AI in financial modeling: Applications, benefits, architecture, implementation, and future trends
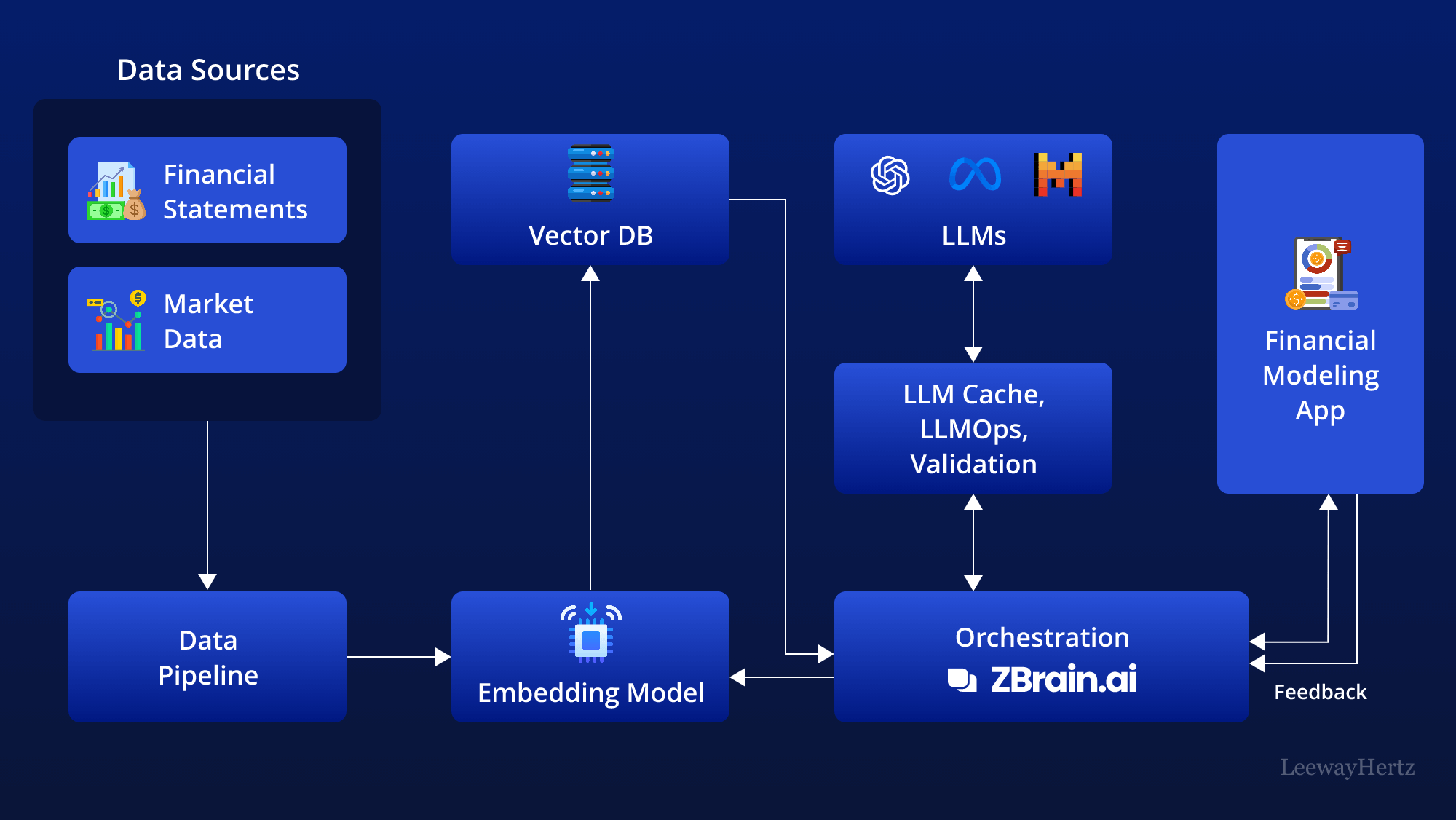
Financial modeling is the cornerstone of strategic decision-making in finance. It serves as the guiding tool for investment decisions, forecasting cash flows and uncovering opportunities in the dynamic financial business setting. However, traditional financial modeling, such as handling massive datasets and adapting to dynamic market conditions, is time-consuming and prone to human errors. AI steps in as a game-changer in such a scenario, altering how financial modeling works and making decision-making in finance even better.
