Decision intelligence: Benefits, applications, implementation, and future trends
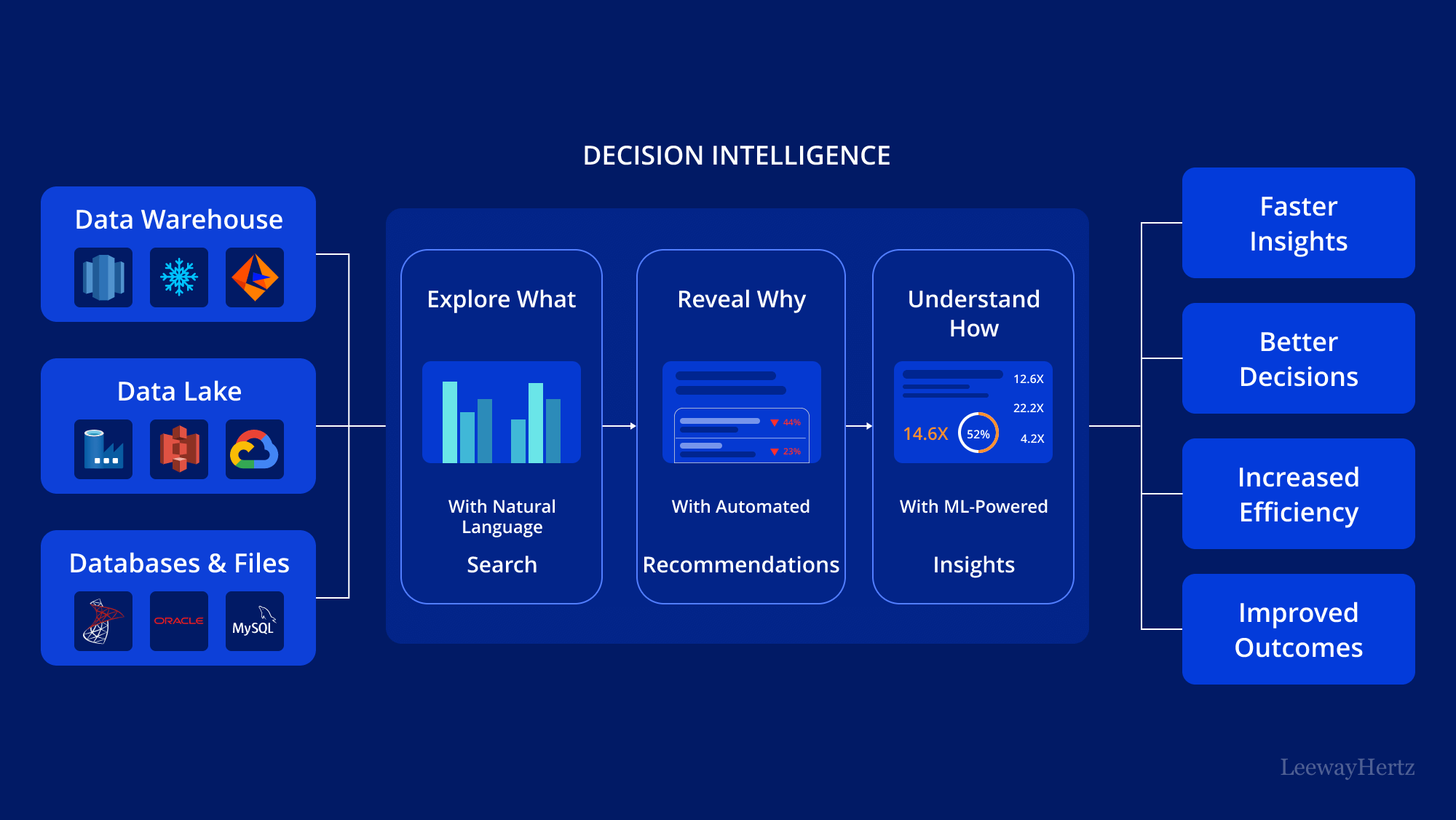
Decision intelligence combines data analytics, artificial intelligence (AI), and human judgment to enhance decision-making processes. Unlike conventional business intelligence, which focuses on historical data analysis, DI aims to provide actionable insights that anticipate future outcomes and recommend the best courses of action. The global decision intelligence market was valued at $9.8 billion in 2021 and is projected to reach $39.3 billion by 2031, growing at a compound annual growth rate (CAGR) of 15.2% from 2022 to 2031. This substantial growth underscores the increasing reliance on DI in today’s data-driven world.
