AI in trade promotion optimization: Use cases, benefits and implementation
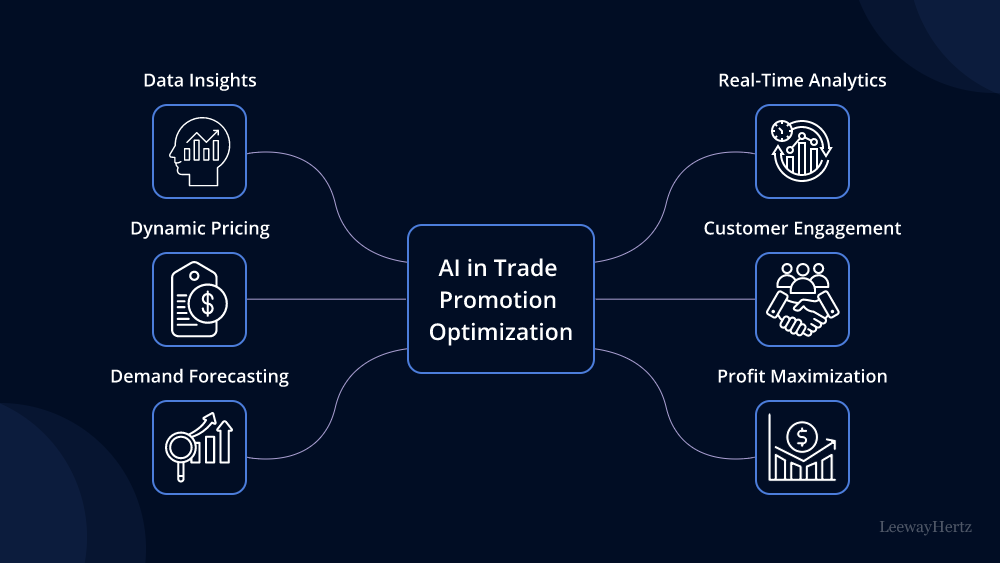
Every decision matters in today’s fast-paced business landscape, especially when it comes to promotions. Your promotion strategies can catapult your brand to unprecedented success or leave you lost amidst the clamor of competitors. Envision a scenario where you could devise promotions that not only capture immediate attention but also guarantee a substantial positive effect on your financial performance. This is precisely where the application of Artificial Intelligence (AI) steps in, specifically within the domain of Trade Promotion Optimization (TPO), merging innovation with tangible, impactful outcomes.
A research study conducted by McKinsey revealed that even a 1% enhancement in price realization could result in a notable 8.7% surge in operating profits. Now, picture a scenario where each promotion you introduce is not a risk but a strategic step toward success. AI has ushered in this new era, where data-driven strategies take the lead and decisions are backed by deep insights, not instincts. TPO is not just about increasing sales; it’s about creating a well-tailored strategy that connects with your target audience. And AI isn’t just a buzzword; it’s your trusted partner in decoding customer preferences, predicting trends, and fine-tuning strategies for optimal outcomes. For those seeking to harness the potential of AI in trade promotion optimization, the integration of AI and TPO is your gateway to promotions that attract and yield conversions.
This article explores how AI reshapes trade promotion optimization, reinventing how promotions are conceived, executed, and measured. Additionally, we will delve into real-life examples of organizations leveraging AI for TPO, uncover best practices for implementing AI-driven strategies, and offer insights into the future of this dynamic landscape.
- Understanding trade promotion optimization
- The multifold benefits of implementing trade promotion optimization
- Key challenges faced by organizations during TPO
- The trade promotion optimization process
- Streamlining trade promotion optimization with generative AI
- Benefits of AI in trade promotion optimization
- Advanced AI algorithms and models for trade promotion optimization
- AI use cases in trade promotion optimization
- Real-life examples: Organizations leveraging AI for TPO
- LeewayHertz’s AI development services for trade promotion optimization
- Best practices for implementing AI in promotion optimization
- The future of trade promotion optimization
Understanding trade promotion optimization
Trade promotion optimization refers to the process of using advanced analytics, algorithms, and data-driven insights to enhance the planning, execution, and evaluation of trade promotions. The goal is to maximize the Return on Investment (ROI) from promotional activities while minimizing waste and inefficiencies. TPO takes a comprehensive approach, considering factors such as pricing, timing, promotion duration, product assortment, and targeting to create a well-rounded strategy that resonates with both consumers and retailers.
TPO leverages historical sales data, market trends, consumer behavior insights, and other relevant information to create more accurate forecasts and develop optimal promotion plans. Unlike traditional methods that rely on intuition and limited data, TPO harnesses the power of artificial intelligence and machine learning to uncover patterns, identify correlations, and make data-driven recommendations for promotional activities.
