AI in LTV model: Applications, ML techniques and importance in business deicsion making
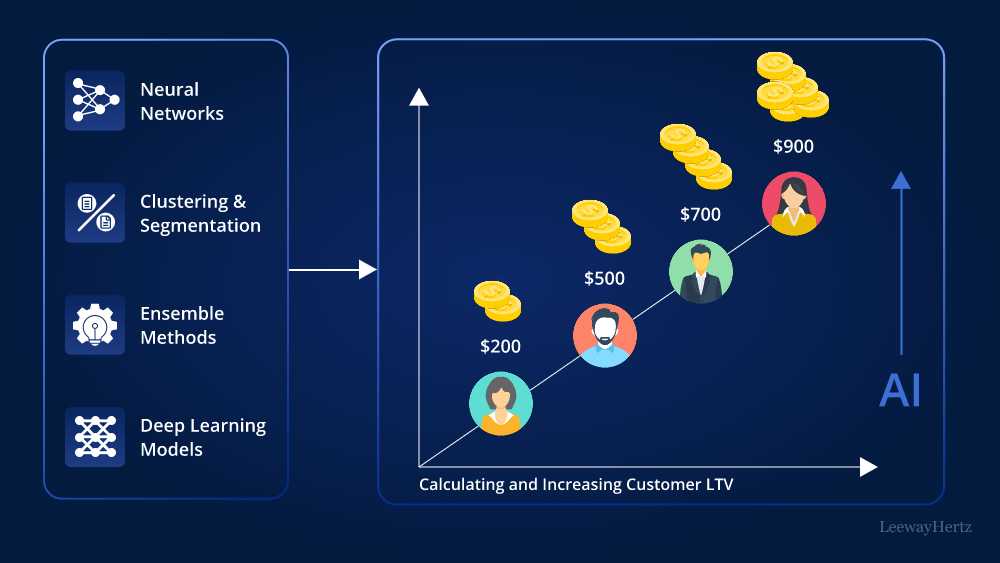
What if you could foresee the future behaviors of your customers? How would this power reshape your marketing strategies, sales tactics, and resource allocations? Imagine the impact on your business if you could identify the customers who will remain fiercely loyal versus those who might slip away after a single purchase. It’s time to harness the potential of Customer Lifetime Value (CLV) prediction, the game-changing metric that is redefining acquisition and retention marketing strategies.
In today’s dynamic business landscape, understanding the essence of CLV has emerged as the north star guiding successful organizations. Acquiring a new customer is expensive and time-consuming. It can cost five times more than retaining an existing customer. The money spent on acquiring a new customer ranges from 5 to 25 times that of retaining an existing one. Also, elevating customer retention by 5% can increase profits from 25-95%. The implications are clear and profound—optimizing your Customer Lifetime Value (LTV) can be the catalyst for sustained business growth.
However, despite this compelling logic, the spotlight often gravitates towards customer acquisition, leaving a void in efforts to nurture and enhance the value of existing customers. This brings us to a pivotal juncture in modern business strategy. The question arises: How can companies make informed decisions about customer retention, acquisition, and marketing investments?
Here comes LTV prediction into play, which is powered by Machine Learning (ML) and Artificial Intelligence (AI). In an era where data reigns supreme, LTV serves as a compass, leading organizations toward their most profitable customers. This predictive power can redefine the way businesses allocate resources, fortify customer loyalty, and unlock growth.
This article delves into the intricate realm of AI in LTV prediction and its pivotal role in shaping business strategic decisions. From exploring the challenges of traditional LTV prediction to unveiling the transformative influence of AI and Machine Learning, the article navigates through diverse lifetime value models, advanced techniques, and real-world applications across industries. By unraveling the complexities of LTV prediction, readers gain insights into its profound impact on modern business landscapes.
- What is Customer Lifetime Value?
- Importance of LTV prediction in business decision-making
- Challenges of traditional customer lifetime value (LTV) prediction
- Applications of LTV models across various industries
- The role of AI and ML in redefining LTV predictions
- ML techniques used for LTV prediction
- How to predict customer LTV using machine learning?
- LeewayHertz’s AI development services for building AI-powered LTV models
- Examples of AI-enhanced customer LTV prediction and utilization
- The future of LTV prediction: Navigating the evolving landscape
What is Customer Lifetime Value?
