AI in risk management: Applications, benefits, solution and implementation
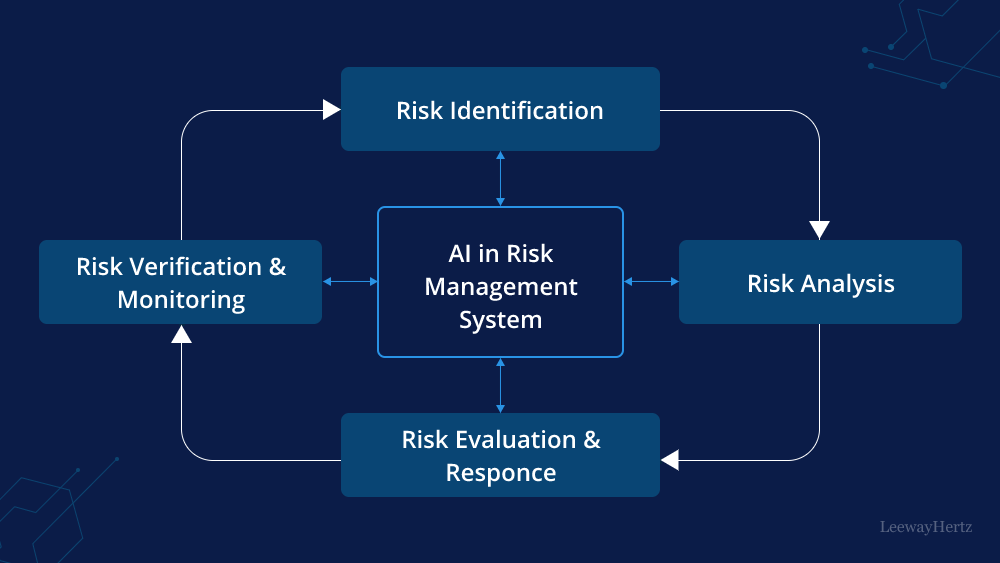
Risk management has traditionally been a complex and resource-intensive endeavor, often relying on historical data and manual analysis. However, with the advent of AI, organizations can now proactively identify, assess, and respond to risks in real time, enhancing decision-making and fortifying resilience. In e-commerce, AI optimizes inventory and demand predictions, mitigating overstocking or shortages. In manufacturing, it pre-empts breakdowns by monitoring real-time machinery data, while in retail, it enhances decision-making through consumer insights. Moreover, AI ensures vehicular safety in the automotive industry by analyzing sensor data and predicting maintenance needs. The role of AI in risk mitigation is reshaping the business landscape across industries, offering a proactive shield against uncertainties and facilitating more informed decisions.
This article delves into the realm of AI in risk management, exploring its diverse industry applications and the transformative outcomes it offers.
- Understanding AI in risk management
- How do AI and ML help in risk management?
- Applications of AI in risk management across industries
- How can GenAI automate risk management processes across sub-departments?
- Streamlining risk management workflows with GenAI
- How does LeewayHertz’s generative AI platform optimize risk management processes?
- LeewayHertz’s AI development services for risk management
- Benefits of AI in risk management
- Difference between traditional risk management and AI-based risk management systems
Understanding AI in risk management
AI in risk management involves leveraging AI’s unique abilities to analyze vast datasets, uncover hidden patterns in them, and anticipate potential risks. This process has AI continuously monitoring data streams, employing predictive analytics, and simulating various scenarios. These practices empower finance, cybersecurity, supply chain management industries and other industries to respond to emerging risks swiftly, predict their occurrence, and take preventive measures.
In simpler terms, think of AI as a powerful detective that examines enormous amounts of information to uncover hidden clues. Just like how a detective might predict where a crime could happen based on patterns, AI can predict where problems might arise in areas like finance, healthcare and education and ensure the smooth functioning of business operations.
One of the inherent strengths of artificial intelligence lies in its capacity to discern and flag unusual patterns, similar to the keen instincts of a seasoned detective who identifies elements warranting investigation. In the context of business operations, AI can adeptly identify irregularities, such as instances of fraud or unforeseen circumstances that deviate from established norms. Additionally, AI helps develop risk management strategies, empowering organizations to formulate and execute more effective contingency plans.
How do AI and ML help in risk management?
AI and ML facilitate risk management by extracting valuable insights from unstructured data. However, organizations need to follow several preparatory steps to process unstructured data effectively:
-
-
- Systematic storage: Data should be structured and scalable, enabling efficient retrieval and analysis. This organized storage lays the foundation for subsequent data processing.
- Data organization: Unstructured data, such as text, images, and videos, must be organized into meaningful categories or segments. This categorization facilitates accurate analysis and interpretation.
- Data cleaning: Unstructured data often contains noise, inconsistencies, and irrelevant information. Data cleaning involves filtering out these elements, ensuring that the data used for analysis is accurate and relevant.
-
Once the data is prepared, AI tools can extract valuable insights, particularly those employing Natural Language Processing (NLP) and Machine Learning (ML) capabilities. Let us see how AI and ML contribute to risk management:
-
-
- Text analysis tools: AI-based text analysis tools powered by NLP can process textual data from various sources, such as news articles, social media, and reports. These tools extract key information, sentiment, and context, enabling organizations to gauge public perception, market sentiment, and emerging risks.