The multifold benefits of implementing trade promotion optimization
Implementing trade promotion optimization offers various benefits for consumer goods companies and retailers. Here are some of the key advantages:
Enhanced ROI: TPO’s data-driven approach helps companies allocate their promotional budgets more effectively. By analyzing historical data and predicting consumer behavior, TPO ensures that promotional activities are directed toward products, channels, and timeframes that yield the highest return on investment. This leads to increased revenue and profits.
Improved decision-making: TPO provides actionable insights based on accurate demand forecasts and scenario simulations. This empowers decision-makers to make informed choices about pricing, product assortment, promotion duration, and other critical aspects of trade promotions. It reduces guesswork and increases the likelihood of successful outcomes.
Effective resource allocation: With TPO, companies can allocate their resources, such as marketing spend and inventory, in a more targeted manner. This prevents overstocking or understocking issues, reduces wastage, and ensures that products are available when and where customers expect them.
Optimized promotion plans: TPO’s algorithms consider multiple variables, such as pricing, discounts, and competitor actions, to generate promotion plans that maximize sales and profits. These plans are tailored to align with the company’s objectives, customer preferences, and market dynamics.
Real-time adaptability: As TPO systems incorporate real-time data and insights, companies can adjust their promotional strategies on-the-fly to respond to changing market conditions, competitor actions, and unexpected events. This agility helps maintain competitiveness and relevance.
Collaborative relationships: TPO encourages collaboration between manufacturers and retailers. By sharing insights and data, both parties can co-create promotion plans that benefit both sides. This collaborative approach strengthens relationships and builds trust between trading partners.
Reduced risk: Through scenario planning and predictive modeling, TPO allows companies to evaluate the potential outcomes of different promotional strategies before implementation. This minimizes risks associated with uncertain outcomes and helps avoid costly mistakes.
Competitive edge: TPO enables companies to respond more swiftly to market trends and shifts in consumer behavior. By offering relevant and enticing promotions, businesses can secure a competitive advantage and expand their market presence.
Personalization: TPO leverages AI and data analytics to segment customers according to their preferences and behaviors. This enables companies to tailor promotions to specific customer segments, delivering personalized offers that resonate with individual consumers.
Comprehensive insights: TPO generates valuable insights about the effectiveness of different promotion strategies over time. This information informs future planning and allows companies to refine their promotional efforts for better results continuously.
Improved shelf placement and merchandising: TPO considers shelf placement and product merchandising as part of the promotional strategy. This ensures that products are positioned optimally for maximum visibility and consumer engagement.
Enhanced customer experience: Companies can enhance the overall customer experience by offering relevant and appealing promotions. Satisfied customers become loyal clients, driving long-term business success.
Trade promotion optimization provides a strategic advantage to businesses by leveraging data and analytics to optimize promotional efforts. The benefits include increased ROI, improved decision-making, efficient resource allocation, real-time adaptability, collaborative relationships, reduced risk, and more. As companies continue to embrace TPO, the potential for enhancing profitability and customer satisfaction remains significant.
Optimize Your Operations With AI Agents
Optimize your workflows with ZBrain AI agents that automate tasks and empower smarter, data-driven decisions.
Key challenges faced by organizations during TPO
Organizations entering trade promotion optimization often face challenges that affect successful implementation and benefits realization. These challenges include:
Data quality and availability: TPO heavily relies on accurate and comprehensive data from various sources, including historical sales, promotions, market trends, and external factors. Organizations often struggle with data inconsistencies, inaccuracies, and gaps, undermining the effectiveness of TPO algorithms and insights.
Data not in proper shape & form: Decision-makers still use spreadsheets or subpar TPO tools with limited insights. Accessing data from multiple systems complicates data consumption and integration into decision-making workflows.
High dependence on finding a skilled crew: Accessing data and generating custom reports often requires IT or MIS teams. Business users can’t customize dashboards independently, delaying obtaining fresh or updated reports.
Missing/difficult what-if analysis: Many trade promotion strategies involve forecasting “what-if” scenarios, but some tools lack in-depth analysis. Generic analyses provided may not offer substantial business value.
New plans are based on incomplete insights: Ineffective data harmonization and predictive intelligence hinder measuring promotion effectiveness. This leads to a lack of data-driven optimization for upcoming promotions, relying on past experiences, instincts, or partial intelligence.
Integration complexity: Integrating TPO systems with existing IT infrastructure, including ERP and CRM systems, can be complex and time-consuming. Compatibility issues and data synchronization between different platforms may hinder the seamless flow of information required for effective optimization.