Lifetime Value (LTV), or Customer Lifetime Value (CLV), is a crucial metric that quantifies the total value a customer brings to a business over the entire duration of their relationship with the company. It is an essential concept in modern marketing and business strategies, providing valuable insights into customer profitability and informing decision-making processes. LTV considers the revenue generated from their purchases minus the costs associated with acquiring and serving the customer. The core idea is to determine the long-term impact of a customer on a company’s bottom line rather than just focusing on short-term transactions.
Components of LTV prediction
LTV prediction is a complex process that takes into account various customer behaviors and attributes:
- Purchase history: Understanding a customer’s past purchase behavior is crucial. This includes the frequency of purchases, the monetary value of transactions, and the products or services they’ve bought.
- Customer engagement: How frequently a customer interacts with the business, engages with marketing campaigns, and interacts with customer support plays an important role in predicting their value over time.
- Churn probability: Predicting the likelihood of a customer churning (stopping their engagement) is a critical component. A customer who stays engaged for a longer period contributes more to LTV.
- Demographics and behavior: Customer characteristics like age, location, and preferences influence their spending behavior. Incorporating these attributes helps tailor predictions to specific customer segments.
Optimize Your Operations With AI Agents
Optimize your workflows with ZBrain AI agents that automate tasks and empower smarter, data-driven decisions.
Importance of LTV prediction in business decision-making
Strategic marketing allocation: Understanding LTV allows businesses to allocate marketing resources more effectively. High-LTV customers deserve more attention and investment, as they have the potential to yield greater returns over time. On the other hand, customers with lower LTV might not warrant the same level of marketing spend.
Customer segmentation and personalization: LTV helps in segmenting customers based on their potential value to the business. This enables companies to tailor their marketing efforts and services to different segments. High-LTV customers might receive exclusive offers, loyalty programs, and personalized experiences, fostering stronger relationships and increasing retention.
Optimized customer acquisition: By knowing the LTV of different customer segments, businesses can make well-informed decisions about how much to invest in new customer acquisition. It helps in calculating the maximum acceptable cost for customer acquisition, ensuring that the company doesn’t overspend on acquiring low-value customers.
Pricing strategies: LTV plays a crucial role in pricing decisions. Companies can set prices that align with the expected value a customer will bring over their lifetime. Premium pricing for high-LTV customers and value-based pricing for lower-LTV segments can be employed.
Churn prediction and retention strategies: Predicting when a customer is likely to churn (stop engaging with the business) is essential for retention efforts. By identifying at-risk customers, companies can implement targeted retention strategies to prolong their customer lifespan and increase LTV.
Product and service development: LTV insights can guide new product or service development decisions. Understanding what high-LTV customers value can help in creating offerings that cater to their preferences, leading to increased satisfaction and loyalty.
Investment decisions: LTV influences long-term business decisions such as expansion, partnerships, and mergers. Companies can assess potential opportunities based on their impact on overall customer value.
Financial forecasting: LTV provides a basis for financial projections, allowing businesses to estimate future revenue and profitability accurately.
Challenges of traditional customer lifetime value prediction
Enterprises often face several challenges when attempting to predict LTV using traditional methods. Here are the challenges they encounter:
- Data quality and availability: Enterprises often struggle to gather accurate and comprehensive data about their customers. LTV prediction requires a wide range of data, including purchase history, customer interactions, demographic information, and more. Incomplete, inconsistent, or inaccurate data can lead to unreliable predictions.
- Data integration: Enterprises may have data stored in various systems and platforms, making consolidating the data for analysis challenging. Integrating data from different sources is a complex task that can result in errors and inconsistencies if not handled properly.
- Customer behavior changes: Customer behaviors and preferences are not static. They evolve over time due to changing market trends, economic conditions, competition, and external events. Traditional LTV models may struggle to adapt to these changes quickly.
- Lack of personalization: Traditional LTV models often treat all customers in the same way, not accounting for individual variations in behavior and preferences. Personalization is crucial for accurate LTV prediction, as different customers have different spending patterns and engagement levels.
- Dynamic customer journeys: The path a customer takes from discovery to purchase is rarely linear. They might interact with the business through multiple touchpoints (website, social media, physical store), making it challenging to track and analyze their journey accurately.
- Short-term focus: Many traditional LTV models are designed for short-term predictions rather than estimating long-term LTV. Focusing solely on immediate returns can lead to missed opportunities for nurturing high-potential customers.
