Exploring AI TRiSM: Need, pillars, framework, use cases, implementation, and future scope
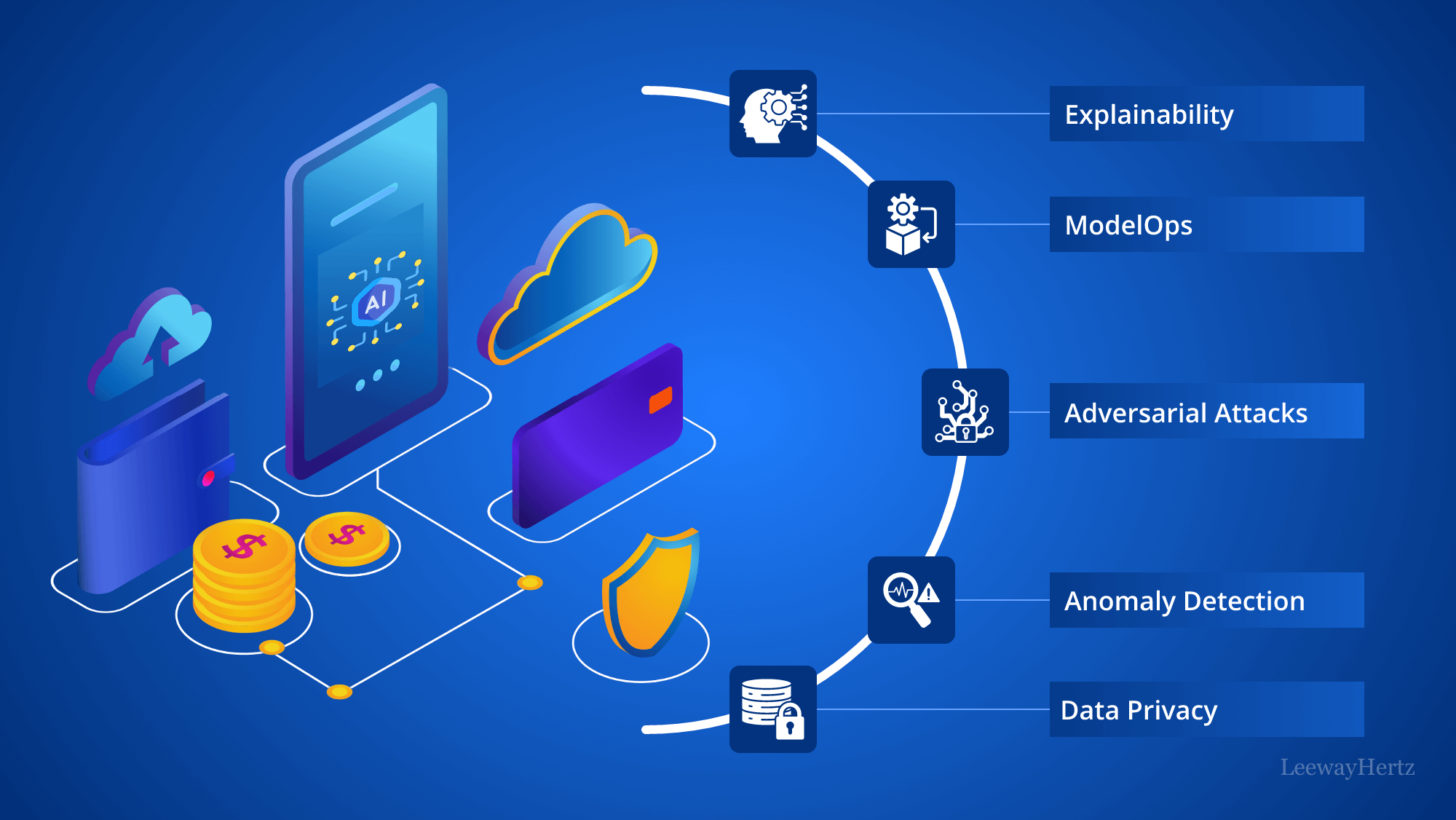
The rapid evolution of technology has ushered in a new era of Artificial Intelligence (AI), significantly impacting various facets of society, from the economy to technological advancements. This surge in artificial intelligence capabilities encompasses advancements in fields like machine learning and natural language processing. These advancements have led to the development of sophisticated tools like voice recognition systems, reshaping everyday experiences through applications like smart traffic management systems in cities, self-driving features in cars, and AI-powered tutoring platforms for personalized learning. As AI technologies permeate critical sectors such as healthcare, finance, and transportation, their adoption presents unique challenges and concerns, particularly related to trust, risk, and security.