- Risk appetite analysis: AI and ML models can analyze historical data and market trends to help organizations assess their risk appetite. By identifying patterns and correlations, these technologies assist in making informed decisions about the level of risk an organization is willing to tolerate.
- Interpretable risk management models: ML algorithms can enhance the interpretability of risk management models. By analyzing data and providing insights, these models can help risk managers understand the factors influencing risk, making it easier to explain decisions and strategies to stakeholders.
- Stress testing: AI and ML can simulate a variety of scenarios and stress tests to evaluate how different risk factors might impact an organization’s operations. This predictive capability helps identify vulnerabilities and develop strategies to withstand adverse conditions.
- Back-testing: AI can automate back-testing processes, which involve evaluating the performance of a strategy or model using historical data. This helps assess the effectiveness of risk management approaches and refine them for future decision-making.
- Continuous monitoring: AI-powered tools provide real-time monitoring of data streams and generate alerts when unusual patterns or anomalies are detected. This enables organizations to respond swiftly to emerging risks and potential threats.
- Customized risk solutions: AI and ML can analyze an organization’s unique characteristics, industry trends, and risk preferences to provide customized risk management solutions tailored to specific needs.
- Ethical and bias mitigation: Integrating ethical considerations and bias mitigation techniques ensures fair and responsible risk management practices.
-
By leveraging AI and ML, organizations can gain valuable insights from unstructured data, enhance risk management strategies, make informed decisions, and proactively address emerging risks and challenges.
Optimize Your Operations With AI Agents
Optimize your workflows with ZBrain AI agents that automate tasks and empower smarter, data-driven decisions.
Applications of AI in risk management across industries
The applications of AI in risk management include:
Risk management in finance
Advanced risk modeling
AI facilitates the creation of advanced risk models by analyzing extensive historical data, market trends, and relevant factors, uncovering hidden risks and correlations that traditional methods might overlook. This empowers financial institutions to predict and assess risks accurately across diverse asset classes such as stocks, bonds, real estate, commodities, and cryptocurrency.
Fraud detection and prevention
AI-powered systems play a crucial role in risk management by utilizing advanced algorithms to analyze real-time transactional data, identifying anomalies and potential financial fraud. These systems learn from historical data, adapting to evolving fraud techniques and enabling proactive prevention. Machine learning models process and analyze transaction details like amounts, locations, and merchants, helping detect patterns and ensure high-quality data for accurate risk assessment.
Compliance and regulatory monitoring
AI in risk management aids financial institutions in navigating complex regulations by automating compliance processes and monitoring adherence to regulatory standards. These systems analyze extensive data, promptly identifying non-compliance to minimize the risk of penalties and reputational harm.
Credit risk assessment
Utilizing AI algorithms, financial institutions enhance risk mitigation in credit assessment by analyzing diverse data sources like financial records, credit history, and market trends. AI in risk management aids in informed decisions on creditworthiness, loan approvals, and risk-based pricing, effectively lowering default risks and enhancing portfolio performance.
Real-time market surveillance
AI in risk management empowers financial institutions to proactively mitigate risks by monitoring real-time market conditions and swiftly identifying anomalies and potential threats. Through analysis of news sentiment, social media data, and market feeds, AI offers insights into market trends, liquidity risks, and other vital factors, enhancing risk assessment and informed decision-making.
Risk management in retail & e-commerce
Market trend analysis
By monitoring market trends, competitor actions, and consumer sentiment, AI facilitates proactive risk mitigation by offering insights into potential shifts in demand and market preferences. This empowers businesses to adjust strategies, reducing market-related risks’ impact pre-emptively.
Price optimization
Through AI-driven analysis of pricing data, competitor pricing, and market dynamics, businesses can mitigate risks by implementing dynamic pricing strategies. These strategies ensure competitiveness while optimizing profitability, safeguarding against potential revenue loss and market vulnerabilities.
Supply chain risk management
AI in risk management evaluates critical supply chain factors like supplier dependability, transportation challenges, and geopolitical incidents, aiding in identifying and mitigating potential disruptions. This proactive approach empowers businesses to minimize operational risks and ensure smoother continuity.
Customer behavior analysis
By scrutinizing customer behavior, preferences, and purchase records, AI detects patterns signaling risky actions like potential returns or fraud. This analysis contributes to proactive risk mitigation, allowing businesses to take preventive measures and ensure a secure customer experience.
Fraud detection and prevention
Through AI algorithms, transaction data is scrutinized to uncover patterns of fraudulent activity, effectively lowering the threat of financial losses stemming from unauthorized transactions and chargebacks. This proactive analysis aids in robust risk mitigation within financial operations.
Regulatory compliance
AI aids retailers and e-commerce platforms in adhering to diverse regulations like data protection and consumer rights, reducing the likelihood of legal fines and damage to reputation. This proactive support enhances risk management and regulatory compliance.
Customer data security
AI aids in safeguarding customer data by tracking access patterns, recognizing abnormal data retrieval, and pinpointing potential breaches, thus ensuring customer privacy and upholding the company’s reputation through effective risk mitigation.
Operational risk management
By monitoring operational processes, AI detects bottlenecks, system failures, and anomalies that could disrupt e-commerce business operations. This early identification aids in effectively mitigating potential risks and minimizing their impact.