As businesses face unprecedented challenges and competition, the ability to make informed, timely decisions can be a game-changer. DI not only helps in predicting future trends but also in prescribing actions to optimize outcomes. Its application ranges from supply chain management to customer experience, offering businesses a significant edge.
This article explores decision intelligence from multiple angles. We will start by defining DI and how it differs from traditional business intelligence. Then, we’ll examine the core principles and key benefits of implementing DI in your organization. The article also delves into the steps for successful DI implementation, discusses the tools and technologies involved, and addresses ethical considerations. Finally, we’ll look at DI’s impact across industries and offer insights into its future outlook.
- What is decision intelligence?
- How does decision intelligence work?
- Decision intelligence vs. business intelligence: A comparison
- Use cases of Decision Intelligence (DI) across industries
- The benefits of implementing decision intelligence
- Implementing decision intelligence: From strategy to execution
- The ethical considerations of decision intelligence
- What is the future outlook for decision intelligence?
What is decision intelligence?
Decision Intelligence (DI) is a data-driven approach to decision-making that leverages advanced analytics, machine learning, and artificial intelligence (AI) to empower organizations with actionable insights. It goes beyond traditional business intelligence (BI) by not only providing information but also actively guiding and automating the decision-making process.
At its core, decision intelligence is about:
- Data-driven insights: Harnessing the power of data to extract meaningful patterns, trends, and predictions that inform strategic decisions.
- Automated decision-making: Leveraging AI and machine learning to automate repetitive and complex decision tasks, freeing up human resources for more strategic initiatives.
- Optimizing outcomes: Using data-driven insights to improve the quality, consistency, and effectiveness of decisions, ultimately leading to better business outcomes.
Key principles of decision intelligence
Decision Intelligence (DI) is built on several foundational principles that guide its implementation and ensure its effectiveness. These principles help organizations harness the power of data and technology to make informed, strategic decisions. Here are the key principles of Decision intelligence:
- Data-centricity: Decision intelligence relies heavily on high-quality data, ensuring its accuracy, completeness, and accessibility for analysis and modeling.
- Analytical rigor: Employing a range of advanced analytical techniques, including statistical modeling, machine learning, and predictive analytics, to derive insights from data.
- Transparency and explainability: Providing clear and understandable explanations for the insights derived, enabling stakeholders to trust and confidently act upon the recommendations.
- Continuous improvement: Encouraging a culture of experimentation, learning, and feedback loops to refine decision-making processes and models over time.
How does decision intelligence work?
Decision intelligence (DI) works by bringing together data, analytics, and domain expertise to inform and automate better decisions. It’s like a bridge between the world of data and the world of action, enabling organizations to make smarter choices that drive better results.
Here’s a breakdown of how decision intelligence works in practice:
1. Data collection and integration
- Gathering data from multiple sources: DI begins by collecting data from various internal and external sources, including structured data (such as databases and spreadsheets) and unstructured data (like emails, social media, and text documents). This data could encompass financial records, customer interactions, market trends, sensor data, and more.
- Data integration: The next step is integrating this data into a cohesive framework. DI systems pull data from disparate sources, creating a unified data repository. This allows for a holistic view of the organization’s operations, eliminating silos and enabling comprehensive analysis.
2. Data preprocessing and quality assurance
- Data cleaning and transformation: Before analysis, the data is cleaned and transformed. This involves removing duplicates, correcting errors, filling in missing values, and converting data into a standardized format. High-quality data is crucial for accurate and reliable analysis.
- Data normalization and enrichment: Data normalization ensures consistency across the data set, while enrichment involves adding relevant external data to enhance the dataset’s value. This step is vital for ensuring that the data is robust and ready for complex analysis.
3. Model building and simulation
- Developing predictive models: Using AI and ML algorithms, DI systems build predictive models that analyze historical data to identify patterns and trends. These models can forecast future scenarios based on past behavior, enabling proactive decision-making.
- Simulation of scenarios: DI systems use these models to simulate various scenarios. By altering variables, organizations can explore different outcomes and strategies without risk. This helps decision-makers understand potential impacts and optimize strategies.
4. Analysis and insights generation
- Descriptive and diagnostic analysis: This step involves understanding what has happened in the past and diagnosing why it happened. By identifying trends, anomalies, and relationships in the data, DI helps organizations grasp the underlying factors influencing outcomes.
- Predictive and prescriptive analysis: Predictive analysis uses models to anticipate future events or trends, while prescriptive analysis provides recommendations on the best courses of action. Together, these analyses offer both foresight and actionable strategies.
