AI in incident response: Exploring use cases, solutions and benefits
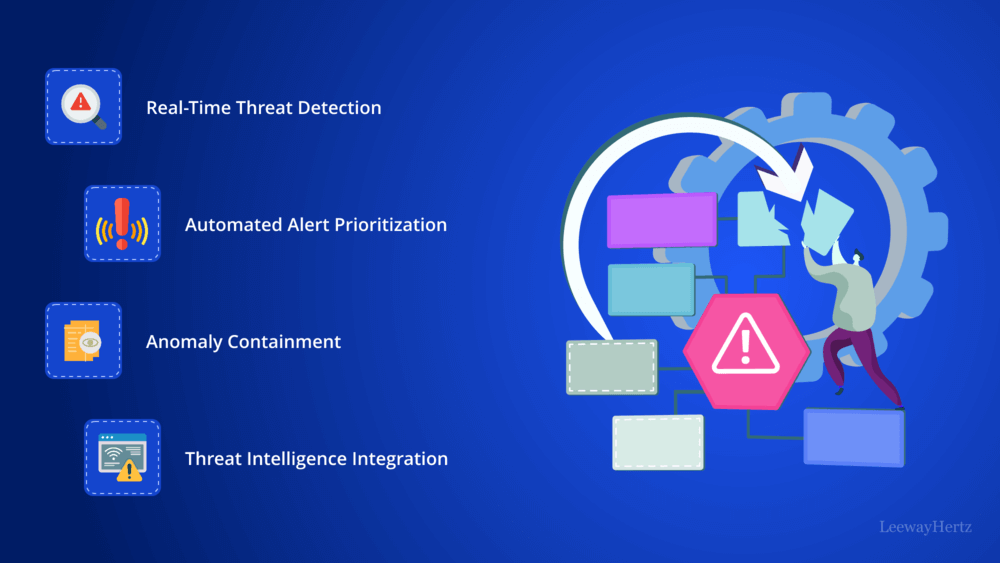
In today’s digital age, organizations continuously battle cyber threats, striving to stay ahead of adversaries who use sophisticated and stealthy methods. Traditional incident response methods, while effective, often need help to keep pace with the sheer scale and complexity of modern attacks. This leaves organizations vulnerable, with critical assets and sensitive data hanging in the balance.
Artificial Intelligence (AI) is a disruptive force poised to transform how we approach incident response. AI’s ability to rapidly analyze large datasets and detect real-time patterns significantly boosts the efficiency and effectiveness of incident response strategies, offering a major advancement in managing cybersecurity threats.
But what sets AI apart in the realm of incident response? Unlike conventional methods that rely solely on manual analysis, AI-powered solutions offer a proactive and predictive approach to threat detection. By using machine learning (ML) algorithms, these systems can detect subtle anomalies and potential threats that may evade human detection, enabling organizations to respond swiftly and decisively.
Moreover, AI’s adaptive capabilities enable continuous learning and improvement, ensuring that incident response strategies remain agile and effective in the face of evolving threats. This approach minimizes the impact of security incidents and enables organizations to stay ahead of emerging threats and change their overall cyber resilience.
AI represents a paradigm shift in incident response, offering organizations the tools to proactively detect, analyze, and mitigate threats in real-time. By embracing AI-powered solutions, organizations can strengthen security posture, mitigate risks, and safeguard their most critical assets in an increasingly digital world.
In this article, we will learn more about AI incident response, its use cases and key benefits.
- What is AI-powered incident response?
- Difference between traditional and AI-powered incident response
- Use cases of AI-powered incident response
- Streamlining incident response processes with GenAI
- How does AI in incident response work?
- AI-powered incident lifecycle management
- What is incident management, and how does it differ from incident response?
- Benefits of AI-powered incident response
- How does LeewayHertz aid in building an AI-powered incident response system?
- Incident response automation best practices
What is AI-powered incident response?
Incident response embodies the systematic procedure organizations adopt to handle the consequences of a cybersecurity breach or any other security incident. It involves identifying, containing, mitigating, and recovering from security breaches to minimize damage and restore normal operations swiftly.
