AI in retail: Use cases, applications, solution and implementation
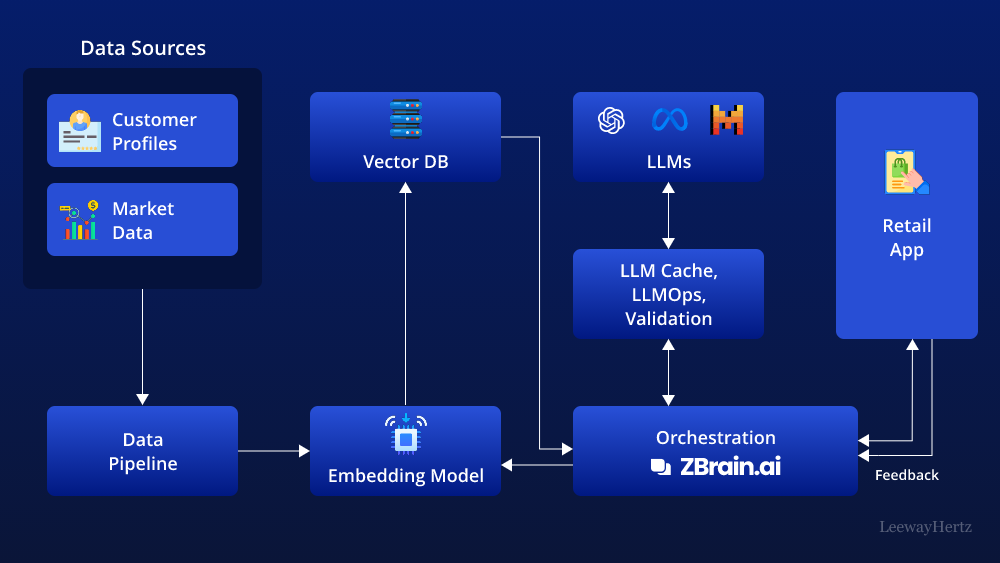
Retailers face various challenges, including managing inventory, meeting changing customer demands, and providing a smooth online and in-store shopping experience. As consumers’ expectations rise, traditional retail strategies often fall short, leading to stock imbalances, inefficient operations, and missed opportunities for personalized engagement. These issues impact profitability, customer satisfaction, and brand loyalty.
Artificial Intelligence (AI) provides an effective solution to many of these challenges. By leveraging advanced technologies, AI enables retailers to tackle critical issues more effectively. For instance, AI can process extensive data to forecast product demand with high accuracy, allowing retailers to manage inventory levels dynamically. This ability helps avoid both stockouts and excess inventory, resulting in more efficient operations and greater customer satisfaction. The adoption of AI in the retail sector is anticipated to surpass $127.09 billion by 2033. Future Market Insights forecasts a robust growth trajectory, with the demand for AI in retail projected to increase by a CAGR of 28% from 2023 to 2033.
AI in retail enhances the shopping experience by delivering personalized recommendations and dynamic pricing based on individual customer behavior and preferences. This improves the relevance of product suggestions and boosts customer engagement and sales. In physical stores, AI tools optimize store layouts and monitor customer behavior to provide valuable insights. These insights help retailers make data-driven decisions to improve store organization and enhance the shopping experience.
By leveraging AI, retailers can overcome key operational challenges, adapt swiftly to market changes, and deliver exceptional service. AI is transforming the retail landscape, making it possible for businesses to stay competitive and meet the evolving needs of their customers. This article explores the landscape of AI in retail, delving into challenges, benefits, and AI applications in retail.
This article explores the landscape of AI in retail, delving into challenges, benefits, use cases, real-world examples, and future trends.
- Understanding AI in retail
- Why is AI crucial for the retail industry?
- How does AI in retail work?
- How can AI address the challenges faced by retail businesses?
- AI applications in retail
- Personalized product recommendations
- Chatbots and virtual assistants
- Visual search
- Sales forecasting
- Customer segmentation
- Fraud detection and prevention
- Price optimization
- Customer lifetime value prediction
- Customer sentiment analysis
- Returns prediction
- Predictive maintenance
- Augmented analytics
- Personalized marketing
- Loyalty programs optimization
- Interactive in-store experiences
- Comprehensive recommendation systems
- Customer service optimization
- Catching shoplifters
- AI use cases across departments within a retail organization
- Merchandising
- Store operations management
- Production management
- Inventory and supply chain
- Logistics department
- Marketing and advertising
- Finance and accounting department
- Loss prevention and security
- Legal and public relations department
- Human Resources (HR) Department
- Customer service department
- E-commerce operations
- Procurement department
- Research and Development (R&D) department
- IT department
- Store development department
- Sustainability initiatives
- Streamlining retail processes using generative AI
- How does LeewayHertz’s enterprise AI solution enhance retail process workflows?
- How to implement AI solutions in retail business?
- LeewayHertz’s AI development services for retail
- Benefits of AI in retail
- Future trends of AI in retail
Understanding AI in retail
AI in retail is a versatile tool that adapts to various business contexts. It harnesses automation, data, and technologies like machine learning (ML) algorithms to create highly personalized shopping experiences for consumers, enhancing both physical and digital store environments.
In the online shopping store, AI-driven chatbots and virtual personal assistants transform the shopping experience. They offer tailored recommendations and dynamic pricing based on a shopper’s behavior, purchase history, and other relevant data points. These AI tools analyze real-time interactions to provide suggestions that not only increase the likelihood of purchase but also significantly enhance the overall shopping experience.
AI applications are equally transformative in physical retail stores. AI can provide insights into shopping behaviors and preferences by utilizing data from customer interactions on mobile devices and in-store sensors. For instance, AI can optimize store layouts by analyzing sales data and other relevant metrics to determine the most effective product placements. This might include predicting the likelihood of a customer purchasing two items together if displayed in proximity. Such insights help retailers enhance product placement strategies and improve sales performance.
AI enables retailers to tailor their offerings more precisely to consumer needs and preferences, driving engagement and boosting online and offline sales.
Why is AI crucial for the retail industry?
Artificial Intelligence (AI) is transforming retail by offering critical advantages that set successful businesses apart. Here is why you should integrate AI into retail:
- Engage customers effectively: With numerous competitors delivering immersive shopping experiences, traditional retailers must offer personalized and engaging interactions across all touchpoints to capture and retain customer interest.
- Enhance the shopping experience: To sustain customer interest and loyalty, retailers must differentiate themselves by offering unique products and compelling experiences. With the help of predictive analytics, AI enables retailers to keep up with trends, stay ahead, and innovate proactively.
- Transform data into insights:Retailers face a deluge of data from various sources, including supply chains, stores, and customer interactions. AI helps distill this information into actionable insights, allowing retailers to craft strategies focused on consumer needs.
- Unifying online and offline channels: Managing digital and physical shopping channels separately can create customer friction and inefficiencies. AI helps unify these channels to provide a seamless shopping experience and streamline operational processes.
- Adapt logistics to changing demands: Traditional supply chains may struggle as consumer preferences shift from mainstream to niche products. AI facilitates the creation of flexible logistics networks that can quickly adapt to evolving customer behaviors.
How does AI in retail work?
Incorporating AI into retail management transforms how retail businesses analyze data, generate insights, and make decisions. By leveraging powerful large language models (LLMs) and integrating them with the business’ unique knowledge base, retailers can go beyond traditional retail management processes. This advanced approach unlocks new levels of insight generation, empowering businesses to make data-driven decisions in real-time.
This architecture streamlines retail management by integrating various components to optimize data analysis and decision-making. Here’s a step-by-step breakdown of how it works:
1. Data sources: The process starts with gathering data from multiple sources relevant to retail management:
- Customer profiles: Detailed information on customer demographics, purchasing behavior, preferences, and transaction history.
