collected from other agents or human demonstrations. This dataset includes sequences of states, actions, and rewards.
- Normalize features: Subtract the mean and divide by the standard deviation for each feature.
- Pre-compute discounted returns: Calculate the cumulative reward for each trajectory.
- Scale rewards and returns: Apply a scaling factor to the rewards and returns.
Step 2: Data collation
A custom data collator is essential for preprocessing the dataset into batches suitable for training. This involves:
- Sampling trajectories: Adjust the sampling distribution based on trajectory lengths to ensure diverse training data.
- Creating batches: Form batches containing states, actions, rewards, returns, timesteps, and attention masks.
- Normalization and padding: Normalize states and pad sequences to ensure uniform batch sizes.
Step 3: Model training
Training the Decision Transformer involves the following key steps:
- Model configuration: Define the Transformer architecture, specifying input dimensions for states and actions.
- Loss function: Use the L-2 norm of the difference between predicted actions and target actions as the loss function.
- Training loop: Employ the Hugging Face Trainer class to handle the training loop, optimization, and loss computation.
- Hyperparameters: Set hyperparameters like learning rate, batch size, number of epochs, and optimization algorithm.
Step 4: Evaluation and fine-tuning
After initial training, evaluate the model’s performance on validation data and fine-tune hyperparameters or model architecture as needed to improve results.
By following these steps, you can effectively train a Decision Transformer model to generate sequences of actions that achieve desired outcomes, leveraging the strengths of Transformer architectures in the context of reinforcement learning.
Advancements in Decision Transformers
As reinforcement learning (RL) continues to evolve, new architectures are emerging to address specific challenges and enhance performance. Decision Transformers, which initially applied sequence modeling principles to RL, have seen significant advancements. This section explores several notable developments in Decision Transformers, including the Hierarchical Decision Transformer (HDT), Elastic Decision Transformer (EDT), Q-Learning Decision Transformer (QDT), and Online Decision Transformer (ODT). Each of these innovations builds on the foundational ideas of Decision Transformers, offering improvements for various RL scenarios.
Hierarchical Decision Transformer: Transforming sequence learning with hierarchical models
In reinforcement learning (RL), the Hierarchical Decision Transformer (HDT) marks a significant advancement, particularly for tasks characterized by long episodes and sparse rewards. This innovative approach integrates hierarchical models with sequence learning, providing a robust solution to the challenges faced by traditional RL methods.
What is the hierarchical Decision Transformer?
The Hierarchical Decision Transformer (HDT) builds upon the decision transformer architecture by introducing a hierarchical approach to sequence learning. It employs two levels of decision-making—high-level and low-level—to guide the learning process. This dual-level architecture enhances performance, especially for complex tasks with extended time horizons and limited reward signals.
Core components of HDT
- High-level mechanism:
- Purpose: Sets sub-goals based on the sequence of states observed in demonstration data, predicting key sub-goal states that serve as intermediate milestones for the agent.
- Function: Guides the low-level controller through the task by defining these sub-goals, breaking down complex problems into manageable steps, and addressing the challenge of sparse rewards by focusing on valuable states that contribute significantly to the trajectory’s success.
- Low-level controller:
- Purpose: Operates based on the sub-goals provided by the high-level mechanism, learning from the sequence of states, actions, and sub-goals to make decisions and take action.
- Function: Uses a decision transformer architecture to predict the next action in the sequence, conditioned on the history of states, actions, and sub-goals. This helps the agent navigate effectively, even when rewards are sparse.
Advantages of HDT
- Improved performance in sparse reward environments: HDT addresses the challenge of sparse rewards by replacing the sequence of returns-to-go with sub-goal sequences, enhancing the agent’s ability to learn from limited reward signals.
- Eliminates the need for reward specification: Unlike traditional decision transformers, HDT does not rely on specified rewards. It uses sub-goal states derived from demonstration data, simplifying the learning process and making it adaptable to various tasks.
