AI in fleet management: Use cases, benefits, architecture, technologies and solution
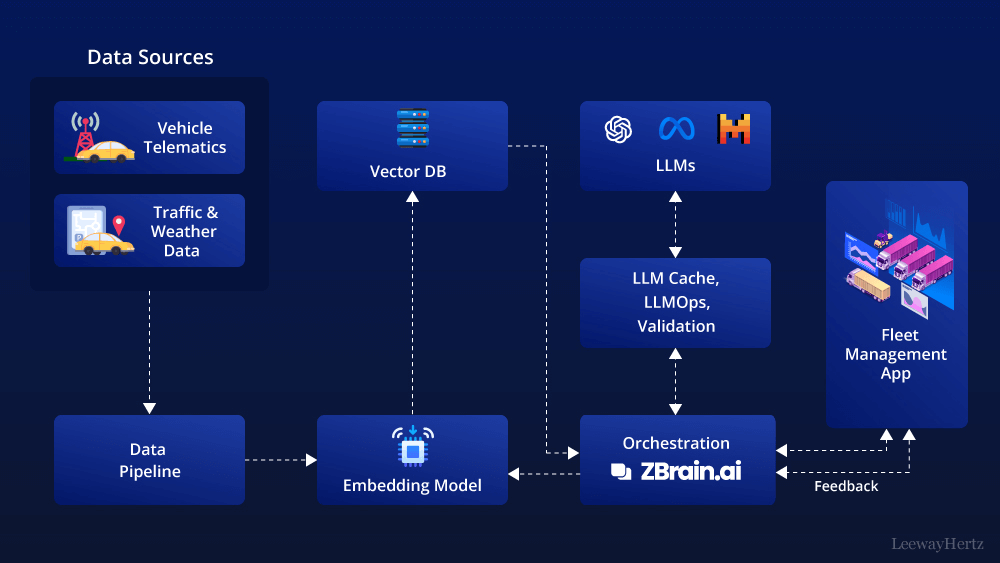
In today’s fast-paced world of transportation and logistics, fleet management plays a crucial role in maximizing efficiency to meet the ever-growing demands of a dynamic market. It is the meticulous orchestration and supervision of vehicles, driving forward operational excellence, cost savings, and peak productivity. With logistics and transportation industries fast gaining traction, there is an escalating demand for avant-garde solutions to elevate fleet performance. Enter Artificial Intelligence (AI)—a transformative force redefining fleet management with unparalleled capabilities. From harnessing data for insightful decisions and predictive maintenance to optimizing routes and enhancing safety to setting new benchmarks, AI is transforming the landscape.
Companies are not merely adopting but actively integrating AI-driven fleet management tools to carve out a distinctive competitive advantage. These AI-driven solutions enhance operational efficacy and equip managers with a comprehensive view for informed decision-making, assuring the optimal use of resources and heightened safety protocols for the entire fleet. A study by Fortune Business Insights paints a promising picture: the global fleet management software market is poised to soar from $23.67 billion in 2023 to a staggering $79.82 billion by 2030, charting a Compound Annual Growth Rate (CAGR) of 19% during this period.
One of the prime reasons behind the growing use of AI in fleet management is its ability to sift through and analyze mountains of data—from GPS coordinates to intricate sensor readings and from historical performance metrics to the here-and-now statistics. Such rich data aids in crafting real-time decisions that streamline processes and minimize costs. For example, advanced AI algorithms can forecast maintenance requirements, allowing companies to preemptively schedule repairs, reducing downtime and lowering repair costs. Furthermore, AI facilitates the dynamic optimization of routes, considering traffic flux, meteorological changes, and other unpredictable factors, leading to quicker deliveries and reduced fuel usage. Beyond operational efficiency, AI champions safety by vigilantly tracking driver patterns, offering instant feedback, and taking steps to avert potential mishaps.
Explore this article to understand the transformative impact of AI in fleet management, its nuances and its diverse applications across sectors.
- What is fleet management?
- What are the technologies behind AI-enabled fleet management?
- The key components of an effective fleet management strategy
- How does AI in fleet management work?
- Use cases of AI in fleet management
- Streamlining fleet management operations with GenAI
- How does LeewayHertz’s generative AI platform optimize fleet management workflows?
- LeewayHertz’s AI development services for fleet management
- Benefits of AI in fleet management
- The future of AI in fleet management
What is fleet management?
Fleet management is the process of organizing, coordinating, and facilitating the operation and maintenance of a fleet of vehicles within a company or organization. It’s a procedural necessity and a strategic function vital for businesses and agencies where transportation is at the heart of service or product delivery. Its primary objective is to control costs, enhance productivity, and mitigate risks associated with operating a fleet of vehicles. This systematic approach drives several essential benefits for an organization, including improved efficiency in vehicle acquisition, effective fuel management, strengthened fleet compliance, heightened employee safety, and reduced carbon emissions.
At its core, fleet management entails various responsibilities overseen by fleet managers. These responsibilities encompass vehicle maintenance, meticulous tracking of fuel consumption and associated costs, effective driver management, optimizing asset utilization, planning efficient routes, and implementing programs aimed at boosting overall company productivity while minimizing waste.
Organizations often leverage specialized tools and technologies, such as vehicle telematics and fleet management software, for efficient fleet management. These advanced solutions enhance fleet efficiency, streamline operations, and reduce operational costs.
What are the technologies behind AI-enabled fleet management?
Explore the pivotal technologies underpinning automated fleet management, driving advancements in both safety and efficiency for fleets.
Telematics
Telematics systems play a crucial role in modern fleet management by collecting and analyzing real-time data from various vehicle sensors. This technology gathers information such as location through GPS, speed via accelerometers, and vehicle health using engine sensors. The wealth of data can be overwhelming for fleet managers, which is where AI steps in to process and translate this data into actionable insights.
By leveraging AI algorithms, telematics systems can turn large data sets into meaningful insights about vehicle performance and driver behavior. These insights can help fleet managers identify potential issues and take proactive measures to enhance fleet safety, efficiency, and productivity. A significant reduction in fleet accidents is a primary advantage of integrating AI into telematics systems.