- Market data: Historical and real-time data on sales trends, product prices, and market conditions.
- Inventory data: Information on stock levels, product turnover rates, and supply chain logistics.
- Sales reports: Analysis of sales performance, promotional effectiveness, and market trends from internal and external reports.
- Supplier information: Data on supplier performance, pricing, and delivery schedules.
2. Data pipelines: The gathered data is processed through pipelines that handle ingestion, cleaning, and structuring of data, preparing it for detailed analysis.
3. Embedding model: An embedding model transforms the cleaned data into numerical representations (vectors), making it understandable for AI models. Common embedding models include those from OpenAI, Google, and Cohere.
4. Vector database: The vectors are stored in a vector database, allowing efficient querying and retrieval. Prominent vector databases include Pinecone, Weaviate, and PGvector.
5. APIs and plugins: APIs and plugins such as Serp, Zapier, and Wolfram connect various components, enabling functionalities like accessing additional data or performing specific operations.
6. Orchestration layer: The orchestrating layer is essential for managing the entire workflow in the architecture. ZBrain is an example of this layer that serves this role by streamlining the sequence of tasks, managing interactions with external APIs to determine when calls are needed, retrieving relevant data from vector databases, and maintaining context across multiple AI interactions. This layer ultimately crafts and organizes prompts for submission to the language model, ensuring a smooth and efficient process for generating insights and recommendations.
7. Query execution: When a user submits a query to the retail management app, the process of data retrieval and generation gets triggered. The query could involve aspects like inventory levels, sales trends, or customer insights.
8. LLM processing: The query is then sent to the orchestration layer, which retrieves relevant data from the vector database and LLM Cache and passes it to the appropriate LLM for processing. The choice of LLM depends on the query’s nature.
9. Output: The LLM produces an output based on the query and the data. The output can be summaries of sales performance, identification of customer trends, or reports on inventory status.
10. Retail management app: The validated output is presented to users through the retail management app. This app serves as a user-friendly interface that delivers the LLM findings to the decision-makers.
11. Feedback loop: User feedback on the LLM’s output helps refine and improve accuracy over time.
12. Agent: AI agents can address complex problems, interact with external systems, and enhance learning through advanced reasoning and planning, memory, recursion, and self-reflection.
13. LLM cache: Tools like Redis, SQLite, or GPTCache are used to cache frequently accessed information to speed up response times.
14. Logging/LLMOps: LLM operations (LLMOps) tools such as Weights & Biases, MLflow, Helicone, and prompt layer help log actions and monitor performance, ensuring optimal functionality and continuous improvement.
15. Validation: A validation layer ensures the accuracy and reliability of the LLM’s output using tools like Guardrails, Rebuff, Guidance, and LMQL.
16. LLM APIs and hosting: LLM APIs and hosting platforms are essential for executing retail management tasks and hosting the app. Based on the project’s needs, developers can choose from LLM APIs offered by OpenAI and Anthropic or open-source models and select hosting platforms from cloud providers like AWS, GCP and Azure or specialized clouds like Databricks, Mosaic, and Anyscale.
This structured approach showcases how AI optimizes retail management. It leverages diverse data sources and technological tools to deliver precise and actionable insights, ultimately enhancing efficiency and decision-making within the retail sector.
How can AI address the challenges faced by retail businesses?
Retailers face various challenges across their operational workflows. AI can help address many of these challenges by providing insights, automating tasks, and improving overall efficiency. Here’s how AI can address these challenges:
Procurement and inventory management
Challenge: Accurately predicting demand is challenging, often causing overstock or stockouts that tie up capital or result in lost sales. Traditional forecasting methods struggle with dynamic factors like seasonality, market trends, and sudden shifts in consumer behavior.
AI solution: Machine learning algorithms analyze vast amounts of historical sales data, seasonal patterns, and external influences like market conditions and social trends. By continuously learning and adapting to new data, these algorithms provide more accurate demand forecasts, allowing businesses to optimize inventory levels, reduce carrying costs, and avoid stockouts.
Receiving and inspection
Challenge: Human error can lead to overlooked defects or discrepancies in manual verification and inspection of received goods. This can result in the acceptance of faulty products, which impacts quality control and customer satisfaction.
AI solution: Computer vision systems can help automate the inspection process by using advanced image recognition to quickly and accurately identify defects or inconsistencies in products. These systems can also compare received goods against expected standards, reducing the reliance on manual checks and minimizing errors.
Storage and warehousing
Challenge: Inefficient picking and storing processes can lead to wasted time, increased labor costs, and delays in order fulfillment. Manual methods struggle with optimizing storage layouts and efficiently navigating complex inventories.
AI solution: AI-driven automation systems improve warehouse efficiency by optimizing product placement based on frequency of access and other factors. Automated Guided Vehicles (AGVs) and robotic picking systems streamline the picking process, reducing human error and speeding up order fulfillment.
Pricing
Challenge: Dynamically adjusting prices as per market conditions is challenging without real-time data analysis. Static pricing strategies can lead to lost revenue opportunities or uncompetitive pricing.
AI solution: AI algorithms can analyze real-time market data, including competitor pricing, supply and demand fluctuations, and customer purchasing behavior. These insights allow businesses to adjust prices dynamically, maximizing profit margins and maintaining competitive positioning in the market.
Visual merchandising
Challenge: Optimizing product placement to maximize customer engagement and sales is difficult without precise insights into customer behavior. Poorly arranged displays can lead to lower sales and a suboptimal shopping experience.
AI solution: AI-powered analytics can track and analyze customer movement and interactions within a store. Retailers can analyze which areas of the store attract the most attention and identify the products that generate the highest engagement. Understanding which products generate the highest engagement helps in arranging them strategically. This approach enhances the shopping experience and boosts sales.
Sales transactions
Challenge: Manual sales transactions can result in long wait times and potential errors, frustrating customers and reducing overall efficiency. Handling peak times efficiently is especially challenging.
AI solution: Automated checkout systems, such as self-checkout kiosks and AI-powered point-of-sale (POS) systems, streamline the transaction process. These systems cut down on wait times, decrease human errors, and improve the customer experience by delivering quicker and more precise service.
Customer service
Challenge: Ensuring uniform and top-notch customer service can be difficult during busy periods or when managing a large influx of inquiries. Consistent responses can lead to customer satisfaction.
AI Solution: AI-powered chatbots and virtual assistants can handle routine customer inquiries and provide instant responses. This allows teams to concentrate on more complex matters, ensuring customers get prompt and precise support while preserving a high standard of service.
Payment processing
Challenge: Securing payment transactions is crucial to prevent fraud and unauthorized activities. Failure to do so can lead to significant financial losses and damage to the company’s reputation.
AI solution: AI-powered fraud detection systems scrutinize transaction patterns and identify irregularities that may suggest fraudulent activity. These systems can flag suspicious activities in real time, allowing businesses to prevent fraudulent transactions and enhance the overall security of payment processing.
E-commerce operations
Challenge: Handling and processing a high volume of online orders efficiently can be challenging, often resulting in delays and mistakes in fulfilling orders. High customer expectations for fast delivery add to the pressure.
AI solution: AI-driven order processing systems automate the processing of online orders, optimizing workflows and reducing fulfillment times. These systems can prioritize orders, manage inventory in real-time, and streamline logistics to ensure timely delivery, enhancing overall efficiency and customer satisfaction.
Promotions and marketing
Challenge: It is difficult to effectively target promotions to the right audience without thorough insights into customer preferences and behavior. Ineffective promotions can lead to wasted marketing spend and missed revenue opportunities.
AI solution: AI algorithms examine customer data to uncover patterns and preferences, allowing for the creation of personalized marketing campaigns. By focusing on specific client segments with customized promotions, businesses can boost engagement and conversion rates, enhancing the effectiveness of their marketing strategies.
