AI for master data management: Use cases and benefits
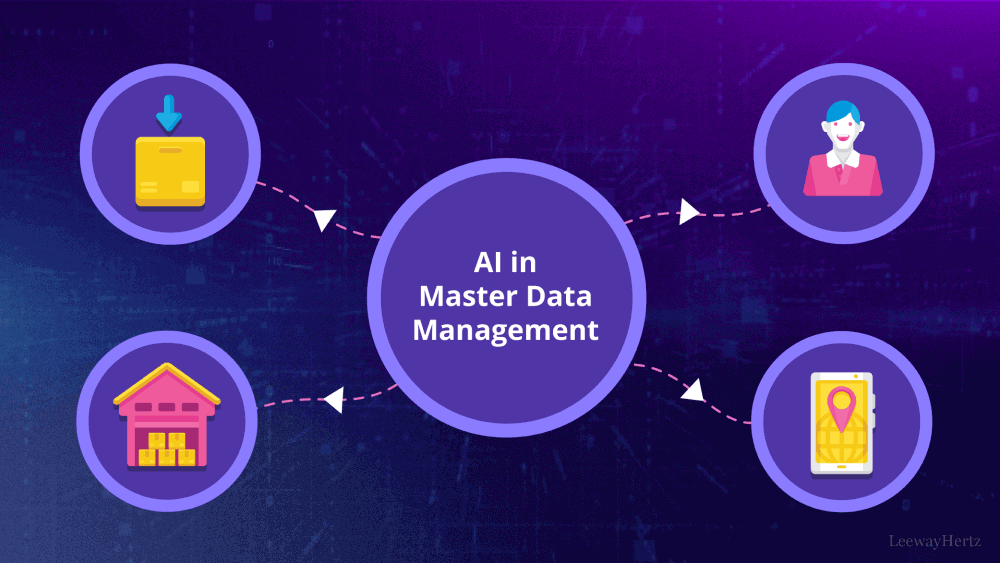
In today’s digitally driven environment, enterprises are confronted with the challenge of not only managing vast volumes of data but also navigating the complexity of various data types, particularly from emerging sources like Internet of Things (IoT) devices and connected technologies. This scenario is further complicated by a significant shift towards cloud technologies, urging businesses to move beyond mere infrastructure procurement to a more strategic utilization of technology and services, aimed at maximizing data asset value.
The concept of ‘Data Agility’ emerges as a crucial response to this situation. It embodies an enterprise’s ability to adapt and respond efficiently to the dynamic demands of global data management. This agility is essential, considering the substantial impact that inaccurate master data can have on organizational revenue. In a market increasingly influenced by AI (Artificial Intelligence) and ML (Machine Learning), the adoption of innovative data management technologies becomes indispensable.
A robust Master Data Management (MDM) strategy is now pivotal for enterprise success in this competitive landscape. MDM solutions stand as the foundational source of truth in the business world, crucial for Big Data analysis and future-proofing data repositories. They empower organizations to extract deeper insights from diverse data types and explore new data categories, thereby enhancing decision-making capabilities.
Integrating AI and ML into data management not only heralds a new era of data quality, cloud readiness, and scalability but also equips enterprises to effectively manage, interpret, and leverage their data. This integration leads to more informed decisions and strategic operational enhancements, preparing businesses for a data-intensive future.
- What is master data?
- What is Master Data Management (MDM)?
- Use cases of AI in master data management
- Advantages of using AI in master data management: An analytical perspective
- How can AI be used in different stages of master data management?
- LeewayHertz’s AI development services for master data management
What is master data?
Master data refers to the critical, core data within an enterprise that is essential for conducting business operations and making informed decisions. This data encompasses vital information about the primary entities around which business transactions revolve and generally changes infrequently. Master data is not transactional but rather plays a key role in defining and guiding transactions. The principal domains of master data typically include customers, products, employees, suppliers, and locations. Each of these domains can be further divided into sub-domains, providing detailed classification and segmentation based on various attributes and contexts. Such thorough segmentation and classification enhance data manageability and utilization, reinforcing strategic data utilization across various business processes and decision-making scenarios. Master data management requires a comprehensive approach that surpasses simple lists, delving into a more intricate, structured, and holistic management of these diverse data types.
It is important to differentiate master data from other types of data commonly found in businesses:
- Unstructured data: This includes non-specific data formats such as emails, white papers, and marketing materials. While important, unstructured data is not classified as master data.
- Transactional data: Contains detailed records of business transactions. It is distinguished by its temporality and specificity to particular events or activities, differing from the more stable nature of master data.
- Metadata: Involves data that describes other data, offering additional context and understanding. Metadata supports and clarifies both master and transactional data but is not considered master data itself.
- Hierarchical data: This data type outlines relationships and dependencies between different data elements. It can be closely related to master data but serves more to illustrate connections and hierarchies within the data.
- Reference data: Consists of a special category of data used to categorize or link other data elements, often to external standards or classifications. While it can be associated with master data, it serves a distinct purpose in providing context and standardization.