Machine learning
You can use machine learning techniques to ‘feed’ algorithms with telematics data, allowing them to learn and build predictive models without explicit programming. This enables data analytics systems to process vast amounts of information and analyze historical events, allowing predictions of future events.
The predictive capabilities derived from ML empower businesses to anticipate events before they occur, providing a competitive advantage. For instance, with the help of historical telematics data and predictive models, businesses can foresee when vehicles require maintenance based on usage patterns. This proactive approach prevents accidents and enhances employee safety while keeping operational costs in check.
Integrating AI and machine learning with telematics amplifies the potential to drive efficiency, safety, and cost-effectiveness in fleet management and other industries that rely on data-driven decision-making.
Computer vision
In contemporary fleet management and automotive technology, lane departure warning, blind spot detection, etc., are noteworthy innovations. These systems employ sophisticated components such as sensors, cameras, Light Detection and Ranging (LIDAR), and image processing algorithms to bolster fleet safety.
Lane departure warning utilizes real-time data to alert drivers about unintentional lane departures, minimizing accident risks. Blind spot detection enhances safety by detecting vehicles in blind spots and issuing timely warnings. Video telematics integrates various data sources and cameras to monitor road conditions, driver alertness, and incidents, aiding safety protocols and risk reduction in fleet management. These technologies represent pivotal advancements in road safety and operational efficiency.
Natural Language Processing (NLP)
NLP technology empowers AI systems to comprehend, interpret, and interact with human language, offering valuable applications in analyzing communication between drivers and fleet managers. This technology enables drivers to issue commands such as making phone calls, accessing real-time traffic information, or receiving alternative route suggestions, enhancing overall efficiency.
Furthermore, fleet managers can utilize NLP to provide immediate feedback to drivers through text-to-speech capabilities when detecting risky driving behavior. This direct and real-time communication contributes to enhanced safety and performance.
Incorporating AI-enabled hands-free interaction ensures drivers can maintain their focus on the road, reducing distractions and, consequently, the likelihood of accidents. NLP is a critical enabler of safer and more efficient communication within fleet management.
The real-world effectiveness of NLP would depend on various factors, including technological integration, system design, and user experience.
Optimize Your Operations With AI Agents
Optimize your workflows with ZBrain AI agents that automate tasks and empower smarter, data-driven decisions.
The key components of an effective fleet management strategy
Asset management
Vehicles stand out as one of the most substantial investments for organizations. The reliable performance of these assets is paramount in achieving operational goals and satisfying customer expectations. Mismanaged assets can result in amplified costs, reduced productivity, and compromised safety. Therefore, asset management is pivotal in the fleet management strategy.
Vehicle maintenance emerges as a cornerstone of asset management. Regular maintenance is indispensable for preserving vehicles in peak condition, averting breakdowns, and ensuring the safety of drivers and transported cargo. Taking a proactive stance toward maintenance entails adhering to recommended service schedules, conducting systematic inspections, and promptly addressing any identified issues. Implementing preventive maintenance programs empowers organizations to curtail unscheduled repairs and curb the risk of unforeseen vehicle failures that could disrupt operations.
Driver management
Effective driver management is the linchpin that can lead to increased productivity, cost reduction, and heightened customer satisfaction. Conversely, neglect in this area can result in accidents, elevated fuel consumption, poor customer experiences, and potential legal liabilities. As such, businesses must recognize driver management as a cornerstone within their fleet management strategy.
Furthermore, AI technology can be leveraged to enhance driver management. AI technology offers instant feedback to drivers regarding their driving habits, promptly notifying them about harsh acceleration, abrupt braking, or unsafe behaviors. This encourages drivers to self-correct, ultimately reducing the chances of accidents.
Cost management
Efficient cost management is essential for organizations relying on a fleet of vehicles, offering a gateway to operational optimization, enhanced profitability, and sustained competitiveness. An enterprise’s financial health and competitive edge hinge on prudent management and control of fleet costs. Fleet operations incur multifaceted expenditures encompassing fuel costs, vehicle maintenance, insurance, licensing, and depreciation. Ineffective cost management can lead to unwarranted expenses, reduced profitability, and compromised financial stability. Hence, businesses must prioritize cost management as a pivotal element of their fleet management strategy.
A reasonable selection of vehicles and their lifecycle management significantly contributes to cost-effective fleet management. Optimal vehicle selection tailored to specific operational requirements, encompassing payload capacity, fuel efficiency, and maintenance costs, can profoundly impact long-term cost management. Managing the lifecycle of vehicles necessitates a strategic approach to vehicle replacement, thereby minimizing maintenance costs associated with depreciated vehicles and capitalizing on new technologies offering improved fuel efficiency and performance.
Fuel management
Fuel management looms as a pivotal component of cost optimization and sustainability. The financial implications of fuel expenses are profound, making it imperative for businesses to manage fuel consumption actively. Fleet managers can meticulously gauge and analyze fuel usage by deploying fuel tracking systems. This data serves as a treasure trove, enabling managers to pinpoint areas for enhancement and execute strategies aimed at curtailing fuel consumption. An illustrative example is the role of a fleet tracker, which, by offering insights into fuel efficiency, empowers managers to make data-informed decisions that optimize routes and reduce delivery time.
The productive management of fuel results in substantial cost savings and aligns with an environmentally responsible agenda. Diligently monitoring fuel consumption and integrating cutting-edge fleet management technologies pave the way for reducing fuel expenses and positively contributing to a greener and more sustainable future.
Trailer tracking
In the complex network of fleet management, trailer tracking stands as a vital thread, offering valuable insights into trailer operations. Fleet management systems with real-time tracking capabilities provide a dynamic view of trailer locations within a fleet. This real-time data serves as a compass, guiding companies to plan and coordinate the utilization of trailers effectively. As a result, operational efficiency is enhanced, and cost savings are realized. By diligently monitoring trailer utilization, fleet managers can ensure that each trailer is maximally utilized, prolonging their lifespans and optimizing their value to the fleet.