Analytics and reporting
Challenge: Manually analyzing sales performance data is time-consuming and can delay decision-making. With real-time insights, businesses may take advantage of opportunities to respond promptly to trends or issues.
AI solution: AI analytics tools automate the data analysis, providing real-time insights into sales performance and trends. These tools create comprehensive reports and dashboards, enabling businesses to make prompt, informed decisions and remain responsive to market changes.
Restocking and reordering
Challenge: Optimizing restocking schedules and order quantities is complex, as inaccurate forecasts can lead to stockouts or excess inventory. Traditional methods may adapt slowly to changing demand.
AI solution: AI-driven inventory management systems use demand forecasts to optimize restocking decisions. By more accurately predicting future needs, these systems help reduce stockouts and minimize excess inventory, ensuring that products are available when needed without tying up unnecessary capital.
Staff management
Challenge: Inefficient staff scheduling and resource allocation can lead to understaffing or overstaffing, affecting customer service and operational efficiency. Manually managing staff schedules is often cumbersome.
AI solution: AI algorithms optimize staff scheduling by analyzing historical sales data and predicted foot traffic. These algorithms ensure adequate coverage during peak times while avoiding overstaffing during slower periods, improving overall resource allocation and staff productivity.
End-of-day procedures
Challenge: Manual reconciliation and closing processes are time-consuming and prone to errors. They affect the accuracy of financial records and increase the staff’s workload.
AI solution: Automated end-of-day processes, such as cash register reconciliation and inventory checks, streamline closing procedures. AI systems handle these tasks with precision and speed, minimizing errors and allowing staff to concentrate on other essential activities.
Feedback and improvement
Challenge: Gathering and analyzing customer feedback at scale is difficult, as traditional methods may not capture the full scope of customer sentiment or actionable insights.
AI solution: AI-driven sentiment analysis tools analyze extensive amounts of customer feedback from diverse sources like reviews and social media. They offer valuable insights into customer sentiment and identify areas needing improvement, aiding businesses in making informed decisions to enhance products and services.
Optimize Your Operations With AI Agents
Optimize your workflows with ZBrain AI agents that automate tasks and empower smarter, data-driven decisions.
AI applications in retail
AI is significantly impacting the retail industry, providing businesses with advanced tools and applications to enhance operations, improve efficiency, and elevate the customer experience. We will explore some of the most significant AI applications in retail:
Personalized product recommendations
A personalized shopping experience involves leveraging AI and customer data to tailor individual shoppers’ interactions, recommendations, and content. Businesses analyze factors like browsing history, purchase behavior, and preferences to create custom product suggestions, targeted offers, and dynamic website content. Collaborative filtering, content-based filtering, and hybrid approaches are used to suggest products similar to those customers have shown interest in or purchased before. This enhances customer engagement and increases conversion rates.
Chatbots and virtual assistants
In retail, chatbots and virtual assistants facilitate tasks like product recommendations, order tracking, and issue resolution, providing efficient and personalized customer engagement while enhancing operational scalability. AI-powered chatbots and virtual assistants provide instant customer support, promptly address inquiries, and assist with purchasing decisions; their utilization of Natural Language Processing (NLP) enhances their capability to understand and respond to customers’ inquiries, thus improving customer satisfaction and reducing the need for human intervention. As per Forbes, chatbots boost sales by 67%.
Visual search
Visual search is one of the most significant AI applications in retail that enables customers to search for products using images. When a user uploads a photo or uses their device’s camera to capture an item, AI-powered algorithms analyze the image to identify key features and attributes. The system then matches these visual cues with items in the retailer’s catalog, presenting the user with visually similar products. This technology enhances the shopping experience by simplifying the search process, allowing customers to find products more easily and quickly, especially when they have a specific item or style in mind but struggle to describe it in text. Visual search can also help retailers reduce bounce rates and increase conversion rates by minimizing the friction in the product discovery process, ultimately driving sales and customer satisfaction.
Sales forecasting
Accurate sales forecasting is a crucial aspect of the retail industry. With the constantly evolving product offerings and shifting customer preferences, businesses face the challenge of predicting demand. Leveraging the power of AI, sales forecasting becomes more effective. AI-driven sales forecasting enables companies to analyze historical, current, and projected sales data. It aids in anticipating shifts in customer demand and monitoring market changes that may affect sales. This predictive capability enhances the accuracy of demand prediction, ensuring businesses have the right products available when needed. As a result, customer satisfaction is improved, operational efficiency is optimized, and sales are maximized.
Customer segmentation
Customer segmentation, a pivotal AI-driven strategy in retail, involves using machine learning and data analysis techniques to categorize customers into distinct groups based on their behaviors, preferences, demographics, and purchase history. AI algorithms analyze vast datasets to identify customer patterns and similarities, allowing retailers to create targeted marketing campaigns, personalized product recommendations, and tailored promotions for each segment. This approach enhances the shopping experience, boosts customer engagement, and increases conversion rates by delivering content and offers that align with the specific preferences of each customer group. Customer segmentation also aids in inventory management and product development, as it provides insights into which products and features resonate most with different customer segments, ultimately driving revenue growth and customer satisfaction.
Fraud detection and prevention
Fraud detection is a critical AI application in retail that employs advanced algorithms to analyze transaction data and identify potentially fraudulent activities. This process involves the continuous monitoring of payment transactions, customer behaviors, and patterns to detect anomalies and potential signs of fraudulent behavior, such as unusual purchasing patterns, high-risk IP addresses, or mismatched card information. AI-driven fraud detection systems use machine learning and pattern recognition techniques to adapt and evolve their fraud detection models, becoming more effective at recognizing new and emerging fraud tactics. By promptly flagging suspicious transactions, retailers’ platforms can mitigate financial losses, reduce chargebacks, protect customers, and maintain the integrity and trustworthiness of their online operations.
Price optimization
Price optimization involves leveraging advanced AI algorithms and data analytics to determine the most effective pricing strategy for products or services. It entails analyzing various factors such as historical sales data, market demand, competitor pricing, customer behavior, and economic conditions to set prices that maximize profitability while remaining competitive. AI algorithms can continuously adjust prices in real-time, considering dynamic factors, and even personalize pricing for individual customers on the basis of their preferences and buying history. This not only enhances revenue but also improves customer satisfaction and loyalty by offering fair and attractive pricing, making it a crucial tool for retailers to remain competitive in today’s dynamic market landscape.
Customer lifetime value prediction
Customer Lifetime Value (CLV) prediction is a pivotal AI use case in retail that harnesses data-driven insights to forecast the future value a customer is expected to generate over their entire relationship with a business. AI models use machine learning techniques like Recurrent Neural Networks (RNNs) or Gradient Boosting Machines (GBMs) to analyze historical customer data, including purchase history, browsing behavior, demographic information, and interaction patterns. These algorithms then generate CLV predictions, enabling businesses to make informed decisions regarding customer segmentation, personalized marketing strategies, and resource allocation to maximize long-term profitability, enhance customer engagement, and foster loyalty.
Customer sentiment analysis
Customer Sentiment Analysis is a crucial use case of AI in retail, employing Natural Language Processing (NLP) techniques, such as Recurrent Neural Networks (RNNs) and Transformers like BERT or GPT, to analyze and understand customer opinions and emotions expressed in reviews, social media posts, and feedback. By evaluating the sentiment, AI systems have the capability to categorize customer feedback as positive, negative, or neutral and even extract specific aspects like product quality or customer service satisfaction. This deep insight into customer sentiment helps businesses make data-driven decisions, improve product offerings, enhance customer experiences, and respond effectively to issues, ultimately driving higher customer satisfaction and loyalty.