Risk management in healthcare
Fraud detection and billing accuracy
AI supports healthcare institutions in spotting fraudulent activities and billing discrepancies by scrutinizing extensive datasets. This enables AI to alert healthcare providers to irregular billing patterns, preventing fraud and ensuring adherence to regulatory standards such as HIPAA for robust risk mitigation.
Predictive analytics for disease outbreaks
Leveraging diverse data sources like social media, health records, and environmental data, AI predicts disease outbreaks, empowering timely interventions by public health authorities. This proactive approach aids in risk mitigation, facilitating efficient containment of disease spread and optimal resource allocation.
Drug safety and adverse event monitoring
Utilizing AI, electronic health records and clinical trial data are scrutinized to uncover potential adverse reactions to medications or treatments, enabling timely intervention by healthcare providers and regulatory bodies. This proactive approach enhances patient safety through effective risk mitigation.
Operational risk management
AI enhances hospital operations by forecasting patient admissions, resource demands, and staffing requirements, ensuring efficient resource allocation and minimizing wait times. This strategic approach mitigates operational risks, elevating care quality and patient contentment.
Risk management in telecommunication
Network security and anomaly detection
AI-driven tools can monitor network traffic patterns in real-time, rapidly identifying anomalies or unusual activities that might indicate a cyberattack or security breach. These systems can then trigger immediate responses or alerts to mitigate potential threats.
Fraud detection and prevention
AI algorithms scrutinize extensive call data records to swiftly uncover fraudulent actions like SIM card cloning or toll fraud, leveraging historical insights to identify intricate patterns and preclude financial losses arising from fraud. This robust risk mitigation approach complements human analysis and bolsters fraud prevention measures.
Customer data protection
AI-driven systems oversee and safeguard customer data, averting compromise by identifying abnormal access and unauthorized data transfers. This vigilant oversight enhances data privacy, effectively mitigating the risk of breaches and maintaining customer trust.
Capacity planning
Through historical data analysis and future demand prediction, AI supports telecommunications firms in pre-emptively expanding and upgrading networks. This proactive strategy averts capacity-related concerns, mitigating the risk of service deterioration and ensuring a seamless user experience.
Emergency response optimization
AI analyzes emergency call patterns for telecommunications providers, aiding in resource optimization by detecting call surges and pinpointing incident locations. This data-driven approach ensures effective resource allocation, mitigating risks during emergencies and improving response outcomes.
Natural disaster preparedness
AI predicts and monitors the influence of natural disasters on network infrastructure by assessing weather patterns and historical data. This proactive analysis empowers telecom companies to implement pre-emptive safeguards, ensuring network resilience and uninterrupted connectivity, even during challenging circumstances, for effective risk mitigation.
Risk management in the automotive industry
Vehicle diagnostics and predictive maintenance
Leveraging AI-enabled sensors and data analytics, real-time vehicle performance monitoring can be implemented to anticipate mechanical problems, pre-empting breakdowns and accidents. This predictive maintenance can minimize the risk of unforeseen failures, slashing downtime and prolonging vehicle longevity, effectively mitigating potential hazards.
Driver assistance systems
AI-driven Advanced Driver Assistance Systems (ADAS) can enhance road safety by employing algorithms to identify collision risks, enabling features like adaptive cruise control, lane departure alerts, and automatic emergency braking. These technologies can proactively mitigate risks, aiding drivers in preventing accidents and promoting safer journeys.
Fraud detection in insurance
AI-driven analysis of driver behavior, vehicle data, and accident patterns can help insurance companies detect fraudulent claims. By identifying inconsistencies and anomalies, AI systems contribute to fair and accurate claims processing, reducing the risk of insurance fraud.
Supply chain optimization
By examining automotive supply chain data, AI can detect possible disruptions like part shipment delays and production bottlenecks, proactively reducing the risk of production delays, ensuring on-time vehicle and component delivery and mitigating potential setbacks effectively.
Recall management
AI applications aid in recognizing and rectifying vehicle safety-related flaws by analyzing data from customer feedback, vehicle sensors, and quality control procedures. This early identification enables swift recalls, effectively mitigating the risk of accidents due to faulty components and bolstering overall safety standards.
Risk management in insurance
Risk assessment
By leveraging AI algorithms, insurers can comprehensively evaluate diverse data sources, including historical claims, demographics, and external factors like weather, leading to precise risk assessment. This strategic approach aids in setting policy prices correctly and effectively mitigates risk exposure to high-risk clients.
Fraud detection
AI in risk management helps identify fraudulent insurance claims by spotting patterns and anomalies in large datasets. AI systems can flag potentially suspicious claims for further investigation by comparing new claims to historical fraudulent cases. This not only saves money for insurers but also helps in maintaining fair premiums for honest policyholders.
Predictive analytics
AI predicts forthcoming losses using historical data and risk indicators, aiding insurers in proactive risk management and resource allocation. This predictive approach mitigates potential losses, optimizing risk mitigation strategies.
Real-time monitoring and alerts
AI can provide real-time monitoring of insured assets using IoT devices and sensors. In case of potential risks, such as sudden changes in environmental conditions or equipment malfunctions, AI can trigger alerts for timely intervention.