5. Decision modeling and optimization
- Creating decision models: Decision models represent the different choices available to decision-makers and their potential outcomes. These models incorporate various factors, such as constraints, objectives, and risk assessments, to evaluate the potential impact of each decision.
- Optimization techniques: DI uses optimization algorithms to identify the most effective decision based on desired outcomes. This could involve maximizing profit, minimizing risk, enhancing customer satisfaction, or achieving a balance among multiple objectives.
6. Visualization and reporting
- Interactive dashboards: DI systems present insights through interactive dashboards and visualizations that make complex data more understandable. These dashboards allow users to drill down into specific areas of interest, compare scenarios, and monitor key performance indicators (KPIs).
- Automated reporting: Automated reporting tools generate comprehensive reports that summarize findings and provide actionable recommendations. These reports can be customized for different stakeholders, ensuring relevant insights are communicated effectively.
7. Decision execution and monitoring
- Implementing decisions: Once a decision is made, DI helps in executing it by providing detailed action plans and monitoring tools. This ensures that strategies are implemented effectively and efficiently across the organization.
- Continuous monitoring and feedback loop: DI doesn’t end with decision execution. Continuous monitoring ensures that decisions are delivering the expected outcomes. If deviations are observed, DI systems can provide feedback, allowing for adjustments and recalibrations in real-time.
8. Learning and adaptation
- Learning from outcomes: As decisions are implemented and outcomes are measured, DI systems learn from these results. This ongoing learning process enables the refinement of models and strategies over time, improving the quality of future decisions.
- Adaptive decision-making: By incorporating feedback and adapting to new data, DI ensures that decision-making remains relevant and effective, even as conditions change. This adaptive approach helps organizations stay agile and responsive in a dynamic environment.
9. Collaboration and augmented intelligence
- Facilitating human-AI collaboration: DI enhances decision-making by augmenting human judgment with AI-driven insights. While AI provides data-driven recommendations, human expertise and intuition are critical for interpreting these insights and making nuanced decisions.
- Collaborative decision-making platforms: DI platforms often include tools for collaboration, enabling teams to discuss insights, align on strategies, and make collective decisions. This fosters a more inclusive and transparent decision-making process.
Example:
Imagine a retail company using DI to optimize its inventory management.
- Data: The company collects data on sales, demand patterns, supplier performance, and inventory levels.
- Analytics: DI models analyze historical data to predict future demand and forecast inventory needs.
- Decisions: The system recommends optimal inventory levels for each product, minimizing stockouts and excess inventory.
- Automation: The system automatically triggers orders when inventory reaches predefined thresholds.
- Evaluation: The company tracks metrics like sales, customer satisfaction, and inventory costs to evaluate the effectiveness of the DI-driven decisions.
Decision intelligence works by leveraging data, analytics, and automation to empower organizations to make smarter, more informed decisions that lead to better outcomes. It’s a powerful tool for driving business performance, improving customer experiences, and staying ahead of the competition.
Decision intelligence vs. business intelligence: A comparison
Aspect | Decision Intelligence (DI) | Business Intelligence (BI) |
---|---|---|
Definition | A field that combines data analysis, AI, and cognitive science to improve decision-making by providing actionable insights and recommendations. | A set of technologies and practices for collecting, analyzing, and presenting business data to help managers make informed decisions. |
Focus | Emphasizes improving decision-making processes by integrating advanced analytics, and AI. | Focuses on analyzing historical data and generating reports for monitoring business performance and supporting decision-making. |
Data processing | Uses predictive and prescriptive analytics to forecast future scenarios and recommend actions. | Primarily uses descriptive analytics to summarize past performance and provide insights based on historical data. |
Analytics type | Includes predictive (what might happen) and prescriptive (what should be done) analytics. | Primarily uses descriptive (what happened) and diagnostic (why it happened) analytics. |
Decision support | Provides actionable insights and recommendations to guide decision-making and optimize outcomes. | Provides historical data analysis and reporting to support decision-making based on past performance. |
Technology integration | Integrates with AI and cognitive technologies to enhance decision-making capabilities. | Utilizes traditional data warehousing, reporting tools, and dashboards for data visualization and analysis. |
Real-time analysis | Often incorporates real-time or near-real-time data analysis to provide timely recommendations. | May focus on periodic reports and batch processing of historical data, often with less emphasis on real-time analysis. |
