Understanding AIOps: Its working, phases, types, use cases, benefits and implementation
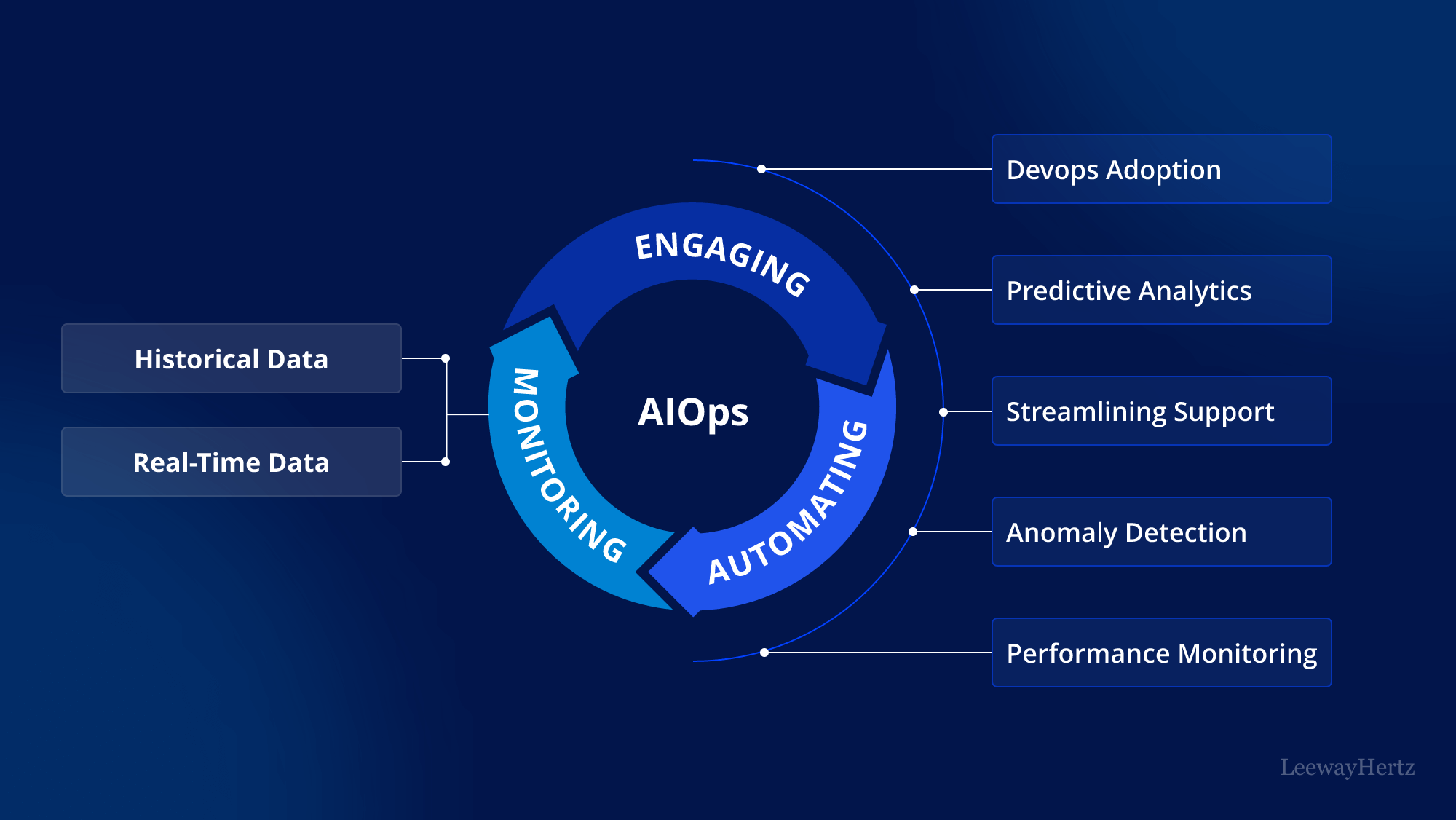
As technology evolves, the management of IT infrastructures becomes increasingly complex, posing greater challenges for IT departments. The rapid pace of digital transformation underscores the essential requirement for streamlined IT operations capable of effectively handling intricate datasets. Moreover, modern IT operations extend beyond merely managing and servicing systems and devices. They now emphasize the strategic implementation of AI-driven solutions to adeptly navigate and lead within the constantly shifting technological environment. AI for IT operations (AIOps) meets this demand by integrating AI with automated algorithms, providing IT teams comprehensive visibility into system performance amidst escalating data loads and dynamic application architectures. As per Markets and Markets, in 2023, the global market for AIOps platforms was valued at USD 11.7 billion and is projected to grow to USD 32.4 billion by 2028, with a compound annual growth rate (CAGR) of 22.7%.
