How AI helps in Early Infectious Disease Surveillance?
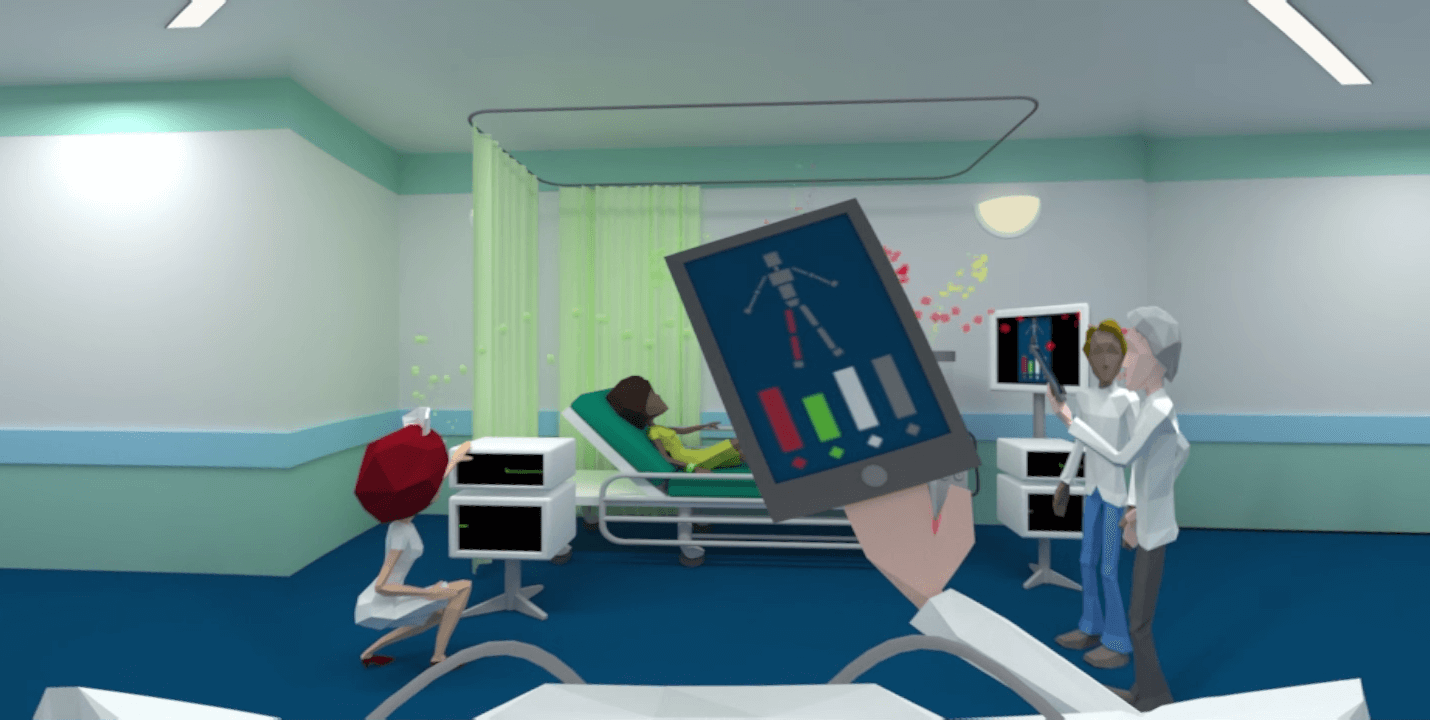
Identifying an infectious disease outbreak is crucial for both the initiation of public health intervention measures and timely informing of the general public and government agencies. Since John Snow studied maps carefully to investigate London’s Cholera Outbreak in the 19th Century, technology started to aid disease surveillance efficiently. AI, combined with its capabilities in data processing and predictive modeling, is transforming infectious disease surveillance to predict early diseases and prevent millions of lives
The emergence of novel infectious diseases is linked to human factors, including travel, trade, population density, and ecological factors. But various technologies are becoming available for the rapid identification of pathogens and accurate monitoring of infectious disease activity.