Risk management in manufacturing
Predictive maintenance
AI-driven predictive maintenance analyzes data from sensors and equipment to predict when machinery is likely to fail. This enables manufacturers to perform maintenance proactively, reducing unplanned downtime and minimizing production disruptions.
Anomaly detection
AI algorithms can identify anomalies in production processes by comparing real-time data to historical patterns. This helps in detecting deviations that might indicate equipment malfunctions, quality issues, or safety concerns.
Quality control and defect detection
AI-powered image recognition and computer vision technologies can identify defects in products during manufacturing. This ensures that only high-quality items reach the market, reducing recalls and enhancing customer satisfaction.
Process optimization
AI can optimize production processes by analyzing data from various sources, such as production lines, sensors, and environmental conditions. This leads to increased efficiency, reduced waste, and improved resource allocation.
Safety monitoring
AI can monitor workers’ activities and equipment conditions to identify safety risks. It can provide real-time alerts when workers are exposed to hazardous situations, helping prevent accidents.
Inventory management
AI algorithms can predict demand patterns and optimize inventory levels, ensuring that manufacturers have the right amount of materials and finished products at the right time. This minimizes excess inventory costs and shortages.
Risk assessment and mitigation
AI can analyze historical data, market trends, and external factors to assess potential risks to production. This enables manufacturers to develop strategies to mitigate risks associated with factors such as market volatility, supply chain disruptions, and changing customer demands.
Customized production
AI can analyze customer preferences and trends to help manufacturers tailor their production processes and product offerings. This reduces the risk of producing items that might not align with market demands.
Risk management in education
Predictive analytics for student behavior
AI can analyze student data and behavior patterns to identify potential signs of distress or risky behavior. This allows educators and administrators to intervene and provide support before issues escalate.
Threat detection and prevention
AI-powered cybersecurity tools can monitor network traffic, detect anomalies, and identify potential cyber threats. This helps prevent data breaches and ensures the security of sensitive student and institutional information.
Video analytics
AI-powered video surveillance systems can analyze footage for unusual activities, helping improve campus security.
Optimize Your Operations With AI Agents
Optimize your workflows with ZBrain AI agents that automate tasks and empower smarter, data-driven decisions.
How can GenAI automate risk management processes across sub-departments?
This section outlines the sub-departments within risk management and the related processes that GenAI can transform. By leveraging automation and advanced predictive insights, GenAI significantly enhances risk detection, mitigation strategies, and overall decision-making, improving operational efficiency and reducing vulnerabilities across all areas of risk management.
Operational risk management
This sub-department identifies and mitigates risks arising from internal business operations.
Sub-processes and GenAI automation:
- Process risk identification: GenAI can assess workflows and operational data to predict potential risks in business processes.
- Incident tracking and analysis: Automates the tracking of operational incidents and provides insights into patterns for future prevention.
- Failure prediction: Uses historical data to predict when and where operational failures might occur, enabling preventive actions.
- Compliance monitoring: GenAI can monitor business activities to ensure they align with regulatory requirements, identifying non-compliance risks in real-time.
- Control effectiveness assessment: Automates evaluating existing risk controls and suggests improvements.
- Audit trail analysis: Analyzes audit logs to detect anomalies or irregular activities that may indicate operational risks.
- Supply chain risk assessment: GenAI can evaluate supplier performance and external factors to predict supply chain disruptions.
- Real-time risk reporting: GenAI automates risk reporting by consolidating data from various systems, providing management with real-time risk insights.
Financial risk management
This sub-department manages risks related to financial operations, including market and liquidity risks.
Sub-processes and GenAI automation:
- Credit risk evaluation: GenAI evaluates borrower profiles by analyzing credit data and behavior patterns to provide a more accurate risk assessment.
- Liquidity risk forecasting: Uses real-time financial data to predict liquidity crunches and recommend cash management strategies.
- Market risk simulation: Simulates market scenarios to analyze potential financial impacts and helps create risk-adjusted strategies.
- Fraud detection: Analyzes transaction data to identify unusual patterns or suspicious activities, reducing fraud risks.
- Stress testing: Automates the stress testing of financial portfolios under various economic scenarios to evaluate potential risks.
- Portfolio risk optimization: Assesses the risk-return profile of investment portfolios and suggests optimization strategies.
- Counterparty risk assessment: Analyzes the financial health of counterparties to predict default risk in contracts or trades.
- Interest rate risk modeling: Predicts the impact of interest rate fluctuations on financial assets and liabilities, helping manage exposure.
Compliance risk management
This sub-department ensures adherence to regulations, laws, and internal policies to avoid legal penalties.
Sub-processes and GenAI automation:
- Regulatory compliance monitoring: GenAI automates the review of policies and regulations, flagging any changes that affect the organization.
- Policy enforcement analysis: Analyzes internal policy implementation and highlights areas with compliance gaps.
- Automated reporting: Generates compliance reports based on current regulatory requirements and organizational data.
- Real-time compliance audits: Continuously monitors operations to ensure adherence to regulatory standards, identifying potential non-compliance in real-time.
- KYC (Know Your Customer) automation: Automates identity verification and checks against regulatory requirements for onboarding customers.
- Regulatory change management: Tracks regulatory changes and assesses their impact on the organization’s policies and procedures.