AIOps integrates artificial intelligence (AI) with IT operations (Ops), leveraging machine learning, analytics, and data science to detect and resolve IT operational issues autonomously. By analyzing vast amounts of data in real time, AIOps enhance operational efficiency and predict and mitigate potential disruptions before they occur. This convergence of AI and IT operations marks a paradigm shift, empowering organizations to streamline processes, reduce downtime, and optimize resource utilization. As businesses navigate increasingly intricate IT landscapes, AIOps are pivotal in maintaining agility, scalability, and reliability in the digital era.
This article delves into AIOps, covering its various types, phases, use cases, and operational mechanisms. It provides a comprehensive overview of its application in modern IT environments.
- What are AIOps?
- What drives AIOps?
- Core elements of AIOps
- Types of AIOps
- Understanding different phases of AIOps
- How does AIOps work?
- AIOps compared to other IT concepts
- Use cases of AIOps
- AIOps tools
- Benefits of AIOps
- Why is LeewayHertz your ideal partner for AIOps implementation?
- How to implement AIOps in your organization?
- Best practices for building AIOps strategy
What are AIOps?
Artificial Intelligence for IT Operations (AIOps) leverages AI techniques to maintain IT infrastructure by automating essential operational tasks such as performance monitoring, workload scheduling, and data backups. By utilizing machine learning (ML), natural language processing (NLP), and other advanced AI methodologies, AIOps enhances IT operational efficiency. These technologies provide proactive, personalized, and real-time insights by collecting and analyzing data from various sources, thus improving the overall effectiveness of IT operations.
AIOps utilizes big data, analytics, and machine learning to accomplish the following:
- Data collection and aggregation: Collect and consolidate the massive and continually growing volumes of data generated by various IT infrastructure components, application demands, performance-monitoring tools, and service ticketing systems.
- Signal identification: Effectively distinguishes important events and patterns related to application performance and availability issues from irrelevant or less significant data.
- Root cause diagnosis: Diagnose root causes and report them to IT and DevOps teams for swift response and remediation, or in some cases, automatically resolve issues without human intervention.
AIOps enhances IT operations in real-world scenarios by automating routine tasks and providing proactive insights. For example, AIOps platforms can analyze vast amounts of IT data to detect anomalies, predict potential issues before they occur, and automate incident response. This capability is essential for maintaining system reliability, optimizing resource use, and enhancing overall operational efficiency within IT environments.
AIOps integrates disparate manual IT operations tools into a unified, intelligent platform, enabling faster and more proactive responses to slowdowns and outages. This enhances end-to-end visibility and context for IT operations teams.
AIOps bridges the gap between a diverse, dynamic, and challenging-to-monitor IT landscape on one side and user expectations for minimal to no interruption in application performance and availability on the other. Most experts view AIOps as the future of IT operations management, with demand growing alongside the business focus on digital transformation initiatives.
What drives AIOps?
The shift towards AIOps is driven by several key trends and demands, primarily stemming from the increased complexity of modern technology. Here are some specific factors behind this evolution:
Expanding IT environments
Modern, dynamic IT environments have grown beyond the capabilities of manual oversight. Their complexity and scale necessitate automated solutions for effective management.
Exponentially increasing ITOps data
The proliferation of mobile apps, IoT devices, and machine users, coupled with the widespread use of APIs to access and transfer data, has led to the generation and availability of vast amounts of valuable data. Machine learning and AI are essential for efficiently analyzing and reporting this data.
More computing power at the network edge
The decentralization of networks through cloud computing and third-party services has created an IT ecosystem in which significant budget and computing power are allocated to the network’s edge.
Growing developer influence but not accountability
As applications become more central to business operations, developers are increasingly involved in monitoring and other areas. However, the core accountability for IT remains with the IT department, which must manage increased complexity and responsibility as technologies advance.