User interaction | Offers interactive and adaptive decision support systems that can evolve based on user feedback and data patterns. | Provides static reports and dashboards, often requiring manual updates and less adaptive to real-time changes. |
Use cases | Suitable for complex decision-making scenarios where recommendations and forecasting are critical, such as in strategic planning, risk management, and operational optimization. | Primarily used for operational and tactical decision-making, such as performance tracking, trend analysis, and routine reporting. |
Outcome orientation | Focuses on optimizing outcomes by recommending actions and predicting future trends. | Focuses on understanding past performance and providing insights to monitor and manage current business operations. |
Data sources | Integrates diverse data sources, including structured and unstructured data, IoT, and external data feeds. | Typically relies on structured data from internal sources such as databases, ERP systems, and spreadsheets. |
Complexity | Often involves complex algorithms, machine learning models, and sophisticated data processing techniques. | Generally involves data aggregation, simple queries, and reporting tools with less emphasis on complex analytics. |
Customization | Highly customizable to adapt to specific business needs and decision-making processes. | Customizable to an extent but often constrained by the capabilities of the BI tools and predefined report formats. |
Adoption | Emerging field with growing adoption as organizations seek advanced decision-making capabilities. | Well-established with widespread adoption across various industries for traditional data analysis and reporting. |
Use cases of Decision Intelligence (DI) across industries
Decision Intelligence (DI) is transforming how industries operate by leveraging data, advanced analytics, and AI to improve decision-making processes. Its impact spans across various sectors, driving efficiency, innovation, and competitive advantage. Below is an overview of how DI is affecting key industries:
Healthcare
- Personalized medicine: DI enables the analysis of large datasets, including genetic information, patient history, and treatment outcomes, to tailor personalized treatment plans. This improves patient outcomes and optimizes resource use.
- Impact: Reduced treatment costs, improved patient care, and more accurate diagnoses.
- Operational efficiency: DI helps healthcare providers optimize scheduling, resource allocation, and inventory management. This leads to more efficient operations and reduces wait times for patients.
- Impact: Increased patient throughput, lower operational costs, and improved service delivery.
- Predictive analytics: By predicting patient admission rates, disease outbreaks, and resource needs, DI assists in proactive healthcare management.
- Impact: Better preparedness, reduced strain on healthcare systems, and timely intervention.
Finance and banking
- Risk management: DI enhances risk assessment by analyzing market trends, customer behaviors, and financial transactions. It helps in identifying potential risks and frauds before they escalate.
- Impact: Improved risk mitigation, reduced losses, and enhanced regulatory compliance.
- Customer insights: By analyzing customer data, DI helps banks and financial institutions personalize services, from loan offers to investment advice.
- Impact: Increased customer satisfaction, higher retention rates, and improved cross-selling opportunities.
Retail and e-commerce
- Demand forecasting: DI helps retailers predict demand for products based on historical sales data, seasonal trends, and external factors.
- Impact: Optimized inventory levels, reduced stockouts, and minimized excess inventory.
- Personalized marketing: Retailers use DI to analyze customer behavior and preferences, enabling the creation of highly targeted marketing campaigns.
- Impact: Increased conversion rates, higher customer loyalty, and improved marketing ROI.
Manufacturing
- Predictive maintenance: DI uses sensor data and historical maintenance records to predict equipment failures before they happen, enabling timely interventions.
- Impact: Reduced downtime, lower maintenance costs, and extended equipment life.
- Quality control: DI analyzes production data to identify defects and inefficiencies in the manufacturing process, leading to better quality products.
- Impact: Improved product quality, reduced waste, and higher customer satisfaction.
- Production optimization: DI helps optimize production schedules, resource allocation, and energy consumption, enhancing overall operational efficiency.
- Impact: Increased productivity, reduced costs, and sustainable manufacturing practices.
Telecommunications
- Network optimization: DI analyzes network usage patterns to optimize the allocation of bandwidth and resources, improving service quality for customers.
- Impact: Reduced network congestion, improved user experience, and enhanced network reliability.
- Customer service: Telecommunications companies use DI to predict customer needs and issues, enabling proactive customer support and personalized service.
- Impact: Increased customer satisfaction, lower churn rates, and better service delivery.
- Fraud detection: DI helps identify and prevent fraudulent activities, such as identity theft and unauthorized access, by analyzing usage patterns and transaction data.
- Impact: Reduced fraud losses, improved security, and enhanced trust.
Transportation and logistics
- Route optimization: DI enables the optimization of transportation routes based on real-time traffic data and delivery schedules.
- Impact: Reduced fuel consumption, faster delivery times, and lower operational costs.