AI-powered incident response automation is a branch of cybersecurity that uses artificial intelligence (AI) and machine learning (ML) to streamline and expedite handling security incidents. It builds upon the concept of Security Orchestration and Automation Response (SOAR) but with the added intelligence of AI to make decisions and take action. Integrating artificial intelligence (AI) into Security Orchestration, Automation, and Response (SOAR) amplifies its capabilities by infusing intelligent decision-making and action-taking into incident response processes. AI-driven automation empowers SOAR platforms to autonomously triage incidents, recommend response actions, and dynamically orchestrate response workflows based on evolving threat landscapes.
AI can significantly aid incident response by providing advanced threat detection, analysis, and response automation capabilities. AI-powered tools can continuously monitor networks, systems, and endpoints to detect unusual behavior or potential threats in real time, enabling rapid identification of security incidents. Additionally, AI can analyze vast amounts of security data to uncover patterns, trends, and anomalies that may indicate malicious activity, helping security teams prioritize and respond to threats effectively. Furthermore, AI-driven automation can streamline incident response processes by automatically executing predefined actions such as isolating compromised systems, blocking malicious traffic, and deploying patches or updates, thereby reducing response times and minimizing the impact of security incidents. AI in incident response can enhance the efficiency and effectiveness of incident response efforts, enabling organizations to protect their assets better and mitigate security risks.
Difference between traditional incident response and AI-powered incident response
Aspect | Traditional Incident Response | AI-Powered Incident Response |
---|---|---|
Data Analysis | Relies heavily on manual analysis of security logs and alerts | Utilizes AI and machine learning to analyze and correlate data from diverse sources in real-time |
Incident Triage | Triage is often manual, based on predefined rules and procedures | Automated incident triage using AI algorithms to prioritize incidents based on severity, urgency, and potential impact |
Task Execution | Relies on the manual execution of tasks by human analysts | Automates routine tasks such as ticket generation, alert validation, and resource allocation, reducing human intervention |
Response Time | Response time may be slower due to manual analysis and task execution | Faster response times due to real-time analysis and automated task execution, reducing the time to detect and respond to incidents |
Scalability | Limited scalability as response capabilities depend on human capacity | Highly scalable, capable of handling large volumes of incidents with minimal human intervention |
Adaptability | Limited adaptability to changing threat landscapes and evolving attack techniques | Adapts to evolving threats by continuously learning from new data and updating response strategies accordingly |
Decision Making | Relies on human decision-making based on experience and predefined procedures | Supports decision-making with AI-driven insights, recommendations, and predictive analytics |
Performance Monitoring | Monitoring of incident response performance may be manual and retrospective | Automated performance monitoring and reporting, providing real-time insights into response effectiveness and areas for improvement |
Continuous Improvement | Improvement is reliant on manual feedback analysis and process refinement | Facilitates continuous improvement through AI-driven analysis of incident data, identifying trends, and optimizing response strategies |
AI-powered incident response offers faster, more scalable, and adaptive capabilities compared to traditional methods, thanks to its reliance on AI, machine learning, and automation technologies.
Optimize Your Operations With AI Agents
Optimize your workflows with ZBrain AI agents that automate tasks and empower smarter, data-driven decisions.
Use cases of AI-powered incident response
The use cases of AI in incident response include:
Real-time threat detection
AI algorithms continuously analyze network traffic and system logs to detect abnormal behavior that may swiftly signal potential security threats. By leveraging ML and pattern recognition, AI identifies emerging risks proactively, enabling rapid response and mitigation measures to safeguard organizational assets and data integrity.
Automated alert prioritization
Utilizing advanced algorithms, AI automatically categorizes and ranks security alerts according to their severity and potential impact on the organization’s infrastructure. This streamlined approach empowers security teams to prioritize their efforts, focusing first on addressing the most significant threats and vulnerabilities. By optimizing resource allocation and response time, AI-driven alert prioritization enhances overall incident response effectiveness and resilience against cyber threats.
Anomaly containment
Employing advanced anomaly detection mechanisms, the system swiftly identifies and isolates change activities, like unexpected data access or transfer spikes, by comparing them against established norms. This proactive response effectively halts potential threats, mitigating the risk of data breaches or insider incidents and safeguarding organizational assets.
Targeted threat neutralization
AI utilizes continuous scanning capabilities; the system proactively disrupts cyber adversaries’ activities by detecting signs of hidden threats, such as irregular network communications. Upon identifying suspicious patterns, it intervenes promptly, terminating malicious processes and securing compromised data points to prevent further harm and change cybersecurity defenses.