Digital transformation
The ongoing digital transformation drives organizations to adopt cloud computing, IoT, and other advanced technologies, significantly increasing the complexity of their IT environments. AIOps addresses this complexity by automating tasks like monitoring, troubleshooting, and optimizing IT operations, ensuring efficient management of modern IT landscapes.
Business agility
In the current fast-paced market, companies must respond quickly to evolving customer needs and shifting market conditions to stay competitive. AIOps enhances agility by offering real-time insights into IT performance and health. This approach enables organizations to detect and resolve issues quickly, minimizing downtime and ensuring continuous service delivery.
The driving forces behind AIOps include the need to manage expanding and complex IT environments, handle vast amounts of data, resolve issues quickly, adapt to decentralized computing power, and balance developers’ growing influence with IT accountability.
Optimize Your Operations With AI Agents
Optimize your workflows with ZBrain AI agents that automate tasks and empower smarter, data-driven decisions.
Core elements of AIOps
To understand AIOps better, let’s look at its key elements:
- Comprehensive IT data: AIOps breaks down data barriers by gathering information from IT service and operations management. This helps quickly pinpoint problems and sets the stage for automation.
- Big data: AIOps thrives on big data. By organizing and freeing up data, it can analyze historical and real-time information with advanced tools.
- Machine learning: AIOps uses machine learning to analyze vast amounts of data swiftly and accurately. This helps find patterns and insights that take time to uncover manually.
- Observation: AIOps observe data and behaviors across different IT systems, like cloud services. Real-time data collection ensures the most current insights.
- Engagement: AIOps manage systems and software across various IT areas, integrating management for smoother operations and automation.
- Taking action: The ultimate goal of AIOps is full automation, freeing up IT teams for more strategic work. While adoption varies, many organizations see its value in effectively handling simple tasks and complex challenges.
Types of AIOps
Here’s an explanation of the two types of AIOps solutions:
Domain-centric AIOps
Domain-centric AIOps are tailored to operate within specific domains or organizational scopes. These are focused on optimizing and managing IT operations within defined areas such as networking, application performance, or cloud computing.
- Example: Operational teams use domain-centric AIOps platforms to monitor and manage the performance of networking infrastructure, applications, and cloud services within their domain.
- Purpose: They provide deep insights and actionable intelligence specific to their domain, helping teams optimize operations, detect issues, and enhance performance within their specialized area.
Domain-agnostic AIOps
Domain-agnostic AIOps solutions are designed to scale predictive analytics and AI automation across broader network and organizational boundaries. These platforms collect and analyze event data from diverse sources across the IT landscape.
- Functionality: Domain-agnostic AIOps solutions aggregate and correlate event data from multiple domains, such as networking, applications, security, and more. They provide holistic insights and correlations across different IT domains.
- Benefit: By integrating data from various sources, domain-agnostic AIOps platforms offer comprehensive visibility and actionable insights that help organizations make informed decisions, optimize resources, and improve overall operational efficiency.
There are various other types of AIOps solutions, including:
On-premises vs. cloud-based AIOps:
- On-premises: Deployed within an organization’s infrastructure, offering direct control and better integration with existing systems.
- Cloud-based: Hosted and accessed via cloud platforms, providing scalability, flexibility, and reduced maintenance overhead.
Open-source vs. proprietary AIOps:
- Open-source: Allows users to access and modify the source code, fostering community collaboration and customization.
- Proprietary: Closed-source solutions offered by commercial vendors, typically offering polished features, support, and integration with proprietary systems.
Reactive vs. proactive AIOps:
- Reactive: Focuses on identifying and resolving issues as they arise, minimizing downtime and disruptions.
- Proactive: Emphasizes predicting and preventing issues before they impact operations, leveraging AI to anticipate and mitigate potential problems.
Full-stack vs. specialized AIOps:
- Full-stack: Provides comprehensive coverage across all aspects of IT operations, from infrastructure to applications, offering a holistic view for management and optimization.
- Specialized: This approach targets specific areas such as network performance, security monitoring, or application management, tailored to address focused operational needs with depth and specificity.
AI-assisted vs. fully autonomous AIOps:
- AI-assisted: Offers insights, recommendations, and automated actions based on AI analysis but requires human intervention for decision-making and oversight.
- Fully autonomous: Operates independently to detect, analyze, and resolve issues without human intervention, utilizing advanced AI algorithms and machine learning to optimize operations and minimize downtime.