- Fleet management: DI helps in managing vehicle fleets by predicting maintenance needs, optimizing vehicle utilization, and reducing downtime.
- Impact: Improved fleet efficiency, lower maintenance costs, and increased vehicle lifespan.
- Supply chain visibility: DI provides end-to-end visibility into the supply chain, enabling better decision-making regarding inventory management, supplier performance, and logistics.
- Impact: Enhanced supply chain agility, reduced lead times, and improved customer satisfaction.
Education
- Personalized learning: DI enables educational institutions to tailor learning experiences based on individual student data, such as performance, learning style, and engagement levels.
- Impact: Improved student outcomes, higher engagement, and reduced dropout rates.
- Resource allocation: Schools and universities use DI to optimize the allocation of resources, such as faculty, classrooms, and funding, based on demand and performance data.
- Impact: More efficient use of resources, better student support, and enhanced educational quality.
- Curriculum development: DI helps in analyzing trends in the job market and student performance to develop curricula that are aligned with industry needs and student capabilities.
- Impact: More relevant education, better career readiness, and increased student satisfaction.
Legal
- Case outcome prediction: DI can analyze historical case data, judge rulings, and legal precedents to predict the likely outcome of legal disputes. This helps lawyers develop more effective strategies.
- Impact: Improved case preparation, increased success rates, and more informed decision-making.
- Contract analysis: DI can review and analyze contracts to identify potential risks, compliance issues, and clauses that may lead to disputes.
- Impact: Reduced legal risk, faster contract review processes, and better compliance.
- Legal research automation: DI tools can sift through vast legal databases to find relevant cases, statutes, and regulations quickly.
- Impact: Increased research efficiency, reduced time spent on case preparation, and enhanced legal accuracy.
Insurance
- Risk assessment: DI enhances underwriting processes by analyzing vast datasets, including customer demographics, behavior, and historical claims, to assess risk more accurately.
- Impact: More precise premium pricing, reduced fraud, and improved customer satisfaction.
- Claims management: DI helps in automating claims processing by predicting fraudulent claims and streamlining the assessment process.
- Impact: Faster claims resolution, lower operational costs, and reduced fraud incidence.
- Customer retention: By analyzing customer data, DI enables insurers to offer personalized policies and identify at-risk customers who may cancel their policies.
- Impact: Increased customer loyalty, higher retention rates, and improved cross-selling opportunities.
Real estate
- Property valuation: DI uses market data, historical property prices, and neighborhood trends to provide accurate property valuations.
- Impact: Better investment decisions, more accurate pricing, and increased transparency in real estate transactions.
- Market trend analysis: DI analyzes buyer behavior, and demographic trends to predict market movements and inform investment strategies.
- Impact: Improved investment returns, reduced risk, and more informed decision-making.
- Customer preferences: DI can analyze buyer preferences and behaviors to help real estate companies tailor their offerings and marketing strategies.
- Impact: Enhanced customer satisfaction, increased sales, and more effective marketing.
Automotive
- Product development: DI analyzes customer feedback, market trends, and competitive data to guide the development of new vehicles and features.
- Impact: Faster time-to-market, higher customer satisfaction, and more innovative products.
- Supply chain management: DI helps automotive manufacturers optimize their supply chains by predicting demand, managing inventory, and coordinating logistics.
- Impact: Reduced production costs, improved supply chain resilience, and faster delivery times.
- Autonomous vehicles: DI is critical in the development and operation of autonomous vehicles, enabling real-time decision-making based on sensor data and AI models.
- Impact: Enhanced vehicle safety, reduced human error, and accelerated adoption of autonomous technology.
- Customer experience: DI tools analyze customer preferences and driving patterns to offer personalized services, such as maintenance reminders and customized in-car experiences.
- Impact: Improved customer satisfaction, increased brand loyalty, and better service delivery.
Decision intelligence is transforming industries by enabling smarter, data-driven decisions that improve efficiency, reduce costs, and drive innovation. As DI continues to evolve, its impact on various sectors will likely grow, leading to even more significant advancements in how businesses operate and compete in the global market.
The benefits of implementing decision intelligence
Implementing decision intelligence offers a wide range of benefits that can significantly enhance an organization’s decision-making capabilities, leading to improved outcomes across various aspects of the business. Here are some of the key benefits:
1. Enhanced decision-making accuracy
- Data-driven insights: Decision intelligence ensures that decisions are based on comprehensive, real-time data analysis, reducing reliance on intuition and guesswork. This leads to more accurate and reliable decisions.
