AI in inventory management: Redefining inventory control for the digital age
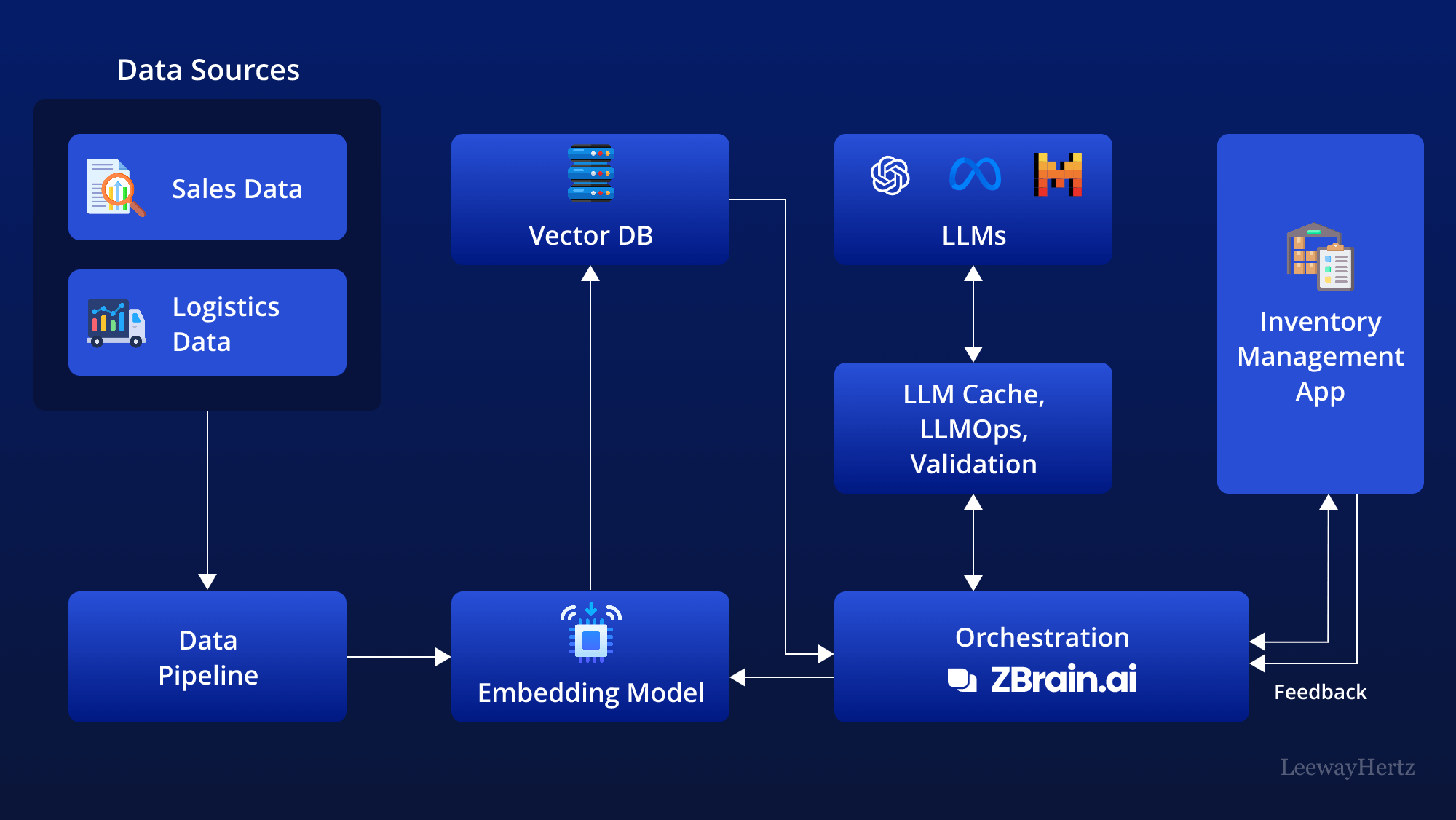
Managing inventory has evolved into a complex puzzle in today’s fast-paced business world. As companies expand their reach and cater to a global audience, tracking inventory and maintaining an accurate “available-to-promise” status in real-time has become a challenge. In the current competitive landscape, retailers prioritize a seamless approach to inventory management, especially with the growing importance of omnichannel fulfillment. The art of balancing supply and demand while keeping costs in check has become a high-stakes game where every piece of the inventory puzzle must fit perfectly.
There is a delicate balance between having enough inventory to keep operations smooth and efficient while avoiding excess stock that ties up precious capital. Handling this job demands anticipation and strategy, often turning urgent calls into calmly managed solutions. Without effective inventory management, inefficiencies can result and cause significant financial losses. Consequently, businesses must master the art of inventory optimization to ensure that resources are allocated wisely, orders are met on time, and customer satisfaction remains high, all while safeguarding the organization’s financial health. AI plays a pivotal role in transforming traditional inventory management practices and maintaining this delicate inventory balance. AI-driven predictive forecasting, for instance, considers historical data, seasonality, and market dynamics to provide exceptionally accurate demand forecasts. This enables businesses not only to maintain sufficient stock levels but also to proactively adapt to changing customer preferences and market conditions.
In this article, we explore the remarkable role of artificial intelligence in inventory management. We will discuss how businesses can harness the power of AI to optimize their inventory processes, what the future of AI-driven inventory management holds and more.