These different AIOps help organizations choose the right solution based on their specific operational requirements, desired level of control, and strategic goals for IT management and performance.
Understanding different phases of AIOps
The phased approach in AIOps maturity allows organizations to evolve systematically from reactive problem-solving to proactive, automated IT operations. It fosters improved efficiency, better decision-making through data-driven insights, and readiness to leverage advanced technologies for sustained business success. Let’s break down the five phases of AIOps maturity in organizations:
Reactive phase: In the initial stage, organizations operate reactively, primarily addressing immediate IT issues and incidents. Data is collected and managed in silos, with minimal integration between different IT systems and limited alignment with broader business objectives.
Integrated phase: As organizations progress, they integrate various data sources into a unified framework. Silos between IT operations teams start breaking down, fostering improved communication and collaboration. The emphasis shifts towards enhancing IT service management (ITSM) through consolidating tools and processes to streamline operations and boost efficiency.
Analytical phase: In this phase, organizations establish a cohesive analytics strategy and promote data transparency across the organization. Organizations can enhance their ITSM processes by integrating data-driven insights, establishing measurement criteria, and defining foundational metrics. This approach enables them to monitor performance effectively and pinpoint areas for optimization.
Prescriptive phase: In the prescriptive phase, organizations prioritize automation and leverage machine learning capabilities. Automation becomes deeply embedded in ITSM processes, complementing human decision-making. Comparative analytics evaluate enhancements, while predictive analytics, leveraging machine learning models, help foresee and avert potential issues before they affect operations.
Automated phase: At the pinnacle of AIOps maturity, organizations achieve seamless data integration and complete automation of IT operations. Machine learning models are essential for predictive analysis and proactive decision-making. Transparency in analytics is critical, enabling organizations to make informed decisions that drive significant business value through optimized IT operations.
Each phase represents a progression towards greater integration, automation, and sophistication in leveraging AI and analytics to manage IT operations effectively. As organizations advance through these phases, they enhance their ability to proactively manage IT environments, optimize resources, and align IT activities with broader business objectives.
How does AIOps work?
Here’s an explanation of how AIOps works:
Step 1: Observation
In the observation step, AIOps involves intelligently collecting and analyzing data from various IT environments and sources. This phase enhances observability across the organization’s network using big data analytics and machine learning (ML) technologies.
- Data collection: AIOps gathers data in real time from diverse devices, applications, and data sources.
- Data aggregation and analysis: It aggregates and analyzes massive data volumes to identify patterns, anomalies, and correlations. For example, it can trace API request paths to pinpoint performance issues.
- Enhanced situational awareness: By processing data in real-time, AIOps improve situational awareness, enabling IT teams to detect issues early and understand their impact.
Step 2: Engaging human cognitive skills
After observation, AIOps engage human experts to respond to and resolve issues identified during the observation phase. This step reduces reliance on traditional IT metrics and alerts by providing contextual and actionable insights.
- Coordination and collaboration: AIOps facilitates efficient workload coordination across multi-cloud environments.
- Real-time alerts and notifications: It generates personalized, real-time alerts preemptively or in response to incidents, ensuring timely intervention and resolution.
- Unified dashboard: Operational teams use a shared dashboard to streamline collaborative efforts in diagnosis and assessment.
Step 3: Action
The action involves AIOps technologies taking automated actions to enhance and maintain IT infrastructure based on insights from previous phases.
- Process automation: AIOps automate operational processes, reducing manual intervention and enabling teams to focus on strategic tasks.
- ML-driven insights: It uses ML algorithms to derive analytics and insights that drive automated responses and actions. For instance, automated scripts can resolve recurring issues based on historical data and patterns.
- Continuous improvement: AIOps systems learn from past events to proactively optimize operations and prevent future incidents, enhancing overall system reliability.
- Support for business decisions: By enforcing IT policies and automating routine tasks, AIOps ensures IT resources are aligned with business objectives, supporting informed decision-making.
AIOps transforms IT operations by leveraging AI, big data analytics, and machine learning to enhance visibility, automate processes, and improve responsiveness. This approach enables organizations to shift from reactive to proactive management of IT environments, ultimately boosting operational efficiency and supporting business goals.
AIOps compared to other IT concepts
AIOps is a relatively new concept that leverages machine learning and big data processing to enhance IT operations. Here’s how it differs from several related terms:
AIOps vs. DevOps
- DevOps: It enables collaboration between development and operations teams, enabling quick deployment of changes and effective resolution of user concerns through shared information.