Unified defense across environments
Acting as a cohesive security framework, the AI system seamlessly bridges on-premises and cloud infrastructures to ensure a coordinated response to incidents across the organization. By automatically enforcing security protocols, it contains and mitigates incidents while preserving operational continuity across all platforms, enhancing overall security posture and resilience.
Threat intelligence integration
AI-driven systems seamlessly integrate and analyze threat intelligence feeds in real time to identify emerging threats and vulnerabilities. These systems enhance threat detection capabilities by continuously updating and correlating threat data, enabling proactive responses to evolving cybersecurity threats.
Automated malware analysis
AI-powered malware analysis tools automate the analysis of suspicious files and executables to identify malware signatures and behavior patterns. By leveraging ML algorithms, these tools can quickly and accurately detect and classify malware, enabling rapid response and mitigation measures to protect against cyber threats.
Phishing detection and response
AI algorithms analyze various aspects of emails, including content, attachments, and sender behavior, to detect phishing attempts and prevent successful attacks. By examining email attributes and patterns indicative of phishing, AI enhances detection accuracy and enables timely response actions, such as quarantining malicious emails or alerting users to potential threats.
Incident triage and escalation
AI-driven incident response platforms streamline incident triage by automating the initial assessment process. Through intelligent analysis of incoming alerts and data, AI identifies high-priority incidents requiring immediate attention. These platforms automatically escalate such incidents to security analysts for further investigation, ensuring timely response and mitigation efforts.
Automated remediation
Using AI, incident response platforms automate remediation actions to contain and mitigate security incidents effectively. AI algorithms assess the nature and severity of incidents and orchestrate predefined response actions, such as isolating compromised endpoints, blocking malicious IPs, or applying security patches across affected systems. By automating these remediation steps, AI accelerates response times, minimizes manual intervention, and reduces the overall impact of security breaches.
Automated incident response playbooks
AI dynamically analyzes historical incident data, threat intelligence, and best practices to generate playbooks tailored to specific security incidents. By learning from past incidents, AI ensures that playbooks are continuously refined and optimized to address emerging threats effectively. AI continuously learns from the outcomes of response actions, adjusting playbook strategies to improve effectiveness over time. This adaptive approach ensures that playbooks evolve alongside the threat landscape, enabling security teams to stay ahead of emerging threats.
Security Information and Event Management (SIEM) integration and optimization
AI enriches SIEM systems by analyzing event data from various sources and enhancing it with contextual information, leading to more accurate threat detection. By reducing false positives through intelligent analysis, AI helps security teams focus on genuine security incidents, improving overall response efficiency. Additionally, AI continuously learns from historical data to enhance threat detection accuracy, identifying emerging threats that may evade traditional methods.
Threat hunting and adversary emulation
AI-powered threat-hunting platforms utilize machine learning to seek signs of compromise and suspicious behaviors actively, empowering security teams to detect and address threats before they escalate. Additionally, these platforms simulate adversary tactics and techniques, enabling organizations to evaluate their security stance and preparedness against advanced cyber threats. Through analysis of adversary behavior, AI identifies potential weaknesses in defensive measures and informs proactive security enhancements.
Multilingual support
In incident response, AI’s language processing abilities empower SOAR platforms to transcend linguistic barriers. By supporting multiple languages, AI enables seamless analysis of incident reports, extraction of information from non-English sources, and communication with global teams. This multilingual capability ensures that security teams can efficiently manage incidents and collaborate effectively across diverse international environments, thereby enhancing the overall effectiveness and reach of SOAR platforms in mitigating security threats on a global scale.
Summarization of an incident
AI systems use machine learning and advanced analytics to analyze security data and quickly create concise incident summaries. With natural language processing, AI extracts key insights from logs, alerts, and threat intelligence. This helps security analysts understand the incident’s nature, potential impact, and necessary mitigation steps. By providing clear incident descriptions, AI in SOAR facilitates informed decision-making and swift actions, changes defenses and minimizes the impact of security breaches.
Automated decision making
AI-driven automated decision-making is crucial for streamlining incident response processes by assessing available response actions and their potential outcomes. Security teams often have several response options when confronted with an incident, like blocking an IP address or launching a forensic investigation. AI utilizes historical incident data, threat intelligence feeds, and machine learning models to determine the most suitable response action. AI determines the best course of action by analyzing factors such as incident characteristics, severity, involved assets, and security policies. This automated decision-making saves time for security teams and reduces the risk of human error associated with manual decision-making.