-
- How is AI transforming inventory management?
- How does AI overcome the challenges faced by traditional inventory management systems?
- How does AI in inventory management work
- Use cases of AI in inventory management
- Demand forecasting
- Inventory optimization
- Reordering
- Inventory tracking
- Returns management
- Inventory reconciliation
- Inventory analytics
- Material shortage management
- Warehouse automation
- Dynamic pricing
- Batch tracking
- Inventory classification
- Seasonal trend analysis
- Expiry date management
- Inventory risk assessment
- Localization strategies
- Inventory auditing
- Streamlining inventory management workflow with generative AI
- How does LeewayHertz’s generative AI platform streamline inventory management workflows?
- Advanced AI technologies and techniques used in inventory management
- Benefits of integrating AI in inventory management
- How to implement AI in an inventory management system?
- Use cases of AI in inventory management
How is AI transforming inventory management?
Artificial Intelligence (AI) transforms inventory management by leveraging advanced algorithms and ML techniques to analyze vast datasets quickly and accurately. AI-powered systems can process data at an unparalleled scale and speed, unlike traditional methods that depend on manual input and analysis.
AI’s prowess in inventory management lies in its capacity to detect patterns and trends within data. By scrutinizing historical sales data, customer behavior, market trends, and other pertinent factors, AI reveals insights that manual analysis may overlook. This enables businesses to understand their inventory needs better, optimize stock levels, and anticipate changes in demand.
Additionally, AI demonstrates proficiency in predictive analytics by forecasting future demand through the analysis of historical data and current market conditions. By accurately predicting future trends, businesses can make informed decisions about inventory replenishment, pricing strategies, and product promotions. This proactive approach helps minimize stockouts, reduce inventory, and improve operational efficiency.
Furthermore, AI-powered inventory management systems can continuously learn and adapt over time. Through feedback loops and ongoing data analysis, these systems can refine their algorithms and models to reflect better-changing market dynamics and business conditions. This adaptive capability enables businesses to stay agile and responsive in an increasingly competitive marketplace.
How does AI overcome the challenges faced by traditional inventory management systems?
Artificial Intelligence (AI) plays a crucial role in overcoming the challenges and complexities faced by traditional inventory management systems. Here’s how AI aids in addressing each of these challenges:
Inaccurate demand forecasting: Traditional inventory management systems often rely on historical data and simplistic methods for demand forecasting, leading to inaccuracies in predicting future demand patterns. This can result in overstocking or understocking issues.
AI utilizes sophisticated algorithms and machine learning techniques to analyze extensive datasets comprising historical sales data, market trends, and external influences. By identifying complex patterns and correlations, AI can improve demand forecasting accuracy, reducing the risk of overstocking or understocking.
Limited visibility: Lack of real-time data and visibility across the supply chain can lead to poor decision-making. Traditional systems may need to provide insights into inventory levels at various locations or stages, making it difficult to respond quickly to changes.
AI-powered inventory management systems provide real-time visibility across the entire supply chain. By integrating data from multiple sources, AI enables businesses to monitor inventory levels, track shipments, and promptly respond to fluctuations in demand or supply.
Manual data entry: Conventional systems often rely on manual data entry, a time-consuming process susceptible to errors and inefficiencies. This can lead to discrepancies in inventory records and hinder overall accuracy.
AI automates data entry tasks, minimizing errors and inefficiencies associated with manual processes. Through optical character recognition (OCR) and NLP, AI can extract and interpret data from various sources, streamlining inventory management workflows.
High carrying costs: Maintaining excess inventory ties up valuable capital and incurs storage costs. Traditional systems may struggle to optimize inventory levels, potentially leading to higher carrying costs due to inefficiencies.
AI optimizes inventory levels based on demand forecasts, lead times, and other factors, reducing excess inventory and lowering carrying costs. By dynamically adjusting reorder points and safety stock levels, AI helps businesses optimize working capital and improve profitability.
Supply chain disruptions: Traditional systems may struggle to handle unexpected supply chain disruptions, such as production or distribution delays, which can ripple through the entire inventory management process.
AI-powered predictive analytics can anticipate potential supply chain disruptions and proactively mitigate risks. By examining historical data and external factors, AI identifies vulnerabilities in the supply chain and recommends contingency plans to minimize disruptions.
Lack of flexibility: Traditional systems may adapt poorly to changes in market trends, seasonality, or shifts in customer preferences. This lack of flexibility can result in obsolete or slow-moving inventory.
AI continuously learns from data and adapts to changing market conditions, enabling businesses to respond quickly to shifts in demand, seasonality, or customer preferences. AI enhances agility and flexibility in inventory management by providing real-time insights and recommendations.
Poor supplier collaboration: Limited communication and collaboration with suppliers can lead to challenges in managing lead times, order quantities, and replenishment schedules.
AI facilitates better supplier communication and collaboration through data sharing and predictive analytics. AI enhances supplier relationships and improves overall supply chain efficiency by optimizing lead times, order quantities, and inventory replenishment schedules.
Inefficient order fulfillment: With real-time visibility into inventory levels, traditional systems may lead to efficient order fulfillment processes, delayed shipments, and dissatisfied customers.
AI optimizes order fulfillment processes by dynamically allocating inventory, optimizing routes, and prioritizing orders based on urgency and availability. AI streamlines order fulfillment workflows by providing real-time insights into inventory levels and order status and enhancing customer satisfaction.