Incorporating a fleet tracking system equips fleet managers with the means to monitor the movements of trailers, ensuring they are strategically positioned when needed, thus bolstering overall fleet productivity. Beyond optimizing productivity, trailer tracking enhances security, mitigating the risks of theft or unauthorized use. Fleet managers can receive immediate alerts in case of any unauthorized movement, courtesy of geofences that act as digital perimeters. These alerts prompt swift action, safeguarding assets and minimizing potential losses. Trailer tracking is a potent tool in the modern fleet manager’s toolkit, enhancing efficiency and security within fleet management.
How does AI in fleet management work?
Incorporating AI into fleet management significantly enhances fleet operations by employing advanced Large Language Models (LLMs) and connecting them with an organization’s comprehensive data sets. This innovative approach enhances data analysis and generates deep insights, helping in route optimization, vehicle maintenance, and enhancing driver safety and compliance.
This AI-driven architecture leverages various components to streamline fleet management. Here’s a step-by-step breakdown of how it works:
- Data sources: Effective fleet management relies on diverse and detailed data inputs, including:
- Vehicle telematics: Real-time data from vehicle sensors that monitor location, speed, fuel usage, and engine status are crucial for tracking and managing fleet performance.
- Driver behavior data: Information on driver habits, such as speed, braking patterns, and hours of operation.
- Maintenance records: Historical data on vehicle repairs and service checks, which aid in predictive maintenance and reducing vehicle downtime.
- Logistics and delivery data: Information on delivery schedules, cargo details, and customer feedback used to optimize delivery routes and improve customer satisfaction.
- Regulatory compliance data: Updates on transport regulations and compliance requirements essential for ensuring that fleet operations adhere to legal standards.
Data pipelines: Data from these varied sources are ingested and processed through sophisticated data pipelines that handle their cleaning and structuring, preparing the data for analysis.
Embedding model: An embedding model processes the prepared data, transforming it into a format that AI systems can effectively analyze. Popular models from providers like OpenAI, Google, and Cohere are utilized.
Vector database: The processed data is stored in a vector database such as Pinecone, Weaviate, or PGvector, enabling efficient and rapid querying.
APIs and plugins: APIs and plugins like Serp, Zapier, and Wolfram play a key role by connecting different components and enabling additional functionalities, such as accessing extra data, integrating with external tools or platforms or performing specific tasks with ease.
Orchestration layer: The orchestrating layer is critical in managing the workflow. ZBrain is an example of this layer that simplifies prompt chaining, manages interactions with external APIs by determining when API calls are required, retrieves contextual data from vector databases, and maintains memory across multiple LLM calls. Ultimately, this layer generates a prompt or a series of prompts that are submitted to a language model for processing. The role of this layer is to orchestrate the flow of data and tasks, ensuring seamless coordination across all components of the AI-based fleet management architecture.
Query execution: The data retrieval and generation process begins when the user submits a query to the fleet management app. Users can submit queries related to vehicle status, driver performance, or route optimization to the fleet management app.
LLM processing: Once received, the app transmits the query to the orchestration layer. This layer retrieves relevant data from the vector database and LLM cache and sends it to the appropriate LLM for processing. The choice of LLM depends on the nature of the query.
Output: The LLM generates an output based on the query and the data it receives. This output can come in various forms, such as optimized routes, maintenance schedules, and driver performance reports.
Fleet management app: This specialized app delivers AI-generated insights in an accessible format, enabling fleet operators to make informed decisions quickly and efficiently manage their fleet operations.
Feedback loop: User feedback on the LLM’s output is another important aspect of this architecture. The system incorporates user feedback to continuously improve the accuracy and relevance of the LLM’s outputs.
Agent: AI agents step into this process to address complex problems, interact with the external environment, and enhance learning through post-deployment experiences. They achieve this by employing advanced reasoning/planning, strategic tool utilization, and leveraging memory, recursion, and self-reflection.
LLM cache: Tools like Redis, SQLite, or GPTCache are used to cache frequently accessed information, speeding up the response time of the AI system.
Logging/LLMOps: Throughout this process, LLM operations (LLMOps) tools like Weights & Biases, MLflow, Helicone and Prompt Layer are employed to help log actions and monitor performance. This ensures the LLMs operate at the desired efficiency and evolve consistently through ongoing feedback mechanisms.
Validation: A validation layer is employed to validate the LLM’s output. This is achieved through tools like Guardrails, Guidance, Rebuff, and LMQL to ensure the accuracy and reliability of the information provided.
LLM APIs and hosting: LLM APIs and hosting platforms are essential for executing fleet management tasks and hosting the application. Depending on the requirements, developers can select from LLM APIs, such as those offered by companies like OpenAI and Anthropic or opt for open-source models. Similarly, they can choose hosting platforms from cloud providers like AWS, GCP, Azure, and Coreweave or opt for opinionated clouds like Databricks, Mosaic, and Anyscale. The choice of LLM APIs and cloud hosting platforms depends on the project’s needs and preferences.
This structured flow offers a comprehensive overview of how AI enhances fleet management processes. It utilizes advanced data analysis and AI-powered tools to streamline operations, improve safety, and optimize resource utilization.
Use cases of AI in fleet management
AI and ML technologies have been instrumental in innovating the development of fleet management software. AI in fleet management enables fleet managers to efficiently manage their entire fleet operations. The use cases of AI in fleet management include:
Rental and leasing
The car rental and leasing industry is experiencing rapid growth in adopting AI and ML technologies to improve the customer experience and optimize business performance. Key applications of AI and ML in this sector include:
- Customer service enhancement: AI-powered chatbots and virtual assistants are central to customer interactions in car rental and leasing companies. These AI-driven tools facilitate advanced services like booking rentals in advance, addressing common queries, and tailoring personalized packages based on individual preferences and historical rental and leasing data.
- Predictive maintenance: Car rental and leasing firms leverage AI and ML algorithms for predictive maintenance. These algorithms forecast when a vehicle may require maintenance by analyzing data such as vehicle mileage and the date of the last service. This proactive approach minimizes breakdowns during rental or lease periods, enhancing vehicle reliability, customer satisfaction, and overall trust in the company.