- High customer churn: Customer churn (attrition) can significantly impact LTV predictions. If the lifetime value model doesn’t account for churn accurately, it might overestimate the value of customers who are likely to leave soon.
- Market shifts and external factors: Changes in the market, technological advancements, economic downturns, or unexpected events can disrupt customer behavior and render historical data less relevant for predicting future LTV.
Addressing these challenges often requires a shift towards more advanced techniques like machine learning and AI, which can handle complex patterns, adapt to changes, and incorporate more data sources for better prediction accuracy.
The role of AI and ML in redefining LTV predictions
The landscape of business and marketing has undergone a profound disruption with the advent of AI and ML technologies. This transformation is particularly evident in the realm of Lifetime Value (LTV) predictions. AI and ML have reshaped the way businesses estimate and leverage LTV, offering unprecedented accuracy, insights, and personalized strategies. Let’s delve into the emerging role of AI and ML in reshaping LTV predictions.
Enhanced customer understanding through advanced analytics
AI-driven LTV models possess the ability to analyze extensive data from a variety of sources. This empowers companies to develop a thorough comprehension of customer actions and patterns. Traditional LTV models rely on limited historical data, whereas AI-driven lifetime value models can analyze complex data sets, including transaction history, browsing patterns, social media interactions, etc. This holistic approach results in a richer understanding of each customer’s preferences, allowing for more accurate LTV predictions.
Improved segmentation and personalization
AI and ML techniques excel in customer segmentation, which is crucial for tailoring marketing strategies to specific groups. These technologies can identify subtle patterns within customer data, enabling businesses to create micro-segments with unique characteristics. By understanding these segments’ preferences, buying habits, and engagement patterns, companies can craft highly personalized experiences and offers that resonate with individual customers, ultimately boosting their LTV.
Dynamic LTV predictions
Traditional LTV models often assume static customer behavior, overlooking the dynamic nature of customer interactions. AI and ML-powered LTV models, on the other hand, can capture the evolving relationships between businesses and customers. By incorporating real-time data and adapting to changes in customer behavior, these LTV models provide more accurate and up-to-date LTV predictions.
Churn prediction and proactive retention
Churn prediction, the ability to anticipate when a customer is likely to disengage, is a critical aspect of LTV optimization. AI and ML algorithms can identify subtle cues that precede churn, such as reduced engagement or changes in purchase patterns. Businesses can implement proactive retention strategies by predicting potential churn, thereby extending the customer’s lifetime value.
Complex pattern recognition
AI and ML techniques are adept at uncovering intricate patterns within data that traditional methods might miss. They can identify non-linear relationships between variables and unveil hidden insights that contribute to LTV. For instance, AI can uncover correlations between seemingly unrelated behaviors, helping businesses make informed decisions about customer engagement strategies.
Real-time personalized recommendations
AI-driven LL models can power real-time personalized recommendations. By analyzing a customer’s current behavior, historical data, and contextual information, businesses can offer relevant suggestions, discounts, or products that align with the customer’s preferences. This increases immediate sales and enhances customer loyalty, ultimately boosting LTV.
Predictive analytics for upselling and cross-selling
AI and ML-powered predictive analytics empower businesses to anticipate what products or services a customer might be interested in next. This ability to predict future purchases supports effective upselling and cross-selling strategies, maximizing the value extracted from each customer.
By harnessing the power of AI and ML, businesses can uncover hidden insights, engage customers more effectively, and make data-driven decisions that elevate customer value over time.
Optimize Your Operations With AI Agents
Optimize your workflows with ZBrain AI agents that automate tasks and empower smarter, data-driven decisions.
ML techniques used for LTV prediction
Customer Lifetime Value (LTV) prediction is crucial in marketing and business analytics. Various machine learning techniques can be employed to predict LTV. The choice of technique depends on the available data, the problem’s complexity, and the desired level of accuracy. Here are some commonly used ML techniques for LTV prediction:
Regression models:
- Linear regression: Linear regression is a simple and widely used technique for predicting a continuous target variable based on one or more input features.
- Ridge and Lasso regression: These regularization techniques can handle multicollinearity and prevent overfitting.
- Gradient boosting regression: Models like XGBoost, LightGBM, and CatBoost can capture complex nonlinear relationships.
Time series analysis:
- Autoregressive Integrated Moving Average (ARIMA): Suitable for modeling time-dependent data with trends and seasonality.
- Exponential Smoothing (ETS): Good for capturing seasonality and trend patterns in time series data.