Trust in AI is fundamental, as it underpins the confidence users place in the technology’s reliability, honesty, and ethical deployment. However, the risks associated with AI, such as biases, data privacy breaches, and potential harm, necessitate robust frameworks to manage these issues effectively. AI Trust, Risk, and Security Management (AI TRiSM) emerged as a critical framework designed to address these challenges. It offers a structured approach for evaluating AI systems’ transparency, explainability, and accountability, fostering a safer and more reliable AI infrastructure.
According to a Gartner® report, organizations that adopt AI TRiSM can expect a 50% increase in AI model adoption and operational efficiency by 2026, underscoring the framework’s crucial role in facilitating secure and trustworthy AI implementations. Furthermore, Chief Information Security Officers (CISOs) championing AI TRiSM can enhance AI results significantly—by increasing the speed of AI model-to-production, enabling better governance, and rationalizing AI model portfolios, potentially eliminating up to 80% of faulty and illegitimate information.
Further supporting these insights, Emergen Research predicts robust growth in the AI TRiSM market. According to this report,
- The AI TRiSM market is projected to grow at a Compound Annual Growth Rate (CAGR) of 16.2%, and in 2022, it was valued at approximately USD 1.72 billion.
- A significant driver of market revenue growth is the increasing government initiatives to implement AI technology in various industries, especially in the Banking, Financial Services, and Insurance (BFSI) sectors.
- The solutions component of the AI TRiSM market is expected to register a CAGR of 16.0%, indicating robust growth and innovation in this sector’s solutions.
AI TRiSM stands at the forefront of AI governance, emphasizing fairness, efficacy, and privacy. This framework is vital for organizations aiming to implement systematic methods to mitigate AI-related risks and ensure ethical considerations are central to AI deployments. As we delve deeper into the AI TRiSM framework, this article will explore its need, pillars, implementation and pivotal role in advancing trustworthy AI solutions.
- Introducing AI TRiSM
- Why is AI TRiSM important?
- Six essential reasons to integrate AI TRiSM into your AI projects
- The foundational pillars of AI Trust, Risk, and Security Management (AI TRiSM)
- Overview of different AI TRiSM frameworks
- Use cases of AI trust, risk, and security management
- AI TRiSM: Challenges and effective implementation strategies
- The future of AI trust, risk, and security management
- LeewayHertz’s approach to AI trust, risk, and security management
Introducing AI TRiSM
AI TRiSM, an acronym coined by Gartner, stands for AI Trust, Risk, and Security Management. It is a comprehensive framework designed to guide organizations in identifying and mitigating risks associated with the reliability, security, and trust of AI models and applications. As AI technologies become integral to various business functions, ensuring robust governance around these systems—particularly concerning security and privacy—has become imperative.
Leveraging AI TRiSM, organizations automate repetitive tasks, enhance predictive capabilities for potential issues, and enable proactive solutions for customers. The primary objective of AI TRiSM extends beyond merely improving operational efficiency; it is also aimed at significantly enhancing customer satisfaction and building trust in AI applications across various sectors.
Why is AI TRiSM important?
The rapid ascent of artificial intelligence (AI) has catalyzed a revolution in various sectors, delivering matchless efficiency, automation, and enhanced decision-making capabilities. Yet, this technological advancement comes with its set of challenges and risks that organizations need to confront.
As AI technologies become increasingly commonplace, many users and organizations deploy these tools without fully understanding the underlying mechanisms. There is often a gap in knowledge regarding how AI models function, the data they use, and their potential biases. This lack of clarity can lead to misinterpretations and unintended consequences. Moreover, third-party AI tools introduce additional complexities, including serious data privacy issues, inaccuracies, and the risk of malicious use, such as generative AI attacks that can manipulate or fabricate information.
The absence of robust risk management, continuous monitoring, and stringent controls may result in AI models that are unreliable, untrustworthy, or insecure. This is particularly concerning as AI applications become more integral to critical functions across industries. In response, governments and regulatory bodies are stepping up to create comprehensive laws aimed at regulating AI use, but there is still a significant need for internal governance.