- Competitive pricing strategies: AI and ML are instrumental in optimizing pricing strategies. Rental and leasing companies harness these technologies to utilize data on vehicle preferences, demand patterns, and customer rental and leasing behaviors. This data-driven approach enables them to set competitive prices and identify and flag potentially fraudulent activities, such as using stolen credit cards or fake identifications. This helps minimize losses and ensures that prices remain competitive in the market.
E-hailing and ride-sharing
E-hailing services, including platforms like Uber, Lyft, and Bolt, rely on AI and ML as pivotal components to deliver efficient ride-sharing services. Here are several ways in which AI and ML are integral to the operations of e-hailing platforms:
- Connecting riders and drivers: E-hailing services harness AI and ML to connect riders with suitable drivers. These technologies consider multiple factors such as location, driver availability, and rider preferences (vehicle type or gender preference) to ensure the most convenient and efficient pairing.
- Route optimization: Upon matching a driver with a rider, the primary objective is to reach the destination swiftly and safely. AI and ML algorithms play an important role in optimizing routes for drivers, accounting for variables like traffic congestion, accidents, and other real-time factors. This benefits riders with affordable fares calculated at the end of the trip and enables drivers to use their time more productively, reduce fuel costs, and maximize their earnings.
- Enhancing safety: Safety is paramount in e-hailing services, protecting passengers and drivers while upholding a trustworthy reputation. ML algorithms are instrumental in prioritizing safety by collecting and analyzing data such as driver behavior, personal information, and driver ratings. This data enables e-hailing companies to identify potential safety hazards and take the necessary precautions, contributing to a secure and reliable experience for all stakeholders.
Transportation and logistics
The evolution of e-commerce and heightened delivery expectations has transformed the logistics landscape, with goods moving from point A to B to diverse locations, including homes and offices. AI and ML play pivotal roles in modern transportation and logistics in the following ways:
- Route optimization: Meeting the demands of swift delivery and handling sensitive products necessitates finding the quickest and safest routes. AI and ML systems analyze various data sources to optimize routes, helping to avoid delays, reduce delivery times, and minimize fuel consumption.
- Asset tracking: Asset tracking is paramount in ensuring goods’ security throughout the supply chain. AI and ML technologies, such as GPS, are utilized to monitor the location and condition of vehicles and cargo. Additionally, real-time performance analysis of drivers, including factors like driving speed and braking patterns, ensures safety and security at every stage of the supply chain.
- Enhanced security: Cargo theft poses a significant risk in the supply chain, leading to substantial losses. AI and ML-powered security measures, including cameras in warehouses and vehicles for detecting suspicious activities and driver identification tags to prevent unauthorized vehicle use or warehouse access, provide real-time alerts and notifications, contributing to the prevention of theft and loss.
Optimize Your Operations With AI Agents
Optimize your workflows with ZBrain AI agents that automate tasks and empower smarter, data-driven decisions.
Healthcare
- Medical supply and equipment distribution
AI-enhanced fleet management ensures the seamless flow of medical supplies, pharmaceuticals, and equipment to healthcare facilities. AI algorithms analyze various dynamic variables to optimize delivery routes and schedules in real-time, safeguarding a consistent supply chain that prevents disruptions and maintains adequate inventory levels. - Home healthcare services
AI plays a significant role for home healthcare providers, who frequently rely on fleets to reach patients within their residences. AI-driven tools optimize scheduling and route planning by processing vast amounts of data to make intelligent decisions, enabling healthcare providers to extend their services to more patients efficiently. - Maintenance and compliance
AI significantly enhances fleet management systems in the healthcare sector, where vehicle maintenance is crucial for patient safety. AI can predict when vehicles need maintenance, facilitating the proactive scheduling of vehicle maintenance, ongoing monitoring of vehicle conditions, and ensuring rigorous adherence to safety regulations imperative for maintaining quality patient care. - Cost efficiency
AI in fleet management helps healthcare organizations streamline operations and reduce operational costs. Through predictive analytics, AI helps optimize fuel consumption, minimizing maintenance expenses and improving route planning, resulting in significant cost savings that can be reinvested in patient care. - Emergency response
AI-powered fleet management systems are invaluable during emergencies such as natural disasters or large-scale accidents. They enable healthcare providers to mobilize swiftly by providing real-time insights and optimizing the deployment of vital resources to areas requiring immediate attention, ensuring a prompt and effective emergency response.
Travel
- Demand forecasting: AI can analyze historical booking data, travel trends, and external factors like holidays or events to predict demand for travel services. Fleet managers can adjust their vehicle allocation and schedules accordingly, ensuring they have the right number of vehicles and drivers to meet demand.
- Dynamic pricing: AI-driven pricing algorithms can adjust fares in real time based on demand, availability, and other variables. This helps maximize revenue during peak travel times and offers competitive pricing during off-peak periods.
- Safety and security: AI can enhance passenger safety by monitoring in-vehicle cameras for incidents and alerting fleet managers or authorities in real-time. It can also analyze driver behavior to ensure that safety protocols are followed.
- Real-time traffic updates: AI can provide real-time traffic updates and suggest alternative routes to drivers, helping them avoid congestion and reduce travel time. This is particularly valuable for shuttle and bus services in urban areas.
- Luggage tracking: AI-powered luggage tracking systems enhance the passenger experience by providing real-time location updates for travelers’ bags, minimizing the risk of lost luggage. This technology ensures greater peace of mind and convenience for air and ground travelers.
Manufacturing
- Optimized route planning: AI algorithms analyze real-time traffic data, delivery schedules, and vehicle capacity to optimize delivery routes. This ensures that manufacturing fleets reach their destinations efficiently, reducing fuel consumption and operational costs.
- Inventory management: AI helps manage and optimize inventory levels for raw materials, parts, and finished products. It ensures that manufacturing facilities have the right inventory at the right time, preventing delays and production stoppages.
- Fleet utilization: AI can determine the fleet’s most efficient allocation of vehicles and resources, minimizing idle time and operational expenses. Manufacturers maximize efficiency and utilization by intelligently assigning assets, ultimately enhancing productivity and cost-effectiveness.