This article explores the transformative impact of AI-based financial modeling in insurance, banking, private equity, investment and other financial sectors. We will examine the key differences between AI-based and traditional financial modeling, highlighting the challenges faced by traditional methods and how AI addresses them. Finally, we will discuss the practical implementation of AI in financial modeling and explore the exciting future trends and innovations shaping this field.
- An overview of AI-based financial modeling
- AI-based financial modeling vs. traditional financial modeling
- Challenges in traditional financial modeling and how AI addresses them
- How does AI in financial modeling work?
- Applications of AI in financial modeling
- Data processing and analysis
- Automation of routine tasks
- Predictive analytics
- Natural Language Processing (NLP)
- Risk assessment and management
- Scenario analysis and sensitivity analysis
- Fraud detection and security
- Compliance and regulatory reporting
- Personalized financial advice
- Valuation
- Investment analysis
- Mergers and acquisitions (M&A)
- Budgeting and forecasting
- Loan and credit analysis
- Financial reporting
- Tax planning
- Pension fund management
- Strategic planning and analysis
- How does AI for financial modeling benefit the private equity and investment sector?
- Benefits of AI in financial modeling
- How to implement AI in financial modeling?
- LeewayHertz’s AI development services for financial modeling
- Ethical considerations for implementing AI in financial modeling
- Future trends and innovations in AI for financial modeling
An overview of AI-based financial modeling
Financial modeling is the process of creating a mathematical representation of a company’s financial performance, used for forecasting, investment evaluation, and risk assessment. It is a crucial tool for financial analysts to make data-driven decisions. This traditional process often relies on human analysts to gather data, make assumptions, and build models.
AI-based financial modeling is transforming this process by utilizing advanced machine learning algorithms and data analytics. These AI systems can analyze massive volumes of data, identify intricate patterns, and generate highly accurate forecasts, enabling financial professionals to make more informed decisions and respond rapidly to market changes.
In addition to enhancing accuracy and efficiency, AI-based models can process unstructured data from diverse sources, such as social media and news articles. This broader data integration provides a more comprehensive view of market dynamics, leading to more robust financial insights. As AI technology continues to evolve, its integration into financial modeling is poised to offer even greater opportunities for strategic advancements and operational improvements in the financial sector.
AI-based financial modeling vs. traditional financial modeling
Aspect | AI-based Financial Modeling | Traditional Financial Modeling |
---|---|---|
Data processing | Handles vast amounts of data quickly and efficiently using advanced algorithms and machine learning techniques. | Processes data manually or with basic tools, often limited in handling large volumes or complex datasets. |
Accuracy | Provides higher accuracy through sophisticated algorithms and continuous learning from new data. | Relies on historical data and static formulas, which may not capture recent market changes effectively. |
Real-time analysis | Offers real-time or near-real-time analysis and updates, adapting to market changes instantaneously. | Analysis is often periodic, with updates based on scheduled intervals, which may lag behind real-time changes. |
Scalability | Easily scalable to handle increased data volume and complexity without significant manual intervention. | Scalability is limited, often requiring additional manual work or increased resources as data volume grows. |
Predictive capabilities | Uses advanced predictive analytics and machine learning models to forecast trends and outcomes. | Predictive capabilities are often limited to historical data and simpler extrapolation methods. |
Automation | Automates repetitive tasks such as data entry, cleansing, and reporting, reducing human error and increasing efficiency. | Requires manual input for data entry, cleaning, and report generation, leading to higher chances of errors. |
Complexity handling | Effectively manages complex variables and interactions using advanced modeling techniques. | Handles simpler models with limited ability to integrate multiple complex factors. |
Flexibility | Highly adaptable to different scenarios and able to incorporate diverse data sources and variables. | Less flexible, often requiring significant manual adjustments to account for new scenarios or data. |
Decision support | Provides data-driven insights with high precision, supporting more informed and strategic decision-making. | Relies on historical data and human judgment, which may not fully capture new market dynamics. |
Cost and time efficiency | Reduces time and costs associated with manual data processing and analysis, especially for large datasets. | Higher time and cost investment in manual data handling and analysis processes. |
Risk management | Enhances risk management with real-time monitoring and predictive analytics for early warning systems. | Risk management is based on periodic reviews and static models, which may not capture real-time risks effectively. |
Integration with other systems | Seamlessly integrates with other AI-driven systems and data sources for comprehensive analysis. | Integration with other systems is often manual or limited, leading to potential data silos and inconsistencies. |
Learning and adaptation | Continuously learns from new data, improving model accuracy and insights over time. | Static models do not learn or adapt, requiring periodic manual updates and adjustments. |
Challenges in traditional financial modeling and how AI addresses them
AI can address several challenges in traditional financial modeling, offering solutions that improve accuracy, efficiency, and decision-making. Here are some key challenges and how AI can help overcome them:
Handling large and complex data sets
- Challenge: Traditional financial models struggle to process and analyze vast amounts of data quickly and accurately.