The AI Trust, Risk, and Security Management (AI TRiSM) framework emerges as a vital tool in this context. By integrating AI TRiSM, organizations can not only improve the results of their AI implementations but also ensure these technologies are used safely and ethically. AI TRiSM helps understand and mitigate the risks associated with AI, ensuring compliance with evolving regulations and maintaining the integrity and security of AI systems.
Thus, while AI continues to offer significant advantages, the importance of managing its risks through frameworks like AI TRiSM cannot be understated. As AI technologies evolve, the implementation of comprehensive risk and security management strategies will be crucial in harnessing their full potential responsibly.
Optimize Your Operations With AI Agents
Optimize your workflows with ZBrain AI agents that automate tasks and empower smarter, data-driven decisions.
Six essential reasons to integrate AI TRiSM into your AI projects
As the deployment of AI continues to expand across various sectors, implementing a robust AI Trust, Risk, and Security Management (AI TRiSM) program becomes indispensable. Here are six compelling reasons why building AI TRiSM into your AI models is crucial:
- Enhancing understandability and transparency
- Most stakeholders need a deeper understanding of how AI models operate. AI TRiSM facilitates the clear articulation of AI functions, strengths, weaknesses, behaviors, and potential biases. This transparency is vital for enabling all users, from managers to consumers, to fully grasp the intricacies of AI operations and trust the outcomes.
- Addressing risks from widespread access to generative AI
- Tools like ChatGPT have democratized access to powerful AI capabilities, introducing new risks that traditional controls cannot manage. AI TRiSM helps mitigate these risks, particularly in environments like the cloud, where the pace of change and potential vulnerabilities can be significant.
- Securing data privacy with third-party AI tools
- Integrating third-party AI tools often involves handling sensitive data and large datasets, which can pose serious confidentiality and privacy risks. AI TRiSM ensures that data management practices comply with privacy standards and safeguard against breaches, thereby protecting both the organization and its customers.
- Continuous monitoring and adaptation
- AI models require ongoing monitoring to ensure they remain compliant, fair, and ethical throughout their lifecycle. AI TRiSM involves setting up continuous oversight mechanisms through specialized ModelOps processes, which adapt to new challenges and maintain the integrity of AI systems.
- Protecting against adversarial attacks
- AI systems are increasingly targeted by sophisticated attacks such as prompt injections that can cause significant harm. AI TRiSM addresses these threats by implementing advanced security measures and robust testing protocols to effectively detect, prevent, and respond to adversarial attacks.
- Regulatory compliance
- Compliance has become more complex and critical, with global regulatory frameworks like the EU AI Act shaping the landscape. AI TRiSM ensures that AI models are not only compliant with current regulations but are also prepared to meet future legislative changes. This proactive compliance helps avoid penalties and supports sustained business growth.
By incorporating AI TRiSM, organizations can enhance the efficiency and effectiveness of their AI models and build a foundation of trust and security that supports long-term success in an AI-driven world.
The foundational pillars of AI Trust, Risk, and Security Management (AI TRiSM)
AI TRiSM (AI Trust, Risk, and Security Management) is anchored by five basic pillars that form the core of its framework. These pillars address the complexities and challenges of managing AI systems and ensure that AI systems are not only effective but also operate within ethical and secure boundaries.
Explainability
Explainability ensures that AI systems are transparent in their operations. This pillar focuses on making the internal mechanisms of machine learning models clear and understandable to users. By enhancing the visibility of how decisions are made, organizations can monitor AI performance and identify areas for improvement, thereby optimizing productivity and ensuring that AI acts within the intended parameters.
ModelOps
ModelOps is the governance and lifecycle management of AI models, encompassing everything from development to deployment and maintenance. This process ensures that AI systems are kept up-to-date and perform optimally across their operational life. ModelOps facilitates efficient scaling, ensures consistent performance, and adapts to new challenges as they arise.
Data anomaly detection
This pillar focuses on identifying and correcting data inconsistencies that could skew AI outcomes. Effective data anomaly detection helps maintain the accuracy and integrity of AI decision-making processes, ensuring that AI systems produce reliable and fair results.
Adversarial attack resistance
Adversarial attack resistance safeguards AI systems against malicious attacks that seek to manipulate or corrupt their functioning. This includes implementing strategies like adversarial training, defensive distillation, model ensembling, and feature squeezing to fortify AI against external threats and ensure robust performance.