- Load balancing: AI-driven load allocation ensures that manufacturing vehicles are loaded with precision, considering weight, volume, and delivery schedules. This optimized approach minimizes vehicle wear and tear, reduces fuel consumption, and enhances the fleet’s efficiency.
E-commerce
- Last-mile delivery optimization: AI helps determine the best strategies for last-mile delivery, including package handoff points, delivery time windows, and optimal routes for delivery personnel. This ensures prompt and efficient delivery to customers.
- Predictive analytics for demand planning: AI analyzes historical order data and external factors (e.g., holidays, promotions) to predict future demand. This helps e-commerce companies plan their fleet capacity and distribution strategies to meet customer expectations during peak times.
- Return logistics optimization: AI helps optimize return logistics by planning efficient routes for collecting returned items. This streamlines the return process, reduces transit time, and improves customer satisfaction.
- Warehouse and inventory management: AI optimizes inventory management by predicting product demand, allowing for efficient warehouse operations, reducing storage costs, and ensuring product availability for timely order fulfillment.
- Load balancing and vehicle utilization: AI algorithms optimize the allocation of deliveries to available vehicles, considering package sizes and weights. This maximizes vehicle utilization, reduces fuel consumption, and improves overall fleet efficiency.
Streamlining fleet management operations with GenAI
Generative AI is transforming fleet management, optimizing every aspect, from vehicle acquisition to maintenance and route optimization. From precisely predicting vehicle malfunctions and automating tasks like scheduling and dispatching to optimizing fuel consumption and enhancing driver safety, GenAI empowers fleet managers to make data-driven decisions, boost efficiency, and improve overall profitability.
Here are the roles of fleet management personas:
- Fleet manager: Oversees all aspects of fleet operations, maximizing efficiency, safety, and profitability.
- Dispatch coordinator: Ensures timely deliveries and efficient vehicle utilization through strategic scheduling and routing.
- Maintenance supervisor: Keeps the fleet in top condition, minimizing downtime and upholding safety standards.
- Driver trainer: Equips drivers with the skills and knowledge for safe, compliant, and efficient operations.
- Compliance officer: Guarantees adherence to all relevant regulations, ensuring the fleet operates legally and responsibly.
Here’s how GenAI is making a significant impact across the fleet management lifecycle:
- Vehicle acquisition
Steps involved | Sub steps | Role of GenAI |
Initiate acquisition |
|
Analyzes historical data, trends, and business projections to recommend vehicle acquisitions. |
Needs assessment |
|
Creates detailed profiles of suitable vehicle models, including cost of ownership analysis and regulatory compliance checks. Analyzes spending, depreciation, and financing options to recommend strategies. |
Vehicle selection |
|
Compares shortlisted vehicles, highlighting key features, performance metrics, and TCO projections Streamlines quote gathering and vendor negotiations using historical data and market benchmarks. |
Procurement and integration |
|
Assists in drafting and reviewing contracts, highlighting key clauses and potential risks. Populates fleet management systems with vehicle details, maintenance schedules, etc. Generates tailored notifications for stakeholders, including vehicle assignment details, training schedules, etc. |
Acquisition closure and reporting |
|
Automates task checklists, approvals, and notifications. Delivers data-driven insights on acquisition metrics, budget, and forecasts through interactive dashboards. Enables efficient storage, categorization, and retrieval of relevant documents using AI-powered search and tagging. |
- Compliance management
Steps involved | Sub steps | Role of GenAI |
Capture data and assess requirements |
|
Gathers data from diverse sources (DMV, EPA, internal systems) and standardize formats for efficient processing. Monitors and interprets evolving regulatory requirements, alerting stakeholders of relevant updates. |
Proactive compliance monitoring |
|
Schedules and sends timely reminders for license renewals, permit expirations, and upcoming inspections. Analyzes sensor and maintenance data to anticipate compliance issues and schedule maintenance. Analyzes driving patterns and fuel consumption to recommend strategies for reducing emissions. |
Compliance review and enforcement |
|
Routes compliance tasks to specialized team based on the type of violation or vehicle class. Extracts details from inspection reports, identifies discrepancies, and flags potential violations. Generates notifications to relevant parties, including drivers, managers, and regulatory bodies. |
Remediation and risk mitigation |
|
Suggests corrective actions based on the specific violation, vehicle type, and historical data. Streamlines the approval process for corrective actions and compliance plans. Evaluates the potential impact of compliance violations and prioritizes high-risk cases. |
Resolution and reporting |
|
Tracks compliance activities, generates regulatory reports, and provides insights for optimization. Enable stakeholders to accesss compliance status, documentation, and relevant communications. |
- Route optimization
Steps involved | Sub steps | Role of GenAI |
Data acquisition and analysis |
|
Identifies traffic patterns, seasonal trends, and historical route performance indicators. Forecasts traffic conditions based on historical data, real-time events, and external factors. Combines data from multiple sources to create a comprehensive operational view |
Route planning and optimization |
|
Finds the best routes based on various factors like delivery windows, vehicle capacities, and driver availability. Simulates different route scenarios and variations to identify the efficient options. Suggests adjustments based on real-time events or conditions. |
Route implementation and communication |
|
Automatically dispatches drivers to optimized routes, based on proximity, vehicle availability, and preferences. Provides drivers with real-time route guidance and updates. Monitors performance (deliveries, fuel, routes) and provides data-driven insights. |
Post-optimization review and feedback |
|
Analyzes route deviations and delays to identify patterns and optimize exception handling procedures. Visualizes route performance and optimization insights for stakeholders. |
- Incident management
Steps involved | Sub steps | Role of GenAI |
Capture and report incident |
|
Detects incidents in real-time via telematics, triggering automated workflow. Extracts details from unstructured data sources (e.g., witness statements, images) using NLP and computer vision. |
Impact assessment and initial response |
|
Estimates the operational and financial impact of the incident. Alerts key personnel based on incident severity for prompt response. Provides real-time location data and incident details to emergency responders. |
Recovery and repair coordination |
|
Optimizes maintenance and repair scheduling based on incident data. Streamlines the process of scheduling repairs and tracking repair progress. Pre-fills insurance claim forms with relevant data and evidence, expediting the claims process. |
Compliance and safety verification |
|
Auto-verifies compliance before returning vehicles to service. Streamlines the approval process for vehicle deployment based on specific criteria and risks. |
Resolution and reporting |
|
Captures insights from incidents and updates internal knowledge bases to improve prevention. Reports incident trends, root causes, and process effectiveness. |
- Maintenance request
Steps involved | Sub steps | Role of GenAI |
Capture and prioritize request |
|
Analyzes sensor data, maintenance history, and driver descriptions to diagnose issues and estimate repair urgency. Proactively generates maintenance alerts based on usage, sensor data, and historical trends to prevent breakdowns. Provides technicians with instant access to vehicle repair manuals, historical data, and recommended procedures. |
Assign and schedule maintenance |
|
Matches maintenance requests to technicians with the appropriate skills and availability. Forecasts parts demand based on history and automatically triggers purchase orders for parts. Optimizes appointment scheduling considering technician availability, downtime, etc. |
Service execution and completion |
|
GenAI optimizes AR (Augmented Reality) guidance for maintenance using data and feedback. Automates quality inspections, identifying potential defects or incomplete repairs. Generates detailed service reports, updates vehicle maintenance logs, and tracks repair costs automatically. |
Feedback and continuous improvement |
|
Sends automated notifications to operators and collects feedback on service quality. Analyzes customer feedback to identify areas for improvement. Analyzes maintenance data to improve scheduling and longevity. |
Optimize Your Operations With AI Agents
Optimize your workflows with ZBrain AI agents that automate tasks and empower smarter, data-driven decisions.