- Risk assessment documentation: Automatically generates risk assessment documents based on compliance evaluations and internal data.
- Whistleblower reporting management: Streamlines and anonymizes whistleblower reports, ensuring proper compliance with whistleblowing regulations.
Enterprise Risk Management (ERM)
This sub-department oversees an integrated approach to identify and mitigate organizational risks.
Sub-processes and GenAI automation:
- Risk assessment and prioritization: GenAI assess organizational risks and rank them based on severity and likelihood.
- Scenario analysis: Generates risk scenarios based on historical and real-time data to help executives make informed decisions.
- Risk aggregation: Aggregates data from different business units to provide a comprehensive view of risk exposure.
- Risk mitigation planning: Automates the creation of risk mitigation strategies by analyzing potential risks and suggesting tailored actions.
- Early risk detection: Continuously monitors data sources to detect emerging risks before they escalate.
- Risk communication: Automates the distribution of risk updates and reports to relevant stakeholders across the organization.
- Compliance alignment: Ensures risk management strategies align with evolving regulatory requirements and standards.
- Risk performance metrics: Tracks and analyzes the effectiveness of risk management initiatives, providing key performance indicators (KPIs) for improvement.
Strategic risk management
This sub-department focuses on identifying and managing risks that could impact the long-term business strategy.
Sub-processes and GenAI automation:
- Strategic forecasting: Uses GenAI to predict how external market trends and competition could impact strategic goals.
- Competitive risk analysis: Automates the evaluation of competitive threats and suggests adjustments to the company’s strategic positioning.
- Mergers and acquisitions risk assessment: Assesses risks associated with M&A by analyzing market conditions, competitors, and legal challenges.
- Innovation risk evaluation: Analyzes risks tied to adopting new technologies or innovative strategies, helping to balance risk with growth potential.
- Strategic scenario planning: Automates the creation of future scenarios to explore how different strategic choices might play out under various market conditions.
- Market entry risk assessment: Assesses risks related to entering new markets, such as regulatory challenges, competitive landscape, and cultural differences.
- Long-term risk monitoring: Continuously monitors long-term risks to strategic initiatives, providing updates and recommendations for adjustment.
Cybersecurity risk management
This sub-department manages risks related to data breaches, hacking, and information security threats.
Sub-processes and GenAI automation:
- Threat detection and prevention: GenAI can detect anomalies in network activity and predict potential cybersecurity threats.
- Incident response automation: Automates immediate responses to detected threats, minimizing the impact of cyber incidents.
- Vulnerability analysis: Identifies system vulnerabilities by continuously scanning networks and applications for weak points.
Reputational risk management
This sub-department focuses on protecting the organization’s public image and customer trust.
Sub-processes and GenAI automation:
- Sentiment analysis: Analyzes social media, reviews, and public statements to gauge public sentiment and identify potential reputational risks.
- Crisis communication automation: Automates the creation of crisis responses, generating draft statements based on the nature of the issue.
- Media monitoring: Tracks media mentions and flags negative press or online chatter that could impact reputation.
- Reputation score monitoring: Continuously monitors and updates a company’s reputation score by analyzing public perception and stakeholder feedback.
- Influencer risk assessment: Evaluates the potential reputational risk associated with partnerships or endorsements from influencers or public figures.
- Brand risk forecasting: Predicts future reputational risks by analyzing industry trends, market events, and public sentiment shifts.
- Employee reputation impact analysis: Assesses how employee behavior or statements might impact the organization’s reputation and suggests mitigation strategies.
Third-party risk management
This sub-department manages risks posed by vendors, suppliers, and external partners.
Sub-processes and GenAI automation:
- Vendor risk assessment: GenAI evaluates third-party risk profiles by analyzing contracts, past performance, and financial stability.
- Supplier performance monitoring: Continuously monitors supplier data to identify any performance risks that may affect operations.
- Contract risk analysis: Analyzes the legal risks associated with vendor contracts, flagging terms that may pose potential liabilities.
- Third-party due diligence: Automates the collection and analysis of data on third parties, including financial records, legal history, and compliance standing.
- Continuous risk scoring: Provides real-time risk scores for third-party vendors by analyzing external factors like regulatory changes or economic conditions.
- Cybersecurity risk monitoring: Monitors the cybersecurity practices of third-party vendors and flags potential vulnerabilities.
- Vendor compliance tracking: Tracks and ensures third-party adherence to contractual obligations, regulatory requirements, and industry standards.
Insurance risk management
This sub-department manages insurance policies and risk transfer mechanisms.
Sub-processes and GenAI automation:
- Policy optimization: GenAI reviews insurance policies to recommend optimal coverage levels based on organizational risks.
- Claims prediction: Predicts potential insurance claims by analyzing historical claims data and operational risks.
- Risk transfer scenario modeling: Models different risk transfer strategies to find the most cost-effective solutions for the organization.
- Claims fraud detection: Analyzes claims data for unusual patterns to identify potential fraud.
- Premium forecasting: Predicts future insurance premium costs based on historical trends and risk exposure.
- Loss prevention recommendations: Provides actionable insights to minimize risks and prevent future losses by analyzing operational data.
- Coverage gap analysis: Identifies gaps in existing insurance coverage by assessing organizational risks and insurance policy terms.