Data protection
Data is at the heart of AI functionality. Protecting this data is crucial for operational integrity and maintaining user privacy and compliance with data protection regulations such as GDPR. This pillar ensures that all data used by AI systems is securely managed and protected against unauthorized access and breaches.
Integrating the AI TRiSM framework
By deploying these five pillars, AI TRiSM creates a robust framework that enhances AI applications’ security, reliability, and efficacy. The comprehensive approach of AI TRiSM ensures that:
- AI systems are transparent, and their decision-making processes are understandable to users, which enhances trust.
- The lifecycle of AI models is managed efficiently from creation to retirement, ensuring they remain relevant and effective.
- Data integrity is maintained, which is crucial for the accuracy and fairness of AI outputs.
- AI systems are safeguarded against potential security threats, maintaining their integrity and trustworthiness.
- Data privacy and protection measures are strictly enforced, aligning with global compliance standards.
Each pillar of AI TRiSM addresses specific risks associated with AI deployments, ensuring that organizations can leverage the benefits of AI technologies while mitigating potential drawbacks. This structured approach to AI governance facilitates better performance and fosters a regulatory-compliant and ethically aligned operational environment.
Overview of different AI TRiSM frameworks
Although the term AI TRiSM has been coined by Gartner, numerous Trust, Risk, and Security Management frameworks exist that guide effective AI implementation. These frameworks are crucial for organizations seeking to identify, assess, and mitigate risks associated with AI systems. They offer structured approaches and guidelines to ensure the development of ethical, fair, and secure AI systems. Notable frameworks include Gartner’s TRiSM, the NIST AI Risk Management Framework, Microsoft Responsible AI Framework, Google AI Principles, and the World Economic Forum’s Principles of Responsible AI.
Gartner’s Trust, Risk, and Security Management (TRiSM)
Gartner’s TRiSM is a comprehensive framework that provides extensive guidelines and best practices for managing trust, risk, and security in AI systems. This framework aims to ensure AI systems are ethical, fair, reliable, and secure, helping organizations to deploy AI solutions that align with these core principles.
National Institute of Standards and Technology (NIST) AI Risk Management Framework
The NIST AI Risk Management Framework offers detailed strategies for identifying, assessing, and mitigating risks in AI systems. It covers crucial areas such as data privacy, security, and the potential for bias, providing a holistic approach to managing the complexities and challenges of AI risk.
Microsoft Responsible AI Framework
Microsoft’s framework provides comprehensive guidance on developing and deploying AI systems that adhere to ethical, fair, and accountable standards. It addresses critical aspects, including bias, fairness, transparency, and privacy, promoting responsible AI development and usage practices.
Google AI Principles
Google’s AI Principles outline the company’s commitment to developing and deploying AI in a manner that upholds fairness, privacy, and security. These principles serve as a guide not only for Google’s own AI initiatives but also offer valuable insights for other organizations aiming to implement ethical AI practices.
World Economic Forum’s (WEF) Principles of Responsible AI
Crafted by a panel of international experts, the WEF’s Principles of Responsible AI provide recommendations on creating ethical, fair, and accountable AI systems. These guidelines address a broad spectrum of issues, including bias, fairness, transparency, privacy, and security.
These frameworks provide essential guidance for organizations navigating the intricate landscape of AI deployment. By outlining key ethical considerations, risk management strategies, and accountability measures, they help organizations align their AI practices with globally recognized standards of responsibility and security. Each framework brings a unique perspective to the table, enriching the collective understanding of how to best manage the evolving challenges presented by AI technologies.