- Elimination of bias: By leveraging AI and advanced analytics, decision intelligence helps minimize human biases in decision-making, resulting in more objective and consistent outcomes.
2. Increased operational efficiency
- Automation of routine decisions: Decision intelligence can automate routine decision-making processes, freeing up human resources for more complex and strategic tasks. This leads to faster decision cycles and improved efficiency.
- Optimized resource allocation: By providing insights into resource utilization, decision intelligence enables organizations to optimize their resources, reducing waste and maximizing productivity.
3. Improved risk management
- Proactive risk identification: Decision intelligence helps identify potential risks before they materialize by analyzing historical data and predicting future trends. This allows organizations to take proactive measures to mitigate risks.
- Better contingency planning: With scenario analysis and predictive modeling, decision intelligence facilitates the development of robust contingency plans, ensuring that organizations are better prepared for uncertainties.
4. Enhanced strategic planning
- Informed long-term decisions: Decision intelligence provides data-driven insights that inform long-term strategic planning, helping organizations align their actions with their goals and objectives.
- Scenario analysis and forecasting: By simulating various scenarios, decision intelligence allows organizations to explore different strategic options and assess their potential impact, leading to more informed and confident decision-making.
5. Greater agility and adaptability
- Real-time decision-making: Decision intelligence enables organizations to make decisions in real-time, allowing them to respond quickly to changes in the market, customer behavior, or other external factors.
- Adaptation to market dynamics: With continuous monitoring and analysis, decision intelligence helps organizations stay agile and adapt to evolving market conditions, ensuring sustained competitiveness.
6. Enhanced customer insights and personalization
- Understanding customer behavior: Decision intelligence provides deep insights into customer preferences, behaviors, and needs, enabling organizations to tailor their products, services, and marketing strategies accordingly.
- Personalized customer experiences: By leveraging data on individual customer preferences, decision intelligence allows organizations to create personalized experiences that enhance customer satisfaction and loyalty.
7. Competitive advantage
- Innovation and differentiation: Organizations that implement decision intelligence can innovate more effectively and differentiate themselves from competitors by making better-informed decisions that drive business growth.
- Speed to market: Decision intelligence enables faster and more accurate decision-making, reducing the time required to bring new products or services to market, thereby gaining a competitive edge.
8. Cost reduction and efficiency gains
- Reduction in decision-making costs: Automating decision-making processes reduces the time and resources required for decision-making, leading to cost savings.
- Optimized operations: Decision intelligence tools help identify inefficiencies in business operations, leading to cost reduction through process optimization and better resource utilization.
9. Improved compliance and governance
- Regulatory compliance: Decision intelligence can assist organizations in ensuring compliance with regulations by analyzing data to detect potential compliance issues and recommending corrective actions.
- Enhanced governance: With transparent and well-documented decision-making processes, Decision intelligence supports better governance, ensuring accountability and alignment with organizational values and policies.
10. Scalability and flexibility
- Scalable decision-making framework: Decision intelligence frameworks are scalable and can be applied across various levels of the organization, ensuring consistent decision-making processes as the organization grows.
- Flexibility across use cases: Decision intelligence is versatile and can be tailored to different industries, functions, and decision-making scenarios, making it applicable across a wide range of business contexts.
11. Long-term business sustainability
- Sustainable growth: By enabling more strategic, informed, and ethical decision-making, decision intelligence contributes to the long-term sustainability and success of the organization.
- Alignment with ESG goals: Decision intelligence can help organizations align their decisions with environmental, social, and governance (ESG) goals, promoting responsible business practices.
Implementing decision intelligence provides organizations with the tools and insights needed to make better decisions, improve efficiency, and drive sustainable growth. These benefits contribute to a stronger competitive position, enhanced customer experiences, and overall business success.
Implementing decision intelligence: From strategy to execution
Implementing Decision Intelligence (DI) within an organization involves a structured approach that integrates data, technology, and human expertise to improve decision-making processes. Here are the essential steps to successfully implement DI:
Define your scope and objectives:
- Identify key decision areas: Pinpoint the areas where DI can have the most significant impact, focusing on high-value decisions with clear metrics for success.
- Set clear goals: Define specific, measurable, achievable, relevant, and time-bound (SMART) objectives for your DI initiative. What outcomes do you want to achieve?
- Determine your budget and resources: Assess the financial and human resources needed for successful implementation. Consider the technology, expertise, and training required.
Establish a data foundation:
- Data inventory and assessment: Identify all relevant data sources within your organization. Evaluate data quality, accessibility, and relevance to your decision-making needs.