Survival analysis:
- Cox proportional hazards model: Useful when considering time-to-event data, such as predicting when customers might churn or make a purchase.
- Kaplan-Meier estimator: Used to estimate survival curves in non-parametric survival analysis.
Neural networks
- Feedforward Neural Networks: These can capture complex nonlinear relationships but might require more data and tuning.
- Recurrent Neural Networks (RNNs): Useful for sequential data where past interactions impact future behavior.
- Long Short-Term Memory (LSTM) Networks: A type of RNN that can model longer-term dependencies.
Clustering and segmentation
- K-Means clustering: Can help segment customers based on similar behavior and characteristics.
- Hierarchical clustering: Can reveal hierarchical relationships among customer segments.
Feature engineering
- Creating custom features based on domain knowledge can enhance the predictive power of models. Features like recency, frequency, monetary value (RFM), and customer demographics can be useful.
Ensemble methods
- Random forest: Averages predictions from multiple decision trees to improve accuracy and generalization.
- Stacking: Combines predictions from multiple models to create a stronger ensemble prediction.
Deep learning models
- Autoencoders: Can learn a compact representation of customer behavior and use it for prediction.
- Variational Autoencoders (VAEs): Can capture underlying distributions in the data and generate new samples.
Matrix factorization
- Techniques like Singular Value Decomposition (SVD) and Alternating Least Squares (ALS) are commonly used in collaborative filtering for recommendation systems. While primarily associated with recommendations, these techniques indirectly influence LTV predictions by enhancing customer experiences and personalizing interactions, which can lead to improved customer retention and loyalty.
The effectiveness of these techniques depends on factors like data quality, feature engineering, model tuning, and the specific characteristics of the business problem. It’s often recommended to try several techniques and compare their performance to find the best approach for a given LTV prediction task.
How to predict customer LTV using machine learning?
Predicting customer lifetime value (LTV) through machine learning involves several steps, from data collection and preprocessing to model selection and evaluation. Here’s a guide to help you through the process in detail:
Step 1: Define your objective
Before diving into the technical aspects, it’s crucial to understand the objective. Customer Lifetime Value (LTV) prediction aims to estimate the potential revenue a customer will generate throughout their relationship with your business. LTV prediction using machine learning helps businesses allocate resources effectively, tailor marketing efforts, and make strategic decisions to maximize profitability.
Step 2: Data collection and preprocessing
- Data gathering: Collect historical customer data, including transaction history, purchase amounts, transaction frequency, demographics, and other relevant information.
- Data cleaning: Handle missing values, duplicates, and outliers. Inaccurate or incomplete data can detrimentally affect the model’s performance.
- Feature engineering: Create meaningful features that can influence LTV, such as RFM (Recency, Frequency, Monetary) metrics, customer demographics, and behavioral features. For instance, calculate the time since the customer’s first purchase, the average transaction amount, and the total number of purchases.
- Normalization and scaling: Normalize numerical features to ensure they have similar scales, preventing some features from dominating the model due to their larger values.
- Encoding categorical features: Convert categorical variables into a numerical format using one-hot or label encoding techniques.
Step 3: Data preparation
This step involves the splitting of the dataset into training and testing sets. A common split is 80% for training and 20% for testing. This helps you evaluate your model’s performance on unseen data.
Step 4: Model selection
Choose an appropriate algorithm for LTV prediction. Common choices include:
- Regression models: Ridge Regression, Linear Regression, Lasso Regression
- Tree-based models: Decision Trees, Random Forest, Gradient Boosting Trees
- Neural networks: Deep Learning models can capture complex patterns
The choice of the algorithm depends on the nature of your data, the complexity of the relationships you want to capture, and the computational resources available.
Step 5: Feature selection
Identify the most influential features for LTV prediction. This can be done through techniques like correlation analysis or feature importance from tree-based models.
Step 6: Model training
Train the selected model on the training data. Make sure to use appropriate hyperparameters and techniques to avoid overfitting. Consider techniques like cross-validation to find the best hyperparameters.
Step 7: Model evaluation
Evaluate your model’s performance using appropriate metrics for regression tasks:
- Mean Squared Error (MSE): Evaluates the average of the squared disparities between predicted and observed values.
- Root Mean Squared Error (RMSE): It is referred to as the square root of MSE, offering a more easily understandable scale for evaluating the model’s prediction accuracy.