Streamlining incident response processes with GenAI
Streamlining incident response processes with generative AI enhances the speed and accuracy of detecting, analyzing, and resolving system issues. Automating the incident analysis reduces downtime and improves overall system resilience. Here is how generative AI streamlines incident response processes:
Incident identification
Steps involved | Sub-steps involved | Role of GenAI |
---|---|---|
Data collection |
|
|
Pattern recognition |
|
|
Initial assessment |
|
|
Alert and escalation |
|
|
Incident analysis
Steps involved | Sub-steps involved | Role of GenAI |
---|---|---|
Root cause analysis |
|
|
Incident review |
|
|
Containment and security measures
Steps involved | Sub-steps involved | Role of GenAI |
---|---|---|
Immediate containment |
|
|
Segmentation of threat |
|
|
Risk mitigation |
|
|
Collaboration and communication |
|
|
Security enhancement |
|
|
System restoration and verification
Steps involved | Sub-steps involved | Role of GenAI |
---|---|---|
Restoration planning |
|
|
System recovery |
|
|
Verification and testing |
|
|
Post-incident analysis |
|
|
Post-incident activity
Steps involved | Sub-steps involved | Role of GenAI |
---|---|---|
Conduct a post-mortem review |
|
|
Update the incident response plan |
|
|
Communicate findings and recommendations |
|
|
Optimize Your Operations With AI Agents
Optimize your workflows with ZBrain AI agents that automate tasks and empower smarter, data-driven decisions.
How does AI in incident response work?
AI-driven incident response operates through a series of interconnected processes, each contributing to detecting, analyzing, and mitigating security threats effectively. Here’s a detailed explanation of how AI enhances these processes:
Data ingestion and normalization
The process begins by gathering data from various sources, such as network devices, security solutions, and application logs. This data is then normalized, meaning it is converted from various formats into a standard format.
For instance, log files from different systems are standardized so that timestamps, event types, and other key elements are consistently represented. This uniformity enables accurate analysis across disparate data sets, ensuring that AI models have a clear and consistent data landscape to work with.
ML and AI in threat detection
Machine Learning (ML) algorithms analyze historical security data to identify patterns of normal behavior and anomalies that could indicate potential threats.
For example, an ML model might analyze login patterns to detect brute-force attacks. It learns from each event to enhance its predictive accuracy, continually improving its ability to distinguish between regular and suspicious activities. This ongoing learning process allows AI systems to stay ahead of evolving threat patterns.
Event correlation and analysis
AI algorithms correlate events across different systems and, over time, identify relationships that signal complex multi-stage attacks.
For instance, a correlation engine might link an alert from an intrusion detection system about an external IP address with suspicious login attempts from the same IP. This correlation helps pinpoint coordinated attack efforts, allowing for a more in-depth understanding of the threat landscape.
Automated prioritization and response
After potential threats are identified, the system ranks incidents as per the severity and potential impact. Automated workflows then initiate responses according to predefined playbooks tailored to the threat’s nature.
For example, the system might isolate compromised systems, block malicious traffic, or implement other defensive measures automatically. This instant response is crucial for minimizing the damage and preventing the threat from spreading further.
Active defense through deception technologies
AI-driven incident response also includes deploying deception technologies such as decoys and honeypots. These technologies mislead attackers and gather intelligence about their tactics.
Decoys create simulated vulnerabilities or fake data assets that attract attackers. By analyzing attackers’ interactions with these decoys, the system gains valuable insights into cybercriminals’ methods and intentions without exposing real assets.
Feedback loops for adaptation and improvement
After an incident, AI systems use feedback loops to learn and adapt. The system evaluates the effectiveness of the response and uses this information to refine its detection algorithms and response strategies.
For example, if a particular response successfully mitigates a threat, the system will incorporate those strategies into future playbooks. Conversely, if the response is lacking, the system will adjust its processes to address those weaknesses. This continuous improvement cycle ensures that the AI-driven incident response system becomes more effective over time.
AI enhances incident response by automating data ingestion and normalization, utilizing ML for threat detection, correlating events for comprehensive analysis, and prioritizing and responding to threats swiftly. Active defense mechanisms like decoys add an extra layer of security, while feedback loops ensure constant adaptation and improvement. Together, these AI-driven processes enable organizations to detect, analyze, and mitigate security threats more efficiently and effectively than traditional methods.