How does LeewayHertz’s generative AI platform optimize fleet management workflows?
LeewayHertz’s generative AI platform, ZBrain, plays a transformative role in optimizing fleet operations by offering solutions to optimize processes, fortify decision-making, and elevate overall operational efficiency. As a comprehensive, enterprise-ready platform, ZBrain empowers businesses to design and implement applications tailored to their specific operational requirements. The platform uses clients’ data, whether in the form of text, images, or documents, to train advanced LLMs like GPT-4, Vicuna, Llama 2, or GPT-NeoX for developing contextually aware applications capable of performing diverse tasks.
Within the realm of fleet management, enterprises face persistent challenges that include inefficient route planning, vehicle downtime, and the ongoing need for continuous tracking of performance metrics. ZBrain effectively addresses these challenges through its distinctive feature called “Flow,” which provides an intuitive interface that allows users to create intricate business logic for their apps without the need for coding. Flow’s easy-to-use drag-and-drop interface enables the seamless integration of large language models, prompt templates, and media models into your app’s logic for its easy conceptualization, creation, or modification.
ZBrain’s robust applications optimize fleet management operations by converting complex logistics data into actionable insights, resulting in enhanced vehicle performance, increased overall efficiency, and the establishment of a safer, more cost-effective, and customer-centric operation. The example below demonstrates how ZBrain transforms fleet management within the logistics industry:
AI-driven fleet management in logistics
Leverage ZBrain apps to optimize key facets of fleet management, including route planning, vehicle maintenance, and logistics coordination. The platform acquires pertinent data from diverse sources, including fleet details, operational data, GPS and telematics data, and maintenance records, which undergo automated Exploratory Data Analysis (EDA), revealing valuable insights to deepen the understanding of fleet management for informed decision-making. To enhance data analysis efficiency, ZBrain employs advanced embedding techniques, transforming textual data into numerical representations that capture semantic relationships, facilitating streamlined retrieval and analysis.
When a user submits a query for the fleet management report, ZBrain fetches relevant data based on query requirements. This data is then transmitted to the selected LLM model to generate a comprehensive and coherent fleet management report. Following report generation, ZBrain meticulously parses the output, extracting crucial details and structuring the data according to desired formats and guidelines. This integrated process ensures that ZBrain automates and refines data analysis and delivers actionable insights for optimized fleet management and decision-making.
ZBrain applications enable organizations to enhance fleet operations, elevate performance monitoring, and efficiently manage fleets while maintaining rigorous data privacy standards. The results span from improved resource allocation and heightened productivity to elevated service levels, fostering a comprehensive enhancement of operational efficiency. Dive into this detailed Flow to understand how ZBrain fundamentally redefines fleet management, providing a more agile and efficient logistics system adeptly adapting to dynamic market changes and evolving customer needs.
LeewayHertz’s AI development services for fleet management
At LeewayHertz, we specialize in crafting AI solutions tailored to the unique needs of fleet management companies. Our strategic AI/ML consulting empowers fleet management firms to leverage AI for enhanced decision-making, streamlined operations, and optimized resource utilization.
Through our expertise in developing Proof of Concepts (PoCs) and Minimum Viable Products (MVPs), we enable firms to preview the potential impacts of AI tools in real-world scenarios, ensuring that the solutions are effective and aligned with the fleet management sector’s specific requirements.
Our work in generative AI transforms routine tasks such as route optimization and vehicle maintenance scheduling, automating these processes to maximize fleet efficiency and minimize operational costs.
By refining large language models to comprehend the nuances of fleet management terminology and operational workflows, LeewayHertz enhances the accuracy and relevance of AI-driven communications and analyses.
Moreover, we ensure seamless integration of AI systems with existing technological infrastructures, enhancing operational efficiency and decision-making across fleet management operations.
Our AI solutions development expertise
AI solutions development for fleet management encompasses systems that enhance decision-making, automate routine tasks, and optimize fleet performance. These solutions integrate key components such as data aggregation technologies, which compile and analyze operational data from diverse sources. This comprehensive data foundation supports predictive analytics capabilities, enabling the forecasting of operational trends to inform strategic decisions. Additionally, machine learning algorithms are employed to tailor fleet management strategies to individual vehicle performance metrics and operational requirements. These solutions often cover areas like route optimization, vehicle maintenance scheduling, fuel efficiency management, driver behavior analysis, and regulatory compliance.