- AIOps: AIOps uses AI technologies to support and improve existing IT processes. DevOps teams utilize AIOps tools to assess code quality and accelerate software delivery times.
AIOps vs. MLOps
- MLOps: MLOps integrates machine learning models into digital products. It encompasses model selection, data preparation, training, evaluation, and deployment of ML applications in production.
- AIOps: AIOps applies ML solutions to generate actionable insights and enhance the efficiency of new and existing IT systems.
AIOps vs. SRE
- Site Reliability Engineering (SRE): It automates system operations and performs checks using software tools to improve software reliability and customer experience by automatically detecting and resolving issues.
- AIOps: AIOps shares similar goals with SRE. It utilizes large datasets and ML-generated predictive insights to assist site reliability engineers in reducing incident resolution times.
AIOps vs. DataOps
- DataOps: DataOps optimizes data usage for business intelligence by setting up data pipelines for ingestion, transformation, and transfer across different domains to support business operations.
- AIOps: AIOps builds on the data provided by DataOps, using it to detect, analyze, and resolve IT incidents, making it a more complex and comprehensive practice.
AIOps overlaps with other concepts like DevOps, MLOps, SRE, and DataOps; its primary focus is on using AI and ML to enhance IT operations through improved efficiency, predictive insights, and proactive incident management.
Optimize Your Operations With AI Agents
Optimize your workflows with ZBrain AI agents that automate tasks and empower smarter, data-driven decisions.
Use cases of AIOPs
The use cases of AIOps include:
Proactive problem-solving for seamless IT
AIOps rapidly processes and analyzes incident alerts, offering solutions before issues escalate. Detecting and addressing problems early prevents them from spiraling out of control. This ensures smoother IT operations and minimizes potential downtime, enhancing overall system reliability.
Predictive analytics
AIOps can analyze historical data from IT systems, including hardware and software performance metrics. By identifying patterns and anomalies, AIOps can predict potential failures or performance degradation before they occur. This proactive approach helps IT teams schedule maintenance activities, prevent unplanned downtime, and optimize resource utilization.
Streamlining support
AIOps enhances root cause analysis, enabling support agents to identify and resolve issues quickly, thus improving customer experience. It also allows IT desks to manage more tickets more accurately, benefiting businesses and customers.
Automated incident response
AIOps can automatically address issues as they arise with the right data and directives. This enables highly accurate identification, diagnosis, and remediation of problems much faster than human operators can achieve.
Digital transformation
AIOps facilitates digital transformation by helping IT operations manage the complexities associated with new technologies. This enables businesses to more readily adopt technological advances and pursue strategic goals, as AIOps provides tools to handle the increased workload and complexity in IT environments effectively. While IT operations still play a crucial role, AIOps significantly enhances their ability to support and drive digital initiatives.
Root cause analysis
AIOps conducts root cause analyses to pinpoint the source of problems, enabling teams to apply targeted solutions and avoid unnecessary work on symptomatic issues. For instance, an AIOps platform can swiftly identify and resolve a network outage while implementing preventive measures to forestall future occurrences.
Anomaly detection
AIOps tools analyze large amounts of historical data to identify atypical data points or outliers. These outliers signal and predict problematic events, such as data breaches, allowing businesses to avoid costly consequences like negative press releases, regulatory fines, and declines in consumer confidence.
Performance monitoring
AIOps enhances the visibility and management of modern applications by monitoring cloud infrastructure, virtualization, and storage systems. It reports crucial metrics like usage, availability, and response times, ensuring proactive maintenance and performance optimization. Furthermore, AIOps employs event correlation to consolidate and aggregate data, improving user insight and decision-making capabilities.
Smooth cloud migration
AIOps enhances visibility into the interdependencies of hybrid multi-cloud environments, mitigating operational risks during cloud migration and hybrid cloud adoption. This capability is valuable for organizations gradually adopting cloud services, enabling better management of complexities and ensuring smooth transitions between different cloud environments.
Enhanced DevOps
AIOps enhances DevOps by offering visibility and automation for efficient infrastructure management. It empowers development teams to provision and reconfigure infrastructure while enabling IT to maintain control seamlessly, minimizing additional management efforts.