To complement these frameworks, various AI TRiSM tools are available to support organizations in effectively managing and implementing AI systems. Below is a table that details the categories of these tools, their functions, and some examples, providing a practical guide for organizations to enhance their AI deployments:
Category | Details | Example Tools |
---|---|---|
Risk Management Tools | Tools that assist in identifying, assessing, and mitigating risks associated with AI implementations. | Fairlearn, AI Fairness 360 (OSS), RiskLens, SAS Risk Management |
ModelOps Tools | Tools focused on managing and optimizing the lifecycle of AI models. | MLflow, Kubeflow (OSS), TFX, Polyaxon |
AI Ethics and Governance Tools | Ensure AI systems adhere to ethical guidelines and governance policies. | IBM Watson OpenScale, Deloitte’s Cortex Ethical AI, PwC Responsible AI Toolkit |
Data Anomaly Detection Tools | Tools designed to identify anomalies and irregularities in data sets used by AI systems. | Great Expectations, Apache Griffin, Datadog, Splunk |
Content Anomaly Detection Tools | Tools that focus on identifying anomalies in the content processed by AI systems. | Revuze, spaCy, ContentWatch, Google Perspective API |
Data Privacy Assurance Tools | Tools that provide safeguards for data privacy, including encryption tools and access controls. | Cryptomator, HashiCorp Vault, OneTrust, TrustArc |
AI Observability Tools | Tools that provide insights into AI system operations, helping to monitor, debug, and ensure the performance of AI models. | New Relic AI, Dynatrace, Datadog AI Ops |
These tools and frameworks provide essential guidance for organizations navigating the intricate landscape of AI deployment. By outlining key ethical considerations, risk management strategies, and accountability measures, they help organizations align their AI practices with globally recognized standards of responsibility and security.
Optimize Your Operations With AI Agents
Optimize your workflows with ZBrain AI agents that automate tasks and empower smarter, data-driven decisions.
Use cases of AI trust, risk, and security management
AI TRiSM frameworks are critical for organizations deploying enterprise AI to manage and mitigate risks effectively. Here are some key areas where AI TRiSM is particularly valuable:
1. Financial services
- Fraud detection: Implementing AI TRiSM ensures that AI-driven fraud detection systems operate within ethical guidelines while maintaining accuracy and minimizing false positives.
- Regulatory compliance: AI TRiSM helps financial institutions comply with evolving regulations like GDPR and the Fair Credit Reporting Act, ensuring AI applications in credit scoring and risk assessments meet legal standards.
2. Healthcare
- Patient data management: AI TRiSM frameworks ensure the protection of sensitive patient data, aligning with HIPAA regulations and enhancing patient trust.
- Diagnostic accuracy: Through continuous monitoring and testing, AI TRiSM ensures that AI diagnostic tools remain accurate and reliable, reducing the risk of misdiagnoses due to model drift or bias.
3. Retail and e-commerce
- Customer personalization: AI TRiSM guides the ethical use of AI in personalizing customer experiences, ensuring that data handling and customer profiling respect privacy norms.
- Supply chain optimization: Monitoring AI applications in supply chain logistics helps identify risks and biases, ensuring efficient and fair operations.
4. Autonomous vehicles
- Safety and reliability: AI TRiSM frameworks manage risks in the development and deployment of autonomous driving technology, focusing on safety, compliance, and ethical considerations.
- Data security: An autonomous vehicle system needs a vast array of data crucial for its operation and passenger convenience and safety, including real-time location data, sensor outputs for navigation, and personal passenger information for user-specific services. Ensuring the security of this data against cyber threats is critical to maintain privacy, functionality, and compliance with data protection regulations. Moreover, integrating AI Trust, Risk, and Security Management (AI TRiSM) ensures that these cybersecurity measures are continuously evaluated and improved upon, aligning them with evolving threats and regulatory requirements. AI TRiSM frameworks help automate risk assessments, enforce governance policies, and enhance incident response strategies, ensuring a robust defense mechanism is always in place.
5. Public sector
- Smart city initiatives: AI TRiSM ensures that AI applications in traffic management, public safety, and utility services are transparent, fair, and secure.
- Regulatory enforcement: Leveraging AI TRiSM helps government agencies enforce regulations effectively, ensuring AI tools used in surveillance or data analysis respect civil liberties.
6. Cybersecurity
- Threat detection and response: AI TRiSM frameworks enhance the reliability and accuracy of AI systems used to detect and respond to cyber threats, minimizing false positives and ensuring rapid response to real threats.
7. Education
- Adaptive learning systems: Ensuring that AI-driven adaptive learning platforms operate transparently and fairly, enhancing educational outcomes without bias.
- Data privacy: AI TRiSM protects student data, aligning educational tools with privacy laws and ethical standards.
These use cases demonstrate the broad applications of AI TRiSM frameworks across various industries, ensuring that AI systems are used responsibly, ethically, and securely. By addressing the inherent risks associated with AI, these frameworks help organizations not only comply with regulatory requirements but also build trust with their stakeholders.