Returns prediction
Returns prediction, a strategic AI application in retail, foreseeing which products customers will likely return. AI algorithms scrutinize historical data, customer behaviors, and product characteristics to identify patterns that signal potential return risks. This insight empowers retailers to proactively address quality issues, adjust return policies, and optimize inventory management. It’s like having a predictive navigator helping businesses with returns management. Retailers can enhance customer satisfaction, reduce operational costs, and boost overall profitability by reducing return rates and efficiently handling product returns.
Predictive maintenance
Predictive maintenance, a powerful use case of AI in retail, employs ML algorithms such as Support Vector Machines (SVM), random forests or Convolutional Neural Networks (CNNs) to anticipate and prevent equipment and infrastructure failures. By analyzing historical data from sensors, devices, and machinery, AI models can predict when equipment will likely malfunction or require maintenance, allowing retailers to schedule proactive repairs, reduce downtime, minimize operational costs, and ensure seamless supply chain and logistics operations. This enhances operational efficiency and elevates customer satisfaction by preventing disruptions in the retail processes.
Augmented analytics
AI-powered analytics tools are pivotal in transforming raw data into actionable insights for retailers. By leveraging sophisticated algorithms and machine learning, these tools analyze vast datasets to uncover patterns, trends, and customer behaviors. Retailers can then make informed decisions regarding marketing strategies and product assortments. The real-time nature of this analytics process allows businesses to adapt swiftly to market changes, optimizing their promotional efforts and ensuring that product offerings align with current demand. As a result, the overall business performance is significantly enhanced, leading to increased efficiency, better-targeted marketing campaigns, and a more competitive edge in the dynamic retail landscape. In essence, AI-powered analytics grants retailers the capability to navigate the intricate landscape of the market with precision and agility, cultivating sustained growth and enhancing customer satisfaction.
Personalized marketing
AI’s analysis of customer behavior and preferences is the cornerstone for retailers to execute highly targeted advertising campaigns. By delving into intricate patterns of customer interactions and transaction histories, AI identifies individual preferences, allowing for the creation of personalized marketing strategies. Tailoring promotional activities to match specific customer profiles enhances the relevance of advertisements and boosts their overall effectiveness. This personalized approach facilitates a deeper connection between the retailer and the customer, as the advertisements align more closely with the individual’s interests and needs. Consequently, improved customer engagement becomes evident, as consumers may respond positively to messages that resonate with their preferences.
Loyalty programs optimization
AI empowers retailers to anticipate and understand customer preferences and behaviors by leveraging the power of predictive analytics. This proactive insight becomes a cornerstone for optimizing loyalty programs and rewards on an individualized level. AI can analyze vast datasets to identify trends and patterns, enabling retailers to tailor loyalty incentives that resonate with each customer. The personalized nature of these incentives enhances customer satisfaction, as individuals feel recognized and valued. Rather than employing a one-size-fits-all approach, retailers can offer rewards that align precisely with a customer’s preferences and shopping habits. This level of customization not only fosters stronger loyalty but also contributes to a more positive overall customer experience.
Interactive in-store experiences
Integrating AI-driven interactive displays and kiosks within physical stores marks a transformative enhancement to the retail landscape. These technologies leverage artificial intelligence to deliver personalized information about products, creating a dynamic and immersive shopping experience for customers. By tailoring information based on individual preferences, purchase history, and real-time interactions, these displays captivate customers’ attention and cater to their specific interests. Beyond elevating the in-store experience, these interactive solutions facilitate informed decision-making. Customers can explore detailed product information, compare features, and even receive personalized recommendations, empowering them with the knowledge to make confident purchasing decisions. This enhances customer satisfaction and contributes to increased sales by aligning product offerings more closely with individual preferences.
Comprehensive recommendation systems
The reach of AI recommendation systems extends beyond mere product suggestions, encompassing a broader spectrum of content like blog posts, articles, and videos. By leveraging machine learning algorithms, AI discerns individual preferences and browsing patterns to curate a personalized content journey for each customer. The ability of AI to understand and anticipate a customer’s interests enables retailers to provide content that resonates on a personal level. These tailored recommendations contribute to a more immersive and educational shopping environment, whether it’s informative articles related to product usage, video demonstrations, or relevant blog posts.
Customer service optimization
By integrating AI tools with sentiment analysis capabilities into customer service operations, retailers gain a powerful tool for understanding and responding to customer emotions. These tools analyze the sentiment behind customer interactions, whether positive, negative, or neutral, providing valuable insights into customer satisfaction levels. This proactive approach enables retailers to address issues in real time, as they can swiftly identify and respond to customer concerns. Whether it’s resolving a problem with a product, addressing service issues, or providing additional assistance, retailers can enhance customer service by taking prompt and informed actions.
Catching shoplifters
Catching shoplifters is a critical concern, with retail inventory shrinkage due to theft and other criminal activities recently making headlines. The National Retail Federation reported that the average shrink rate increased to 1.6% in FY 2022, up from 1.4% in 2021. When taken as a percent, this represents over $112 billion in losses—a significant issue for retailers.
AI tools offer effective solutions to address this problem in physical stores. For instance, during self-checkout, object detection and motion analytics can help prevent theft in near real-time. This technology enables the detection of shoplifters as they attempt to bypass secure scanning systems.
These use cases of AI in retail demonstrate the versatility and impact of artificial intelligence across various aspects of the industry, from improving customer interactions to optimizing supply chain operations and boosting profitability.
Optimize Your Operations With AI Agents
Optimize your workflows with ZBrain AI agents that automate tasks and empower smarter, data-driven decisions.
AI use cases across departments within a retail organization
AI is reshaping various departments of retail, leading to increased efficiency, improved profitability, and heightened security measures. Let’s explore department-wise AI use cases in the retail industry:
Merchandising
AI is a game-changer in merchandising, redefining product selection and inventory management. AI-driven demand forecasting, fueled by historical sales data and external factors, accurately predicts trends and consumer preferences. This empowers retailers to optimize product assortments, minimizing the risks of overstock or stockouts. Moreover, dynamic pricing algorithms, guided by AI, adapt prices in real-time, responding to market conditions, competition, and demand, ensuring optimal pricing strategies. Through AI, merchandisers can precisely tailor their product offerings and pricing strategies, enhancing sales and profitability.
Store operations management
Within store operations management, AI is a silent efficiency booster. AI-driven inventory management systems continuously monitor stock levels, helping streamline restocking processes and reduce costly stockouts or overstock situations. Energy efficiency is enhanced through AI-controlled systems that optimize lighting, heating, and cooling in real time, leading to reduced operational costs. Moreover, AI-driven predictive maintenance ensures that equipment and assets are well-maintained, minimizing downtime and ensuring a smooth store operation. Overall, AI empowers store managers with data-driven insights to enhance efficiency, reduce operational expenses, and deliver an improved shopping experience to customers.
Production management
Efficiency and product quality reach new heights in production management, thanks to AI. AI-powered computer vision systems, utilizing techniques like convolutional neural networks (CNNs), inspect products for defects with remarkable accuracy, reducing wastage and ensuring consistent quality. AI-driven predictive maintenance uses machine learning algorithms to analyze equipment sensor data, enabling proactive servicing and minimizing downtime. Moreover, AI optimization algorithms optimize production schedules, resource allocation, and workflow, improving overall production efficiency. By integrating AI techniques, production managers can optimize operations, maintain product quality, and reduce costs, ultimately leading to increased productivity and profitability.