Data privacy and security: TPO uses sensitive data, including sales figures and customer behavior. Ensuring compliance with data protection regulations while maintaining data security is critical to avoid legal and reputational risks.
Customization and scalability: Organizations have unique business processes and strategies. Tailoring TPO solutions to meet specific requirements can be resource-intensive, and ensuring the scalability of these customized solutions as the business grows is a challenge.
The complexity of market dynamics: Markets are dynamic, with constantly changing consumer preferences, competitive landscapes, and economic conditions. TPO solutions must be flexible enough to adapt to these changes in real time.
Communication with retailers: Collaborating with retailers requires effective communication, data sharing, and negotiation. Differences in goals, priorities, and expectations can complicate the process of co-creating optimized promotion plans.
Bias and fairness: Algorithms used in TPO may inadvertently introduce bias, leading to unfair promotion strategies that favor certain customer segments over others. Ensuring fairness and mitigating bias is a critical ethical consideration.
Continuous monitoring and improvement: TPO is not a one-time implementation; it requires continuous monitoring, evaluation, and refinement of algorithms and strategies to adapt to changing market conditions and ensure long-term success.
By harnessing the capabilities of AI, organizations can effectively overcome the challenges associated with trade promotion optimization, streamline decision-making processes, and achieve more accurate, data-driven results.
The trade promotion optimization process
Trade promotion optimization has entered a new era of efficiency and effectiveness with the integration of artificial intelligence. AI has redefined the way businesses plan, execute, and evaluate promotional activities, allowing for more accurate forecasts, personalized targeting, and data-driven decision-making.
The TPO process
The TPO process entails a series of strategic steps to plan, execute, and evaluate trade promotions to achieve optimal return on investment and business outcomes. This iterative process includes essential stages such as data collection and integration, demand forecasting, promotion planning, utilization of optimization algorithms, resource allocation, promotion execution, real-time monitoring, performance assessment, continual learning, and adaptation. This structured approach empowers businesses to navigate the complexities of promotions while maximizing their impact.
Key aspects of AI integration in trade promotion optimization
Data collection and preprocessing
AI systems gather and preprocess data from various sources, including historical sales, market trends, and customer behaviors. This data forms the foundation for AI-driven TPO, enabling accurate insights and predictions.
Machine learning algorithms for demand forecasting
AI employs machine learning algorithms to predict future demand accurately. These forecasts guide promotion planning and help optimize strategies for maximum ROI.
AI-driven customer segmentation
AI segments customers based on purchasing behaviors and preferences through advanced clustering algorithms. This segmentation enhances engagement and conversion rates by tailoring promotions to specific customer groups.
Dynamic pricing strategies
AI enables dynamic pricing by adapting to market changes, competitor actions, and demand fluctuations. This responsive pricing strategy maximizes revenue while staying competitive.
Real-time adaptability using AI
AI continuously monitors data sources, recommending adjustments to ongoing promotions in response to unexpected events or trends. This adaptability minimizes missed opportunities.
Personalization and customer insights
AI uncovers purchasing patterns and preferences for individual customers, enabling hyper-personalized promotions. Recommender systems leverage AI to suggest products, driving cross-selling and upselling opportunities.
Addressing bias and fairness with AI
AI ensures unbiased decisions and prevents discrimination across customer segments. Transparent algorithms identify and rectify unintended biases in the promotion planning process.
From data collection to personalized targeting, from demand forecasting to real-time adaptability, AI enables businesses to create promotion strategies that are data-driven, responsive, and customer-centric. As industries continue to embrace AI in TPO, the potential for elevating the effectiveness and impact of promotions remains unparalleled.
Streamlining trade promotion optimization with generative AI
Generative AI is transforming trade promotion optimization, empowering businesses to plan, execute, and analyze promotional campaigns with unprecedented effectiveness. By harnessing the power of advanced analytics and real-time insights, GenAI streamlines workflows, enhances decision-making and maximizes the ROI of trade promotions. Let’s explore the key ways GenAI is transforming trade promotion optimization:
Strategic promotion planning
Steps involved | Sub-steps involved | Role of GenAI |
Promotion planning |
|
|
Strategy development |
|
|
Trade promotion analysis
Steps involved | Sub-steps involved | Role of GenAI |
Data collection |
|
|
Data analysis |
|
|
Promotion design |
|
|
Implementation planning |
|
|
Executing promotions
Steps involved | Sub-steps involved | Role of GenAI |
Launch promotions |
|
|
Execute promotions |
|
|
Engage customers |
|
|
Monitoring promotions
Steps involved | Sub-steps involved | Role of GenAI |
Set monitoring parameters |
|
|
Performance monitoring |
|
|
Reporting and optimization |
|
|
Benefits of AI in trade promotion optimization
In the fast-paced realm of trade promotion optimization, where the success of promotional strategies hinges on accuracy and adaptability, the integration of AI brings forth a plethora of substantial benefits. By leveraging advanced algorithms and data-driven insights, AI significantly impacts the landscape of promotional planning and execution. Here are the key advantages of integrating AI in trade promotion optimization:
Micro-segmentation strategy enhancement
AI facilitates the seamless alignment of global strategies with local tactics, making the complex task of combining different priorities across categories, markets, and brands considerably easier. With its ability to assign optimal strategies to micro-segments, AI ensures a harmonious synthesis of overarching objectives and daily promotional activities. This leads to targeted and effective campaigns at various levels, from individual retailers to broader regions.