AI TRiSM: Challenges and effective implementation strategies
Implementing AI Trust, Risk, and Security Management (AI TRiSM) is essential for harnessing AI’s benefits while managing the associated risks. This section outlines the primary challenges in deploying AI TRiSM frameworks and offers comprehensive strategies for effective implementation.
Challenges of implementing AI TRiSM
- Data privacy and security concerns: AI systems require extensive data to operate, which raises significant concerns about privacy and the potential for data breaches. Ensuring the security of this data and compliance with international data protection regulations like GDPR is a persistent challenge.
- Integration as an afterthought: AI TRiSM is often overlooked during the initial stages of AI model and application development. Organizations typically consider these frameworks only after the systems are already in production, which can make integration more cumbersome and less effective.
- Ethical considerations and bias: AI can inadvertently perpetuate the biases present in its training data, leading to unethical outcomes and decision-making. Addressing these biases to ensure fair and ethical AI operations is a critical challenge for organizations.
- Algorithmic transparency and accountability: The complex nature of AI algorithms can lead to a lack of transparency, making it difficult for users to understand how decisions are made. This “black box” nature of AI systems challenges the accountability and trust users have in AI solutions.
- Handling of hosted Large Language Models (LLMs): Enterprises using hosted LLMs face challenges with the lack of native capabilities to filter inputs and outputs automatically. This includes preventing policy violations related to confidential data or correcting inaccurate information used for decision-making.
- Dependency and overreliance on AI: There is a risk of becoming overly dependent on AI systems, potentially leading to a degradation in human decision-making skills and increased vulnerability in the event of AI failures.
- Complexity of AI threats: The threats associated with AI are complex and not always well-understood, which can lead to inadequate responses to security vulnerabilities. Without a deep understanding of AI-specific threats, organizations may fail to implement effective measures to detect, prevent, and mitigate these risks, leaving systems vulnerable to attacks.
- Cross-functional team coordination: AI TRiSM requires the collaboration of cross-functional teams, including legal, compliance, security, IT, and data analytics, to establish and maintain a unified approach to AI governance. Coordinating these diverse teams and aligning their objectives can be challenging, often leading to fragmented efforts and suboptimal implementation of AI TRiSM frameworks.
- Technical challenges: Implementing AI TRiSM involves navigating algorithmic complexity, scalability issues, and integration with existing technological infrastructures, which can be daunting and resource-intensive.
- Integration of lifecycle controls: While the integration of lifecycle controls within AI TRiSM is feasible, it presents significant challenges due to the complex nature of AI operations and the continuous evolution of AI technologies. Effective lifecycle management requires dynamic and adaptable frameworks that can evolve with the AI models and applications, demanding ongoing adjustments and updates to ensure continuous protection and compliance.
- Regulatory compliance: AI deployments must navigate a rapidly evolving regulatory landscape, making compliance a moving target. Keeping up with varied and changing regulations across different jurisdictions remains a significant challenge.
- Insufficient understanding and lack of human participation: There is often a gap in AI knowledge among stakeholders, which can hinder effective deployment and oversight. Additionally, insufficient human oversight can exacerbate the risk of errors and ethical issues.
Implementation strategies for AI TRiSM
- Establishing strong data governance: To tackle privacy and security issues, organizations should implement strong data governance policies that include data encryption, regular security audits, and robust access controls. These measures help protect data integrity and ensure compliance with privacy laws.
- Promoting transparency and explainability: Organizations should strive to demystify AI processes by adopting explainable AI (XAI) technologies. This involves developing AI systems whose actions can be easily understood and traced by humans, thus enhancing transparency and building stakeholder trust.
- Define granular acceptable use policies: Establish clear, detailed and acceptable use policies for AI applications. These policies should be granular enough to enforce specific standards of use at every level of interaction, preventing misuse and ensuring alignment with organizational ethics.
- Regular auditing and bias mitigation: Regular audits should be conducted to assess AI models for potential biases to ensure AI systems are fair and unbiased. Implementing bias detection techniques and corrective measures is essential for fostering equity in AI outputs.
- Leverage specialized AI TRiSM toolsets: Utilize advanced AI TRiSM toolsets designed to manage and mitigate risks associated with AI models, applications, and agents. These tools should help in maintaining trust, security, and compliance across all AI operations.