- R-squared (R2): R-squared (R2), also known as the coefficient of determination, is a statistical metric employed in regression analysis to evaluate the goodness of fit of a regression model.
These metrics quantify how well your model’s predictions match actual LTV values.
Step 8: Hyperparameter tuning
If you’re using algorithms with tunable hyperparameters (like regularization strength in regression models or the number of trees in a random forest), perform hyperparameter tuning using techniques like grid search or random search to find the optimal settings.
Step 9: Predictions and interpretation
Once your model is trained and assessed, you can utilize it to make predictions on new data. Interpret the results to understand which features are most important in predicting customer LTV. This can provide valuable insights for business strategies.
Step 10: Model deployment
Deploy your trained model to make real-time predictions. This can be done through APIs or integration with your enterprise systems.
Step 11: Monitoring and maintenance
Regularly monitor your model’s performance in a production environment. Retrain the model periodically with new data to ensure it remains accurate over time.
Predicting LTV using machine learning is a multi-step process that involves collecting, preprocessing, and analyzing data, selecting and training a suitable model, and ultimately using the model’s predictions to inform business decisions. This process requires a combination of data science, domain knowledge, and ongoing optimization to effectively harness the power of machine learning for enhancing customer relationships and driving business growth.
Applications of LTV models across various industries
AI has found numerous applications in customer lifetime value (LTV) modeling across various industries. Let’s explore AI applications in LTV modeling across different industries:
E-commerce
AI-driven LTV models in e-commerce can analyze customer behavior, purchase history, and interactions with the website. By leveraging machine learning algorithms, businesses can predict which customers are likely to make repeat purchases and become high-value customers. This allows them to tailor personalized marketing campaigns, recommend products, and optimize pricing strategies. For example, Amazon uses AI to recommend products, leveraging insights from customers’ past purchases and browsing history, enhancing customer engagement and increasing LTV.
Subscription services
In industries like streaming services (e.g., Netflix), subscription-based models rely on accurate LTV predictions to optimize content recommendations, reduce churn, and improve customer retention. AI analyzes viewing patterns, user preferences, and engagement metrics to predict which subscribers are likely to stay engaged and renew their subscriptions. By targeting these customers with tailored content, subscription services can increase LTV. Netflix’s recommendation engine uses AI to suggest shows and movies based on user viewing habits, enhancing user satisfaction and LTV.
Retail
Retail businesses can leverage AI to analyze customer purchase history, preferences, and online interactions to identify high-value customers. AI-powered lifetime value models can segment customers based on their buying behavior, allowing retailers to design targeted loyalty programs, personalized offers, and effective retention strategies. For instance, Starbucks uses AI to analyze customer preferences and send personalized offers through its mobile app, driving customer retention and increasing LTV.
Gaming
In the gaming industry, AI can analyze player behavior, gameplay patterns, and in-game interactions to predict which players are likely to spend more time and money on the platform. By identifying high-value players, gaming companies can offer personalized rewards, in-game incentives, and targeted advertisements to enhance engagement and increase LTV. Epic Games, the creator of Fortnite, uses AI to analyze player data and customize in-game experiences, leading to higher player retention and LTV.
Financial services
AI-driven lifetime value models in the financial sector can analyze customer transaction history, account activity, and financial behavior to predict which clients are likely to utilize multiple services and products over the long term. Banks can offer personalized financial advice, customized product recommendations, and loyalty programs to increase customer retention and LTV. For example, Capital One uses AI to provide personalized credit limit increases and targeted rewards, improving customer satisfaction and LTV.
Telecommunications
Telecommunication companies can use AI to analyze customer call patterns, data usage, and service history to predict which customers are likely to upgrade their plans, adopt additional services, or remain loyal subscribers. By offering personalized bundles, promotions, and proactive customer support, telecom companies can increase LTV. Verizon employs AI-powered analytics to understand customer preferences and tailor their service offerings, leading to higher customer retention and LTV.
Hospitality and travel
AI can analyze customer booking history, preferences, and feedback in the hospitality and travel industry to predict which travelers are more likely to become loyal customers. Hotels and airlines can offer personalized packages, exclusive offers, and tailored recommendations to enhance customer satisfaction and increase LTV. For instance, hotels use AI to analyze guest preferences and offer customized room amenities, resulting in improved guest experiences and repeat bookings.