Complexity of multi-location operations: For businesses with multiple warehouses or distribution centers, coordinating inventory across various locations can become complex and challenging with traditional systems.
AI centralizes inventory management across multiple locations, providing a unified view of inventory levels and movements. AI simplifies multi-location operations and improves overall supply chain efficiency by optimizing inventory allocation and replenishment strategies.
Lack of data-driven insights: Traditional systems may need more analytical capabilities to provide meaningful insights into trends, patterns, and opportunities for improvement in inventory management.
AI generates actionable insights from data, identifying trends, patterns, and opportunities for improvement in inventory management. By leveraging advanced analytics techniques like machine learning and predictive modeling, AI empowers businesses to make data-driven decisions and drive continuous improvement.
Manual reordering: Reordering inventory manually can result in delays and errors. Traditional systems may not have automated reorder triggers based on actual demand.
AI automates reordering by setting intelligent reorder triggers based on demand, lead times, and inventory policies. By eliminating manual intervention, AI ensures timely replenishment and reduces the risk of stockouts or excess inventory.
Difficulty in demand planning: Traditional systems may need help incorporating factors beyond historical sales data, such as market trends, external influences, and changing customer behavior.
AI incorporates many factors into demand planning, including historical data, market trends, and external influences. By leveraging advanced forecasting models and predictive analytics, AI provides more accurate and granular demand forecasts, enabling businesses to anticipate future demand better and optimize inventory levels accordingly.
AI enhances traditional inventory management systems by providing advanced analytics, automation, and real-time visibility. It enables businesses to overcome challenges, improve efficiency, and stay competitive in the dynamic marketplace.
Optimize Your Operations With AI Agents
Our AI agents streamline your workflows, unlocking new levels of business efficiency!
How does AI in inventory management work?
Incorporating AI into inventory management involves various components to streamline data analysis, generate insights, and support decision-making. It goes beyond traditional inventory management practices by integrating powerful Large Language Models (LLMs) and connecting them with an organization’s unique data sources. This approach unlocks a new level of insight generation and empowers businesses to make data-driven decisions in real time.
This architecture leverages various components to streamline the inventory management process. Here’s a step-by-step breakdown of how it works:
- Data sources: Multiple data sources contribute to an inventory management system employing this framework, augmenting decision-making and operational efficiency. This data can include:
- Inventory levels: Real-time stock quantities across various locations.
- Sales data: Transaction records indicating demand patterns.
- Supplier information: Details about suppliers, their performance, and delivery schedules.
- Order data: Records of incoming and outgoing orders.
- Logistics data: Information on shipping, transportation, and delivery times.
- Warehouse data: Data on warehouse conditions, storage capacities, and inventory organization.
- Market trends: External data on market trends and consumer behavior.
- Production data: Information on manufacturing schedules and production capacities.
- Data pipelines: Data from the sources listed above are then routed through data pipelines. These pipelines are responsible for the ingestion, cleaning, and structuring of data, making it ready for further analysis.
- Embedding model: Advanced embedding models like those from OpenAI, Cohere, or Google transform raw data into meaningful representations that the AI system can understand and analyze. These models transform the textual data into numerical representations called vectors that AI models can understand.
- Vector database: Processed data is stored in a vector database (e.g., Pinecone, Weaviate, Chroma), facilitating efficient retrieval and querying.
- APIs and plugins: APIs and plugins like Serp, Zapier, and Wolfram play a key role by connecting different components and enabling additional functionalities, such as accessing extra data or performing specific tasks with ease.
- Orchestration layer: The orchestrating layer is critical in managing the workflow. ZBrain is an example of this layer that simplifies prompt chaining, manages interactions with external APIs by determining when API calls are required, retrieves contextual data from vector databases, and maintains memory across multiple LLM calls. Ultimately, this layer generates a prompt or series of prompts that are submitted to a language model for processing. The role of this layer is to orchestrate the flow of data and tasks, ensuring seamless coordination across all inventory management components.
- Query execution: The data retrieval and generation process begins when the user submits a query to the inventory management app. The app handles user queries regarding inventory status, stock levels, order fulfillment, and demand forecasting.
- LLM processing: Once received, the app transmits the query to the orchestration layer. This layer retrieves relevant data from the vector database and LLM cache and sends it to the appropriate LLM for processing. The choice of LLM depends on the nature of the query.
- Output: The LLM generates an output based on the query and the data it receives. This response can include actionable insights and recommendations, such as optimal stock levels, reorder points, and demand forecasts.
- Inventory management app: The validated output is then presented to the user through the inventory management app. This is the core application where all the data, analysis, and insights converge. It presents the findings in a user-friendly format for decision-makers.
- Feedback loop: User feedback on the LLM’s output is another important aspect of this architecture. A continuous feedback loop allows the system to learn and improve over time, refining its predictions and recommendations based on new data and user input.
- Agent: AI agents step into this process to address complex problems, interact with the external environment, and enhance learning through post-deployment experiences. They achieve this by employing advanced reasoning/planning, strategic tool utilization, and leveraging memory, recursion, and self-reflection.
- LLM cache: Tools like Redis, SQLite, or GPTCache are used to cache frequently accessed information, speeding up the response time of the AI system.
- Logging/LLMOps: Throughout this process, LLM operations (LLMOps) tools like Weights & Biases, MLflow, Helicone and Prompt Layer help log actions and monitor performance. This ensures the LLMs are functioning optimally and continuously improve through feedback loops.