- Enhanced learning efficiency: By structuring learning into high-level and low-level components, HDT effectively handles tasks with long episodes. The high-level mechanism breaks tasks into smaller, achievable sub-goals, improving overall efficiency and performance.
- Flexibility and adaptability: HDT is versatile and applicable to both short-horizon problems and complex, long-horizon challenges. Its adaptability to various environments and reward structures makes it a powerful tool for sequential decision-making.
Applications and validation
HDT’s effectiveness has been validated across multiple benchmark tasks, including OpenAI Gym, D4RL, and RoboMimic. Experimental results show that HDT outperforms traditional decision transformers and baseline methods, particularly in tasks with longer episodes and sparse rewards. For instance, in tasks such as Half-Cheetah and Hopper, HDT achieved higher accumulated rewards compared to both the original decision transformer and other baseline methods. By leveraging hierarchical models and focusing on sub-goals, HDT addresses critical limitations of traditional RL methods, paving the way for more effective learning strategies in complex environments.
Elastic Decision Transformer: Enhancing offline reinforcement learning
The Elastic Decision Transformer (EDT) is a pioneering advancement in offline reinforcement learning, building upon the Decision Transformer (DT) architecture. Here’s an overview of EDT:
Key features
- Trajectory stitching: EDT overcomes a limitation of DT by enabling the combination of segments from sub-optimal trajectories to create an optimal trajectory.
- Dynamic history length: Unlike DT, which uses a fixed history length, EDT adjusts the input history length dynamically based on the quality of the current trajectory.
- Maximum return estimation: EDT uses expectile regression to estimate the maximum achievable return for different history lengths.
Mechanism
- Action inference: EDT searches for the optimal history length that maximizes the estimated return during action inference.
- Variable-length input: Utilizes a variable-length input sequence for more effective decision-making.
- History retention: Maintains longer history when the trajectory is optimal and shorter history when it is sub-optimal.
Training and implementation
- Training procedure: EDT’s training is similar to DT but includes an additional objective to estimate the maximum return.
- Computational efficiency: It is computationally efficient and can be integrated with other DT variants.
Performance
- Datasets: EDT outperforms DT and its variants on most datasets, particularly “medium-replay” datasets.
- Multi-task learning: Shows strong performance in multi-task scenarios like locomotion tasks and Atari games.
- Single-task Scenarios: While it may not fully surpass some Q-learning methods, EDT bridges the gap between DT-based and Q-learning-based approaches.
Applications
EDT’s ability to perform trajectory stitching is especially valuable in scenarios where learning from sub-optimal data is necessary, such as robotics, autonomous systems, and other real-world applications where collecting optimal data is challenging or costly.
Significance
The Elastic Decision Transformer represents a significant advancement in offline reinforcement learning by addressing the trajectory stitching problem. It opens up new possibilities for learning effective policies from sub-optimal datasets, potentially expanding offline RL’s applicability to more complex and real-world scenarios.
Q-learning Decision Transformer: Combining dynamic programming and sequence modeling
The Q-Learning Decision Transformer (QDT) is a groundbreaking approach to offline reinforcement learning, merging the strengths of Q-learning and Decision Transformer. This hybrid algorithm aims to address the limitations of each approach while leveraging their advantages.
Key features of QDT
- Fusion of dynamic programming and sequence modeling:
- Combines Q-learning’s dynamic programming capabilities with the sequence modeling architecture of Decision Transformer.
- Improved stitching ability:
- Enhances the ability to construct optimal trajectories from suboptimal data, overcoming a major limitation of standard Decision Transformers.
- Robust performance:
- Demonstrates consistent performance across various environments, handling both sparse reward scenarios and complex control tasks effectively.
How does QDT work?
- Initial Q-learning phase:
- Trains a Conservative Q-Learning (CQL) model on the offline dataset.