Accurate and timely insights
Utilizing real-time point-of-sale data, AI processes and analyzes information swiftly, creating highly specific and relevant promotional offers. This means that promotions can be deployed promptly, precisely when and where they are needed most. The speed and accuracy of AI-generated insights allow businesses to seize opportunities and respond to market dynamics swiftly.
Pattern identification with ML and AI
Incorporating ML elevates promotional planning by identifying patterns and trends from historical data. Whether analyzing past promotions or handling new scenarios, ML models generate accurate predictions. This empowers businesses to fine-tune their strategies and forecasts according to the latest category performance trends.
Precision in promotional spend allocation
AI’s predictive capabilities provide unparalleled accuracy in forecasting promotions’ performance. By considering multiple variables and their potential impact, AI assists retailers and CPGs in understanding and optimizing their promotional and advertising investments. Additionally, AI-driven algorithms automate the allocation of promotions, optimizing circular design processes and ensuring optimal in-store foot traffic.
Enhanced returns on promotional channels
CPGs and retailers rely on trade fund dollars to run promotions. AI’s optimization prowess helps strike a balance between meeting retailer category targets and allocated CPG targets. By suggesting alternative promotion tactics that align with desired margins, AI empowers category managers to strategize efficiently, maximizing investment returns and achieving mutually beneficial outcomes.
Data-informed insights and recommendations
AI serves as a bridge across different stages of TPO, from forecasting to evaluation. Its ability to analyze complex scenarios and extract valuable insights from vast datasets is unparalleled. By effortlessly uncovering hidden patterns and suggesting actionable recommendations, AI empowers businesses to make well-informed decisions for effective promotional activities.
Integrating AI in trade promotion optimization brings multifaceted benefits that redefine the way promotional strategies are conceived and executed. From precise micro-segmentation strategies to optimizing promotional spending and maximizing returns on investments, AI empowers enterprises to navigate the intricacies of modern retail environments with confidence and achieve unparalleled success.
Optimize Your Operations With AI Agents
Optimize your workflows with ZBrain AI agents that automate tasks and empower smarter, data-driven decisions.
Advanced AI algorithms and models for trade promotion optimization
Several AI models are used for trade promotion optimization to analyze data, predict outcomes, and optimize promotional strategies. Here are some of the commonly used AI models and algorithms in trade promotion optimization:
Machine learning algorithms:
- Regression models: Linear regression, polynomial regression, and other regression techniques are used for demand forecasting. They analyze historical sales data to predict future demand based on various influencing factors.
- Time series analysis: Time series models like ARIMA (AutoRegressive Integrated Moving Average) are used to capture seasonality and trends in sales data, aiding in accurate demand forecasting.
- Decision trees: Decision trees can help segment customers based on factors like purchasing behavior, allowing businesses to tailor promotions to different customer groups.
- Random forest: Random forest algorithms combine multiple decision trees to improve prediction accuracy and handle complex interactions between variables.
- Gradient boosting: Gradient boosting algorithms like XGBoost and LightGBM can handle non-linear relationships in data, making them effective for demand forecasting and customer segmentation.
Neural networks:
- Feedforward neural networks: These multi-layered networks are used for complex pattern recognition tasks. In TPO, they can analyze diverse data sources to predict demand, optimize pricing, and identify customer segments.
- Recurrent Neural Networks (RNNs): RNNs are useful for time series data, as they can capture sequential dependencies and seasonality patterns for more accurate demand forecasting.
- Long Short-term Memory (LSTM): A type of RNN, LSTMs are effective for long-range dependencies and can be employed to predict demand trends over extended periods.
Clustering algorithms:
- K-means clustering: K-means is used to segment customers based on purchasing behavior, allowing for targeted and personalized promotion strategies.
- Hierarchical clustering: This algorithm creates a hierarchical tree of clusters, aiding in identifying subgroups within customer segments.
Association rule mining:
- Apriori algorithm: Apriori identifies frequent itemsets in transaction data, revealing which products are often purchased together. This informs cross-selling and bundling strategies.