- Validation: A validation layer is employed to validate the LLM’s output. This is done through tools like Guardrails, Rebuff, Guidance, and LMQL to ensure the accuracy and reliability of the information provided.
- LLM APIs and hosting: LLM APIs and hosting platforms are essential for executing inventory management tasks and hosting the application. Depending on the requirements, developers can select from LLM APIs such as OpenAI and Anthropic or opt for open-source models. Similarly, they can choose hosting platforms from cloud providers like AWS, GCP, Azure, and Coreweave or opt for opinionated clouds like Databricks, Mosaic, and Anyscale. The choice of LLM APIs and cloud hosting depends on the project’s needs and preferences.
This structured flow provides a detailed overview of how AI facilitates inventory management, leveraging various data sources and technological tools to generate accurate and actionable insights. Overall, this approach enables enterprises to manage their inventory more effectively, reducing costs, optimizing stock levels, and ensuring timely order fulfillment.
Use cases of AI in inventory management
AI offers various use cases in inventory management, equipping businesses with powerful tools to optimize their supply chain and enhance overall efficiency. Some of the key AI use cases in inventory management include:
Demand forecasting
AI in inventory management leverages sophisticated algorithms to meticulously analyze various data points, including historical sales data, market trends, and external influences like seasonality and promotional activities. AI identifies intricate patterns and correlations through this analysis, enabling businesses to generate precise demand forecasts. By accurately anticipating future inventory requirements, companies can optimize stock levels, minimize stockouts, and enhance overall operational efficiency. This proactive approach to inventory management empowers businesses to meet customer demand effectively while reducing excess inventory costs.
Inventory optimization
AI considers various factors, such as lead times, demand variability, and storage costs, to determine optimal inventory levels. By employing sophisticated optimization methods, AI ensures that companies maintain adequate stock to fulfill demand while minimizing surplus inventory and related holding expenses. This enables businesses to operate more efficiently, managing inventory without unnecessary costs or shortages.
Reordering
AI automates the process of generating purchase orders or replenishment requests. AI dynamically adjusts reorder points and order quantities by utilizing demand forecasts and predefined inventory policies. This streamlines the ordering process, ensuring timely inventory replenishment to meet customer demand efficiently. Through AI’s adaptive capabilities, businesses can optimize inventory levels and minimize stockouts, enhancing overall operational efficiency.
Inventory tracking
AI monitors inventory movements throughout the delivery process, utilizing real-time tracking capabilities, offering visibility into shipment status and location. By continuously monitoring inventory levels across different supply chain stages, AI facilitates accurate delivery and mitigates the likelihood of stockouts or overstocking. This proactive approach enhances operational efficiency and customer satisfaction by ensuring inventory levels align with demand throughout the supply chain journey.
Returns management
AI examines return patterns and reasons to streamline returns processing, pinpointing trends in product defects, customer preferences, and supply chain inefficiencies. By automating returns processing and restocking decisions, AI mitigates the impact of returns on inventory levels and optimizes recovery value. This proactive approach enables businesses to efficiently manage returns, enhance customer satisfaction, and improve inventory management practices.
Inventory reconciliation
Employing automated reconciliation, AI seamlessly integrates returned items with inventory records, promptly updating stock levels and initiating restocking or disposal procedures as necessary. By maintaining precise inventory records, AI provides businesses with a comprehensive overview of their inventory status, enabling informed decision-making regarding future inventory management strategies. This capability enhances operational efficiency, minimizes discrepancies, and optimizes inventory control processes for sustained business success.
Inventory analytics
AI scrutinizes inventory data to discern trends, patterns, and optimization opportunities, informing future inventory management strategies and decision-making. By offering actionable insights and recommendations, AI empowers businesses to refine their inventory management processes and adapt to dynamic market conditions. This data-driven approach fosters continuous improvement, enabling businesses to stay agile and competitive in today’s ever-evolving marketplace.
Material shortage management
AI plays a vital role in material shortage management by leveraging predictive analytics to forecast shortages before they occur. AI empowers businesses to proactively address inventory challenges by analyzing historical data and market trends. This includes sourcing alternative suppliers or adjusting production schedules to mitigate potential impacts on inventory levels. Furthermore, AI enhances the reordering process by dynamically adjusting reorder points and quantities using real-time demand forecasts and supplier lead times. This ensures optimal inventory levels while reducing the likelihood of stockouts.
Warehouse automation
AI can automate inventory tracking, picking, packing, and layout optimization tasks. AI-powered sensors and RFID technology enable real-time tracking of inventory movements, improving accuracy and visibility while reducing manual intervention. Additionally, AI optimizes warehouse layout and storage configurations based on historical data, maximizing space utilization and minimizing operational costs. Lastly, AI monitors warehouse equipment in real-time, predicting maintenance needs and scheduling proactive repairs to minimize downtime and ensure the continuous operation of critical warehouse systems.
Dynamic pricing
Utilizing AI-driven dynamic pricing, businesses can adapt prices in real time based on demand fluctuations, competitor pricing strategies, and inventory levels. This proactive approach allows companies to maximize revenue by optimizing prices to reflect market conditions accurately. Additionally, dynamic pricing helps minimize stock clearance by strategically adjusting prices to stimulate demand for slower-moving inventory, reducing the risk of unsold goods and increasing overall profitability.