AI-powered incident lifecycle management
AI plays a significant role in enhancing incident lifecycle management across various phases. Here’s how AI aids in each stage:
Step 1: Detection and identification
AI-driven algorithms can constantly monitor extensive data from diverse sources, such as system logs, network traffic, and user behavior, to identify anomalies and potential security threats.
Machine learning models can recognize patterns indicative of known attack vectors or abnormal activities, enabling early incident detection.
Step 2: Logging and recording
AI-based systems can automate the process of logging and recording incident details by parsing through log files, extracting relevant information, and populating incident reports.
Natural Language Processing (NLP) techniques can be employed to understand and classify incident reports, ensuring they are properly documented and categorized.
Step 3: Classification and prioritization
AI algorithms can analyze an incident’s characteristics and assess its severity, impact, and urgency in real-time, helping prioritize response efforts.
By integrating with risk assessment frameworks and organizational policies, AI can recommend appropriate response actions based on the nature of the incident and its potential consequences.
Step 4: Investigation and diagnosis
AI-powered forensic analysis tools can sift through large datasets to identify the root cause of incidents, such as malware infections, configuration errors, or system vulnerabilities.
Machine learning algorithms can correlate disparate data points and perform causal analysis to uncover hidden relationships between events, facilitating faster and more accurate diagnosis.
Step 5: Resolution and recovery
AI-driven automation can help rapidly contain and mitigate incidents by executing predefined response procedures or orchestrating complex remediation workflows.
AI-based predictive analytics can forecast the impact of different response actions and recommend the most effective strategies for minimizing downtime and restoring normal operations.
Step 6: Closure and documentation
AI-powered systems can generate comprehensive incident reports that summarize an incident’s entire lifecycle, including the actions taken, timelines, and outcomes.
Natural Language Generation (NLG) algorithms can produce narrative descriptions of incidents in human-readable language, making documentation more accessible to stakeholders.
Step 7: Review and analysis
AI tools can analyze historical incident data to identify trends, recurring patterns, and common vulnerabilities, enabling organizations to address underlying issues proactivel.
Machine learning algorithms learn from past incidents and continuously improve incident response processes by recommending policies, procedures, and resource allocation refinements.
AI empowers organizations to streamline incident lifecycle management, enhance response capabilities, and adapt more effectively to evolving cybersecurity threats and operational challenges. By leveraging AI technologies, organizations can achieve greater efficiency, accuracy, and resilience in mitigating and managing incidents across their lifecycle.
What is incident management, and how does it differ from incident response?
The key distinctions between incident management and incident response include:
- Focus and scope:
- Incident management: It is strategic and broad, encompassing the overall strategy, policies, and procedures for managing and mitigating incidents across the organization. It involves coordinating various stakeholders, communication, and compliance aspects.
- Incident response: It is tactical and focused, dealing with the immediate response to a security incident, such as containing the incident, analyzing the root cause, and restoring normal operations.
- Impact:
- Incident management: It tends to have greater long-term business effects as it involves communication with stakeholders, including employees, clients, regulators, and the public. Failure in incident management can lead to reputational damage and loss of organizational trust.
- Incident response: The immediate impact of incident response is substantial as it dictates the speed and effectiveness of an organization’s recovery from an attack or security incident. A proficient incident response effort can reduce the impact and prevent further damage.
- Interdependence:
- Incident management: It serves as the overarching strategy that guides incident response efforts. Its effectiveness heavily influences the success of incident response actions.
- Incident response: This directly impacts the organization’s ability to recover from and mitigate the effects of security incidents, thus shaping the overall success of incident management strategies.
- Testing and preparedness:
- Incident management: Regular testing of incident management plans, including communication protocols and stakeholder engagement strategies, ensures readiness to handle incidents and minimize long-term consequences effectively.
- Incident response: Conducting tabletop exercises to simulate real-world scenarios helps identify gaps and weaknesses in incident response procedures, such as inaccessible documentation during a cyberattack. This allows for refinement and improvement of response capabilities.
Incident management focuses on strategic planning and long-term implications; incident response addresses the tactical aspects of immediate incident handling. Both are integral components of an organization’s cybersecurity posture, and their effective coordination is crucial for mitigating the impact of security incidents and maintaining trust and reputation.