Automotive
The automotive industry can use AI to analyze customer vehicle preferences, maintenance history, and usage patterns. This information can help manufacturers and dealerships predict which customers are more likely to upgrade to new models, purchase accessories, and continue using maintenance services. By offering personalized offers and services, automotive businesses can boost customer retention and LTV. Tesla, for example, uses AI to analyze driving data and provide software updates tailored to individual vehicle needs, enhancing the overall ownership experience.
Healthcare
Healthcare providers can leverage AI to analyze patient medical histories, treatment plans, and interactions with healthcare services. Healthcare organizations can implement personalized communication strategies and targeted wellness programs to improve patient outcomes and increase LTV by predicting which patients are more likely to continue seeking medical care and adhering to treatment regimens.
Insurance
The insurance industry can use AI to analyze customer policy history, claims data, and interactions with insurance services. By identifying customers who are more likely to renew policies, expand coverage, and engage with additional insurance products, companies can tailor their communication strategies and offer discounts or rewards to increase customer retention and LTV.
Energy and utilities
AI-driven lifetime value modeling can be applied to analyze customer consumption patterns, usage history, and engagement with energy-saving programs in the energy and utilities sector. Utility companies can identify customers who are likely to adopt energy-efficient solutions, participate in loyalty programs, and continue using their services. Utility providers can enhance customer satisfaction and LTV by offering targeted energy-saving advice and rewards.
Pharmaceuticals
AI can analyze patient medication history, adherence rates, and interactions with healthcare professionals in the pharmaceutical industry. This information can help pharmaceutical companies identify patients who are more likely to remain on their prescribed medications, allowing them to offer support programs, reminders, and educational resources to improve patient adherence and increase LTV.
AII applications in LTV modeling span a wide range of industries, thereby allowing businesses to make data-driven decisions, enhance customer engagement, and optimize strategies for customer acquisition and retention. These applications ultimately lead to increased customer lifetime value and improved business performance.
Optimize Your Operations With AI Agents
Optimize your workflows with ZBrain AI agents that automate tasks and empower smarter, data-driven decisions.
LeewayHertz’s AI development services for building AI-powered LTV models
At LeewayHertz, we are pioneering the development of AI-driven Lifetime Value (LTV) models that mark a new era in customer value prediction. Our strategic AI/ML consulting and advanced analytics capabilities empower businesses to use artificial intelligence to forecast long-term customer behavior and optimize marketing strategies for maximum ROI.
Our expertise in developing Proof of Concepts (PoCs) and Minimum Viable Products (MVPs) allows businesses to test the practical impact of AI tools, ensuring that solutions are effective, innovative, and tailored to meet specific operational challenges. This step is crucial in demonstrating the viability of AI in real-world applications and helps refine the technology to better suit business needs.
Our deep expertise in generative AI extends to automating routine tasks such as data entry, customer service responses, and content generation. This automation frees up human resources for higher-level strategic roles and creative thinking, significantly enhancing operational efficiency.
By fine-tuning large language models and other AI technologies to industry-specific needs, LeewayHertz enhances the accuracy, speed, and relevance of automated tasks. This fine-tuning process ensures that our AI solutions are effective and directly aligned with our client’s operational goals and industry standards.
Additionally, we ensure these AI systems integrate seamlessly with existing technological infrastructures. This integration is critical for facilitating a smooth transition to more automated and intelligent operations, minimizing disruption and maximizing the enhancement of business processes.
Our AI-powered LTV model development expertise
We excel in creating AI-powered LTV models that leverage the full potential of advanced machine learning techniques to provide deep insights into customer value. LeewayHertz’s approach to developing AI-driven LTV models involves a comprehensive methodology incorporating cutting-edge machine learning techniques and data analytics to accurately predict the value a customer will bring to a business over their entire lifetime. We integrate various predictive models that utilize historical data, customer demographics, transaction patterns, and engagement metrics to forecast future buying behaviors and preferences. Our strategic approach involves:
- Initial needs assessment and strategic planning: We begin by understanding each client’s specific challenges and objectives, ensuring that our AI solutions are perfectly aligned with their business goals.
- Data integration and management: We implement robust systems to gather, clean, and integrate data from various sources, creating a comprehensive dataset essential for accurate LTV prediction.
- AI model development and training: Utilizing state-of-the-art algorithms, we develop predictive models that can assess and forecast the lifetime value of customers based on their interactions, transaction history, and other relevant data points.