More and more scientists are investigating ways to use AI for predicting the spread of infectious diseases before they occur. We will discuss different ways of infectious disease surveillance that can help detect early outbreaks and prevent many lives.
We shall discuss the following topics in the article:
- What are the methods of Infectious Disease Surveillance?
- How is AI transforming Infectious Disease Surveillance?
What are the methods of Infectious Disease Surveillance?
1. Internet-based Surveillance
Internet-based surveillance systems can monitor infectious diseases economically and logistically. The demand for these systems has grown tremendously, with increasing global internet penetration. Internet-based Surveillance can be categorized into two methods: Event-based Surveillance and Real-time Surveillance.
- Event-based SurveillanceTraditional passive surveillance depends on routine reporting by healthcare facilities regarding diseases and events. Such type of surveillance needs the presence of a public health network that is quite expensive and results in a delay of around two weeks from data acquisition to propagation.Using advanced computational science, event-based surveillance method has emerged as a way to gather and analyze unstructured data from various sources, including internet-based searches, news reports and social media. The majority of data related to infectious diseases derive from sources like the internet and media reports.The Global Public Health Intelligence Network (GPHIN) is one of the significant event-based surveillance systems that scans a wide range of sources such as electronic discussion forums, websites, online newspapers, news reports for disease events and outbreaks rumors. GPHIN can process around 300 news items per day, but 1/4th of them could be irrelevant or duplicate data. In some of the event-based systems, captured raw data is investigated, authenticated by official sources, and assessed for epidemiologic significance.
GPHIN was highlighted during the SARS Outbreak in November 2002 and February 2003 when it released the first alert of unusual respiratory illness in Guangdong Province, China. Some of the other widely used event-based systems are HealthMap, EpiSPIDER (Semantic Processing and Integration of Distributed Electronic Resources), HealthMap, and BioCaster. These systems use text mining or data processing to refine and sort articles, increasing information relevance.
In countries with non-existent national public health surveillance systems, event-based surveillance methods can be used to provide real-time information on local disease outbreaks.
- Real-time Surveillance using Web-based ToolsAnalyzing health-related search engine queries can help track local infectious disease activity. Google Flu Trends was one of the first web-based tools designed and built to monitor influenza activity in real-time. Web-based methods depend on the fact that the number of people searching for information on the web related to influenza is correlated with the number of people having influenza symptoms.Health-related queries submitted to other search engines have also been examined to predict influenza intensity. As a large volume of search queries is required for web-based surveillance tools, it can work more effectively when implemented to diseases with moderate to high prevalence.
2. Surveillance using Remote Sensing Technology
Remote Sensing Technology can be used to monitor environmental changes that help predict infectious diseases. Satellite imaging gathers information about sea temperature and surface height and chlorophyll levels using which researchers built an environmental model and predicted the incident rate of a cholera outbreak in Bangladesh. Remote sensing methodology not only helps in predicting water-borne diseases, but it also helps in tracing vector activity such as the distribution of the Anopheles species that drove Malaria transmission in Africa. But vector-borne disease outbreaks are challenging to predict because of the dynamics of human behavior and vector ecology.
Monitoring climatologic parameters and soil and vegetable indices closely are essential to assess changes that can contribute to infectious disease outbreaks. Using all that information in predictive mathematical modeling can generate early warnings to aware public health interventions, resulting in epidemic mitigation.
3. Surveillance using Communication
Communication channels are also one of the most effective ways to predict infectious disease outbreaks. Analysis of data collected through social media applications offers new challenges and opportunities in disease surveillance. The Chicago Department of Public Health launched a social media censoring program for monitoring of food-borne diseases or illnesses. They use an algorithm that can detect and respond to data entries on Twitter related to food poisoning.
Mobile phone data is also being used to track human movements to understand the transmission dynamics and paths of importation of an infectious disease from one region to another. Researchers in Sri Lanka built a mobile phone-based surveillance system for monitoring animal health.
Mobile phones facilitate 2-way sharing of information and can be used for gathering data that can serve surveillance purposes and provide essential public health information to the public.