Understanding these distinctions is crucial for effective data management in any organization, ensuring that each type of data is appropriately categorized and managed for optimal business efficiency and insight.
What is Master Data Management (MDM)?
MDM goes beyond a convergence of technology, processes, and tools designed to manage, coordinate, and safeguard an enterprise’s master data. Ensuring that this vital data is accurate, consistent, and comprehensively available across an organization and its business affiliates, MDM extends beyond a mere technological solution to envelop crucial business processes and policy adjustments often needed to preserve the integrity of master data.
MDM is a multifaceted process that entails the meticulous creation and continuous maintenance of master data. It recognizes that investing in developing a clean and consistent data set is only the first step. Equally important are the mechanisms put in place to ensure the data’s integrity and reliability throughout its lifecycle. This involves ongoing efforts to keep the data accurate, up-to-date, and consistent, thereby preserving its value and usefulness for business operations and decision-making. The intricate nature of MDM lies in this dual responsibility of not only establishing high-quality master data but also in maintaining its quality over time. Depending upon the specific technology and strategies employed, MDM may be singularly focused on one domain—such as customers, products, or locations—or may span multiple domains, with multi-domain MDM offering a slew of advantages, including a consistent data stewardship experience, minimized technological footprint, shared reference data across domains, reduced total cost of ownership, and an elevated return on investment.
To be optimally effective, an MDM strategy must be structured around six fundamental disciplines, each pivotal in orchestrating a robust MDM program:
- Governance: Establishing a strategic framework that directs and manages organizational structures, policies, principles, and standards to facilitate access to validated and certified master data. It involves forming a cross-functional team to articulate and define the various facets of the MDM program.
- Measurement: Continuously evaluating the MDM program against its stated objectives and focusing on data quality and ongoing enhancement.
- Organization: Ensuring that the right individuals are positioned appropriately throughout the MDM program, which includes master data owners, data stewards, and governance participants.
- Policy: Outlining and adhering to a set of standards, policies, and requirements to which the MDM program should comply.
- Process: Instituting defined processes that span the data lifecycle and are employed to manage master data.
- Technology: Implementing a master data hub and any enabling technology supporting the overall MDM program.
In sum, MDM goes beyond the typical boundaries of a technological solution, navigating through the nuanced avenues of organizational politics and technical challenges and ensuring that master data remains an untainted, reliable, and consistent asset across the enterprise. Thus, a robust MDM strategy should holistically integrate these six disciplines, ensuring that master data not only serves its immediate functional purpose but also fosters a sustainable framework for managing data in a continually evolving business landscape.
Optimize Your Operations With AI Agents
Optimize your workflows with ZBrain AI agents that automate tasks and empower smarter, data-driven decisions.
Use cases of AI in master data management
Data discovery
Navigating through the intricate and voluminous landscape of master data has been recognized as a multifaceted challenge, particularly considering the excessive volume of continuously generated data. According to IDC, the world witnessed the creation or replication of 64.2 Zettabytes (ZB) of data in 2020, while data is predicted to increase at a compound annual growth rate (CAGR) of 23% throughout the 2020-2025 forecast period. With organizations, notably larger ones, utilizing an average of 175 applications and their smaller counterparts averaging 73, as per the Businesses at Work Study by Okta, the need for potent data management becomes even more pronounced. Coupled with an expected 30% CAGR growth of data lakes, the imperative for efficient data management methodologies is clearly underscored.
In such a scenario, resorting to manual approaches for scrutinizing data, especially when it spans across potentially millions of columns in a myriad of sources, is neither sustainable nor pragmatic. Machine learning (ML) techniques, employing clustering, data similarity assessment, and semantic tagging strategies, have surfaced as pivotal tools. These ML methodologies have the capacity to automate the intricacies of master data discovery and domain identification, thereby simplifying discovery processes, enhancing scalability, and bolstering productivity across the board.
In the field of master data management, the use of Artificial Intelligence (AI) for data discovery has led to the development of various advanced mechanisms and engines designed to simplify this intricate process. One notable example of such innovation is an AI engine that has been instrumental in classifying data fields by assigning semantic labels to data columns. This engine employs tagging to fundamentally streamline data discovery and labeling. For unclassified columns, users can simply assign a basic tag (e.g., “email”) to indicate the column’s content. The system then uses this information to automatically propagate similar tags across related columns, learning and adapting through associations, much like how tagging a person in one social media photo can enable the system to auto-tag the same individual in other images.
Once the domains for columns are established, sophisticated AI techniques, such as entity discovery, are employed to integrate these individual fields into comprehensive master data entities. This process might involve combining various data fields, like names, addresses, and contact details, into a single customer master data record. These AI methods learn from user interactions, observing how different data fields are combined during master data stewardship tasks. This learning is then applied to identify and formulate master data entities throughout the enterprise’s data environment.
Ultimately, the use of AI in MDM not only boosts efficiency and simplifies data management processes but also enhances the accuracy and usability of master data within the organization. This represents a significant step forward in ensuring that master data is effectively managed and utilized to support business decisions and operations.