App development support
DevOps teams leverage AIOps tools to enhance code quality through automated code reviews, implementation of programming best practices, and early detection of bugs in the development lifecycle. This approach shifts quality assurance processes earlier in the development cycle, ensuring issues are addressed promptly before they escalate.
These use cases demonstrate how AIOps can significantly enhance IT operations, making them more proactive, efficient, and aligned with business goals.
AIOps tools
Unlike traditional IT management solutions that struggle with large data volumes and reactive incident handling, AIOps tools offer a transformative approach, effectively replacing traditional ITOps systems. Below, we’ll break down eight AIOps software recommendations that can revolutionize your IT landscape:
1. Moogsoft
Moogsoft is a cloud AIOps platform specializing in incident resolution through machine learning-enabled anomaly detection and IT noise reduction. Its patented technologies focus on providing immediate ROI by enhancing incident remediation. Moogsoft integrates with multiple tools for seamless collaboration, automates incident response to improve MTTR (Mean Time to Restore), and offers early detection to reduce MTTD (Mean Time to Detect). This makes Moogsoft ideal for continuous AIOps availability, ensuring zero downtime for ITOps teams.
2. Splunk
Splunk’s IT service intelligence (ITSI) platform simplifies AIOps for enterprise IT teams, integrating with multiple applications and cloud solutions for comprehensive data analysis. It provides complete visibility into KPIs, allowing for the monitoring of service health and uses predictive analysis of historical data to detect and prevent incidents. Splunk’s capabilities enhance visibility and enable proactive incident prevention, making it a robust solution for enterprise IT environments.
3. BigPanda
BigPanda’s open integration hub processes alert use proprietary machine learning to diagnose IT incidents and determine resolution strategies. Its generative AI creates automatic incident-finding reports, greatly reducing time spent on incident resolution. Additionally, BigPanda automates workflows by allocating tasks to teammates and integrates with other tools to streamline collaboration, making it highly effective for efficient incident diagnosis and resolution.
4. Datadog
Datadog offers comprehensive monitoring for cloud applications, network devices, logs, and databases, with customizable dashboards and AI-powered distributed tracing. It excels in root cause analysis across different data points and provides valuable security insights while filtering alerts to prevent anomalies. Datadog is ideal for in-depth monitoring and security management across diverse IT environments.
5. LogicMonitor
LogicMonitor provides extensive visibility into IT infrastructure, suitable for monitoring multiple servers, databases, and websites and automating various ITOps tasks. It allows for simultaneous monitoring of diverse IT assets and automation for processes like client onboarding, making it a structured ITOps management solution, particularly beneficial for managed service providers (MSPs).
6. New Relic AI
New Relic AI combines AIOps and observability to quickly detect anomalies, identify root causes, and implement remediation plans. It delivers actionable insights into end-user interactions with products and tracks metrics like error rate, web vitals, and app performance. This combination enhances anomaly detection and performance monitoring, ensuring optimal application performance and user experience.
7. PagerDuty
PagerDuty automates alert filtering and minor issue resolution while providing context and historical data for significant incidents, connecting users with experts for rapid response. This capability ensures quick incident response with expert support, boosting productivity and reducing response times, making PagerDuty a vital tool for maintaining IT team efficiency.
8. Dynatrace
Dynatrace is a robust AIOps platform that covers infrastructure monitoring, advanced analytics, security support, and digital experience management. Its application performance monitoring feature provides insights into user behavior and tracks application performance over time. The proprietary AI engine “Davis” leverages predictive, causal, and generative AI to conduct root cause analysis and produce solutions for performance issues, making Dynatrace a comprehensive tool for performance and user experience monitoring across mobile, web, and IoT channels.
Benefits of AIOps
Leveraging AI and advanced analytics can transform IT operations by enhancing efficiency, improving performance, and reducing disruptions. The following section highlights key benefits and improvements achieved by implementing intelligent systems in managing IT infrastructure and services.
- More efficient use of infrastructure and capacity: Through advanced analytics and automation, organizations can optimize resource allocation, ensuring that infrastructure operates efficiently, minimizing waste and reducing costs.
- Prevent problems before customers are impacted: AI-powered anomaly detection systems can identify potential issues early, allowing for proactive interventions that prevent customer-facing disruptions.
- Unify the view of the IT environment: AI and integrated management platforms can provide a comprehensive, unified view of the entire IT landscape, enabling better monitoring, management, and optimization.