Batch tracking
Utilizing AI-powered tracking systems, businesses can meticulously track and trace inventory batches across the entire supply chain. This comprehensive visibility enables enhanced inventory management, ensuring optimal stock levels and efficient resource allocation. Additionally, AI facilitates compliance with regulatory requirements by accurately documenting inventory movement and storage conditions. AI facilitates rapid and targeted responses during recalls or quality issues, minimizing the impact on customers and mitigating potential risks to the business effectively.
Inventory classification
AI can automatically categorize inventory items based on various attributes such as size, weight, and demand patterns, facilitating better organization and management. This automation streamlines organization and management processes, ensuring efficient handling of diverse inventory. By accurately categorizing items, businesses can optimize storage, streamline retrieval processes, and make informed inventory replenishment and allocation decisions.
Seasonal trend analysis
Utilizing AI, businesses can analyze seasonal trends and patterns in inventory sales to optimize stock levels, promotions, and marketing strategies. By identifying fluctuations in demand across different seasons, AI enables businesses to anticipate and respond to changing consumer preferences more effectively. This proactive approach helps businesses avoid stockouts during peak seasons while minimizing overstocking during off season. Furthermore, AI-driven insights inform targeted promotions and marketing campaigns tailored to specific seasonal trends, maximizing revenue potential and enhancing overall profitability.
Expiry date management
Through AI, businesses can monitor inventory items with expiration dates, automatically prioritizing the sale or disposal of products nearing expiry to minimize waste and losses. By analyzing expiry dates and historical sales data, AI predicts demand for perishable goods and recommends optimal pricing or promotion strategies to accelerate sales before expiration. Additionally, AI alerts inventory managers to impending expirations, enabling timely action such as markdowns, donations, or returns to suppliers, thus reducing inventory holding costs and mitigating the risk of spoilage. This proactive approach minimizes waste and maximizes revenue potential, ensuring efficient inventory management and profitability.
Inventory risk assessment
AI is crucial in assessing various risks, including market volatility, supplier reliability, and geopolitical factors, to proactively manage inventory-related risks and uncertainties. AI enables businesses to make informed decisions and proactively implement mitigation strategies by analyzing extensive data and identifying potential risk factors. This proactive approach enables companies to address risks before they escalate, ensuring smoother operations and minimizing potential disruptions. This proactive risk management approach helps minimize the impact of unforeseen events on inventory levels, ensuring continuity in supply chain operations and safeguarding business interests. Additionally, AI-driven risk assessment enables businesses to maintain resilience in dynamic market environments and optimize inventory management practices for long-term success.
Localization strategies
Utilizing AI, businesses can analyze regional preferences and demand patterns to customize inventory assortments, pricing strategies, and marketing campaigns for specific geographic markets. By understanding customers’ unique preferences and behaviors in different regions, AI enables businesses to tailor their offerings to meet local demand effectively. This targeted approach enhances customer satisfaction, improves sales performance, and strengthens brand loyalty in diverse markets. Additionally, AI-driven insights inform strategic decisions regarding inventory allocation, pricing adjustments, and promotional activities, optimizing overall business performance and competitiveness in regional markets.
Inventory auditing
AI-powered drones autonomously conduct inventory audits in warehouses or retail stores, minimizing manual labor and enhancing accuracy. Using advanced computer vision and machine learning algorithms, these drones navigate the inventory space efficiently, capturing and analyzing real-time data. This automation streamlines the auditing process, reduces human error, and provides timely insights into inventory levels and discrepancies. Ultimately, AI-driven inventory audits improve operational efficiency, optimize inventory management, and support better business decision-making.
Streamlining inventory management workflow with generative AI
Generative AI is transforming inventory management by automating tasks, optimizing workflows, and improving decision-making. By leveraging advanced GenAI capabilities, key personnel involved in inventory management can enhance their efficiency and effectiveness across various stages of the inventory lifecycle.
Key personas involved in the inventory management processes
- Inventory manager: Utilizes GenAI to analyze stock data, forecast demand, and optimize inventory levels, ensuring efficient stock management and accurate order placement.
- Warehouse supervisor: Employs GenAI to streamline the receipt, storage, and dispatch of goods, leveraging real-time data for efficient warehouse operations and inventory accuracy.
- Procurement officer: Leverages GenAI to evaluate supplier information, automate order creation, and negotiate contracts, optimizing the procurement process and ensuring cost-effective sourcing.
- Shipping coordinator: Uses GenAI to manage transportation logistics, track shipments, and ensure timely delivery, enhancing efficiency in the shipping and logistics processes.
- Quality control inspector: Uses GenAI to monitor and assess inventory quality, using automated tools to detect defects and ensure compliance with quality standards.