Inventory and supply chain
In the inventory and supply chain department, retailers face the constant challenge of maintaining an optimal balance between inventory levels and customer demand. Predicting stock requirements while avoiding overstock or stockouts can be daunting. AI is the game-changer, employing cutting-edge techniques like ML and deep learning to provide real-time visibility into the complex supply chain ecosystem. It excels in demand forecasting using precision algorithms like ARIMA or LSTM, minimizing excess inventory and preventing stockouts. Additionally, AI-driven route optimization via algorithms such as genetic algorithms and reinforcement learning minimizes transportation costs and delivery times. AI’s efficient inventory management further reduces carrying costs while ensuring products are available when and where customers need them. AI’s transformative power enhances inventory and supply chain management, offering innovative solutions to long-standing challenges and propelling retail operations to new heights of efficiency and competitiveness.
Logistics department
In the world of retail logistics, AI is driving efficiency and cost savings through innovative techniques and algorithms. AI-driven predictive maintenance, utilizing machine learning algorithms, monitors the condition of vehicles and equipment, reducing unplanned downtime and maintenance costs. Route optimization, powered by algorithms like genetic algorithms or reinforcement learning, minimizes transportation costs, fuel consumption, and delivery times. Real-time tracking and monitoring, enabled by AI, provide better visibility into the movement of goods and assets. Additionally, AI-enhanced risk management identifies potential challenges within the supply chain, such as delays or interruptions, allowing for proactive mitigation. By leveraging these AI techniques, the logistics department can streamline operations, enhance customer satisfaction, and optimize resource utilization, ultimately contributing to the retail sector’s cost-effective and efficient logistics processes.
Marketing and advertising
AI is a powerful tool for creating highly targeted and effective campaigns in the marketing and advertising department. AI utilizes techniques like natural language processing (NLP) and ML to analyze vast amounts of customer data, social media sentiment, and historical campaign performance. This data-driven approach allows for the creation of hyper-personalized marketing strategies that cater to individual customer preferences. AI also optimizes ad placement and bidding strategies in real-time, using algorithms like reinforcement learning to maximize ad spend efficiency and campaign ROI. Moreover, AI-powered chatbots and conversational AI enhance customer engagement by providing instant responses and personalized recommendations.
Finance and accounting department
AI is a vital ally for streamlining processes, reducing errors, and improving financial decision-making in the finance and accounting departments. AI-driven automation, often using Robotic Process Automation (RPA), takes care of repetitive tasks like data entry, invoice processing, and reconciliation, increasing efficiency and accuracy. Machine learning algorithms analyze financial data to identify anomalies and patterns, enhancing fraud detection and risk management. Predictive analytics, incorporating techniques such as regression analysis, helps forecast financial trends and optimize budget allocation. Additionally, AI supports expense tracking, budget management, and financial reporting, providing real-time insights for better financial control and strategy formulation. By leveraging these AI techniques, the finance and accounting department can operate more efficiently, reduce operational costs, and make data-driven decisions, ultimately contributing to better financial health for the organization.
Loss prevention and security
In the domain of retail, the loss prevention and security department’s top priority is safeguarding assets and preventing theft or security breaches. AI-powered video analytics, utilizing techniques like object detection and facial recognition, analyze surveillance footage in real-time, detecting suspicious activities and potential threats. These systems can trigger alerts to security personnel, enabling quick responses to security incidents. Moreover, AI-driven algorithms analyze patterns in inventory data to identify irregularities and potential cases of shrinkage or fraud. Predictive analytics and anomaly detection algorithms help in proactively identifying vulnerabilities or areas of concern within the security infrastructure. By harnessing AI, the loss prevention and security department enhances surveillance capabilities, reduces losses, and fortifies security measures, ultimately safeguarding the organization’s assets and preserving its reputation in the retail landscape.
Legal and public relations department
Streamlining processes, managing legal documents, and shaping public perception are critical tasks within the legal and public relations department in the retail sector. AI-driven contract review systems, utilizing natural language processing (NLP) algorithms, assist legal teams by quickly analyzing and identifying key clauses and potential issues within contracts, saving time and reducing human error. Sentiment analysis powered by AI monitors social media and news sources, providing real-time insights into public sentiment and helping shape PR strategies and crisis management responses. Additionally, AI-driven e-discovery tools assist in sifting through vast amounts of legal documents and data to uncover critical information for legal cases in the fast-paced retail environment. By harnessing AI techniques, the legal and public relations department enhances efficiency in legal operations, gains deeper insights into public sentiment, and ensures effective communication with the public and stakeholders.
Human Resources (HR) Department
AI can streamline recruitment by automating resume screening and using predictive analytics to identify the best candidates. Chatbots can assist in answering queries from employees and facilitating onboarding processes. Additionally, AI can help manage the workforce, analyze employee performance, and predict and address any forthcoming HR issues.
Customer service department
AI can enhance customer service by powering virtual assistants and chatbots that provide immediate responses to customer inquiries. Additionally, AI can analyze customer feedback and communication to identify areas for improvement, develop better communication strategies, and enhance overall customer experience.
E-commerce operations
AI can be utilized to enhance user experience on e-commerce platforms through personalized recommendations, chatbots for instant customer interaction, and automated systems for efficient order management. Moreover, it can help in fraud detection by analyzing customer behavior to identify and prevent potential fraudulent activities.
Procurement department
AI can optimize procurement processes by analyzing data to identify the best suppliers, negotiate optimal terms, and streamline purchase processes. Moreover, AI can predict when new stock will be needed and automate ordering processes, ensuring consistency and efficiency in procurement operations.
Research and Development (R&D) department
AI can assist in analyzing market trends, customer preferences, and competitive products to guide the development of new products or improve existing ones. Machine learning algorithms can predict which product features or innovations might resonate with consumers in future offerings.
IT department
AI can enhance cybersecurity, providing predictive analytics to identify and mitigate potential threats. It can also automate routine IT tasks and support IT operations in managing the network, system, and data efficiently, ensuring the tech infrastructure operates smoothly.
Store development department
For retailers that have physical stores, AI can assist in analyzing geographical and demographic data to identify optimal locations for new stores. Furthermore, it can predict which areas are likely to experience growth or decline, ensuring strategic store development.
Sustainability initiatives
AI can aid in developing and monitoring sustainability initiatives by analyzing data related to energy usage, waste production, and supply chain operations. This will help retailers to identify areas where they can reduce their carbon footprint and implement more environmentally friendly practices.
In all these departments, AI technologies offer the possibility to leverage data for making informed decisions, automating repetitive tasks, and enhancing the overall functionality and efficiency of operations in the retail sector. It can address unique challenges faced by each department and contribute to optimizing performance across the entire retail enterprise.
Streamlining retail processes with generative AI
Generative AI is transforming the retail industry, streamlining operations, enhancing workflows, and improving decision-making. This sub-section highlights the pivotal role and the impact of generative AI across various personas involved in retail processes:
- Sales representatives manage customer relationships, track sales progress, and leverage AI for lead qualification within the Sales Automation application.
- Sales managers oversee team performance, using AI tools to monitor activities, validate deals, and provide targeted coaching to enhance sales outcomes.
- Sales support executives utilize generative AI tools to support the sales framework, optimizing competitor analysis and territory management and ensuring the efficiency and daily functionality of sales applications.
- Partner sellers and managers collaborate on managing sales pipelines and client engagements, integrating AI to improve partner relationships and sales strategies.