Overall, AI solutions in fleet management aim to optimize operational outcomes, improve efficiency, and enhance the overall fleet performance and safety.
AI agent/copilot development for fleet management
LeewayHertz develops custom AI agents and copilots that augment various fleet management operations, enabling companies to save time and resources while facilitating smarter decision-making. Here is how they help:
Route optimization:
- Analyzing historical and real-time data to optimize route planning and scheduling.
- Identifying potential route inefficiencies and suggesting alternative routes to minimize fuel consumption and travel time.
Vehicle maintenance management:
- Predicting maintenance requirements based on vehicle health data and usage patterns.
- Scheduling proactive maintenance tasks to prevent breakdowns and minimize downtime.
Fuel efficiency management:
- Analyzing fuel consumption patterns and identifying opportunities for fuel savings.
- Recommending fuel-efficient driving practices and vehicle configurations.
Regulatory compliance:
- Automating compliance documentation and reporting processes.
- Ensuring adherence to regulatory standards and requirements.
Customer service and engagement:
- Providing real-time updates on vehicle status and estimated arrival times to customers.
- Offering 24/7 virtual assistance to address customer queries and concerns.
By leveraging advanced AI solutions in fleet management, companies can achieve significant improvements in operational efficiency, cost savings, and customer satisfaction, gaining a competitive edge in the dynamic transportation industry.
Benefits of AI in fleet management
The benefits of AI in fleet management include:
Improved driver and vehicle safety: AI in fleet management systems uses real-time monitoring and analysis of driver behavior, identifying risky practices such as speeding, harsh braking, and risky driving habits. This immediate feedback allows for corrective action and training, promoting safer driving habits among drivers. As a result, this proactive approach reduces the likelihood of accidents, enhances overall road safety, and helps maintain a positive safety record for the fleet.
Vehicle tracking in real-time: Real-time vehicle tracking offers real-time location data and status updates for each vehicle in the fleet. This enables fleet managers to monitor the progress of deliveries, respond promptly to customer inquiries with accurate delivery times, and ensure efficient vehicle usage by optimizing routes and resource allocation. This increased visibility enhances operational transparency and customer satisfaction while reducing operational costs.
Improved vehicle routing: AI-driven fleet management systems use real-time data on traffic and road conditions to calculate the most efficient vehicle routes. Considering these factors, the system ensures vehicles take paths with minimal congestion and delays, reducing travel time. This enhances on-time delivery and lowers fuel consumption, leading to cost savings and a more eco-friendly fleet operation.
Improved dispatching: Real-time tracking provides up-to-the-minute information on the exact location and status of each vehicle in the fleet. This data empowers fleet managers to make informed decisions, swiftly delegating tasks to the closest available vehicles. This optimized dispatching reduces response times, improves delivery efficiency, and enhances overall fleet productivity, ensuring that resources are used effectively, and customer needs are met promptly.
Improved fuel efficiency: AI-driven fleet management optimizes routes, ensuring vehicles take the shortest and most fuel-efficient paths. It also monitors and improves driver behavior, minimizing practices like excessive idling or harsh acceleration, which wastes fuel. These measures lead to significant cost savings by reducing fuel consumption, and they also have a positive environmental impact by lowering greenhouse gas emissions, making fleet operations more sustainable and eco-friendly.
Improved safety: Real-time monitoring in fleet management involves constantly tracking and analyzing vehicles and drivers’ behavior. This approach allows fleet managers to receive immediate updates on vehicle location, driver actions, and performance metrics. In cases of safety violations, deviations from planned routes, or other issues, managers can swiftly intervene to maintain safety standards, reduce risks, and ensure that the fleet operates efficiently and by established guidelines. It promotes real-time decision-making and proactive responses to keep the fleet safe and productive.
Lower vehicle maintenance expenses: Predictive maintenance, driven by AI, utilizes data from sensors and historical performance to forecast when a vehicle or equipment is likely to experience issues or failures. This early detection enables proactive maintenance measures, preventing costly breakdowns and minimizing downtime. By addressing problems before they escalate, maintenance costs are significantly reduced, and the overall lifespan of fleet vehicles is extended, ensuring smoother and more cost-effective fleet operations.
Increased productivity: Real-time tracking offers complete visibility into fleet activities, enabling fleet managers to monitor vehicles and adjust routes in response to changing conditions. Optimized routing ensures that vehicles take the most efficient paths, saving time and fuel. Efficient dispatching, aided by these real-time insights, allows quicker task assignment to the nearest available vehicles. This holistic approach minimizes idle time, reduces unnecessary travel, and enhances overall operational efficiency, increasing productivity and cost savings.
These benefits collectively contribute to a more efficient and cost-effective fleet management operation, ensuring that vehicles are well-maintained, drivers are safe, and resources are utilized optimally.
The future of AI in fleet management
The evolution of AI promises exciting prospects for the future. AI is poised to enhance connected vehicle technology and communication systems, enabling vehicles to interact in real time, mitigating accidents and traffic congestion in the following ways:
- Vehicle-to-vehicle (V2V): Harnessing ML and Data Analytics, V2V emerges as a forefront technology, facilitating vehicles to share and analyze data seamlessly. It is an intelligent technology that allows vehicles to share data. By accessing speed and position details of nearby V2V-equipped vehicles through a wireless communication protocol akin to Wi-Fi, this information is utilized to notify drivers of potential hazards. V2V plays a pivotal role in curbing accidents and alleviating traffic congestion. Within a 300-meter radius, V2V can detect risky traffic conditions, road issues, ultimately enhancing road safety and predictability for all drivers.
- Vehicle-to-infrastructure (V2I): Integrating AI and Data Analytics, V2I becomes a cornerstone of the Intelligent Transport System. It gathers critical data like traffic congestion, bridge clearance levels, and traffic signal statuses. This data is transmitted wirelessly to inform drivers about pertinent conditions, greatly enhancing safety. Including smart traffic signals through V2I aids drivers in gaining a deeper understanding of traffic conditions, allowing for more accurate arrival time estimations. As this technology advances, it holds the potential to enhance driver-assistance systems and may play a pivotal role in future city planning for traffic lanes, parking facilities, and more.