Here’s a breakdown of the key inventory management processes and how generative AI is significantly impacting each step:
Inventory planning and forecasting
Steps Involved | Sub-Steps Involved | Role of GenAI |
---|---|---|
Inventory Forecasting |
|
|
Inventory Planning |
|
|
Inventory Request and Requisition |
|
|
Supplier Selection and Ordering |
|
|
Supplier selection and ordering
Steps Involved | Sub-Steps Involved | Role of GenAI |
---|---|---|
Gather Supplier Information |
|
|
Validate Supplier Information |
|
|
Generate Contract Documents |
|
|
Monitor Supplier Performance |
|
|
Inventory procurement and receiving
Steps Involved | Sub-Steps Involved | Role of GenAI |
---|---|---|
Inventory Restocking |
|
|
Inventory Replenishment |
|
|
Inventory Receiving and Inspection |
|
|
Inventory handling
Steps Involved | Sub-Steps Involved | Role of GenAI |
---|---|---|
Inventory Serialization |
|
|
Inventory Transfer |
|
|
Inventory auditing and disposal
Steps Involved | Sub-Steps Involved | Role of GenAI |
---|---|---|
Inventory Auditing |
|
|
Inventory Compliance Verification |
|
|
Inventory Disposal |
|
|
By integrating GenAI into the inventory management workflow, businesses can achieve enhanced accuracy, efficiency, and real-time insights across all processes. However, while GenAI offers powerful tools and automation, human judgment remains essential for overseeing complex scenarios, making nuanced decisions, and ensuring compliance with ever-evolving regulations.
How does LeewayHertz’s generative AI platform streamline inventory management workflows?
LeewayHertz’s generative AI platform, ZBrain, is a transformative solution for businesses seeking to optimize inventory management, forecast demand accurately, and achieve operational excellence. By harnessing clients’ proprietary data in various formats, including texts, images, and documents, ZBrain utilizes cutting-edge large language models (LLMs) like GPT-4, Vicuna, Llama 2, and GPT-NeoX to build context-aware applications that can improve decision-making, deepen insights, and boost productivity, all while prioritizing data privacy. By creating highly tailored LLM-based applications, ZBrain redefines inventory workflows, offering a unique opportunity to enhance efficiency, minimize costs, and elevate customer satisfaction.
In the realm of inventory management, challenges such as inaccurate demand forecasting, suboptimal stock levels, and complex supply chain dynamics are prevalent. ZBrain addresses these issues with specialized flows that act as detailed, step-by-step guides, illustrating how ZBrain systematically addresses industry-specific use cases. This also empowers businesses to establish seamless, code-free logic for efficient inventory workflows.
By leveraging AI-driven automation and advanced data analysis, ZBrain’s flows optimize inventory management. This involves translating complex data into actionable insights, ensuring improved operational efficiency, reduced error rates and enhanced overall inventory quality. Through these advancements, ZBrain provides comprehensive solutions to address key challenges in the inventory management domain. Here are a few examples of how ZBrain transforms inventory management:
AI-driven inventory management in logistics
LeewayHertz utilizes ZBrain’s flow to streamline demand forecasting and warehouse planning, automating and accelerating processes traditionally burdened by fluctuating demand patterns, seasonality, market trends, and supply chain dynamics. By significantly reducing the time and effort invested in demand forecasting and warehouse planning, ZBrain empowers logistics managers to proactively optimize operations, leading to superior resource allocation, reduced costs, and elevated service levels. You can explore this detailed flow to understand how ZBrain redefines inventory management, providing a more responsive and efficient supply chain that adapts swiftly to market changes and customer needs.
AI-driven inventory management in retail
LeewayHertz harnesses ZBrain to enhance retail efficiency through advanced inventory optimization and demand forecasting strategies. In the complex landscape of maintaining optimal inventory levels and predicting product demand, the LLM-based apps created using ZBrain flow simplify and accelerate processes, mitigating challenges associated with analyzing vast volumes of data. This significantly reduces the time and effort traditionally required for inventory optimization and demand forecasting, enabling retail managers to forecast product demand and manage inventory more efficiently and accurately. To understand how ZBrain redefines inventory optimization and demand forecasting for retail success, delve into this detailed flow here.
Advanced AI technologies and techniques used in inventory management
Advanced AI techniques are the reason behind improved inventory management, offering innovative solutions to optimize supply chain processes, enhance forecasting accuracy, and streamline operations. Here are some of the advanced AI techniques being applied in inventory management:
- Machine learning for demand forecasting: Machine learning algorithms are used to analyze historical sales data, market trends, weather patterns, and other relevant factors to predict future demand more accurately. These algorithms continuously learn from new data, refining their forecasts and adapting to changing market conditions.
- Reinforcement learning for inventory optimization: Reinforcement learning, a subfield of machine learning, enables AI systems to make decisions and take actions to maximize a specific objective, such as minimizing inventory costs or reducing stockouts. The AI agent gains knowledge by trial and error, optimizing inventory levels and reordering points in response to different scenarios and feedback.
- Natural Language Processing (NLP) for data analysis: With the help of NLP, AI systems can effectively extract valuable insights from unstructured data, encompassing social media posts, customer reviews, and industry news. By analyzing textual data, businesses can better understand consumer preferences and market sentiments, informing their inventory strategies accordingly.
- Computer vision for automated inventory checks: Computer vision technology allows AI systems to process visual information from cameras and sensors to monitor inventory levels, identify damaged goods, and track inventory movements automatically. This eliminates the need for manual stock counting and reduces human errors.
- Predictive analytics for maintenance optimization: Combined with sensor data from machinery and equipment, predictive analytics helps predict maintenance needs. AI systems have the capability to identify potential issues before they escalate, enabling proactive maintenance scheduling and minimizing equipment downtime.
- Generative Adversarial Networks (GANs) for inventory simulation: GANs are used to create synthetic data that mimics real-world inventory scenarios. These simulated environments allow businesses to test different inventory strategies and their potential outcomes without risking actual resources.
- Autonomous mobile robots for warehouse management: AI-powered autonomous mobile robots navigate through warehouses, picking and delivering items efficiently. They can optimize the picking route, reduce human involvement, and increase warehouse throughput.