Recommender systems:
- Collaborative filtering: This technique recommends products based on the preferences of similar customers. It’s used for personalized promotions by analyzing historical behaviors.
- Content-based filtering: Content-based filtering recommends products similar to those a customer has shown interest in, using attributes like product descriptions and features.
- Hybrid recommender systems: These combine collaborative and content-based filtering for more accurate and diverse recommendations.
Optimization algorithms:
- Genetic algorithms: Genetic algorithms optimize promotion strategies by evolving and selecting the best-performing combinations of promotional elements over time.
- Simulated annealing: This optimization technique is used to fine-tune pricing strategies and allocation of promotional resources.
Natural Language Processing (NLP):
- Sentiment analysis: NLP techniques are used to analyze customer reviews and social media sentiments, providing insights into customer perceptions that can influence promotion strategies.
Ensemble methods:
- Ensemble learning: Ensemble methods combine multiple models to improve prediction accuracy and generalization. Techniques like bagging, boosting, and stacking can be applied to TPO for more robust insights.
Each AI model and algorithm brings unique capabilities to trade promotion optimization, enabling businesses to extract valuable insights from data, make accurate predictions, and optimize their promotion strategies for maximum impact. The choice of model and algorithms to be used depends on the specific TPO objectives and the nature of the data being analyzed.
AI use cases in trade promotion optimization
Here are some AI use cases in trade promotion optimization that showcase the practical applications of AI techniques to enhance promotional strategies:
Demand forecasting and planning
AI uses historical sales data, market trends, and external factors (such as holidays or economic indicators) to predict future demand accurately. By analyzing patterns and correlations in this data, AI algorithms generate forecasts that guide businesses in planning their promotions. For instance, AI can make predictions about increased demand for specific products during a holiday season, allowing businesses to allocate resources and adjust promotion strategies accordingly.
Dynamic pricing optimization
AI-driven dynamic pricing adapts prices in real-time based on factors like competitor prices, demand fluctuations, and market trends. Machine learning algorithms analyze historical sales data and current market conditions to determine the optimal price for a product. For instance, if a competitor lowers their prices, AI-powered recommendations can help you adjust your prices to remain competitive while maximizing revenue.
Customer segmentation and personalization
AI analyzes customer behaviors, preferences, and demographics to segment them into distinct groups. These segments enable personalized promotion targeting. Consider the scenario where AI identifies a specific group of health-conscious customers. In this case, AI can be employed to tailor promotions aimed at this segment. These promotions can be strategically designed to highlight and prioritize healthier product options by leveraging AI-driven insights. This approach is likely to result in increased customer engagement and higher conversion rates within this health-conscious customer segment.
Optimizing promotion duration and timing
AI analyzes historical sales data and identifies patterns regarding when customers are most active. This helps determine the optimal duration and timing of promotions. For instance, if sales typically peak during weekends, utilizing AI techniques could lead to the recommendation of launching promotions on Fridays to capitalize on the increased customer activity.
Real-time adaptive strategies
AI constantly monitors real-time data streams, allowing it to adapt ongoing promotions based on changing market conditions, competitor actions, and customer behavior. If a sudden trend emerges or a competitor launches a new promotion, AI can provide recommendations to adjust your ongoing promotions accordingly.
Promotion mix optimization
AI analyzes past promotional campaigns to understand the effectiveness of different types of promotions. By assessing the impact of discounts, bundles, and other strategies on sales and profitability, AI helps businesses allocate resources to the most effective promotion types.
Scenario simulations and what-if analysis
AI runs simulations to predict the outcomes of different promotion strategies under various conditions. This allows businesses to explore hypothetical scenarios and understand potential risks and rewards. For example, AI can simulate the impact of offering a 20% discount versus a buy-one-get-one promotion.
Optimizing the allocation of resources
AI optimizes the allocation of promotional resources such as budget, manpower, and time. By considering constraints and objectives, AI helps businesses distribute resources to maximize the impact of promotions and achieve the desired outcomes.
Market basket analysis
AI analyzes customer purchase patterns to identify products frequently purchased together. This information informs cross-selling and bundling strategies. For instance, if customers often buy chips with soda, AI might recommend offering a bundled promotion for both items.
Promotion effectiveness analysis
AI evaluates the effectiveness of past promotions by comparing sales data during promotion periods to non-promotion periods. This analysis provides insights into which promotions lead to significant sales increases and which ones might need adjustment.
Competitor analysis and response
AI monitors competitor activities, including pricing and promotions. By analyzing these factors, AI provides recommendations on how to respond effectively. For example, if a competitor introduces a buy-one-get-one promotion, AI might suggest offering a similar promotion to stay competitive.