The following section covers crucial processes involved in sales and how generative AI is influencing each step within these processes:
1. Insight gathering and market analysis
Steps involved | Sub-steps | Role of generative AI |
---|---|---|
Gather information |
|
|
Data analysis |
|
|
Identify market needs |
|
|
Guide product development |
|
|
Dynamic pricing and inventory management |
|
|
2. Customer segmentation and marketing
Steps involved | Sub-steps | Role of generative AI |
---|---|---|
Segmentation |
|
|
Targeting |
|
|
Execution and performance monitoring |
|
|
3. Sales and opportunity development
Steps involved | Sub-steps | Role of generative AI |
---|---|---|
Lead management |
|
|
Opportunity management |
|
|
Proposal development |
|
|
Negotiation and closure |
|
|
4. Order processing and post-transaction support
Steps involved | Sub-steps | Role of generative AI |
---|---|---|
Order processing |
|
|
Post-support |
|
|
Customer feedback |
|
|
5. Performance analysis and continuous improvement
Steps involved | Sub-steps | Role of generative AI |
---|---|---|
Conduct performance analysis |
|
|
Strategy development |
|
|
Evaluate impact |
|
|
Conclude analysis |
|
|
Optimize Your Operations With AI Agents
Optimize your workflows with ZBrain AI agents that automate tasks and empower smarter, data-driven decisions.
How does LeewayHertz’s enterprise AI solution enhance retail process workflows?
LeewayHertz’s generative AI platform, ZBrain, serves as a powerful tool for retail enterprises looking to optimize their operations and enhance customer experiences. ZBrain enables the development of customized applications based on advanced large language models like GPT-4, Vicuna, Llama 2, and GPT-NeoX. These applications are designed to address specific challenges in the retail sector, providing businesses with the tools to streamline workflows, improve customer interactions, and boost overall operational efficiency. In the realm of retail, challenges such as inventory management complexities, dynamic customer preferences, and the need for personalized shopping experiences are prevalent. ZBrain effectively addresses these challenges through its specialized Flow feature, offering an intuitive interface for seamless business logic creation for apps without the need for coding skills. Flow enables you to integrate various prompt templates, large language models, and other generative AI models into your app’s logic with its user-friendly drag-and-drop interface. With Flow, you can develop and modify sophisticated and intelligent applications tailored to your business’s specific needs. This results in highly efficient and error-resistant retail operations, presenting holistic solutions to key challenges faced in the retail industry. Explore the following section, illustrating how ZBrain transforms inventory management and customer engagement in the retail industry, elevating overall operational efficiency and fostering a seamless shopping experience.
AI-driven next-best action planning in retail
ZBrain redefines next-best action planning in retail by automating the aggregation of customer data, including purchase history and social media behaviors. Through an automated Exploratory Data Analysis (EDA), ZBrain unveils valuable patterns, enabling informed decision-making based on customer interactions. ZBrain redefines the next-best action planning for retailers, addressing the intricate challenge of effectively engaging customers in the dynamic retail landscape. In the realm of retail, identifying the optimum next steps, be it personalized product recommendations or targeted offers, significantly influences customer satisfaction, loyalty, and overall business performance. ZBrain simplifies this process by seamlessly aggregating customer data from various sources, including purchase history, wishlists, social media interactions, and past promotion responses. Through an automated Exploratory Data Analysis (EDA), ZBrain uncovers patterns and anomalies, extracting vital insights into customer behaviors and preferences. When a retailer seeks guidance for the next best action, ZBrain leverages an LLM to process relevant data and formulate actionable recommendations. Explore this Flow to understand how ZBrain seamlessly orchestrates next-best-action planning in retail.
AI-driven in-store replenishment strategies
ZBrain redefines in-store replenishment strategies, a critical element for retail success. Maintaining consistently stocked shelves without overstocking is a delicate balance, compounded by the challenges of fluctuating consumer demand, supply chain disruptions, and seasonal variations. ZBrain rises to this challenge, streamlining the replenishment process with its automated approach. The platform aggregates vital data, including stock levels, promotion details, customer purchase patterns, and product demand data from diverse sources, subjecting them to a comprehensive Exploratory Data Analysis (EDA). This analysis rectifies anomalies, revealing stock movement patterns, sales trends, and potential replenishment needs. ZBrain’s unparalleled automation minimizes stockouts, elevates customer satisfaction, and optimizes inventory levels, allowing retailers to address stock needs swiftly, increase sales, and maintain optimal inventory turns. To gain a comprehensive understanding of how ZBrain enhances in-store replenishment strategies, explore through this informative Flow.
AI-driven customer retention strategies
ZBrain redefines customer retention strategies, addressing the crucial need for sustained customer loyalty in the competitive retail landscape. The platform aggregates pertinent customer data, encompassing purchase histories, reviews, interactions, and social media engagements from diverse sources. Following this data compilation, an automated exploratory data analysis delves into deeper insights, unraveling customer behaviors, preferences, and potential issues. ZBrain employs embedding techniques to transform textual data into numerical representations, capturing sentiments and patterns that inform precise insights and trends. By automating churn prevention and customer retention, ZBrain significantly reduces the time and effort traditionally required, enabling retailers to comprehend customer needs and preferences for more effective strategies swiftly. Explore the intricate Flow to comprehend how ZBrain redefines customer retention strategies, offering actionable insights derived from comprehensive data analysis.
AI-driven customer offer targeting
ZBrain reshapes customer offer targeting in the retail domain, recognizing the pivotal role it plays in driving business growth and enhancing customer satisfaction. ZBrain Flow adeptly tackles the intricate challenge of selecting ideal offers for diverse customer segments by seamlessly gathering pertinent data from various sources. This includes customer profiles, purchase history, social media engagements, and prevailing market trends. The platform processes this data meticulously, unveiling correlations and patterns and effectively segmenting customers based on their preferences and behaviors. ZBrain employs advanced embedding techniques to convert text-based data into numerical representations, facilitating precise analysis and insightful information extraction for targeted offer strategies. The automated, AI-powered process significantly reduces the time and effort traditionally associated with offer targeting. To explore the streamlined Flow and witness how ZBrain transforms the offer targeting process, click here.
AI-driven store performance analysis and KPI tracking
ZBrain transforms store performance analysis and KPI tracking, recognizing these metrics’ critical role in achieving retail success. The platform streamlines the complex process of gathering and interpreting data from various sources, such as point-of-sale systems, customer databases, and inventory management platforms. ZBrain automates data collection, integrating information from sales, store operations, inventory, and customer behavior into a centralized database for comprehensive analysis. Following data compilation, ZBrain initiates an automated Exploratory Data Analysis (EDA) process, thoroughly examining the data to reveal meaningful patterns, trends, and relationships. The platform then generates a final store performance analysis and KPI tracking report, providing actionable insights for informed decision-making. ZBrain apps significantly enhance accuracy, facilitate strategic planning, and foster a data-driven culture. Explore this detailed Flow to witness how ZBrain transforms store performance analysis and KPI tracking, empowering retail businesses with efficient and impactful insights.
AI-driven inventory and demand forecasting
ZBrain transforms the landscape of inventory and demand forecasting, recognizing these processes’ vital role in sustaining retail success and customer satisfaction. ZBrain helps balance optimal inventory levels with accurate demand forecasting. The platform autonomously collects relevant data, including historical sales, current inventory levels, product catalog, seasonality information, and supplier lead times from diverse sources. Automated Exploratory Data Analysis (EDA) follows, extracting valuable insights and identifying patterns that influence demand forecasting and inventory optimization. ZBrain employs advanced embedding techniques to convert textual data into numerical representations, capturing contextual relationships for efficient retrieval and analysis. This seamless transformation equips businesses with precise insights, enhancing decision-making. Explore this detailed Flow to understand how ZBrain redefines inventory and demand forecasting, offering a data-driven approach to retail success.
AI-driven cross-selling for retail businesses
ZBrain reshapes cross-selling strategies for retail success, recognizing the importance of seamless product recommendations in driving customer engagement. The platform streamlines cross-selling by automating data collection from diverse sources, including customer purchase history, product catalog, and inventory data. This data is consolidated into a central database, creating a comprehensive view of products and customer preferences. Recommender systems built on ZBrain leverage this consolidated data to provide personalized recommendations, facilitating efficient cross-selling efforts. When users request a product recommendations report, the system retrieves necessary data based on query criteria and employs an LLM for report generation. With insights into customer preferences and product associations, ZBrain empowers retailers to significantly enhance cross-selling efficiency, increase sales, and foster customer loyalty. Explore this detailed Flow to understand how ZBrain transforms cross-selling, offering personalized recommendations derived from comprehensive data analysis.