- Sentiment analysis for demand sensing: AI-powered sentiment analysis interprets customer feedback and social media sentiments to gauge changing demand patterns. This data helps businesses adapt their inventory levels and product offerings to match consumer preferences effectively.
- Multi-echelon Inventory Optimization (MEIO): MEIO uses AI algorithms to optimize inventory levels across multiple locations and tiers in the supply chain. By coordinating inventory decisions throughout the network, MEIO prevents excess stock and reduces supply chain costs.
- Predictive stock replenishment: AI-driven systems can automatically trigger stock replenishment based on real-time sales data, inventory levels, and demand forecasts. This ensures that businesses maintain optimal stock levels, avoiding stockouts or overstock situations.
By harnessing the power of these advanced AI techniques, businesses can achieve higher efficiency, reduced costs, and improved customer satisfaction in their inventory management processes. The continuous advancements in AI technology are likely to unlock even more possibilities and drive further innovation in this field.
Optimize Your Operations With AI Agents
Our AI agents streamline your workflows, unlocking new levels of business efficiency!
Benefits of integrating AI in inventory management
- Enhanced efficiency: AI streamlines inventory management processes by automating repetitive tasks such as data entry, analysis, and decision-making. By eliminating manual effort, businesses can allocate resources more efficiently, freeing time for employees to focus on higher-value activities like strategic planning and customer service.
- Improved accuracy: AI-powered algorithms leverage advanced data analytics and machine learning techniques to process vast amounts of inventory data accurately and quickly. This reduces the risk of human error associated with manual data entry and analysis, leading to more precise inventory forecasts, order recommendations, and inventory tracking.
- Real-time Insights: AI gives businesses real-time visibility into inventory levels, movements, and trends across multiple channels and locations. This enables companies to make informed decisions on inventory replenishment, allocation, and pricing, ensuring they can effectively meet customer demand and avoid stockouts or overstock situations.
- Cost reduction: By optimizing inventory levels and minimizing carrying costs, AI helps businesses reduce operational expenses associated with inventory management. AI-driven demand forecasting and inventory optimization algorithms enable companies to maintain optimal stock levels, reducing the need for excess inventory storage and associated costs.
- Better customer service: AI enables businesses to anticipate and respond to customer demand more effectively by providing accurate inventory forecasts and personalized product recommendations. By ensuring product availability and delivering faster order fulfillment, companies can enhance the customer experience, leading to higher satisfaction and loyalty.
- Scalability: AI-driven inventory management solutions can scale with business growth and adapt to changing needs and complexities without requiring significant manual intervention. This flexibility allows companies to expand their operations, enter new markets, or introduce new product lines confidently, knowing that their inventory management system can support their evolving business requirements.
- Enhanced inventory accuracy: AI-driven algorithms significantly improve inventory accuracy, reducing the need for manual checks and audits. This leads to more precise resource allocation decisions, as businesses can rely on accurate inventory data to determine optimal stock levels, allocate resources efficiently, and minimize the risk of stockouts or overstock situations.
- Increased productivity: AI-powered inventory management systems boost productivity by automating time-consuming tasks and providing actionable insights. By handling routine operations such as demand forecasting, replenishment recommendations, and inventory tracking, AI allows employees to focus on higher-value activities, contributing to business growth and innovation.
- Resource allocation: AI-generated insights enable better decision-making regarding resource allocation in inventory management. By analyzing trends, patterns, and market dynamics, businesses can allocate resources more strategically, such as adjusting inventory levels, optimizing supply chain processes, and implementing targeted marketing strategies, leading to improved business outcomes and profitability.
How to implement AI in an inventory management system?
Implementing artificial intelligence (AI) in inventory management can significantly improve efficiency, accuracy, and decision-making processes. Here’s a step-by-step guide on how to implement AI in inventory management:
- Identify pain points: Understand your inventory management processes’ current challenges and pain points. This could include stockouts, overstocking, inaccurate forecasts, and inefficient replenishment processes.
- Data collection and integration: Gather relevant data from various sources such as sales records, purchase orders, historical demand data, supplier information, and external factors like market trends and seasonality. Integrate this data into a centralized system for analysis.
- Data cleaning and preprocessing: Clean and preprocess the data to ensure consistency and accuracy. This involves removing duplicates, handling missing values, and standardizing formats.
- Choose AI techniques: Select the appropriate AI techniques based on your specific requirements. Common AI techniques used in inventory management include machine learning algorithms (e.g., regression, classification, clustering), predictive analytics, and natural language processing (NLP) for analyzing unstructured data like customer feedback and supplier emails.
- Model development: Develop AI models tailored to your inventory management needs. For example:
- Demand Forecasting: Use historical sales data and external factors to forecast future demand accurately.
- Replenishment optimization: Optimize reorder points, safety stock levels, and order quantities based on demand forecasts, lead times, and service level targets.
- Supplier performance analysis: Analyze supplier performance metrics to identify reliable suppliers and optimize procurement strategies.
- Stockout prediction: Predict potential stockouts to take proactive measures such as expedited orders or alternative sourcing.
- Training and validation: Train the AI models using historical data and validate their performance using holdout datasets or cross-validation techniques. Continuously refine and update the models as new data becomes available.
- Integration with inventory systems: Integrate the AI models with your existing inventory management systems or implement specialized inventory management software that incorporates AI capabilities.