- Get insights into what workloads drive costs: Advanced analytics can help identify the most cost-intensive workloads, providing insights for cost optimization and more efficient resource allocation.
- Reduce costly disruptions: Predictive maintenance and automated monitoring can find and address potential issues before they escalate, reducing the frequency and impact of costly disruptions.
- Faster time to deliver new IT services: Automation and intelligent resource management enable quicker deployment of new IT services, enhancing agility and reducing time-to-market.
Why is LeewayHertz your ideal partner for AIOps implementation?
At LeewayHertz, we recognize the significant impact AIOps can have on IT operations. We are committed to using our expertise in AI and machine learning to drive innovation and efficiency in this domain. With a track record of delivering AI-powered solutions across diverse industries, LeewayHertz is a trusted partner for organizations aiming to enhance their IT operations with AIOps.
Here’s why partnering with us for your AIOps initiatives ensures significant advantages:
Expertise in AI and machine learning
We possess extensive AI and machine learning expertise backed by a team of seasoned professionals proficient in advanced technologies. With years of experience creating custom AI solutions across various sectors, we specialize in developing AIOps solutions that seamlessly integrate with existing IT operations frameworks, enhancing efficiency and reliability.
AIOps strategy consulting
Our consultants specialize in integrating advanced AIOps technologies into IT operations frameworks. By leveraging intelligent automation and predictive analytics, we empower organizations to automate complex processes, enhance system performance, and proactively address IT issues before they impact business operations. We work closely with your team to develop customized AIOps strategies that boost operational efficiency and significantly improve system reliability, ensuring your IT operations are responsive, efficient, and aligned with your strategic business goals.
Tailored AIOps solutions
Understanding that every organization has distinct needs, we specialize in developing bespoke AIOps solutions tailored to our client’s unique requirements and goals. Whether it’s implementing AI-driven anomaly detection, predictive maintenance, or automated incident response, we have the expertise to deliver tailored AIOps solutions that drive tangible business outcomes.
End-to-end support
From ideation and conceptualization to development, deployment, and maintenance, LeewayHertz offers comprehensive end-to-end support throughout the AIOps implementation lifecycle. Our dedicated team works closely with clients’ stakeholders to ensure that the integration of the AIOps solution is seamless and performs optimally. This collaboration ensures peace of mind for our clients and maximizes the value derived from the solution.
Commitment to excellence
At LeewayHertz, we are committed to delivering excellence in every project. Our agile development approach and rigorous quality assurance processes ensure that the final solution meets and exceeds clients’ expectations, driving tangible business value and maintaining a competitive edge in the market.
Scalability and flexibility
Understanding the dynamic nature of IT operations, we build AIOps solutions with scalability and flexibility in mind. Whether your organization is a small business or a large enterprise, our solutions can adapt and scale to meet evolving needs and growing user bases, ensuring long-term viability and continued relevance.
Choosing LeewayHertz for AIOps initiatives brings clear business advantages, such as enhanced operational efficiency, cost savings, higher service standards, and improved customer satisfaction. Our AIOps solutions streamline workflows, automate routine tasks, and offer valuable insights, enabling organizations to leverage data for informed decisions and maintain a competitive edge in their industry.
How to implement AIOps in your organization?
Implementing AIOps in your organization requires a strategic approach to ensure successful integration and maximum benefits. Here are the steps to guide you through the process:
Access your current IT environment
- Inventory: Conduct a comprehensive inventory of your IT infrastructure, tools, and processes.
- Identify pain points: Determine areas where IT operations can be enhanced, such as incident management, performance monitoring, and data analysis.
- Evaluate data sources: Understand the data sources available and their quality. Identify gaps that need to be filled for effective AIOps implementation.
Build a strong business case
- Highlight benefits: Outline the benefits of AIOps, such as reduced downtime, improved efficiency, and cost savings.
- Identify ROI: Provide a clear analysis of the return on investment by showing how AIOps can solve specific issues within your organization.
Choose the right AIOps platform
- Research options: Investigate various AIOps platforms, considering ease of use, scalability, integration capabilities, and support services.
- Evaluate features: Identify key features such as real-time monitoring, predictive analytics, automation capabilities, and user-friendly dashboards.
- Test and compare: Arrange demos, request trials, and compare different solutions to find the best fit for your organization.
Address skills and resources
- Upskill your team: Train your IT staff on AIOps tools and methodologies. Consider partnering with providers that offer robust support and training.