Generative AI automates risk management processes across sub-departments by enhancing threat detection, streamlining incident response, and continuously identifying vulnerabilities. Its ability to enforce policies, manage access, and proactively mitigate risks ensures stronger and more efficient cybersecurity operations.
Streamlining risk management workflows with GenAI
Generative AI is transforming how organizations approach risk management by automating and enhancing various processes across the entire risk management lifecycle. From identifying potential risks to establishing governance structures, GenAI streamlines tasks, offers predictive insights and ensures that risk management practices are efficient and effective. Below is a detailed overview of how generative AI can be integrated into each step of the risk management process, showcasing its pivotal role in modern risk governance.
Risk identification
Steps |
Sub-steps involved |
Role of generative AI |
---|---|---|
Define objectives and scope |
|
|
Gather information |
|
|
Identify potential risks |
|
|
Categorize risks |
|
|
Risk assessment
Steps |
Sub-steps involved |
Role of generative AI |
---|---|---|
Determine risk criteria |
|
|
Analyze risks |
|
|
Prioritize risks |
|
|
Risk mitigation
Steps |
Sub-steps involved |
Role of generative AI |
---|---|---|
Identify mitigation strategies |
|
|
Develop action plan |
|
|
Implement mitigation measures |
|
|
Document and report |
|
|
Establish contingency plans |
|
|
Training and awareness |
|
|
Risk monitoring
Steps |
Sub-steps involved |
Role of generative AI |
---|---|---|
Establish monitoring mechanisms |
|
|
Review risk status |
|
|
Communicate findings |
|
|
Adjust mitigation strategies |
|
|
Risk governance
Steps |
Sub-steps involved |
Role of generative AI |
---|---|---|
Define governance structure |
|
|
Establish risk appetite and tolerance |
|
|
Implement governance frameworks |
|
|
Integrate risk management into decision-making |
|
|
Check compliance and regulatory alignment |
|
|
Report to stakeholders |
|
|
Incorporating generative AI into risk management workflows improves efficiency and enhances accuracy and insight across all stages of the risk management process. By leveraging genAI’s capabilities, organizations can better anticipate potential risks, develop robust mitigation strategies, and ensure that risk management is deeply integrated into their decision-making processes. This comprehensive approach enables businesses to stay ahead of emerging threats and effectively navigate the complex landscape of modern risk governance.
How does LeewayHertz’s generative AI platform optimize risk management processes?
LeewayHertz’s generative AI platform, ZBrain, plays a transformative role in empowering businesses to enhance risk management processes, streamline risk evaluation workflows, and optimize overall risk mitigation strategies. As a comprehensive, enterprise-ready platform, ZBrain empowers businesses to design and implement applications tailored to their specific operational requirements. The platform uses clients’ data, whether in the form of text, images, or documents, to train advanced LLMs like GPT-4, Vicuna, Llama 2, or GPT-NeoX for developing contextually aware applications capable of performing diverse tasks.
Businesses face challenges in risk management, including navigating uncertainty, staying ahead of evolving threats, ensuring data accuracy, managing regulatory compliance, balancing risk and reward, adapting to market fluctuations, and addressing emerging risks like cybersecurity threats. ZBrain effectively addresses these challenges through its distinctive feature called “Flow,” which provides an intuitive interface that allows users to create intricate business logic for their apps without the need for coding. Flow’s easy-to-use drag-and-drop interface enables the seamless integration of large language models, prompt templates, and other genAI models into your app’s logic for its easy conceptualization, creation, or modification.
To comprehensively understand how ZBrain Flow works, explore this resource that outlines a range of industry-specific Flow processes. This compilation highlights ZBrain’s adaptability and resilience, showcasing how the platform effectively meets the diverse needs of various industries, ensuring enterprises stay ahead in today’s rapidly evolving business landscape.
ZBrain’s potent LLM-based applications enhance risk management by converting intricate data into actionable insights, boosting analytical capabilities, automating processes, enabling proactive risk identification, and fostering more effective decision-making. Its comprehensive solutions address pivotal challenges in risk management processes, equipping organizations with powerful tools to navigate uncertainties and elevate their overall risk mitigation strategies. Explore the subsequent section, elucidating how ZBrain enhances risk management within the finance and banking sectors.
AI-driven financial risk management
ZBrain transforms risk management processes, fortifying financial security in the finance and banking sector through automated methodologies. In the initial step, ZBrain seamlessly acquires relevant data from diverse sources, encompassing internal transaction records, regulatory compliance databases, credit ratings, and financial statements. The subsequent automatic Exploratory Data Analysis (EDA) phase reveals insights, comprehends data structures and identifies connections and patterns that significantly impact risk assessment. Leveraging advanced embedding techniques, such as word embeddings or sentence embeddings, ZBrain converts textual data into numerical representations, capturing semantic meanings and relationships among data points to deliver precise insights and enhance decision-making processes. Upon receiving a risk management analysis report query, ZBrain retrieves relevant data based on query requirements and employs the chosen Large Language Model (LLM) for report generation. The LLM understands and contextualizes data using acquired embeddings, dynamically producing a comprehensive report that explores information from the dataset, query particulars, and the intended report structure. Following text-based report generation, a meticulous parsing process extracts essential information. This parsed data is thoughtfully structured to ensure the final report precisely adheres to desired formats, sections, and report guidelines, reflecting ZBrain’s commitment to a meticulous and effective risk management approach.