- Human-centered design: Focusing on user experience ensures that AI systems are designed with end-user needs and expectations in mind, leading to higher adoption rates and user satisfaction. By adopting a human-centered approach, AI TRiSM frameworks can ensure that AI tools are technically proficient and accessible and beneficial to all users, enhancing overall effectiveness.
- Implement diverse AI data protection solutions: Adopt varied data protection methodologies tailored to different AI use cases and components. This diversified approach helps in addressing specific security needs while ensuring comprehensive data protection.
- Interpretability vs. accuracy trade-offs: Balancing these two aspects is crucial for creating AI systems that are both understandable and effective. High accuracy with low interpretability can erode trust, while high interpretability with compromised accuracy can reduce the utility of AI systems. AI TRiSM strategies should include methodologies to assess and balance these trade-offs, ensuring that AI systems maintain an optimal balance for specific use cases.
- Implement data classification and permissioning systems: Deploy robust data classification and permissioning frameworks to maintain strict control over data access and manipulation. This ensures that enterprise policies are enforceable and data is used in compliance with legal and ethical standards.
- Balancing AI and human oversight: Maintaining a balance between AI automation and human oversight is crucial. Implementing protocols that require human intervention in critical decision-making processes can help mitigate risks associated with overreliance on AI.
- Training and infrastructure investment: Addressing technical challenges requires investing in the right skills and infrastructure. Organizations should focus on training their workforce in AI management and deploy advanced technical solutions to handle scalability and integration challenges.
- Set up an organizational unit for AI TRiSM management: Create a dedicated unit within your organization to oversee AI TRiSM. This unit should consist of stakeholders directly interested in the success of AI initiatives, ensuring comprehensive management and alignment with business goals.
- Navigating the regulatory environment: Organizations should stay informed about the latest regulatory changes and integrate regulatory risk management into their AI strategies to manage compliance effectively. Establishing a dedicated regulatory compliance team can help ensure that AI implementations adhere to all applicable laws and regulations.
- Include data protection assurances in vendor agreements: Incorporate stringent data protection and privacy terms in license agreements with vendors, especially those hosting large language models (LLMs). This will safeguard your organization against data breaches and privacy violations.
By understanding these challenges and implementing these strategies, organizations can effectively follow AI TRiSM frameworks that not only mitigate risks but also enhance the reliability and integrity of their AI systems.
Optimize Your Operations With AI Agents
Optimize your workflows with ZBrain AI agents that automate tasks and empower smarter, data-driven decisions.
The future of AI trust, risk, and security management
As AI continues to permeate various aspects of business and society, the evolution of AI TRiSM is expected to bring substantial advancements in how organizations deploy and manage AI technologies. Here’s a look at the key developments anticipated in the AI TRiSM landscape:
Advanced automation
By promoting responsible AI development and deployment, AI TRiSM helps ensure that organizations can leverage AI’s automation potential – across industries from healthcare to finance to public sector administration – while mitigating ethical and practical risks. Automation will also facilitate real-time decision-making and responses, enhancing operational agility.
Personalized experiences
AI TRiSM will enhance the capacity to deliver highly personalized experiences and solutions tailored to individual needs and preferences. This will be evident in sectors like healthcare for personalized treatment plans, in retail for customized shopping experiences, and in financial services for tailored investment advice.
Ethical and responsible AI
There will be an increased emphasis on ensuring that AI systems are ethical and responsible. This includes making AI systems transparent, accountable, and free from biases. Governance frameworks and ethical guidelines will be crucial in guiding organizations on responsible AI use, ensuring that AI acts in the interests of society at large.
Collaborative ecosystems
Collaborative efforts among tech companies, academic institutions, regulatory bodies, and industry groups will likely drive future advancements in AI TRiSM. These collaborations will aim to standardize AI TRiSM practices and share knowledge and resources, fostering a more unified approach to managing AI risks.
Market evolution and predictions
Emerging trends predict significant improvements in AI model adoption and user acceptance as organizations increasingly prioritize transparency and security in their AI deployments. Yet, the landscape will also face regulatory challenges, requiring businesses to remain agile and responsive to new laws and standards that govern AI use.
Operational challenges in AI TRiSM
The unique operational demands of AI systems require new approaches beyond traditional risk management frameworks. Organizations are increasingly relying on specialized vendors to address these needs, highlighting a trend towards specialized AI risk management solutions.