- Fill skill gaps: If necessary, hire experts or work with consultants to fill gaps in knowledge in data science, machine learning, and AI.
Develop a detailed implementation plan
- Set goals: Define clear, achievable goals for your AIOps implementation.
- Create a roadmap: Develop a step-by-step roadmap, including timelines, milestones, and responsible teams.
- Pilot program: Start with a pilot program to test the AIOps solution in a controlled environment. Use the program to refine your approach and address any issues.
Integrate AIOps with existing systems
- Seamless integration: Ensure the AIOps platform integrates smoothly with your existing IT systems, tools, and workflows.
- Data integration: Set up processes for aggregating data from various sources into the AIOps platform.
- Automation: Begin automating routine tasks and incident responses based on the insights generated by the AIOps solution.
Monitor and Optimize
- Continuous monitoring: Keep monitoring the performance of the AIOps platform and its impact on your IT operations.
- Feedback loop: Create a feedback loop to collect insights from IT teams and other stakeholders.
- Iterate and improve: Use feedback and performance data to make ongoing improvements to your AIOps implementation.
Foster a culture of collaboration
- Promote collaboration: Encourage collaboration between IT, development, and operations teams to ensure everyone is aligned with the AIOps strategy.
- Transparent communication: Maintain transparent communication about the benefits and progress of AIOps to keep all stakeholders informed and engaged.
Measure success
- KPIs and metrics: Define key performance indicators (KPIs) and metrics to measure the success of your AIOps implementation.
- Regular reviews: Conduct regular reviews to assess progress and make necessary adjustments to achieve your goals.
By following these steps, you can effectively implement AIOps in your organization, driving improved efficiency, faster incident resolution, and better overall IT performance.
Best practices for building AIOps strategy
Here are some best practices for developing an effective AIOps strategy:
- Align AIOps goals with business objectives: Begin by ensuring that your AIOps objectives directly support your organization’s broader business goals, such as improving customer experience, boosting operational efficiency, or managing risks effectively. This alignment is crucial for gaining executive support and measuring ROI effectively.
- Conduct a readiness assessment: Evaluate your IT operations and infrastructure to understand their maturity level and identify gaps that AIOps can address. This assessment helps in selecting the right tools, allocating resources effectively, and planning phased implementations that are targeted and efficient.
- Create a phased implementation roadmap: Develop a structured roadmap with clear phases, milestones, KPIs, and timelines for implementing AIOps. Starting with a pilot phase allows for testing and refining strategies before broader deployment, ensuring that each stage contributes to achieving your strategic objectives. This approach also facilitates data-driven adjustments as your AIOps strategy evolves.
Endnote
AIOps stands at the forefront of modern IT operations management, transforming how organizations harness technology to drive efficiency and innovation. By integrating advanced AI, machine learning, and big data analytics, AIOps empowers businesses to transcend traditional IT management practices. It enables proactive identification and resolution of issues, predictive insights into system performance, and automation of repetitive tasks, which are crucial for maintaining robust operational efficiency.
Adopting AIOps represents more than a technological upgrade; it signifies a strategic shift towards smarter, data-driven decision-making and operational excellence. By streamlining IT operations, reducing downtime, and enhancing service delivery, AIOps improves internal efficiencies and enhances the overall customer experience.
Furthermore, as organizations face escalating IT complexity and the need for rapid digital transformation, AIOps provides a pivotal toolset to navigate these challenges effectively. It allows businesses to leverage vast amounts of data, derive actionable insights, and deploy proactive measures to mitigate risks and capitalize on opportunities in real-time.
Transform your IT operations with LeewayHertz’s cutting-edge AIOps solutions. Contact us today to transform your infrastructure, reduce downtime, and drive unparalleled efficiency.
Start a conversation by filling the form
All information will be kept confidential.
Insights
Adopting AI for customer success: Shaping a new era of user assistance
Customer success is a strategic approach where businesses proactively guide customers through a product journey to ensure they achieve their desired outcomes, thereby enhancing customer satisfaction, loyalty, and advocacy.
AI in portfolio management: Use cases, applications, benefits and development
AI is reshaping portfolio management by offering powerful tools that enhance investment strategies and decision-making.
The role of AI in logistics and supply chain
By harnessing the capabilities of AI, companies can refine their operations and elevate their business performance, leading to enhanced profitability, operational efficiency, and increased customer satisfaction.