Supply chain optimization
When planning promotions, AI considers supply chain factors like production capacity and distribution logistics. By aligning promotions with supply chain capabilities, AI ensures that promotions do not lead to stockouts or other supply chain disruptions.
These AI use cases demonstrate how AI can optimize trade promotions, boost customer engagement, and yield improved business results. By leveraging these techniques, businesses can create more effective, data-driven promotion strategies that resonate with their target audience.
Optimize Your Operations With AI Agents
Optimize your workflows with ZBrain AI agents that automate tasks and empower smarter, data-driven decisions.
Real-life examples: Organizations leveraging AI for TPO
Here are a few real-life examples of organizations that have leveraged AI for trade promotion optimization:
Procter & Gamble (P&G)
P&G, a global consumer goods company, utilizes AI to optimize their promotion strategies. They employ machine learning algorithms to analyze massive amounts of historical sales and promotional data. By identifying patterns and correlations, P&G gains insights into which promotions are most effective for different products and regions. This AI-driven approach helps P&G make more informed decisions about their promotion plans, resulting in better allocation of resources and improved sales outcomes.
Nestlé
Nestlé, another prominent player in the consumer goods industry, employs AI to optimize their trade promotions. They use machine learning algorithms to analyze sales data, market trends, and external factors that impact consumer behavior. By doing so, Nestlé can predict demand more accurately, leading to better inventory management and optimized promotion strategies. This approach has helped Nestlé improve the effectiveness of their promotions and reduce unnecessary costs.
Walmart
Walmart, a leading retail giant, uses AI to optimize pricing and promotions across its stores. They employ machine learning algorithms to analyze factors such as historical sales data, competitor pricing, and market trends. By continuously adjusting prices and promotions in real time, Walmart aims to offer customers competitive prices while maximizing their own revenue. This AI-driven dynamic pricing strategy helps Walmart maintain its position as a price leader in the retail industry.
Amazon
Amazon, a trailblazer in e-commerce, utilizes AI to personalize promotions for its customers. They leverage recommendation algorithms that analyze customer browsing and purchasing behaviors to suggest products tailored to each individual. Additionally, Amazon employs AI to optimize pricing strategies, offering dynamic prices that respond to market changes and customer preferences. This approach enables Amazon to enhance customer experience and drive higher sales volumes.
Coca-Cola
Coca-Cola uses AI to optimize its trade promotion strategies, particularly for its diverse range of beverages. They employ machine learning algorithms to analyze sales data, historical promotional outcomes, and external factors like weather patterns. Coca-Cola can tailor their promotion plans to maximize impact by understanding how different promotions perform under specific conditions. This AI-driven approach has allowed Coca-Cola to improve its demand forecasting accuracy and enhance its promotions’ effectiveness.
These real-life examples demonstrate how various industries, including consumer goods, retail, and e-commerce, are leveraging AI to optimize their trade promotion strategies. By harnessing the power of AI-driven insights, these organizations can make more informed decisions, enhance customer engagement, and achieve better outcomes in an increasingly competitive market landscape.
LeewayHertz’s AI development services for trade promotion optimization
At LeewayHertz, we excel in creating tailored AI solutions to address the unique needs of trade promotion optimization. Our strategic AI/ML consulting empowers companies to leverage artificial intelligence for more effective decision-making, enhanced customer engagement, and optimized promotional strategies, ensuring maximum return on investment.
Our expertise in developing Proof of Concepts (PoCs) and Minimum Viable Products (MVPs) allows companies to preview the potential impacts of AI tools in real-world scenarios. This approach ensures that the solutions are not only effective but also specifically customized to meet the distinctive needs of trade promotion optimization, providing a clear path to achieving maximum efficiency and return on investment.
Our work in generative AI transforms routine tasks such as report generation, content generation and data management in trade promotion optimization. Automating these processes enables teams to focus on more strategic roles, driving greater efficiency and effectiveness in promotional strategies.
By fine-tuning large language models to the specific nuances of trade-related terminology and client interactions, LeewayHertz enhances the accuracy and relevance of AI-driven communications and analyses, ensuring more precise and impactful trade promotional strategies.
Additionally, we ensure these AI systems integrate seamlessly with existing technological infrastructures, enhancing operational efficiency and decision-making in trade promotion optimization.
Our AI solutions development expertise
AI solutions development for trade promotion optimization typically involves creating systems that enhance decision-making, automate routine tasks, and personalize promotional strategies. These solutions integrate key components such as data aggregation technologies, which compile and analyze sales and market information from diverse sources. This comprehensive data foundation supports predictive analytics capabilities, allowing for forecasting market trends and consumer behavior that guide strategic decisions. Additionally, machine learning algorithms tailor promotional strategies to specific customer segments, ensuring that each campaign’s unique goals and target demographics are considered. These solutions often cover campaign management, market analysis, sales forecasting, regulatory compliance, and customer relationship management.