Data lineage
In the complex ecosystem of master data management, understanding and visualizing the journey of data—its origin, movement, and transformations—holds immense significance, particularly in ensuring compliance, maintaining data quality, and executing informed business decisions. This journey, widely recognized as data lineage, can be meticulously mapped and monitored by utilizing AI technologies, which are becoming progressively instrumental in simplifying and enhancing this pivotal aspect of MDM.
AI technologies, with their capability to scan technical metadata and discern relationships through machine learning-based relationship discovery, can effectively automate data lineage mapping. This allows businesses not only to catalog the origins and types of master data but also to trace the intricate pathways through which this data traverses across various sources and applications throughout the enterprise.
In a practical context, a generic AI engine in the field of master data management can serve as an illustrative example of the capabilities encompassed in modern data management tools. Such an engine goes beyond merely cataloging master data sources and their respective domain types. It also constructs an extensive map that illustrates how master data flows among various sources and applications throughout the business landscape.
This resulting lineage map becomes a powerful tool in guiding critical business activities. It’s not just a visual representation of data flow; when enriched with additional information like linking attributes and relevant business processes, it transforms into an invaluable asset for understanding and optimizing the use of data within an organization. This kind of tool exemplifies how AI can bring a new level of sophistication and insight into the management of master data, helping organizations to better understand the relationships and dependencies that exist within their data landscape.
Such AI-assisted, business-oriented data lineage proves to be particularly vital in contexts where tracing the movement and transformation of data is not just beneficial but often regulatory. For instance, activities like “Know Your Customer” (KYC) in financial services demand a robust understanding of data movement to ensure stringent compliance with regulations. Similarly, product track and trace activities are pivotal to ensuring safety and adherence to regulatory mandates within the life sciences sector.
Consider the case of the U.S. Food and Drug Administration (FDA), which stringently tracks the entire drug supply chain, including ingredients, suppliers, manufacturers, and distributors. This thorough monitoring allows the FDA to promptly identify issues in lots, trace their ultimate destinations, and, if necessary, execute recalls to preclude potential harm. This illustrates how visualizing the complete lifecycle of data, facilitated by AI tools can bring about enhanced visibility and control in managing master data within varied industry contexts.
By employing AI for data lineage in MDM, organizations can not only attain a nuanced understanding of their data journey but also ensure a higher degree of precision, compliance, and strategic utilization of their master data, driving informed decision-making and optimized operations across the enterprise.
Data modeling
Data modeling is pivotal in various digital transformation ventures, such as digital commerce, cloud data warehousing and data lakes, and application modernization, especially within master data management. Establishing a centralized MDM hub, utilized by applications and analytical data stores as a singular source of truth, not only simplifies MDM but also enhances its scalability for both operational and analytical utilization. This mandates the hub to proficiently manage master data models, ensuring that the core attributes and hierarchies are consistent and standardized across all sources.
In this complex scenario, artificial intelligence emerges as a significant enabler, providing sophisticated and automated solutions to challenges associated with data modeling in MDM. One of the key tasks where AI proves invaluable is schema matching – a process vital to achieving coherence and alignment in data models across diverse data sources. Schema matching entails identifying and linking attributes or sets of attributes across semantically related master data models, which can be particularly difficult given the varied and dynamic nature of data across different organizational platforms.
Employing advanced techniques, such as Bayesian probabilistic methods, AI can effectively apply automated approaches to master data models cataloged during discovery. This not only assists in precisely matching attributes across disparate schemas but also enhances the accuracy and efficiency of the process by minimizing the potential for human error.
Furthermore, once the attribute matching has been conducted, AI algorithms can recommend which core attributes and hierarchy structures should be utilized in the data models based on the schema matchings. This implies that AI doesn’t merely facilitate the mechanical aspects of data modeling but also provides intelligent insights that guide decision-making in model construction, ensuring they reflect organizational data realities and needs.
Therefore, in MDM, AI significantly transcends its conventional applications, acting not only as a tool for automation but also as a guide that assists in constructing more coherent, accurate, and relevant master data models. This subsequently contributes to an organization’s ability to maintain a consistent and uniform single source of truth across various sources, enhancing the reliability and efficacy of their digital transformation initiatives. The adept inclusion of AI in the data modeling process thus ensures a more streamlined, intelligent, and strategically aligned approach to managing master data across the enterprise.
Acquisition and categorization
Master data management is inherently intricate and becomes exponentially complex with the voluminous influx of master data from varied sources. Two pivotal components in the MDM domain, namely, ‘Acquisition’ and ‘Categorization’ of master data, entail the assimilation of data and its apt categorization within the overarching data model, respectively. Within the context of artificial intelligence in MDM, these components gain enhanced efficiency, reduced error margins, and exponentially improved scalability.
In the context of master data management, the ‘Acquisition’ phase can be greatly enhanced by using AI for automated ingestion and onboarding of master data. AI technologies like genetic algorithms, named entity recognition (NER), and natural language understanding (NLU) can significantly streamline and automate the process of identifying and categorizing fields in data sources, and then aligning them with existing master data models. This process of structuring and integrating data is not limited to file-based data; it also applies to data from API endpoints and can be incorporated within application workflows. This broad applicability enhances the efficiency of business processes that share master data with customer and partner applications. AI-driven tools exemplify this capability by automating data ingestion and integration, simplifying and accelerating the acquisition stage in MDM.