- Implementation and integration: Our AI models are seamlessly integrated into clients’ existing CRM and ERP systems, enhancing their existing capabilities with powerful predictive analytics.
- Continuous optimization and scaling: We ensure that our AI solutions are scalable and adaptable to new data and changing market conditions, providing ongoing value and support.
AI agent/copilot development for enhanced LTV prediction
LeewayHertz’s custom AI agents and copilots are designed to significantly boost the functionalities of LTV prediction systems, providing businesses with the tools they need for enhanced decision-making:
- Advanced analytics:
- Categorize customers by predicted LTV, enabling targeted marketing and personalized service offerings.
- Leverage historical data to identify and predict future buying behaviors, helping to shape more effective customer retention strategies.
- Marketing strategy enhancement:
- Tailor marketing campaigns based on individual LTV projections, maximizing marketing spend efficiency.
- Guide budget distribution across various channels and customer segments to optimize impact on LTV.
- Risk assessment and mitigation:
- Identify customers at high risk of churn, allowing preemptive action to enhance retention.
- Evaluate the profitability of customer segments and adjust strategies accordingly to maximize overall LTV.
By integrating AI-driven LTV models, companies can transform their approach to customer relationship management. These tools provide deep insights that enable personalized customer experiences, optimized marketing strategies, and informed business decisions that drive loyalty and revenue. LeewayHertz’s solutions ensure that businesses not only meet the current needs of their markets but are also poised for future growth and success.
Examples of AI-enhanced customer LTV prediction and utilization
There are many real-world organizations that are using AI or ML to predict LTV. Here are a few examples:
Netflix uses AI to predict the LTV of its subscribers by analyzing a variety of factors, including their viewing history, demographics, and location. This allows Netflix to target its marketing campaigns more effectively and allocate its resources more efficiently. For example, Netflix might target subscribers who have watched a lot of action movies with ads for new action movies. Netflix also uses AI to personalize its user experience by recommending movies and TV shows that it thinks subscribers will enjoy.
Spotify uses AI to predict the LTV of its listeners by analyzing their listening habits, demographics, and location. This allows Spotify to recommend music more effectively and personalize its user experience. For example, Spotify might recommend a new artist to a listener who has been listening to a lot of similar artists. Spotify also uses AI to personalize its advertising by showing ads for products that it thinks listeners will be interested in.
Amazon uses AI to predict the LTV of its customers by analyzing their purchase history, demographics, and browsing behavior. This allows Amazon to target its product recommendations and promotions more effectively. For example, Amazon might recommend a product to a customer who has previously purchased similar products. Amazon also uses AI to personalize its customer support, such as by providing customers with answers to their questions in a more timely and accurate manner.
Shopify uses AI to predict the LTV of its merchants by analyzing their sales data, demographics, and marketing activity. This allows Shopify to provide its merchants with more personalized advice and support. For example, Shopify might recommend a marketing campaign to a merchant that it thinks will be effective in driving sales. Shopify also uses AI to personalize its merchant tools by making it easier for merchants to track their sales and inventory.
Walmart uses AI to predict the LTV of its shoppers by analyzing their purchase history, demographics, and browsing behavior. This allows Walmart to target its promotions and marketing campaigns more effectively. For example, Walmart might run a promotion on a product that it knows shoppers are interested in. Walmart also uses AI to personalize its shopper experience by recommending products that shoppers are likely to be interested in.
Starbucks uses AI to predict the LTV of its customers by analyzing their purchase history, demographics, and location. This allows Starbucks to target its marketing campaigns more effectively and allocate its resources more efficiently. For example, Starbucks might target customers who live in a particular area with ads for a new store opening nearby. Starbucks also uses AI to personalize its customer experience by remembering a customer’s favorite drink order.
Sephora uses AI to predict the LTV of its customers by analyzing their purchase history, demographics, and browsing behavior. This allows Sephora to target its marketing campaigns more effectively and allocate its resources more efficiently. For example, Sephora might target customers who have purchased a lot of makeup with ads for new makeup products. Sephora also uses AI to personalize its customer experience by recommending products that customers are likely to be interested in.
Uber uses AI to predict the LTV of its drivers by analyzing their driving history, demographics, and location. This allows Uber to target its promotions and marketing campaigns more effectively. For example, Uber might target drivers who are located in a particular area with ads for a promotion on rides in that area. Uber also uses AI to personalize its driver experience by providing drivers with information about upcoming events or traffic conditions.