- Data integration and management: Develop a robust data infrastructure that integrates data from various sources into a unified platform.
- Data governance and security: Implement policies and procedures for data management, quality control, security, and compliance with privacy regulations.
Build your analytics and AI capability:
- Choose the right tools: Select analytical and machine learning tools based on your specific needs, data volume, and technical expertise.
- Develop data science and AI expertise: Hire or train internal personnel in data science, machine learning, and analytics. Partner with external consultants if needed.
- Establish a model development and deployment pipeline: Create a standardized process for building, testing, deploying, and monitoring AI models.
Design and implement decision models:
- Define decision frameworks: Formalize your decision-making processes by creating clear frameworks that define inputs, outputs, and decision rules.
- Develop decision models: Use decision trees, Bayesian networks, influence diagrams, or other modeling techniques to represent your decision-making logic.
- Validate and test models: Thoroughly test and validate models against historical data to ensure accuracy and reliability.
Integrate with existing systems and applications:
- Data pipelines: Develop automated data pipelines to seamlessly integrate data from various sources into your DI platform.
- API integration: Connect your DI platform with existing business systems and applications to enable real-time data access and decision automation.
- User interface development: Design user-friendly interfaces that enable easy access and interpretation of insights for decision-makers.
Automate and optimize decision execution:
- Identify tasks for automation: Analyze decision-making processes to identify tasks that can be automated using robotic process automation (RPA) or AI-powered tools.
- Implement automation solutions: Integrate automation tools into your decision-making processes to streamline operations and improve efficiency.
- Monitor and optimize: Continuously monitor automated processes to identify areas for improvement and optimize for efficiency and accuracy.
Establish a decision intelligence culture:
- Leadership support: Ensure top-level support for the DI initiative to drive adoption and commitment throughout the organization.
- Data literacy training: Provide training programs to equip employees with the necessary data literacy skills to understand and utilize data-driven insights.
- Data-driven communication: Encourage a culture of data-driven communication where decisions are backed by evidence and insights.
Continuously evaluate and improve:
- Track Key Performance Indicators (KPIs): Monitor and track the performance of your DI initiative against predefined objectives and KPIs.
- Iterative improvement: Continuously evaluate and refine your DI approach based on learnings, data insights, and feedback from users.
- Embrace change: Decision intelligence is an evolving field, so stay informed about emerging trends and technologies to ensure your DI initiatives remain cutting-edge.
Key considerations:
- Start small and scale gradually: Begin with a pilot project in a specific area to demonstrate the value of DI before scaling to larger initiatives.
- Foster collaboration and communication: Ensure effective communication and collaboration between data scientists, business analysts, and decision-makers.
- Address ethical considerations: Implement measures to mitigate bias, ensure data privacy, and maintain transparency in your AI models.
By following this structured approach, organizations can successfully implement decision intelligence to drive better decisions, optimize operations, and achieve a competitive advantage in today’s data-driven world.
The ethical considerations of decision intelligence
Implementing Decision Intelligence (DI) comes with several ethical considerations that organizations must carefully navigate to ensure fairness, transparency, and accountability in their decision-making processes. Here are some key ethical considerations of decision intelligence:
1. Data privacy and security
- Protection of sensitive data: DI relies on vast amounts of data, some of which may be sensitive or personal. Organizations must ensure that data collection and analysis processes comply with privacy laws and regulations, such as GDPR or CCPA, to protect individuals’ privacy rights.
- Data security: Securing data against breaches and unauthorized access is critical to maintaining trust. Organizations must implement robust cybersecurity measures to protect data integrity and confidentiality.
2. Bias and fairness
- Algorithmic bias: Decision Intelligence systems can unintentionally incorporate biases present in the data or in the algorithms themselves. These biases can lead to unfair or discriminatory outcomes. Organizations must regularly audit their DI systems for bias and ensure they represent diverse perspectives.
- Fair decision-making: Ensuring that DI systems promote fair outcomes for all stakeholders is crucial. This involves using inclusive datasets, developing fair algorithms, and continually testing systems for unbiased performance.
3. Transparency and explainability
- Explainability of decisions: DI systems often make complex decisions that are not easily understandable. Providing clear explanations for how decisions are made is essential for maintaining trust with stakeholders and ensuring compliance with regulations.
- Transparency in processes: Organizations should be transparent about how their DI systems function, including the data used, the algorithms applied, and the criteria for decision-making. This helps build trust and allows stakeholders to understand the reasoning behind decisions.