Shifting the lens towards ‘Categorization,’ it is pivotal, especially in e-commerce, to map master data into the pertinent hierarchical level within the data model. Here, product categorization becomes an essential activity, influencing not only search and navigation but also impacting the quality and relevance of product recommendations produced by collaborative and content-based filtering algorithms. Since retailers routinely update voluminous product inventories, sometimes involving thousands of products in a single iteration, manual categorization becomes unfeasibly labor-intensive and is prone to errors.
AI significantly mitigates these challenges by employing machine learning approaches utilizing text classification methodologies, such as Multinomial Naïve Bayes and Support Vector Machines (SVM), for accurate and scalable product categorization. For example, an AI engine calculates the probability of a word block (e.g., product title or description) pertaining to specific product categories and, based on this probabilistic scoring, assigns the product to an appropriate level within the category hierarchy.
Integrating AI into the MDM framework, particularly in Acquisition and Categorization, alleviates the labor-intensive nature of these tasks and elevates the precision, reliability, and scalability of these processes. Consequently, this AI-enhanced approach ensures that master data is not only accurately ingested and categorized but also that it aligns seamlessly with organizational structures and processes, thus propelling operational efficiency and strategic alignment in digital commerce endeavors.
Data quality
Ensuring the impeccable quality of master data is a foundational necessity in master data management, directly influencing the credibility, accuracy, and utility of the derived insights and operational functionalities. In this context, artificial intelligence stands out as a transformative catalyst, ingeniously intertwining with various facets of master data quality assurance, thereby not only elevating the accuracy and reliability but also imparting significant automation in the associated processes.
One of the pivotal challenges associated with master data quality is ensuring its accuracy, completeness, and consistency across the board. AI navigates through this complexity by employing Natural Language Processing (NLP) and a blend of machine learning techniques, which may encompass deterministic, heuristic, and probabilistic methodologies. These technologies empower organizations to automate the complex processes of master data profiling, cleansing, and standardization, thereby simplifying the data quality assurance process while also enhancing its scalability and productivity.
Delving deeper into the realm of master data management, the use of AI engines can significantly transform data quality assurance. These engines, when used judiciously, are capable of automating key aspects of data management. They can recommend and autonomously associate data quality rules with the relevant master data fields. This enables the automation of quality assessments based on these rules.
Furthermore, these AI engines proficiently display the results through visually intuitive dashboards. They also execute tasks such as cleansing and standardization of master data across various sources within an enterprise. While specific tools can demonstrate these capabilities, the broader focus is on the instrumental role of AI in navigating the complexities of data quality within MDM. This approach underscores the potential of AI to enhance the accuracy, reliability, and overall management of master data in diverse business environments.
Furthermore, the innovative concept of “Smart Fields” has been introduced at the data model level for common types of master data fields, such as addresses, phone numbers, and emails. Implementing these context-aware fields means the system instinctively comprehends how to standardize and verify the data, providing an additional layer of data quality assurance. A simple engagement, such as clicking a checkbox, can automatically validate the information against authoritative sources, such as national postal databases, ensuring that the data adheres to established quality norms.
In a comprehensive view, AI emerges as a crucial ally in ensuring master data quality within MDM, meticulously weaving through various processes to ascertain the data’s accuracy, completeness, and consistency. It does so not by merely automating tasks but also by integrating a level of intelligence and learning, progressively improving and adapting its approaches to safeguarding master data quality in an evolving data landscape, thereby underpinning the strategic and operational objectives of enterprises in the digital age.
Optimize Your Operations With AI Agents
Optimize your workflows with ZBrain AI agents that automate tasks and empower smarter, data-driven decisions.
Match and merge
In master data management, the match and merge activity plays a pivotal role in refining data quality and integrity by meticulously identifying and consolidating duplicate records, a task that is exponentially amplified in its complexity, given the voluminous and multifaceted nature of organizational data. Here, artificial intelligence becomes instrumental, introducing precision, scalability, and a degree of automation in the matching and merging activities while accommodating an evolving data environment.
Deduplication, which is inherently linked with the match and merge activity, warrants insightful attention as it involves scouring through voluminous data across applications to identify duplicate master data records and judiciously consolidating them into a unified, authoritative version – commonly referred to as the “golden record.” A golden record epitomizes a single, well-rounded view, encapsulating accurate and holistic data extracted and consolidated from myriad sources.
In a practical context, an AI engine developed for master data management can automate the identification of duplicate master data records and suggest strategies for their consolidation. This example illustrates how AI can significantly enhance the efficiency and accuracy of master data match and merge activities. However, it’s important to note that these overarching principles are not exclusive to any single tool but can be applied across various AI technologies in MDM. The primary objective remains consistent: to improve the effectiveness of handling master data, ensuring that it is accurately consolidated and maintained across different systems and platforms.