These are just a few examples of how AI is being used to predict LTV. As AI technology advances, we can expect to see even more organizations using AI in LTV predictions and making better decisions about allocating their resources.
The future of LTV prediction: Navigating the evolving landscape
As businesses continue to adopt advanced technologies and data-driven approaches, the future of Customer Lifetime Value (LTV) prediction is poised to undergo significant transformation. The evolving landscape promises more accurate, personalized, and actionable insights that can shape customer-centric strategies. Let’s delve into the key trends and developments that define the future of LTV prediction.
Enhanced personalization through predictive analytics
As AI algorithms become more refined, the ability to predict individual customer behaviors will lead to hyper-personalized experiences. LTV prediction will estimate a customer’s potential value and guide personalized marketing campaigns, product recommendations, and loyalty programs tailored to each customer’s preferences and needs.
Real-time LTV prediction and adaptation
Real-time data processing and analytics will empower businesses to predict LTV on the fly. This capability will allow for dynamic adjustments to marketing efforts, pricing strategies, and customer interactions based on the most up-to-date information. Real-time LTV prediction will be especially valuable in fast-paced industries with rapidly changing customer behaviors.
Integration of unstructured data sources
The future of LTV prediction will involve the integration of diverse data sources, including unstructured data from social media, customer reviews, and other online interactions. Sentiment analysis and natural language processing will provide insights into customer sentiment, preferences, and engagement levels, enriching the accuracy of lifetime value models.
Ethical considerations and privacy compliance
With the increasing use of personal data for LTV prediction, ethical considerations and privacy compliance will play a pivotal role. Striking a balance between utilizing customer data for insights and respecting individual privacy will be crucial. Transparent data usage and compliance with regulations will be paramount.
Collaborative customer engagement
The future of LTV prediction will extend beyond individual customers to collaborative engagement. Businesses will analyze customer interactions and relationships, understanding how network effects influence LTV. This approach will drive strategies that leverage customer communities and referrals.
The future of LTV prediction is characterized by the convergence of data-driven insights and a customer-centric approach. Ethical considerations, privacy compliance, and the integration of emerging technologies will shape how businesses navigate this evolving landscape, ultimately leading to more effective customer engagement, enhanced loyalty, and sustainable growth.
Final Words
In today’s dynamic business landscape, where data is the new goldmine, the accurate estimation of customer lifetime value has emerged as a critical determinant for shaping strategic decisions. The synergy between LTV prediction models and ML technologies has laid the foundation for innovative business choices that drive sustainable growth.
LTV, a key indicator of a customer’s long-term worth, encapsulates the potential revenue generated over the entire customer lifecycle. Traditional approaches often fall short of capturing the intricate nuances that define customer behavior and preferences. This is where LTV prediction models using ML step in, revolutionizing how businesses perceive and leverage customer data.
Through their ability to analyze vast and diverse datasets, ML algorithms unravel hidden patterns influencing purchasing behaviors and retention rates. By factoring in variables like purchase history, engagement levels, demographic details, and more, these models produce remarkably accurate LTV predictions. Armed with these insights, organizations can tailor their marketing strategies, optimize resource allocation, and personalize customer experiences.
Furthermore, predicting LTV using machine learning empowers businesses to proactively identify high-value customers and design retention strategies that enhance customer loyalty. This, in turn, leads to elevated customer satisfaction and improved brand advocacy.
Predicting LTV using machine learning has emerged as the linchpin of strategic business choices. By harnessing the power of ML, companies can accurately gauge customer potential, steer marketing efforts, and allocate resources judiciously. As industries continue to evolve, embracing this cornerstone approach is essential for staying competitive and achieving sustainable growth.
Supercharge your business strategy using ML-driven LTV predictions. Partner with LeewayHertz’s AI experts to harness the full potential of customer lifetime value forecasting.
Start a conversation by filling the form
All information will be kept confidential.
Insights
AI for startups: Its use cases and role in steering success for early-stage businesses
AI empowers startups to overcome resource constraints, outperform competitors, enhance customer experiences, and make data-driven decisions.
AI for financial planning: Use cases, benefits and development
The integration of AI in financial planning is not just about automation but also about the sophisticated interplay of advanced technologies and data.
Generative AI in drug discovery: Unleashing a new era of pharmaceutical innovation
Generative AI significantly impacts each stage of the drug discovery process—from initial research to post-market surveillance, enhancing efficiency and effectiveness