ZBrain applications enable financial institutions with efficient and data-driven decision-making, substantially reducing the time and effort traditionally invested in manual risk assessments. This results in streamlined risk evaluation, enhanced fraud detection, improved reporting and insights, proactive risk identification, minimized risk exposure, and an overall enhancement in risk management, all while upholding stringent data privacy standards. Examine this comprehensive flow to comprehend how ZBrain transforms financial risk management, delivering a more precise and streamlined approach to identifying, assessing, and mitigating risks, eventually optimizing decision-making processes in diverse business environments.
LeewayHertz’s AI development services for risk management
At LeewayHertz, we develop customized AI solutions that address the specific challenges faced by risk management teams. Our AI/ML consulting services help risk management professionals leverage AI to enhance risk identification, assessment, and mitigation strategies.
Through the development of Proof of Concepts (PoCs) and Minimum Viable Products (MVPs), we enable risk management teams to test AI tools in real-world scenarios, ensuring that the solutions are effective and tailored to the unique requirements of risk management in various sectors.
Our expertise in generative AI streamlines risk reporting, monitoring internal processes and data management processes, automating routine tasks and allowing risk managers to focus on more strategic responsibilities.
By fine-tuning large language models to understand the complexities of risk terminology and industry-specific contexts, LeewayHertz improves the precision and relevance of AI-powered risk analyses and communications.
Furthermore, we ensure these AI systems integrate seamlessly with existing risk management frameworks and technologies, enhancing operational efficiency and decision-making capabilities within risk management departments.
Our AI solutions development expertise
AI solutions development for risk management typically involves creating systems that enhance risk identification, assessment, and mitigation strategies. These solutions integrate key components such as data aggregation technologies, which compile and analyze risk-related information from diverse sources. This comprehensive data foundation supports predictive analytics capabilities, allowing for the forecasting of potential risks and their impact on the organization. Additionally, machine learning algorithms are employed to identify patterns and anomalies in risk data, enabling proactive risk management approaches. These solutions often cover areas like credit risk assessment, operational risk management, fraud detection, and regulatory compliance.
Overall, AI solutions in risk management aim to minimize potential losses, improve risk visibility, and streamline risk-related processes, ultimately strengthening the organization’s resilience and decision-making capabilities.
AI agent/copilot development for risk management
LeewayHertz builds custom AI agents and copilots that enhance various risk management operations, enabling companies to save time and resources while improving risk assessment and mitigation. Here is how they help:
1. Data collection and analysis:
- Scan various data sources, including internal databases, external reports, news feeds, and social media, to collect relevant information for risk identification and assessment.
- Analyze massive datasets, identify potential risks, and pinpoint anomalies that might indicate emerging threats.
- Identify and correct data errors, transforming raw data into usable formats.
- Calculate risk scores for different situations based on historical data and current trends and predict potential future risks.
2. Risk assessment and prioritization:
- Assess the likelihood and impact of identified risks, providing a quantitative risk score for prioritization and resource allocation.
- Monitor the risk environment, updating risk scores and assessments based on real-time changes, ensuring a proactive approach.
- Simulate various scenarios, helping to understand the potential consequences of different risks and evaluate the effectiveness of mitigation strategies.
3. Risk response and mitigation:
- Suggest specific control measures and risk mitigation strategies based on risk assessments and historical data.
- Adjust existing controls or implement new ones in response to evolving risk profiles and external factors.
- Track the effectiveness of risk mitigation efforts, analyze performance metrics, and suggest improvements for better risk management practices.
- Monitor risk indicators and provide timely alerts when risks escalate, enabling quick responses.
4. Reporting and communication:
- Generate comprehensive risk reports, dashboards, and visualizations, providing clear insights into the risk landscape and mitigation strategies.
- Trigger alerts when significant risks emerge or when predetermined thresholds are exceeded, enabling swift response and action.
- Streamline communication by providing transparent and accessible information to stakeholders, fostering better collaboration and decision-making.
AI agents and copilots increase operational process efficiency and significantly enhance the quality of risk assessment and alleviation. By integrating LeewayHertz’s advanced AI solutions into their existing infrastructure, risk management firms can achieve a significant competitive advantage, navigating the complex landscape of risk management with innovative, efficient, and reliable AI-driven tools and strategies.
Benefits of AI in risk management
The benefits of AI in risk management include:
Data-driven insights
AI facilitates the comprehensive collection, analysis, and interpretation of substantial data from diverse origins like market trends, customer feedback, regulatory shifts, or internal audits. It recognizes patterns, anomalies, correlations, and causal links in this data, highlighting potential risks or prospects. By incorporating AI, you can achieve enhanced and precise insights into risk exposure, performance, and consequences, enabling data-driven decisions that transcend intuition and assumptions.
Proactive prevention
AI empowers proactive risk mitigation through predictive analytics, scenario analysis, and simulation methods. By forecasting the probability and repercussions of diverse risk events—like cyberattacks, fraud, or operational glitches—AI recommends optimal measures for mitigation. Additionally, AI facilitates vigilant monitoring, promptly notifying you of alterations or deviations in your risk landscape, including emerging threats, vulnerabilities, or compliance concerns, enabling swift and efficient responses.