An amalgamation of declarative and AI rules often underpin these technologies to bolster data-matching accuracy. In some MDM systems, the use of declarative rules, built upon extensive expertise in the field, aids in the process of indexing and blocking across multiple fields. This approach is designed to quickly filter out non-matching pairs, which results in a faster and more accurate matching process. In contrast, machine learning rules may employ algorithms like Random Forest Classifiers. These rely on active learning, continuously training and retraining the system to adapt to various data scenarios while maintaining high accuracy. These classifiers function based on decision trees that offer insights into the reasons behind the model’s decisions to either match or not match records. This not only enhances the effectiveness of the matching process but also adds a layer of transparency and control.
The role of AI in master data match and merge within MDM cannot be understated, serving as a linchpin that ensures data consistency, reliability, and accuracy through intelligent, automated, and scalable methodologies. While specific tools elucidate these capabilities, the broader perspective of integrating AI into master data management underscores a transformative impact, ensuring organizations navigate through the data web with agility, precision, and intelligence, thereby safeguarding and enhancing the quality and utility of their master data assets.
Data relationship discovery
In today’s digital transformation landscape, organizations are keenly focused on deepening their understanding of customer interactions and business processes. They employ various techniques such as customer experience and journey mapping, business ecosystem modeling, and value stream mapping. The goal of these methodologies is to uncover insights that might be hidden within departmental silos and to ensure that optimization strategies are aligned with overarching business outcomes, rather than favoring specific functional areas.
A key element in this approach is Master Data Relationship Discovery, especially through the use of AI in master data management. This process involves a detailed exploration of the connections among different domains of master data, such as customer, product, and supplier data. By doing so, it allows for a holistic understanding and management of end-to-end business processes.
In this context, AI engines play a crucial role in automatically orchestrating a cross-domain, inter-departmental knowledge graph by identifying relationships within master data. They use various techniques, including column signature analysis, which considers factors like metadata, uniqueness, and the presence of null values in columns. This analysis helps in identifying primary and unique keys, facilitating the inference of potential joins across master data sets. Additionally, these AI engines can delineate relationships between master data and other types of data, like transactions and interactions, enriching the contextual understanding of the data.
Furthermore, Natural Language Processing is employed to extract relevant information from unstructured data sources, such as documents and social media feeds, transforming it into a structured format for further analysis. Advanced AI techniques like Bayesian and genetic algorithms are then used to integrate this extracted information with master data records. This integration by AI not only unifies information across diverse data sources but also provides a comprehensive, detailed view of master data relationships and interactions.
The use of AI in master data management for Master Data Relationship Discovery enhances the precision, speed, and effectiveness of insight generation. This supports more informed decision-making and strategic planning in digital transformation initiatives, positioning organizations to better navigate the complexities of today’s digital business environment.
Data governance
Master Data Governance (MDG) delineates the precise management and assurance of data quality, accessibility, and security across an organization. It involves meticulously orchestrating policies, processes, and standards that dictate how data is utilized, handled, and shared within an enterprise. In this context, employing AI in master data management becomes instrumental in optimizing and automating various facets of MDG by seamlessly integrating data quality, management, and policy enforcement.
For instance, an AI engine in the field of MDM can demonstrate how AI can effectively streamline the process of linking business glossary definitions, policies, and data owners to master data. Utilizing a combination of domain discovery, data similarity analysis, and Natural Language Processing (NLP) techniques, such an AI engine can autonomously improve the productivity and accuracy of these associations. This enhancement significantly benefits cross-functional collaboration in master data governance, ensuring that various aspects of the business are seamlessly integrated and governed with a high degree of precision and efficiency.
In a generic AI context in MDM, mapping stakeholders such as data stewards, application owners, and business subject matter experts to systems containing master data harnesses visibility into who necessitates involvement in master data management stewardship. This explicit mapping is quintessential, as it not only provides clarity but also fortifies the stewardship process by ensuring that all relevant parties are both engaged and accountable for the data they manage.
AI utilized adeptly, has the potential to seamlessly automate associations, thereby proactively enhancing productivity and precision in MDG activities. Employing domain discovery and data similarity techniques assists in identifying and linking similar data elements and entities across diverse data domains, ensuring consistency in definitions and use. Meanwhile, NLP contributes by interpreting unstructured data, transmuting it into valuable insights that aid in establishing and maintaining data relationships, and ensuring policies and definitions are consistently applied and adhered to across all data domains.
In essence, incorporating AI into MDG within the scope of MDM ensures an elevated degree of automation, accuracy, and efficiency in managing data across the organization. It facilitates a unified, coherent, and collaborative approach to data governance, ensuring data quality and management are sustained and perpetually optimized in alignment with evolving business objectives and regulatory landscapes.
Privacy and protection
In the comprehensive realm of master data management, ensuring the privacy and security of master data stands paramount, especially amidst a data-centric operational and technological environment. Incorporating artificial intelligence significantly elevates the capability to safeguard, manage, and optimize sensitive and personal data through precise, automated, and adaptable mechanisms.