Benefits of AI-powered incident response
AI-powered incident response benefits organizations by enabling them to detect, analyze, and respond to security incidents efficiently. Some of the key benefits include:
- Enhanced incident detection and response: AI-driven systems swiftly and accurately detect incidents, outperforming traditional methods. This agility allows organizations to respond promptly, mitigating potential impacts and minimizing disruptions, safeguarding customer satisfaction.
- Faster response times: AI systems can automate incident response processes, enabling organizations to respond rapidly to security incidents. Automated responses can be triggered instantly upon the detection of a threat, reducing the time it takes to mitigate the impact of an attack.
- Accelerated root cause analysis: AI-enabled root cause analysis swiftly identifies underlying incident causes, expediting resolution and preventing recurrence. This rapid analysis reduces mean time to resolution (MTTR) and minimizes operational disruptions.
- Reduced workload: AI-powered incident response systems can significantly reduce security teams’ workloads by automating threat detection, analysis, and response tasks. This enables security professionals to focus on more complex tasks requiring human expertise.
- Improved accuracy: AI algorithms can analyze data with high accuracy, minimizing false positives and negatives in threat detection. This accuracy helps organizations avoid wasting time and resources investigating non-threatening events while ensuring that genuine threats are not overlooked.
- Scalability: AI-powered incident response systems can scale to handle large volumes of data and a growing number of security incidents without requiring a proportional increase in human resources. This scalability is especially valuable for organizations with limited security personnel or rapidly expanding digital infrastructures.
- Improved operational efficiency: AI-powered solutions automate tasks and processes within incident management, substantially reducing manual efforts. This automation liberates valuable resources, enhancing overall operational efficiency.
- Predictive and proactive incident management: AI-based incident management solutions leverage predictive analytics to foresee potential issues and recommend preventive measures. This approach empowers organizations to address vulnerabilities before they escalate, effectively mitigating risks.
- Continuous improvement and knowledge sharing: AI-driven incident management solutions continuously learn from past incidents, fostering a comprehensive knowledge base. This shared knowledge facilitates collaboration and disseminates best practices across teams and departments, driving continuous improvement.
- Cost savings and resource optimization: AI-powered incident management solutions yield substantial cost savings and optimize resource allocation for organizations through task automation, downtime reduction, and enhanced efficiency.
AI-powered incident response can significantly enhance an organization’s cybersecurity posture by improving threat detection, accelerating incident response times, and reducing the burden on security teams.
How does LeewayHertz aid in building an AI-powered incident response system?
LeewayHertz enables the development of an AI-powered incident response system by providing a comprehensive suite of services and expertise tailored to meet the specific needs of managing IT incidents effectively. Here’s how LeewayHertz can facilitate the creation of such a system:
Expert integration of IT events and third-party data
LeewayHertz excels in integrating diverse data sources, ensuring that all relevant IT occurrences and third-party events are seamlessly fed into the AI system. Their expertise in data engineering ensures the creation of a robust data pipeline that aggregates, normalizes, and processes data from various sources, providing a holistic view necessary for effective incident management.
Sophisticated alert management
LeewayHertz employs advanced AI and machine learning techniques to enhance alert management. They enable the system to:
- Color code alerts: Based on severity, ensuring critical issues are promptly identified.
- Categorize alerts: By time of occurrence, co-occurrence likelihood, and environmental factors, facilitating efficient prioritization and response.
- Predictive analytics: They implement predictive models that foresee potential incidents, allowing preemptive measures to be taken.
Automated and live incident analysis
With their deep AI expertise, LeewayHertz enables the system to:
- Conduct real-time analysis: Offering live insights into ongoing incidents.
- Perform root cause analysis: Automatically identifying the underlying causes of incidents, significantly reducing resolution times.
- Provide actionable insights: Delivering recommendations and next steps for incident resolution based on real-time data.
User-centric dashboards and interfaces
LeewayHertz designs intuitive and user-friendly dashboards that:
- Visualize alerts: Using color codes and grouping, making it easy for users to navigate and prioritize.
- Display incident groups: Based on various criteria for better understanding and quicker action.
- Enhance user experience: Ensuring the interfaces are responsive and accessible across different devices, facilitating better user engagement and efficiency.
Automated incident response
LeewayHertz integrates automation into incident response processes by:
- Triggering predefined actions: Based on incident type and severity, automating standard response procedures.