4. Accountability
- Responsibility for outcomes: Organizations must be accountable for the decisions made by their DI systems, especially in high-stakes environments like healthcare, finance, or criminal justice. It is important to establish clear lines of responsibility and governance over the use of DI.
- Monitoring and auditing: Continuous monitoring and regular audits of DI systems are necessary to ensure that they are functioning as intended and that any unintended consequences are promptly addressed.
5. Informed consent
- Consent for data use: Users whose data is being used for decision intelligence should be informed about how their data will be used and should provide consent. This is especially important when data is collected from personal or sensitive sources.
- Opt-out options: Providing users with the ability to opt out of data collection or DI-driven decisions respects their autonomy and enhances trust.
6. Manipulation and influence
- Ethical use of insights: DI systems can provide deep insights into consumer behavior, which could be used to manipulate decisions or influence behaviors unfairly. Organizations must use DI insights ethically and avoid exploiting users or customers.
In summary, while decision intelligence offers significant benefits for improving decision-making processes, it also presents several ethical challenges. Organizations must approach DI with a strong ethical framework to ensure that it is implemented responsibly, fairly, and transparently, minimizing negative impacts while maximizing positive outcomes for all stakeholders.
What is the future outlook for decision intelligence?
The future of Decision Intelligence (DI) is brimming with exciting possibilities, fueled by rapid advancements in technology and a growing recognition of its transformative potential. Here’s a glimpse into the key trends and opportunities shaping the future of DI:
1. Explainable AI (XAI) and transparency:
- Increasing demand for transparency: As DI becomes more widely adopted, there’s a growing demand for explainability and transparency in AI models. Users want to understand how decisions are made, especially in sensitive contexts like healthcare or finance.
- Advancements in XAI: Research and development in explainable AI (XAI) are creating new tools and techniques to provide insights into model predictions and decision-making processes. This will foster trust, accountability, and ethical decision-making.
2. Federated learning and data privacy:
- Addressing data privacy concerns: Federated learning enables collaborative model training without sharing raw data between organizations. This addresses privacy concerns while allowing for the development of more powerful and accurate models.
- Increased adoption: As privacy regulations like GDPR become more stringent, federated learning will likely become a crucial tool for implementing DI in data-sensitive environments.
3. Integration with business processes and platforms:
- Seamless integration: DI is increasingly integrated into existing business processes and platforms, enabling real-time decision-making and automation.
- End-to-end solutions: Vendors are developing end-to-end DI solutions that encompass data collection, analysis, modeling, and deployment, simplifying implementation and adoption for organizations.
The future of decision intelligence is bright, with its potential to transform decision-making across all industries and address pressing global challenges. As the technology continues to evolve, it will be essential to prioritize ethical considerations, foster collaboration, and ensure that the benefits of DI are shared equitably.
Endnote
Decision intelligence is quickly becoming a vital tool for businesses looking to navigate the complexities of modern data and decision-making. By integrating data analytics, AI, and human judgment, DI enhances the ability to make informed decisions that drive better outcomes. Whether it’s improving operational efficiency, optimizing customer experiences, or forecasting future trends, DI offers a structured approach that traditional methods often lack.
As more organizations adopt decision intelligence, its impact across industries like finance, healthcare, and manufacturing is evident. The ability to predict, prescribe, and act on insights in real-time provides businesses with a competitive edge in a rapidly changing landscape. However, implementing DI requires careful planning, ethical considerations, and the right tools and technologies to ensure success.
Looking ahead, the role of decision intelligence is set to grow, with advancements in AI and machine learning pushing the boundaries of what’s possible. For businesses willing to embrace this approach, the potential benefits are significant. By aligning DI strategies with business goals, organizations can not only improve their decision-making processes but also position themselves for long-term success in an increasingly data-driven world.
Ready to harness the power of decision intelligence for your business? Connect with LeewayHertz’s AI experts today to explore tailored solutions that drive smarter decisions and better outcomes.
Start a conversation by filling the form
All information will be kept confidential.
Insights
Generative AI in business: Transforming industry dynamics
Generative AI, powered by advanced machine learning techniques, has emerged as a transformative technology with profound implications for businesses across various industries.
Actionable AI: An evolution from Large Language Models to Large Action Models
Actionable AI not only analyzes data but also uses those insights to drive specific, automated actions.
The role of AI in logistics and supply chain
By harnessing the capabilities of AI, companies can refine their operations and elevate their business performance, leading to enhanced profitability, operational efficiency, and increased customer satisfaction.