LeewayHertz’s AI development services for retail
At LeewayHertz, we build tailor-made AI solutions that meet the specific needs of retail businesses. Our strategic AI/ML consulting helps retailers harness the power of AI for enhanced customer engagement, personalized experiences, improved operational efficiency, and data-driven decision-making.
We specialize in developing Proof of Concepts (PoCs) and Minimum Viable Products (MVPs), enabling retailers to witness the potential impact of AI tools in real-world scenarios. This ensures solutions are effective and specifically adapted to the retail sector’s unique needs.
Our expertise in generative AI streamlines routine tasks like product description generation, customer service automation, personalized recommendations and inventory forecasting. These automated processes free up valuable employee time for more strategic initiatives.
By fine-tuning large language models, we help retailers to understand customer preferences and their buying behaviors. This ensures that AI-powered interactions and recommendations are accurate and relevant and contribute to a seamless and enjoyable shopping experience.
Moreover, we prioritize seamless integration of AI systems with existing retail technology infrastructure, such as inventory management software, Point of Sale (POS) software, and retail ERP systems. This optimizes operations and drives smarter decision-making across all business aspects for increased efficiency and profitability.
Our AI solutions development expertise
AI solutions development for retail typically involves creating systems that automate routine tasks, enhance purchasing decisions and personalize customer experiences. These solutions integrate key components such as data aggregation technologies, which compile and analyze consumer information from diverse sources like point-of-sale systems, online interactions, market trends, sales patterns and loyalty programs. This comprehensive data foundation supports predictive analytics capabilities, allowing retailers to anticipate customer demand and forecast trends that inform strategic inventory and marketing decisions.
Additionally, machine learning algorithms are employed to tailor product recommendations to individual customer profiles, ensuring that each shopper’s unique preferences, past purchases, and browsing behavior are considered. These solutions often cover areas like inventory optimization, demand forecasting, price optimization, personalized product recommendations, targeted marketing campaigns, customer service automation, and visual search functionality.
Overall, AI solutions in retail are designed to optimize operations, enhance customer engagement, improve sales outcomes and create a seamless and personalized shopping experience that drives business growth and customer loyalty.
AI agent/copilot development for retail
LeewayHertz builds custom AI agents and copilots that enhance various functions, improving efficiency and decision-making and creating new growth opportunities. Here’s a breakdown of how they contribute:
- Customer service automation:
- Provide instant customer support, answer frequently asked questions, handle complex inquiries, resolve simple issues, and guide customers through purchasing.
- Analyze customer data and past interactions to understand customer needs and recommend products, offers, and services that align with their preferences and needs.
- Analyze customer feedback to identify trends and areas for improvement, helping retailers tailor their services.
2. Operations optimization:
- Analyze historical sales data, external factors, and current trends to predict future demand, optimizing inventory levels and reducing stockouts or overstocking.
- Track competitor prices, analyze market trends, and adjust prices to maximize revenue.
- Optimize logistics, track shipments, and predict potential disruptions to ensure smooth operations.
- Trigger orders based on predefined thresholds and demand forecasts, minimizing manual intervention and ensuring timely restocking.
- Optimize warehouse layout, picking and packing routes, and even manage robotic systems for more efficient and cost-effective logistics.
3. Marketing automation:
- Analyze customer segments to identify specific needs and preferences, enabling targeted advertising campaigns that ensure the right message reaches the right audience.
- Personalize email marketing campaigns and send targeted notifications based on customer behavior and preferences.
- Manage social media interactions, respond to comments and inquiries, and create engaging content.
4. Fraud detection and prevention:
- Monitor transactions for suspicious activity and flag potential fraudulent behavior, reducing losses and protecting customers.
- Assess customer profiles and purchasing patterns to identify potential risks, enabling retailers to take proactive measures.
5. Store operations optimization:
- Analyze customer movement patterns and optimize store layout and navigation to improve shopping experience and increase sales.
- Analyze customer traffic patterns and schedule staff efficiently to ensure optimal coverage and minimize labor costs.
- Generate and update price tags, reducing manual effort and ensuring accurate pricing information.
AI agents and copilots are transforming the retail industry by automating operational tasks, elevating customer service, and empowering strategic decision-making. By seamlessly integrating LeewayHertz’s advanced AI solutions, retailers can offer personalized experiences, predict trends, optimize operations, and ultimately gain a competitive edge in the dynamic retail landscape. The result is a customer-centric approach that fosters loyalty drives growth and ensures a future where AI is the key to retail success.
How to implement AI solutions in retail business?
Implementing AI solutions in retail involves several steps, from data collection to training models. Here is a general guide to help you through the process:
- Define objectives and use cases: Clearly define the business objectives and identify specific use cases where AI can add value. This could include demand forecasting, personalized recommendations, inventory optimization, fraud detection, and more.
- Data collection: Collect relevant data from various sources, including transaction data, customer data, inventory data, and external data sources. Ensure the data is accurate, comprehensive, and in a format suitable for analysis.
- Data cleaning and preprocessing: Clean and preprocess the data to handle missing values, outliers, and inconsistencies. Convert data into a format suitable for training machine learning models.
- Data integration: Integrate data from different sources to create a unified dataset. This may involve using data integration tools or platforms to combine structured and unstructured data.
- Data security and privacy: Ensure that customer and business data are handled securely and in compliance with privacy regulations. Implement encryption, access controls, and other security measures to protect sensitive information.
- Select AI algorithms: Choose the appropriate machine learning algorithms based on the specific use cases. Common algorithms in retail include linear regression, decision trees, clustering algorithms, and deep learning for more complex tasks.
- Model training: Use historical data to train machine learning models. Split the data into training and testing sets to evaluate the model’s performance. Experiment with different algorithms and hyperparameters to optimize model performance.
- Deploy models: Once the models are trained and validated, deploy them into a production environment. This may involve integrating the models into existing retail systems or deploying them on cloud platforms.
- Monitoring and maintenance: Implement monitoring systems to track the performance of deployed models in real time. Set up alerts for any anomalies or degradation in model performance. Regularly update models to adapt to changing patterns in the retail environment.
- User interface and integration: Develop user interfaces or integrate AI solutions into existing retail systems. This could involve creating dashboards for business users to interact with and interpret AI-driven insights.
- Feedback loop: Establish a feedback loop to improve models continuously. Collect feedback from users and monitor the impact of AI solutions on business metrics. Use this information to enhance models and algorithms iteratively.
LeewayHertz is your trusted partner for cutting-edge AI solutions tailored to elevate your business. Our expert team leverages advanced machine learning algorithms and data analytics to craft custom solutions, empowering enterprises with intelligent automation, predictive analytics, and personalized customer experiences. With a proven track record in delivering innovative AI applications, LeewayHertz is committed to driving your business success through transformative technology solutions. Explore our services for tailored solutions!
Benefits of AI in retail
AI has the potential to streamline operations in the retail industry, enhancing the customer experience and increasing revenue. Here are some of the key benefits of AI in retail and e-commerce:
- Personalization: AI algorithms can analyze customer data, such as browsing history, purchase behavior, and demographic information, to provide personalized product recommendations and create tailored shopping experiences. This level of personalization can lead to increased customer loyalty and higher sales.
- Enhanced customer service: AI-powered chatbots and virtual assistants can help retailers provide 24/7 customer service, answer customer inquiries, and provide support. This can help retailers reduce costs and increase customer satisfaction.