Need for enhanced AI explainability
The explainability of AI decisions remains crucial for operational transparency and accountability. Regular testing of AI models and their behaviors ensures that they perform as expected and allows for timely corrective actions when deviations occur.
Looking ahead, AI TRiSM is poised to become more integrated into the fabric of organizational strategies, helping to navigate the complexities of AI implementation while fostering an environment of innovation and trust. This strategic integration will enhance operational efficiencies and align AI deployments with broader societal and ethical considerations, shaping a future where AI is both transformative and trusted.
LeewayHertz’s approach to AI trust, risk, and security management
At LeewayHertz, we understand the complex dynamics introduced by AI technologies and prioritize robust trust, risk, and security management in all our AI initiatives. Our approach ensures that your AI deployments are secure, reliable, and aligned with ethical standards.
Strategic AI TRiSM consulting: We begin with a comprehensive assessment of your AI landscape to identify potential risks and vulnerabilities. Our experts craft customized AI strategies that focus on risk mitigation, data protection, and establishing trust across all AI operations. Our consultation allows us to tailor AI strategies that not only align with your objectives but also anticipate and mitigate potential risks associated with AI deployments. Our strategic development covers everything from risk assessment to compliance with emerging regulations.
Responsible AI development: We are dedicated to crafting AI solutions prioritizing transparency, fairness, and privacy. Our development process adheres strictly to regulatory standards, ensuring our AI systems are accountable and beneficial to society. By embedding ethical practices into our technological solutions, we strive to build trust and deliver positive societal impacts.
Advanced data security measures: Recognizing the critical importance of data security in AI, we implement stringent measures such as advanced encryption techniques, secure data storage solutions, and strict access controls. These measures protect sensitive data from unauthorized access and breaches, maintaining confidentiality and integrity.
Generative AI expertise and ethical implementation: Our proficiency in generative AI, leveraging models like GPT and Llama, enables us to create innovative solutions that adhere to ethical standards. We ensure that AI implementations are transparent, fair, and explainable, enhancing stakeholder trust and compliance.
Continuous monitoring and adversarial defense strategies: We provide ongoing monitoring and maintenance to ensure AI systems operate effectively and adapt to new challenges. Additionally, we develop strategies to protect against adversarial AI threats, incorporating cutting-edge security solutions that exceed traditional approaches.
Navigating regulatory landscapes: With awareness of global AI regulations, such as the EU AI Act, we guide your organization through the complexities of compliance. Our dedicated team ensures that your AI strategies are future-proofed against regulatory changes, safeguarding your operations against compliance risks.
By partnering with LeewayHertz, you leverage a team dedicated to establishing and maintaining the highest standards of trust, security, and risk management in AI. Our comprehensive AI TRiSM services ensure that your AI initiatives deliver the intended benefits while safeguarding against evolving threats and maintaining regulatory compliance.
Endnote
As we conclude our exploration of AI Trust, Risk, and Security Management (AI TRiSM), it’s clear that navigating the complexities of AI integration demands diligence, foresight, and a commitment to ethical principles. Implementing AI TRiSM enables organizations to harness the transformative power of AI while managing associated risks and ensuring compliance with ethical standards. This proactive approach not only enhances operational efficiencies but also fosters trust among stakeholders, ensuring that AI advancements contribute positively to our technological landscape. Looking ahead, the strategic implementation of AI TRiSM will continue to play a pivotal role in shaping the responsible deployment and evolution of AI technologies across industries.
Ready to ensure your organization’s AI initiatives are secure, ethical, and effectively managed? Explore how our AI consulting services can empower your business to implement robust AI Trust, Risk, and Security Management strategies. Our team of experts is dedicated to helping you confidently navigate the complexities of AI integration.
Start a conversation by filling the form
All information will be kept confidential.
Insights
AI in banking and finance: Use cases, applications, AI agents, solutions and implementation
AI in banking and finance offers various opportunities for process optimization, risk management, and customer engagement. One of the key areas where AI demonstrates its potential is in data analysis.
AI in store replenishment: Navigating the future of retail operations
The integration of AI in store replenishment processes brings forth numerous benefits that significantly enhance operational efficiency, customer satisfaction, and overall business performance
AI for master data management: Use cases and benefits
In the intricate world of data management, the alliance between AI and MDM has surfaced as a vital driver for transforming data into actionable insights and informed decision-making.