AI in the domain of master data privacy and security embodies a steadfast sentinel, vigilantly monitoring, identifying, and classifying sensitive data and deploying real-time protective actions to uphold the integrity and confidentiality of such data. It navigates through the dense and multifaceted data landscape, detecting sensitive and personal information, associating it with respective privacy policies, and dynamically enforcing relevant security rules to shield the data from unauthorized access and usage.
To illustrate, consider an AI engine in the master data management process. Throughout the master data discovery phase, such an AI engine meticulously identifies and classifies sensitive and personal data. Its functionality extends beyond mere identification; it also associates this data with relevant privacy policies and dynamically maps enforcement rules to ensure real-time data protection. For example, based on established privacy policies and user authorizations, master data can be dynamically masked at the point of query, ensuring that sensitive information remains accessible only to authorized personnel, thereby enhancing data security.
Furthermore, the capabilities of this AI engine include real-time protection during API-based master data exchanges integral to business processes. It accurately identifies sensitive elements, like credit card numbers and email addresses, within APIs and immediately applies predefined privacy rules. This ensures that the data is transmitted and handled within secure and compliant frameworks, maintaining strict adherence to privacy standards and regulations. This approach exemplifies how AI-driven technologies can be leveraged to safeguard sensitive data in complex business environments, ensuring both security and compliance.
Broadly speaking, AI significantly enhances the efficacy and robustness of master data privacy and security within MDM. By utilizing intelligent algorithms, AI systems navigate through vast and varied data, detecting and safeguarding sensitive information while ensuring that this data is managed and utilized within the strict confines of organizational and regulatory policies. This dynamic, automated, and intelligent approach ensures that data privacy and security are not only upheld but also continuously optimized in response to evolving data landscapes and regulatory requirements.
In essence, the infusion of AI into master data privacy and security within MDM represents a robust, secure, and forward-looking approach, ensuring that master data is perpetually shielded, compliant, and optimized in its journey through the intricate and evolving digital ecosystem. This integration fortifies data security and catalyzes a systematic, intelligent, and adaptable data management strategy, poised to navigate future challenges and opportunities in the data-driven world adeptly.
Data sharing and use
Advancing towards an era where data has become the linchpin for decision-making and strategic endeavors, the sharing and utilization of master data within an organizational ambit play a critical role in orchestrating consistent and insight-driven operations. Employing AI in master data management elevates the efficacy and strategic utility of sharing and employing master data by embedding intelligent automation, predictive analytics, and dynamic data management throughout the data lifecycle.
AI amplifies the capabilities and productivity of data curators, scientists, and business analysts by embedding a layer of intelligence and adaptability within the processes of preparing and utilizing data for analytical pursuits. It harmonizes the complexity and diversity of data, proactively recommends pertinent master data, and ensures that data sharing and usage are conducted within a framework that is efficient and securely compliant with data governance protocols.
For instance, an AI engine in the realm of master data management distinguishes itself by incorporating content-based filtering and analyzing data characteristics, such as data lineage, user ranking, and data similarity. This analysis enables the engine to make informed recommendations about which master data should be prioritized for use. As a result, data stakeholders can access the most relevant and insightful data, aiding them in navigating through a data landscape that is optimally arranged to support their analytical and operational activities.
Additionally, such an AI engine demonstrates adeptness in managing the compliance and ethical utilization of master data. It dynamically applies data usage terms and conditions based on the type of master data being accessed. This feature ensures that data consumers are continually informed about how to use sensitive and private data in a way that aligns with both organizational and regulatory requirements. Upon acceptance of these usage policies, the AI engine can automate the provisioning of master data sets into respective destinations, like cloud data lakes. This ensures that data is used within compliant parameters and is efficiently managed and transported across the data ecosystem, maintaining both integrity and compliance.
From a broader perspective, AI injects a systematic, intelligent, and adaptable framework into master data sharing and usage within MDM. The intelligent algorithms navigate through the complexities of data, ensure that data consumers are accessing and utilizing data that is relevant and compliant with data governance norms, and automate the management and movement of data across the organizational data landscape.
In essence, the integration of AI into the sharing and usage of master data underpins a strategy that is securely compliant, insightfully relevant, and adaptively efficient. It nurtures a data ecosystem where data is not merely shared and used but managed, moved, and utilized to perpetually optimize the value, security, and compliance throughout the data lifecycle. This synthesis of AI and MDM propels organizations towards a future where data is not merely an asset but a strategic, intelligent, and adaptable ally in navigating the complexities and opportunities of the digital realm.
Optimize Your Operations With AI Agents
Optimize your workflows with ZBrain AI agents that automate tasks and empower smarter, data-driven decisions.
Advantages of using AI in master data management: An analytical perspective
Artificial Intelligence, characterized by its capacity to emulate cognitive faculties such as learning and problem-solving, stands out as a transformative ally in enhancing master data management. The amalgamation of AI and MDM paves the way for a symbiotic relationship, enhancing enterprises’ efficiency, accuracy, and insightfulness of data management practices.