- Improved inventory management: AI can help retailers optimize their inventory management processes by predicting demand, identifying patterns, and automating ordering and restocking. This can help retailers reduce waste and improve efficiency.
- Increased efficiency: AI can automate repetitive tasks and processes, allowing employees to focus on more complex tasks. This can lead to increased productivity, reduced costs, and improved accuracy.
- Better pricing and promotions: AI can help retailers optimize their pricing and promotions strategies by analyzing market trends, consumer behavior, and sales data. This can help retailers offer competitive pricing and targeted promotions that resonate with customers.
- Fraud detection and prevention: AI can help retailers detect and prevent fraudulent activities such as payment fraud, chargebacks, and account takeover. This can help retailers reduce losses and protect their customers.
- Increased customer engagement: Retailers harness the power of AI to offer seamless customer support in diverse scenarios, from automated checkouts to tracking customer moods. AI-based retail solutions also enable personalized and immersive shopping experiences, ultimately driving higher levels of customer engagement.
- Enhanced customer experiences: In order to maintain consumer interest, retailers must differentiate their products and provide compelling services and experiences. By incorporating AI and predictive analytics to gather market insights, retailers can lead with innovation rather than merely reacting to market changes.
- Effective promotion and merchandising: AI-driven retail solutions play a pivotal role in marketing and merchandise planning. They assist in segmenting customers, creating content, and devising and executing targeted advertising campaigns. For example, Walmart has already adopted AI to scan competitors’ offerings and aims to leverage robotics for shelf optimization in the near future.
- Enhanced operational efficiency: AI not only automates routine tasks but also supports and enhances the performance of increasingly demanding work. In the retail industry, AI optimizes labor scheduling, delivery tracking, and route planning, among other tasks.
- Informed business decisions: Collecting data is one thing, but making sense of it is another challenge that many businesses face. Traditional analytics tools have their merits, but AI and machine learning offer a new dimension. AI-driven predictive analytics, in particular, stands out by providing businesses with informed insights and accurate forecasts of future product demand patterns, whether for specific items or entire categories within an e-commerce store.
These are just a few benefits of AI in the retail sector. As AI technology continues to advance, its impact on this sector is likely to grow even further.
Future trends of AI in retail
The use of AI in retail is growing rapidly, thanks to its ability to help retailers better understand consumer behavior, improve operational efficiency, and enhance the overall customer experience.
Automated checkout and cashier-less stores
AI-powered checkout solutions, like cashierless stores, will become more prevalent. Shoppers can simply pick up items and walk out, and the AI system will automatically charge them for their purchases. This streamlines the shopping process and reduces checkout friction.
Sustainability and ethical sourcing
AI will be used to track and verify product sustainability and ethical sourcing. Customers are increasingly conscious of their purchases’ environmental and ethical impact, and AI can provide transparency into the supply chain to support responsible shopping decisions.
Price predictions
AI will help retailers with price adjustments and play a crucial role in price forecasting. Retailers can use AI to consider various factors, such as changing product demand, seasonal trends, and the release dates of new product models within the same category. This will enable them to make accurate price predictions. Customers can benefit from this feature by knowing in advance when and how the price of a particular product is likely to change, similar to how flight rates for upcoming months are currently available, allowing for better shopping planning.
Delivery by drones
Drone deliveries are on the horizon and are closer to becoming a reality. Companies like Amazon have gained approval to use self-piloting electronic drones for package deliveries. While these technologies are currently in the testing phase, it won’t be long before we see drones delivering items in under 30 minutes. This will redefine the speed and efficiency of last-mile delivery in e-commerce.
Voice search
Several companies are already using voice search recognition to provide quick search and discovery experiences. In the future, we can expect advanced versions of this technology to be adopted by industry leaders to make the retail experience even more seamless for customers. As more people rely on voice search for convenience, retailers will likely integrate voice search solutions into their websites, enhancing the overall shopping process.
Tracking customer satisfaction
Some retailers like Walmart have implemented facial recognition systems to detect customer moods using AI at their checkout lanes. This technology allows retailers to assess whether consumers are satisfied or annoyed with their shopping experience. In the future, more retailers are expected to invest in mood-tracking technology to study customer moods. This data will enable retailers to build stronger relationships with customers by offering improved shopping experiences during subsequent visits.
Automated product tagging
AI is set to redefine product tagging within catalogs. Imagine a scenario where a jacket is effortlessly tagged with descriptors like “red,” “short sleeve,” “leather,” and more, all without human intervention. This streamlined and rapid process not only saves time but also enhances efficiency. Furthermore, automated product tagging holds immense potential for improving text and visual search capabilities, delivering targeted recommendations, and creating a more seamless and satisfying shopping experience. In the coming years, automated product tagging will serve as a pivotal factor in elevating the overall shopping experience for consumers.
Emotion-spotting AI in retail stores
Emotion-spotting AI recognizes human emotions through facial expressions, speech, and gestures. It can personalize the in-store shopping experience, analyzing customers’ facial expressions as they explore the products and aisles.
The future of AI in retail demonstrates how AI technologies are continuously evolving to enhance customer experiences, streamline operations, and address emerging challenges in the industry. Retail companies that embrace these trends will be better equipped to thrive in the competitive landscape of the future.
Conclusion
AI has become a game-changer for the retail, providing new opportunities for growth and improved customer experiences. It has seamlessly integrated itself into various facets of business operations, from personalized recommendations to efficient inventory management. This disruptive technology drives innovation and efficiency in sectors that constantly seek ways to improve, whether it’s personalizing customer interactions or optimizing supply chains and pricing strategies. As the adoption of AI continues to surge, AI development companies emerge as pivotal partners in helping retailers harness its vast potential. The expanding landscape of AI use cases includes chatbots, voice assistants, image recognition, and predictive analytics, all poised to enhance these industries further. By collaborating with AI development companies, retail businesses can unlock AI’s full potential, gaining a competitive edge and paving the way for unprecedented growth and success in the years to come. The journey of AI in retail is an ongoing story, marked by continuous evolution and remarkable possibilities.
Redefine your retail business with AI-driven success. Partner with Leewayhertz’s AI experts today to supercharge your sales, streamline operations, and elevate customer satisfaction.
Start a conversation by filling the form
All information will be kept confidential.
FAQs
What is AI in retail?
AI in Retail refers to the application of artificial intelligence technologies in various aspects of the retail sector. It benefits the industry by improving efficiency, enhancing customer experiences, optimizing supply chain management, and providing data-driven insights for better decision-making.
How can AI improve the customer shopping experience?
AI enhances the customer shopping experience through personalized recommendations, virtual assistants, and chatbots. It analyzes customer behavior to offer tailored product suggestions, answers queries in real-time, and provides a seamless and engaging shopping journey.
How does AI contribute to pricing strategies in retail?
AI assists in dynamic pricing by analyzing market trends, competitor pricing, and customer behavior. Retailers can optimize pricing strategies in real time, ensuring competitiveness, maximizing profits, and responding swiftly to market changes.
Can AI be used for fraud detection and security in retail?
Yes, AI is valuable for fraud detection in retail. It analyzes transactions, identifies irregular patterns, and flags potentially fraudulent activities. Additionally, AI-based security systems enhance overall store security by detecting unusual behavior and potential threats.
How does AI improve the efficiency of customer support in retail?
AI-driven chatbots and virtual assistants handle routine customer queries, providing instant responses and freeing up human agents for more complex issues. This enhances customer support efficiency, leading to quicker problem resolution and improved customer satisfaction.
Can AI be used for predicting trends and consumer preferences in retail?
Yes, AI analyzes vast amounts of data, including social media trends and historical purchase behavior, to predict future trends and consumer preferences. This insight helps retailers stock popular products and tailor their offerings to meet evolving customer demands.