Overall, AI solutions in trade promotion optimization aim to maximize promotional effectiveness, improve efficiency, and enhance the customer experience.
AI agent/copilot development for trade promotion optimization
LeewayHertz builds custom AI agents and copilots that enhance various operations associated with trade promotion optimization, enabling companies to save time and resources while facilitating faster decision-making. Here is how they help:
Promotional analysis:
- Performing sales data analysis and generating promotional performance reports.
- Identifying potential promotional opportunities based on predefined criteria or rules.
- Analyzing market trends by processing historical and real-time data, helping to predict future market movements.
Customer engagement:
- Analyzing customer data and past interactions to provide personalized promotional offers and recommendations.
- Automating routine communication tasks like promotion reminders and updates.
- Offering 24/7 virtual assistance to answer customer queries and provide basic information about promotions.
Compliance and regulation monitoring:
- Automating regulatory document analysis, ensuring compliance with industry regulations.
- Monitoring promotions for compliance with predefined rules and policies.
- Automating documentation and reporting processes.
- Flagging any potential compliance violations or discrepancies.
Process automation:
- Streamlining tasks such as data entry and report generation.
- Automating data validation and verification tasks.
- Automating the setup and execution of promotional campaigns.
Promotional planning:
- Gathering and analyzing data from diverse sources gives marketers a holistic view of promotional performance.
- Customizing promotional plans based on individual campaign goals, market conditions, and customer behavior, ensuring personalized and relevant offers.
- Providing marketers with real-time insights into market conditions and promotional performance, supporting timely and informed decision-making.
Resource allocation and optimization:
- Recommending basic resource allocation strategies for promotions based on predefined models or rules.
- Identifying imbalances in promotional spend and suggesting reallocation actions within defined thresholds.
Fraud detection:
- Monitoring promotional activities for predefined patterns or rules associated with potential fraud.
- Flagging suspicious activities based on predefined criteria or models.
Marketing and content generation:
- Generating personalized marketing communications or promotional materials based on templates or structured data inputs.
- Assisting with content creation for websites, social media, and marketing campaigns within defined parameters.
Customer segmentation and targeting:
- Analyzing customer data to segment audiences based on predefined criteria (e.g., demographics, purchase history, preferences).
- Identifying potential cross-selling or upselling opportunities based on customer segments.
AI agents/copilots in trade promotion optimization enhance operational efficiency and elevate customer service quality as well as strategic decision-making. Companies can gain a substantial competitive edge by seamlessly integrating these advanced AI solutions into their existing infrastructure. This integration empowers them to navigate the intricate promotional landscape with innovative, efficient, and dependable AI-driven tools and strategies, ensuring optimized promotional outcomes and enhanced market responsiveness.
Best practices for implementing AI in promotion optimization
Integrating AI into promotion optimization can lead to disruptive results, but it requires a well-thought-out strategy and careful execution. Here are some best practices to guide you through the implementation process, ensuring a successful AI-driven promotion optimization journey.
Clearly define objectives
Begin by outlining your specific objectives for using AI in promotion optimization. Whether it is for improving ROI, enhancing customer engagement, or optimizing pricing, having clear goals will shape your AI strategy and measure its success.
Data quality and preparation
Data is the foundation of AI. Ensure your data is accurate, comprehensive, and representative of your business context. Clean, preprocess, and organize the data to remove inconsistencies and irrelevant information. Consider using data from multiple sources to enrich your analysis.
Identify suitable use cases
Not all aspects of promotion optimization require AI. Identify use cases where AI can add the most value, such as demand forecasting, dynamic pricing, personalized targeting, or scenario planning.
Collaborate across teams
Promotion optimization involves multiple departments, including marketing, sales, data analytics, and IT. Foster collaboration among these teams to ensure that everyone’s expertise contributes to the success of AI implementation.
Choose the right AI techniques
Select AI techniques that align with your objectives and the data at hand. Different techniques like machine learning, deep learning, natural language processing, and reinforcement learning are suited for different tasks within promotion optimization.
Data security and privacy
AI implementation involves handling sensitive customer and business data. Ensure you have robust security measures to protect this data and adhere to relevant data privacy regulations.
Interpretability and transparency
AI decisions should be interpretable and transparent. Ensure that stakeholders understand how AI arrives at its recommendations and predictions. This fosters trust and helps in making informed decisions.
Continuous learning and improvement
AI models are not static; they need continuous learning and refinement. Regularly update and retrain your AI models to ensure they adapt to changing market conditions, customer behaviors, and business goals.