- Data relabeling:
- Uses learned Q-values to relabel return-to-go (RTG) values in the training data, infusing dynamic programming information into the dataset.
- Decision Transformer training:
- Trains a Decision Transformer model on the relabeled dataset, learning to generate actions based on enhanced RTG values.
Advantages
- Versatility: Performs well in environments where either Q-learning or Decision Transformer alone might struggle.
- Long-horizon capability: Maintains the Decision Transformer’s ability to handle long time horizons.
- Improved learning from suboptimal data: Better leverages information from imperfect trajectories.
Experimental results
QDT has shown promising results in various environments, including:
- Simple grid world tasks
- Maze2D navigation challenges
- MuJoCo continuous control tasks with delayed rewards
In many cases, QDT outperformed both CQL and Decision Transformer independently, particularly in scenarios requiring a balance between stitching ability and long-term dependencies.
Online Decision Transformer: Advancing reinforcement learning through hybrid approaches
In the evolving field of reinforcement learning (RL), the Online Decision Transformer (ODT) represents a major breakthrough, combining offline pretraining with online finetuning to enhance policy optimization.
What is the Online Decision Transformer?
The Online Decision Transformer (ODT) builds on sequence modeling principles, originally applied in large-scale language models, to address RL challenges. It merges offline pretraining with online finetuning, offering an effective solution for scenarios where acquiring new data is costly and exploration needs to be efficient.
Key features of ODT
- Unified framework:
- Integrates offline pretraining with online finetuning, leveraging vast amounts of offline data while optimizing performance through online interactions.
- Sequence-level entropy regularization:
- Employs sequence-level entropy regularizers to balance exploration and exploitation, encouraging broader exploration and more diverse data generation.
- Autoregressive modeling objectives:
- Utilizes autoregressive modeling objectives similar to those in language modeling, predicting actions based on past sequences of states and rewards.
- Novel replay buffer:
- Uses a trajectory-level replay buffer to store complete trajectories, ensuring the replay buffer remains relevant and useful for ongoing learning.
- Stochastic policy optimization:
- Shifts from deterministic to stochastic policies, using a probabilistic framework to model action distributions and incorporating max-entropy RL concepts.
Empirical results
ODT performs competitively with state-of-the-art RL algorithms on benchmarks such as D4RL. It demonstrates significant improvements during the finetuning phase, showcasing its ability to adapt and optimize policies more effectively compared to other methods.
Applications and impact
ODT is particularly valuable in scenarios where offline data is abundant but online interactions are costly or limited. Its ability to blend offline learning with online adaptation makes it a powerful tool for real-world applications such as robotics, autonomous driving, and complex decision-making systems. ODT represents a significant advancement in RL, addressing key limitations of traditional approaches and offering a comprehensive solution for both offline and online learning. Its principles and techniques will likely influence future developments in RL and related areas.
Endnote
The Decision Transformer model transforms the way reinforcement learning agents are trained and interact with their environments. By merging the best of both worlds – the unparalleled ability of transformers to process sequential data and the dynamic learning capabilities of reinforcement learning – Decision Transformers unlock new possibilities for a range of applications and industries.
With their efficient offline learning process and proficiency in handling long-term dependencies, Decision Transformers address the limitations of traditional RL approaches and unlock new potential for creating advanced, intelligent systems. The applications for Decision Transformers are vast and far-reaching, from autonomous vehicles and robotics to strategic gameplay and personalized user experiences.
As we look to the future of AI, the Decision Transformer model stands as a testament to the power of innovation and interdisciplinary research. By embracing the synergy between Transformer architectures and reinforcement learning, we can unlock a new era of AI-powered solutions, enhancing our capabilities and improving our understanding of the world around us.
Leverage advanced Decision Transformer models to enhance AI-driven customer experiences. Contact LeewayHertz’s Transformer development services for your consultancy and development needs!
Start a conversation by filling the form
All information will be kept confidential.