- Monitor and fine-tune: Monitor the performance of the AI models in real-time and fine-tune them as needed to adapt to changing business conditions, market dynamics, and seasonal variations.
- User training and adoption: Train users to interpret and utilize the insights generated by AI models effectively. Demonstrate the benefits of AI-driven inventory management, such as improved accuracy, reduced costs, and better decision-making, to encourage adoption.
- Continuous improvement: Continuously evaluate the performance of AI-driven inventory management systems and identify areas for improvement. Stay informed with the latest advancements in AI technology and incorporate new techniques or algorithms to enhance efficiency and effectiveness.
By following these steps, you can successfully implement AI in inventory management to optimize your inventory processes and drive business growth.
Endnote
AI has significantly impacted inventory management, empowering businesses with remarkable efficiency, accuracy, and foresight. It addresses long-standing challenges and unlocks unprecedented opportunities for optimization. With the use of AI in inventory management systems, businesses can experience enhanced order tracking and gain real-time visibility into multiple orders simultaneously. This ability to detect potential bottlenecks and inventory issues enables businesses to take proactive measures, ensuring seamless operations and elevating customer satisfaction to new heights.
Moreover, AI-based inventory forecasting redefines supply chain planning by leveraging historical sales data, consumer trends, and relevant factors. With higher precision in predicting demand patterns, businesses can optimize inventory levels, minimize stockouts, and reduce excess stock, ultimately leading to substantial cost savings and increased operational efficiency.
Automation plays a pivotal role in AI-powered inventory management, introducing automated surveillance systems that monitor inventory levels and detect discrepancies. By relieving the burden of manual labor, businesses can redirect their resources towards more strategic endeavors, fostering innovation and growth. Customization is another hallmark of AI in inventory management, as AI systems’ adaptability and learning capabilities enable the development of personalized solutions for businesses. Tailored insights and recommendations ensure that each organization’s specific needs are met, further enhancing operational efficiency and decision-making. Predictive maintenance, driven by AI, ensures the seamless functioning of warehouse equipment, minimizing downtime and preventing unexpected breakdowns. As a result, warehouse operations run smoothly, ensuring that inventory management remains uninterrupted and efficient.
The future of AI in inventory management holds immense promise, with continuous technological advancements paving the way for even more innovative solutions. Businesses that embrace AI-powered inventory management are poised to gain a significant competitive advantage, maximizing efficiency, minimizing costs, and delivering unparalleled customer experiences.
As we look ahead, it is evident that AI’s influence will only grow stronger, redefining the boundaries of inventory management and reshaping the way businesses operate within their supply chains. Embracing AI has evolved from being an option to an absolute necessity for organizations seeking sustainable growth and success in inventory management’s dynamic and rapidly evolving world. The AI-powered future has arrived, and businesses that seize the opportunity will lead the way toward a new era of efficiency and prosperity in inventory management.
Wondering how to optimize stock levels, reduce carrying costs, and boost supply chain efficiency? Look no further! AI experts at Leewayhertz will craft custom AI-powered inventory management solutions for your business needs and set you up for success.
Start a conversation by filling the form
All information will be kept confidential.
FAQs
What are the benefits of using AI in inventory management?
The benefits of AI in inventory management include improved demand forecasting accuracy, reduced carrying costs, optimized inventory turnover, enhanced supply chain visibility, minimized stockouts, increased customer satisfaction, and better resource allocation.
What AI technologies are used in inventory management?
AI technologies used in inventory management include machine learning algorithms for demand forecasting and predictive analytics, natural language processing (NLP) for sentiment analysis and customer feedback processing, computer vision for product recognition and inventory tracking, and robotic process automation (RPA) for warehouse automation and order fulfillment.
How does AI-driven demand forecasting work in inventory management?
AI-driven demand forecasting uses historical sales data, market trends, seasonal patterns, and external factors to predict future demand for products. Machine learning algorithms analyze these data points to generate accurate demand forecasts, helping businesses optimize inventory levels and prevent stockouts.
How can LeewayHertz assist in implementing AI for inventory management?
LeewayHertz specializes in developing custom AI solutions for inventory management tailored to each client’s specific requirements. Our team leverages advanced machine learning algorithms and data analytics techniques to optimize inventory processes, improve demand forecasting accuracy, and enhance supply chain efficiency.
How does LeewayHertz ensure seamless integration of AI solutions with existing inventory management systems?
LeewayHertz follows a systematic approach to integrating AI solutions with clients’ existing inventory management systems. Our team performs a thorough evaluation of your current infrastructure, identifies integration points, and develops custom APIs or connectors to facilitate smooth data flow between systems.
Can LeewayHertz provide ongoing support and maintenance for AI-powered inventory management solutions?
Yes, LeewayHertz offers comprehensive support and maintenance services for AI-powered inventory management solutions post-deployment. Our dedicated team of experts monitors system performance, troubleshoots issues, and implements updates or enhancements to ensure optimal functionality and performance over time.
How do I initiate a project with LeewayHertz for AI-powered inventory management solutions?
Initiating a project with LeewayHertz for AI-powered inventory management is a straightforward process. Reach out to us via our website or email us at sales@leewayhertz.com. We will schedule a consultation to understand your requirements, propose tailored AI solutions, and align strategies with your goals. Upon approval of the project scope, our expert team begins development, keeping you informed and ensuring timely delivery. We provide ongoing support and maintenance post-deployment to optimize system performance.