Accelerated data processing and enhanced accuracy
- Elevated processing speed: AI algorithms are adept at parsing through voluminous data expeditiously, ensuring that insights derived from data are timely and actionable.
- Minimized human error: The automation provided by AI negates the inherent potential for human error, ensuring data accuracy and consistency across large datasets.
Identification of patterns and insightful data analytics - Unveiling hidden trends: AI aids in discerning patterns and trends within data that may remain obscure to human analysts, fostering an enriched understanding and, subsequently, informed decision-making.
- Optimization of business strategies: The insights generated through AI’s analytics can illuminate pathways to refine marketing strategies, product designs, and other business operations, rendering them more attuned to prevailing consumer behaviors and trends.
Enhanced data governance and security
Automated data quality assurance: Employing AI for tasks like data quality checks ensures that data adheres to prescribed standards, maintaining its accuracy and consistency across the enterprise.
Robust data security: AI algorithms can also bolster data security measures, safeguarding against breaches and ensuring data management adheres to pertinent regulatory compliances.
Revitalizing data cleansing and validation through AI
Automated error rectification: Machine learning algorithms can systematically identify and amend errors or inconsistencies within the data, thereby automating the data cleansing process.
Categorized and structured data: AI’s capability to classify and categorize data ensures a systematic and coherent organization, thereby streamlining subsequent analyses.
Data stewardship support: AI can proactively assist data stewards by proposing corrections or categorizations, which the stewards can review and implement, enhancing the precision and efficacy of the data cleansing process.
Automated and intelligent data enrichment
Attribute completion: AI’s predictive capabilities can be leveraged to discern and complete missing attributes within data by identifying patterns and correlations within existing datasets.
Expansive data utilization: The AI algorithms can extrapolate relevant data from various sources, such as textual documents and social media, thereby enriching the available data for analysis and ensuring a more holistic view.
Symbiotic relationship with data: As AI systems process data, they refine their understanding of underlying patterns and relationships, progressively enhancing their predictive and analytical capabilities.
By intertwining AI’s analytical and predictive prowess with MDM, organizations are empowered to navigate through the complex web of their data ecosystems with heightened insight, accuracy, and efficiency. The automated capabilities of AI not only mitigate potential errors and enhance processing speeds but also unlock deeper, more comprehensive insights from the data, furnishing businesses with the intelligence to navigate toward more informed, strategic decision-making. Consequently, AI in master data management does not merely represent a technological enhancement; it signifies a strategic evolution in managing, understanding, and deriving value from organizational data.
How can AI be used in different stages of master data management?
The application of AI in master data management underscores the transformative effect of integrating advanced technology within traditional data management practices. AI enhances MDM with automated data collection, cleaning, predictive analytics, and data quality improvement, leading to increased accuracy, foresight, and alignment with organizational goals. These advancements enable businesses to navigate complex data landscapes with greater precision, agility, and intelligence.
Data collection, cleaning, and processing with AI
- Automated data collection:
- Web scraping: AI systems utilize web scraping techniques to gather data from various online sources autonomously, ensuring a rich and diverse data pool.
- IoT data collection: AI algorithms can extract and process data from Internet of Things (IoT) devices, enhancing real-time data utility.
- AI-driven data cleaning:
- Error detection: AI algorithms systematically identify errors and discrepancies in the data, such as duplicates or inconsistencies.
- Auto-correction: AI can predict and implement corrections automatically by leveraging historical and correlational data.
- Data processing and preparation:
- Normalization: AI algorithms assist in standardizing data, ensuring a coherent format across diverse datasets.
- Transformation: AI can automate data transformation into usable formats, ensuring compatibility with analytical models.
Data quality analysis with machine learning algorithms
- Consistency checks:
- Cross-validation: Machine learning models cross-validate data entries with predefined rules or historical data to ensure reliability and consistency.
- Pattern recognition: Algorithms are used to discern patterns within data, identifying and addressing aberrations that might indicate data quality issues.
- Data profiling:
- Statistical analysis: Leveraging statistical models, AI examines data for distribution trends, mean, mode, and other metrics to ensure data quality.
- Anomaly detection: Machine learning models identify anomalies within data, flagging potential issues for further examination.
- Data validation:
- Constraint checking: AI systems enforce predefined constraints to maintain data integrity and validity.
- Semantic validation: Utilizing NLP, AI ensures that data adheres to semantic rules, guaranteeing logical consistency.
Predictive analytics and data forecasting with AI
- Predictive modeling:
- Regression analysis: AI utilizes regression models to predict future data points based on historical trends and data relationships.
- Classification models: AI classifies data into predefined categories, facilitating predictive analytics.
- Data forecasting:
- Time series analysis: AI models analyze time-sequenced data to forecast future trends, enabling businesses to anticipate shifts and strategize accordingly.
- Demand forecasting: AI analyses past usage trends, consumer behaviors, and market dynamics to predict future demand.
- Prescriptive analytics:
- Decision trees: AI employs decision tree models to explore potential outcomes of different strategic approaches.
- Optimization algorithms: Through assessing various scenarios and outcomes, AI recommends optimal strategies and operations to attain business objectives.