- Vehicle-to-everything (V2X): V2X, infused with AI technologies like ML and DL, evolves as a comprehensive communication paradigm. V2X technology combines V2V and V2I to make every vehicle smarter and safer by enabling seamless communication with the entire traffic system, including other vehicles and infrastructure. It can swiftly alert drivers to nearby accidents, traffic congestion, and risky behaviors in close proximity. V2X directly channels information to the vehicle, reducing the driver’s reaction time. Additionally, it streamlines driving by automating toll and parking payments. While V2X communication is pivotal for the future of self-driving and autonomous vehicles, there are concerns about software malfunctions, cybersecurity threats, and loss of vehicle control, which could have severe consequences for businesses and their reputations.
In addition to these technologies, other innovations are on the horizon, such as Vehicle-to-network (V2N) for wireless vehicle-to-vehicle communication, Vehicle-to-grid (V2G) that taps into electric vehicle batteries for grid power etc.
Advanced and well-developed Artificial Intelligence holds the potential to reduce, if not eliminate, vehicle crashes significantly. While there will inevitably be challenges, as with any emerging technology, advanced AI is poised to pave the way for safer and more interconnected road systems, benefiting both employees and customers. This transformation promises more precise route planning, enhanced vehicle diagnostics, and the prospect of safer and more intuitive automated vehicles that demand minimal driver and fleet management involvement. AI is driving a rapid transformation of the fleet management industry, ushering in unprecedented efficiency and safety improvements.
Endnote
AI in fleet management has emerged as a significant driving factor, fundamentally reshaping conventional practices and enhancing contemporary transportation and logistics businesses’ efficiency, safety, and sustainability. Its notable capabilities in data analysis, predictive maintenance, route optimization, fuel efficiency improvement, and safety monitoring are distinctly altering the landscape of fleet operations. This synergy between AI and fleet management streamlines operations and ensures a sustainable, competitive edge, ushering in a future where fleets are smarter, more responsive, and safer than ever.
As businesses increasingly integrate AI in fleet management strategies, they unlock a world of possibilities, optimizing their operations and gaining a competitive advantage in a dynamic market. The potential for AI to further evolve, driven by advancements in machine learning and data processing, presents an exciting future for fleet management. Harnessing AI in fleet management will save costs and streamline operations. It also paves the way for a safer and more sustainable future, ensuring that businesses stay at the forefront of innovation in the ever-evolving world of transportation.
Leverage the potential of AI in fleet management to drive efficiency, enhance quality, and optimize your business processes. Contact LeewayHertz today for all your AI consultancy and development needs!
Start a conversation by filling the form
All information will be kept confidential.
FAQs
What is fleet management?
Fleet management is organizing, coordinating, and facilitating the operation and maintenance of a fleet of vehicles within a company or organization. It involves strategic planning to control costs, enhance productivity, and mitigate fleet operating risks.
How does AI enhance fleet management?
AI enhances fleet management in several ways:
- Predictive Maintenance: AI analyzes data from sensors and historical maintenance records to predict when vehicles need maintenance, reducing downtime and preventing unexpected breakdowns.
- Route Optimization: AI algorithms optimize routes based on real-time traffic data, and historical patterns, minimizing fuel consumption and improving delivery times.
- Fuel Efficiency: AI-powered systems monitor driving behavior and vehicle conditions to provide recommendations for improving fuel efficiency, reducing operational costs, and minimizing environmental impact.
- Telematics and Monitoring: AI collects and analyzes data from telematics devices, offering insights into driver behavior, vehicle performance, and overall fleet health for informed decision-making.
- Driver Safety: AI applications monitor driver behavior, detect fatigue or distracted driving, and provide alerts or interventions to enhance overall safety and reduce the risk of accidents.
- Inventory Management: AI helps optimize inventory levels by analyzing demand patterns, ensuring that the right amount of goods is stocked in each vehicle, minimizing excess or insufficient inventory.
- Real-time Tracking: AI enables real-time tracking of vehicles, providing accurate and up-to-date information on the location and status of each vehicle in the fleet.
- Load Optimization: AI algorithms assist in optimizing the distribution of loads among vehicles, ensuring efficient use of resources and reducing transportation costs.
- Dynamic Scheduling: AI dynamically adjusts schedules based on changing circumstances, such as traffic conditions or unexpected delays, to maintain operational efficiency.
- Cost Optimization: AI-driven analytics provide insights into operational inefficiencies and cost-saving opportunities, helping fleet managers make informed decisions to optimize overall fleet performance.
In summary, AI enhances fleet management by improving operational efficiency, reducing costs, enhancing safety, and providing valuable insights for informed decision-making.
What are the key technologies behind AI-enabled fleet management?
The key technologies include telematics for real-time data collection, machine learning for predictive analytics, computer vision for safety features, and natural language processing (NLP) for hands-free interaction and communication.
How does AI enhance cost management in fleet operations?
AI in fleet management optimizes vehicle selection, lifecycle management, and fuel consumption, contributing to cost-effective fleet management. Predictive analytics helps anticipate maintenance needs, preventing costly breakdowns and reducing operational costs.
How does AI enhance safety in emergency response situations?
AI-powered fleet management systems are crucial during emergencies. They provide real-time insights, optimize resource deployment, and enhance communication, enabling swift and effective emergency response in natural disasters or large-scale accidents.
How can organizations benefit from LeewayHertz's AI solutions designed for fleet management?
Organizations partnering with LeewayHertz gain access to cutting-edge AI solutions that improve safety, efficiency, and cost-effectiveness in fleet management. The implemented technologies result in enhanced operational transparency and customer satisfaction.
What support and maintenance services does LeewayHertz provide for AI-enabled fleet management solutions?
LeewayHertz offers comprehensive support and maintenance services for AI-enabled Fleet Management solutions. This includes troubleshooting, updates, and continuous improvements to ensure the solutions’ longevity and optimal performance.