4. Search-term Surveillance
In Search-Term Surveillance, efforts are emphasized on monitoring symptoms or other evidence related to a disease that can be recognized before the diagnosis is confirmed. An outbreak investigation is triggered when specific health-related outcomes exceed expected levels. Examples of search-term surveillance include monitoring increases in emergency department visits, increases in the sales of over-counter medicines, and the volume of calls to health advice centers.
This surveillance uses keyword-searching and web-based clickstreams across the Internet. Eysenbach demonstrated it for the monitoring of Influenza in Canada.
While most of the above existing disease surveillance systems depend on basic statistical methods, we believe that AI can bring a paradigm shift in the field of disease surveillance due to the increase in complexity and quantity of available data sources.
LeewayHertz AI Development Services
Transform your business processes with Smart AI applications
How is AI transforming Infectious Disease Surveillance?
AI-based Infectious Disease Surveillance System
When any mysterious disease pops up, it can be challenging for public health officials and governments to collect information quickly and generate a response. Artificial intelligence technology can help experts identify anomalies that can create a potential epidemic by mining online content and news reports automatically. Though the process seems complicated, the implementation of predictive modeling could provide a way to get rid of infectious diseases.
Automated surveillance systems can classify vast amounts of heterogeneous data for abnormalities and patterns. Recently, one of the Canadian firms, Bluedot, identified the CoronaVirus outbreak before it was officially declared. Bluedot conducts automated infectious disease surveillance using AI and helps hospitals prevent their staff and citizens; governments protect their citizens and companies protect their employees and customers.
Kamran Khan, Bluedot’s founder and CEO and an infectious disease physician, described the Bluedot’s early-warning system in an interview that they use machine learning and natural language processing to analyze about 100,000 articles in 65 languages every day to track over 100 infectious diseases.
Using this data, Bluedot understands when to alert its clients about the spread and presence of an infectious disease. It also uses other data, including flight routes and traveler itinerary information, that provides the company with hints about how a disease is likely to spread.
The Bluedot model aims to provide information to healthcare workers as quickly as possible so that they could diagnose and if required, isolate contagious and infectious people before it becomes an outbreak. Bluedot system also uses an array of data, including information about the area’s temperature, area’s climate and local livestock to predict if someone infected with a disease is likely to cause an outbreak in a specific area. In 2016, Bluedot was able to predict the Zika virus in Florida six months before it occurred.
Similarly, another epidemic-monitoring company, Metabiota, identified that South Korea, Japan, Taiwan and Thailand would have the highest risk of seeing the CoronaVirus more than a week before the cases were reported in these countries by looking at the flight data. Metabiota also uses natural language processing to identify online reports about a potential disease. Based on the factors such as illness’s symptoms, availability of treatment and mortality rate, Metabiota can also predict the risk of a disease’s spread that can lead to political and social disruption.
Beyond Infectious Disease Surveillance, AI can also keep officials and epidemiologists informed when a disease pops up. Researchers have developed AI-based models that can forecast Zika Virus outbreaks in real-time and can tell how physicians respond to potential infections. AI can also be used to train how public health officials should share out resources during a crisis.
Moreover, AI also assists with researching new vaccines and drugs, detecting and tackling rare diseases. A significant benefit of using AI is the ability to predict and monitor outbreaks that are happening around the clock. Since humans can get distracted easily, we can have machines to keep eyes on everything that’s going around with the help of Artificial Intelligence.
Our AI experts can build a disease surveillance system using our expertise in AI and IoT that can fetch the data from diverse sources and generate an outcome to predict the disease outbreak.
Start a conversation by filling the form
All information will be kept confidential.
Insights
Generative AI in internal audit: Importance, use cases, implementation strategies and future trends
Generative AI significantly impacts internal audit practices by enhancing risk evaluation, monitoring compliance, and boosting operational efficiency.
Generative AI in hospitality: Integration, use cases, challenges, and future outlook
Generative AI improves customer experiences by offering personalized services that boost guest loyalty and satisfaction.
The Hackett Group Announces Strategic Acquisition of Leading Gen AI Development Firm LeewayHertz
The Hackett Group, Inc. (NASDAQ: HCKT), a leading Gen AI strategic consultancy and executive advisory firm today announced the acquisition of LeewayHertz, a highly recognized provider of advanced Gen AI solutions.