LeewayHertz’s AI development services for master data management
At LeewayHertz, we develop tailored AI solutions designed specifically for the complexities of master data management (MDM). Our strategic AI/ML consulting services empower organizations to enhance data quality, improve data governance, and enable more effective data integration strategies.
Our proficiency in creating Proof of Concepts (PoCs) and Minimum Viable Products (MVPs) allows companies to explore the practical benefits of AI in managing vast amounts of master data across different data domains, ensuring that the solutions are effective and precisely meet organizational needs.
By leveraging generative AI, we transform the management of master data, automating data cleansing, de-duplication, classification and categorization and data enrichment processes to free up human resources for more strategic data governance roles.
We refine large language models to the specific terminologies and workflows of master data management, significantly enhancing the accuracy and utility of AI-driven data processing and analysis.
Furthermore, we ensure these AI systems integrate seamlessly with existing data management infrastructures, boosting operational efficiency and strategic decision-making in data management practices.
Our AI solutions development expertise
AI solutions in master data management focus on creating robust systems that enhance data accuracy, streamline data consolidation, and automate routine data management tasks. These solutions incorporate advanced machine learning algorithms to improve data categorization and enable predictive data maintenance. Key areas of application include data integration, quality control, and governance.
Overall, our AI solutions aim to enhance the reliability of master data, reduce manual data handling errors, and improve overall operational efficiency in data management.
AI agent/copilot development for master data management
LeewayHertz builds custom AI agents and copilots to enhance various aspects of master data management, helping companies streamline operations, maintain high data quality, and make more efficient data-driven decisions. Here’s how they help:
Data quality enhancement:
- Automatically detect and correct inaccuracies or inconsistencies in master data.
- Identify and merge duplicate data entries without manual intervention.
Governance and compliance:
- AI agents help ensure adherence to data governance policies by automatically applying rules and regulations across all data sets.
- AI agents continuously monitor data to ensure compliance with both internal standards and external regulations.
Integration and interoperability:
- Facilitate the integration of disparate data sources, ensuring unified data views that are accessible across the organization.
- Predict and manage the relationships between different data entities, improving data linkage and usability.
Process automation:
- Automate repetitive tasks such as data entry and report generation to increase efficiency and reduce human error.
- Implement AI-driven workflows that adapt to changing data requirements and business conditions.
Strategic decision making:
- Analyze patterns in master data and predict future trends, aiding strategic planning.
- Provide deep insights into data integrity and operational effectiveness to inform business decisions.
AI agents and copilots developed by LeewayHertz not only increase the efficiency of master data management processes but also significantly enhance the quality of data and strategic decision-making within organizations. By integrating these advanced AI solutions into their existing data management frameworks, companies can achieve a significant competitive advantage, navigating the complex landscape of data management with innovative, efficient, and reliable AI-driven tools and strategies. This leads to improved data quality, enhanced compliance, and optimized business operations, ultimately driving better outcomes and higher organizational performance.
Endnote
In the intricate world of data management, the alliance between AI and MDM has surfaced as a vital driver for transforming data into actionable insights and informed decision-making. This union brings forth an era where data is not merely stored and managed but meticulously utilized, unlocking extensive potential across various organizational operations.
AI introduces to MDM an environment where data quality, security, and consistency are not just standard practices but are elevated to a level where every bit of data intrinsically carries value. From seamlessly automating entity resolution to assuring data privacy and enhancing data quality management, AI becomes an essential cog in the MDM system, guiding through the sophisticated data networks with predictive and analytical acumen.
Peering into the future, the union of AI and MDM promises an agile, predictive, and adaptive data management landscape. Here, real-time, AI-fueled MDM doesn’t only act as a bridge between the present and the future but also pioneers new directions, making data a fundamental enabler of informed decisions and innovative advancements.
The journey from raw data to insightful wisdom is elegantly navigated by the collaboration between AI and MDM, creating a scenario where organizations become adept architects of their data ecosystems. They shape their data realms with a precision informed by AI and safeguarded by sturdy MDM practices. As we step forward into this new era, the expectation is not just of a future that is steered by data but of an odyssey where every data interaction is a pivotal move toward innovation and steadfast growth.
By acknowledging the significance of AI in master data management today, we are not only boosting our current capabilities but also scripting a future where data doesn’t just support, but propels, organizational communication and success into new dimensions.
Unlock the power of your data with LeewayHertz’s AI-driven MDM solutions – connect with us and elevate your data management today!
Start a conversation by filling the form
All information will be kept confidential.
Insights
Understanding generative AI models: A comprehensive overview
Generative AI is a technology used to generate new and original content. It leverages advanced algorithms and neural networks to autonomously produce outputs that mimic human creativity and decision-making.
Generative AI architecture for enterprises: Development frameworks, tools, implementation, and future trends
By understanding the architecture of generative AI, enterprises can make informed decisions about which models and techniques to use for different use cases.
Generative video model: Types, tasks, development and implementation
Generative video models are machine learning algorithms generating new video data based on patterns learned from training datasets. Explore more about it!