Adaptive learning
AI facilitates continuous improvement in risk management by leveraging machine learning, natural language processing, and cognitive computing. It captures and retains insights and best practices from past risk management endeavors, like risk assessments, controls, or mitigation plans, enhancing future performance. Moreover, AI ensures adaptability to evolving conditions and expectations by revising risk models, policies, or strategies by incorporating new data, feedback, and outcomes.
Enhanced collaboration
AI enhances stakeholder collaboration and communication by employing chatbots, voice assistants, and sentiment analysis. It enables the timely dissemination of pertinent information, guidance, and support to employees, customers, partners, and regulators, engaging them in the risk management workflow. Additionally, AI facilitates the integration of feedback, opinions, and preferences from stakeholders, nurturing trust and transparency within risk management endeavors.
AI harnesses data, analytics, and automation capabilities to identify and address industry-specific risk factors. By embracing AI, you enhance your risk management strategies’ efficiency, efficacy, and adaptability, fostering a sense of confidence and resilience as you work toward your business objectives.
Difference between traditional risk management and AI-based risk management systems
Here is a comparison between traditional risk management and AI-based risk management systems:
Aspect | Traditional Risk Management | AI-based Risk Management |
Data handling | Primarily structured data used for analysis | Analyzes both structured and unstructured data for insights |
Decision-making | Relies heavily on human expertise and experience | Combines human expertise with AI-driven insights for informed decisions |
Speed of analysis | Analysis can be time-consuming and manual | Rapid data analysis and real-time monitoring for quicker insights |
Predictive capabilities | Limited predictive capabilities based on historical data | Advanced predictive analytics for anticipating potential risks |
Customization | Generally follows standardized risk models | Tailors risk management strategies based on the organization’s requirements |
Scalability | May struggle to handle large and diverse datasets | Can handle large volumes of data efficiently through AI algorithms |
Interpretable models | The work is done manually, so the risk management strategies might become difficult to understand | AI models are designed for interpretability, aiding understanding |
Continuous monitoring |
Monitoring is periodic and manual |
AI provides real-time monitoring and alerts for proactive responses |
Scenario simulation | Limited ability to simulate complex scenarios | AI can simulate various scenarios and stress tests for better insights |
Bias and ethical concerns | Human bias may impact decision-making | AI’s fairness and bias mitigation techniques enhance ethical decision-making |
Human-AI collaboration |
Primarily reliant on human decisions |
Human-AI collaboration augments decision-making for improved outcomes |
Future adaptability | May face challenges in adapting to new risks | AI can adapt to evolving risks and trends through continuous learning |
Regulatory compliance | Manual compliance tracking and reporting | AI can automate compliance monitoring and ensure adherence |
Endnote
Integrating AI into risk management heralds a new era of precision, agility, and foresight for businesses across industries. As organizations grapple with an increasingly complex and volatile business environment, AI emerges as a beacon of innovation, offering transformative solutions to the age-old challenge of risk mitigation.
The multitude of AI use cases in risk management is a testament to its versatility and power. From fraud detection algorithms that swiftly pinpoint anomalies in vast datasets to predictive models that anticipate market fluctuations, AI’s capabilities transcend traditional methods, providing real-time insights that enable proactive decision-making. These applications of AI in risk management empower enterprises to not only safeguard their assets but also seize opportunities with confidence. By harnessing AI’s power, businesses can confidently navigate uncertainties, adapt swiftly to changing circumstances, and carve a path toward a future defined by resilience, innovation, and success.
Ready to fortify your business? Embrace AI in risk management for enhanced decision-making, proactive risk mitigation, and sustainable growth. Contact LeewayHertz today for AI consulting and development needs!
Start a conversation by filling the form
All information will be kept confidential.
FAQs
How does AI improve risk management processes?
What types of risks can AI detect?
How does AI contribute to regulatory compliance in risk management?
What are the benefits of AI in risk management?
How does AI help detect and prevent fraud in risk management?
How does LeewayHertz help in building AI risk management solutions?
How does ZBrain enhance risk management processes, and what benefits does it offer organizations?
How does LeewayHertz ensure the accuracy and reliability of AI risk management solutions?
How can we initiate a collaboration with LeewayHertz to build an AI risk management solution?
Getting started with building an AI risk management solution with LeewayHertz is easy. Contact our team to schedule a consultation by visiting our website or emailing us at sales@leewayhertz.com to discuss your specific requirements. Our experts will work with you to understand your business objectives, assess your data infrastructure, and identify opportunities for leveraging AI technologies in risk management. From there, we will develop a customized solution tailored to your needs, guiding you through each step of the development process to ensure a successful outcome.
Insights
Generative AI in travel: Navigating the future of tailored tourism
Generative AI allows travel companies to provide real-time updates and personalized recommendations to travelers, guiding them through the complex world of travel information.
Topic modeling in NLP: Extracting key themes/topics from textual data for enhanced insights
Topic modeling is a popular technique used in natural language processing and text mining to uncover latent themes and structures within a collection of documents.
AI in cloud computing: Applications, operational mechanics and benefits
AI in cloud computing represents a fusion of cloud computing capabilities with artificial intelligence systems, enabling intuitive, interconnected experiences.