Measure and monitor the success
Set up metrics to measure the success of your AI-driven promotion optimization efforts. These metrics could include ROI improvements, increased sales, customer engagement metrics, and more.
Regulatory compliance
Ensure that your AI strategies comply with industry regulations and ethical considerations. This is especially significant when dealing with sensitive customer data and making pricing decisions.
Feedback loop and flexibility
Create a feedback loop that encourages users to provide insights on AI-driven recommendations. Use this feedback to refine your AI models and strategies iteratively.
By following these best practices, you can navigate the challenges of implementing AI in promotion optimization and unlock its full potential to enhance your promotional strategies, increase profitability, and deliver greater value to your customers.
The future of trade promotion optimization
The continuous evolution of technology, consumer behavior, and market dynamics shapes the future of trade promotion optimization. As AI and data analytics become more advanced and businesses strive to create more personalized and efficient strategies, TPO is poised for several significant disruptions:
Hyper-personalization
The future of TPO lies in delivering hyper-personalized promotions to individual customers. AI will enable businesses to understand each customer’s preferences, purchase history, and behavior to tailor promotions on a one-to-one level. This level of personalization can greatly enhance customer engagement and loyalty.
Real-time dynamic optimization
AI will empower businesses to make real-time decisions about promotions. Dynamic pricing will become a standard practice based on up-to-the-minute data on demand, competition, and market trends. This agility ensures that promotions remain relevant and effective in a rapidly changing environment.
AI-driven scenario planning
Advanced AI algorithms will simulate various scenarios, predicting the outcomes of different promotional strategies. These simulations will help businesses make informed decisions by providing insights into potential risks and rewards, enabling them to choose the most promising path.
Enhanced customer engagement
TPO will focus on engaging customers throughout their entire journey. AI will create seamless interactions across different touchpoints, combining promotions with personalized recommendations and customer support to enhance the overall experience.
Transparency and ethics
As AI becomes more integrated into TPO, transparency and ethical considerations will gain prominence. Businesses will need to ensure that AI-driven decisions are fair, unbiased, and comply with regulations. Ethical AI practices will be essential to maintain trust with customers and stakeholders.
Predictive analytics and AI-enabled insights
AI will predict future demand and provide actionable consumer preferences and trends insights. Businesses can use these insights to anticipate customer needs and design promotions that resonate with their target audience.
Integration of multiple data sources
AI seamlessly integrates data from various sources, including social media, customer reviews, and competitor activities. This comprehensive data fusion will provide a holistic market view, enabling businesses to design promotions considering a broader spectrum of influencing factors.
Sustainable and socially responsible promotions
AI-powered TPO will help businesses create promotions that align with sustainability and social responsibility goals. Promotions will be designed to minimize waste, reduce excess inventory, and support environmentally friendly practices.
As technology advances, enterprises that embrace these trends will be more favorably positioned to create effective and customer-centric promotion strategies in a rapidly evolving market landscape.
Final words
In the fast-paced world of modern businesses, the utilization of AI in trade promotion optimization emerges as a compass directing toward success. As we traverse the intersection of technology and commerce, a fertile ground for disruption emerges, powered by the force of data-derived insights and astute decision-making.
The integration of AI into trade promotion optimization has ushered in advancements across various dimensions of promotional endeavors, extending beyond the confines of mere transactions. From forecasting demand to adjusting prices in real-time, AI has broken down many barriers, giving businesses the tools to navigate the changing markets.
However, as we wrap up, it’s important to note that the journey of AI is just beginning. The future holds even more possibilities than what we have discussed above. The world of TPO is moving towards smarter decisions, forecasting, and promotions.
As we navigate through this rapidly changing world, it’s important to recognize that AI in TPO is more than just an option; it represents deliberate strides toward advancement and improvement.
Explore how AI in trade promotion optimization can help your enterprise gain a competitive edge. Contact LeewayHertz’s AI experts for tailored solutions for optimized promotions and maximized returns.
Start a conversation by filling the form
All information will be kept confidential.
Insights
What is LLMOps? Exploring the fundamentals and significance of large language model operations
LLMOps, or Large Language Model Operations, encompass the practices, techniques, and tools used to deploy, monitor, and maintain LLMs effectively.
Generative AI for startups: Technologies, applications, benefits, implementation and development
With Generative AI, startups can infuse their products and services with fresh ideas, captivating their clients and setting themselves apart in a crowded market.
Predictive ML models for manufacturing: Use cases, benefits, development and implementation
In today’s modern manufacturing landscape, predictive machine learning models have emerged as powerful tools that transform decision-making, optimize processes, and drive efficiency.