- Implementing notification systems: Ensure stakeholders are promptly informed through preferred communication channels like emails or SMS.
Scalable and reliable infrastructure
Leveraging cloud technologies, LeewayHertz ensures that the incident response system is scalable and reliable. Their cloud solutions can handle vast amounts of data and complex analyses, maintaining high performance and uptime even as data volume and complexity grow.
Compliance and security
LeewayHertz ensures the system complies with industry standards and regulations, implementing robust security measures to safeguard sensitive data. They provide data protection and compliance expertise, ensuring the system meets legal and regulatory requirements.
Continuous improvement and support
LeewayHertz provides ongoing support and continuous improvement for the incident response system. They implement feedback loops where the system learns from past incidents, enhancing its predictive and analytical capabilities. This ensures the system remains effective and up-to-date with AI advancements and incident management practices.
LeewayHertz empowers organizations to build a highly efficient, scalable, and secure AI-powered incident response system. By leveraging their expertise in AI, data integration, cloud computing, and security, LeewayHertz enables the creation of a system that not only automates incident response but also provides deep insights and fast resolutions, thereby enhancing the overall resilience and efficiency of IT operations.
Incident response automation best practices
Integration of the entire security ecosystem seamlessly: Leverage AI-driven incident response automation to seamlessly integrate all components of the security ecosystem, including SIEM, endpoint security, and network defenses. Ensure real-time communication among these components to form a cohesive defense system capable of efficiently identifying and neutralizing threats across the digital environment.
Regular updating and improvement of response strategies: Employ AI to continuously update and refine response strategies in line with the evolving threat landscape. Utilize AI algorithms to analyze the latest threat intelligence and past incidents, incorporating valuable insights to enhance the relevance and effectiveness of automation playbooks over time.
Strategic prioritization of incidents: Utilize AI-powered dynamic evaluation methods to prioritize incidents strategically based on their potential impact on critical business functions. Develop a detailed framework for assessing threats within the organization’s operational context, aiming to safeguard key assets and activities effectively.
Incorporation of the latest threat intelligence: Integrate AI-driven threat intelligence sources to anticipate and counter potential security threats effectively. Utilize comprehensive and globally sourced threat intelligence data to strengthen the defense mechanism and shift the incident response strategy towards a more predictive and proactive direction.
Design automation with compliance in mind: Use AI to build incident response automation workflows prioritizing compliance with regulatory standards. Develop automated response actions that effectively address threats and ensure documentation and adherence to legal and industry requirements.
Integration of automation with human insight: Create an ecosystem where AI-driven automation and human expertise complement each other in incident response. Strike a balance between automation’s speed and consistency with human critical thinking and creativity, allowing security professionals to apply their expertise when needed.
Continuous learning and improvement after each incident: Implement AI-driven post-incident analysis processes to extract valuable lessons from incidents and responses. Utilize in-depth analytics to continuously refine and improve automation strategies, ensuring ongoing enhancement of incident response capabilities.
Endnote
Integrating Artificial Intelligence (AI) into incident response marks a significant advancement in cybersecurity. By leveraging AI’s prowess in data analysis and automated decision-making, organizations can detect and respond to security threats with unprecedented speed and precision. This proactive approach minimizes the impact of security incidents and enables organizations to stay ahead of evolving threats. As AI technology evolves, its role in incident response becomes increasingly crucial, offering organizations the tools to navigate the dynamic cybersecurity landscape effectively. With AI-driven incident response, organizations can strengthen their defenses, mitigate risks, and safeguard their digital assets more effectively.
Unlock the power of AI in incident response today. Elevate your security strategy and safeguard your organization against cyber threats. Contact LeewayHertz experts for all your requirements!
Start a conversation by filling the form
All information will be kept confidential.
Insights
AI in architecture: Use cases, technologies, Implementations and development
AI in architecture is not merely a buzzword; it represents a profound shift in how we design, plan, and build the structures that shape our world.
How to build enterprise AI solutions for manufacturing?
AI in manufacturing leverages technologies like machine learning and deep learning neural networks to analyze vast data from various sources and facilitates improved decision-making by enhancing data analysis capabilities.
Adopting AI for customer success: Shaping a new era of user assistance
Customer success is a strategic approach where businesses proactively guide customers through a product journey to ensure they achieve their desired outcomes, thereby enhancing customer satisfaction, loyalty, and advocacy.