- AI solution: AI algorithms can efficiently process large and complex data sets, extracting meaningful insights from structured and unstructured data sources. Machine learning models can identify hidden patterns and relationships that may be challenging for humans to discern.
Manual data entry and error-prone processes
- Challenge: Manual data entry can introduce errors into financial models, leading to inaccurate results and decisions.
- AI solution: AI automates data gathering, cleansing, and entry, reducing the risk of errors. Natural Language Processing (NLP) can extract information from documents and reports, eliminating the need for manual data input.
Time-consuming forecasting and analysis
- Challenge: Traditional financial modeling often involves time-consuming tasks like data analysis, forecasting, and scenario testing.
- AI solution: AI accelerates these processes. Machine learning models can make rapid and accurate predictions, allowing for faster scenario analysis and decision-making.
Limited data sources and traditional metrics
- Challenge: Traditional models may rely on a limited set of financial metrics and data sources, potentially missing valuable information.
- AI solution: AI can incorporate a broader range of data sources, including non-traditional data like social media sentiment and news articles. This enriches the analysis and provides a more comprehensive view of market conditions.
Difficulty in real-time analysis
- Challenge: Traditional models often struggle to provide real-time insights, which are crucial in fast-moving financial markets.
- AI solution: AI-powered algorithms can analyze data in real-time, making it possible to respond quickly to changing market conditions, assess risks, and seize opportunities.
Risk management complexity
- Challenge: Managing financial risks effectively is a complex task due to the dynamic nature of markets.
- AI solution: AI models can identify and assess various types of financial risks, such as credit risk, market risk, and operational risk, in real-time. They provide early warnings and support proactive risk management.
Regulatory compliance and reporting
- Challenge: Complying with complex financial regulations and reporting requirements can be time-consuming and error-prone.
- AI solution: AI automates compliance tasks, ensuring accurate and timely reporting while reducing the risk of regulatory violations.
Lack of personalization
- Challenge: Traditional models may offer one-size-fits-all solutions without considering individual preferences and risk tolerance.
- AI solution: AI-driven robo-advisors provide personalized investment advice and portfolio management based on individual goals and risk profiles, enhancing the customer experience.
Difficulty in portfolio optimization
- Challenge: Constructing well-diversified portfolios manually can be challenging, leading to suboptimal asset allocation.
- AI solution: AI-powered portfolio optimization models use advanced algorithms to construct and manage portfolios that maximize risk-adjusted returns based on individual preferences.
How does AI in financial modeling work?
Integrating AI into financial modeling entails utilizing various components to streamline data analysis, extract insights, and bolster decision-making. This transcends conventional financial modeling methods by harnessing advanced Large Language Models (LLMs) and integrating them with the organization’s proprietary knowledge base. This architecture unleashes optimized insight generation, enabling businesses to confidently make timely, data-driven decisions in the financial modeling realm.
The architecture leverages various components to streamline the financial modeling process. Here’s a step-by-step breakdown of how it works:
- Data sources: The process begins by gathering data from various sources relevant to the financial modeling process. This data can include:
- Financial statements: Financial modeling often relies on data from company financial statements, such as income statements, balance sheets, and cash flow statements. These documents comprehensively overview a company’s financial health and performance.
- Market data: Market data from various sources such as stock exchanges, financial news outlets, and market research firms offer crucial information on stock prices, interest rates, commodity prices. Incorporating this data enriches financial models with real-time market insights, facilitating more accurate forecasts and risk assessments.
- Company filings and disclosures: In addition to financial statements, company filings such as annual reports, quarterly filings (e.g., 10-Q), and disclosures to regulatory bodies (e.g., SEC filings) contain valuable information on corporate strategy, risk factors, legal proceedings, and management discussions. Analyzing these documents enhances the depth and accuracy of financial models by providing insights into company-specific factors and risks.
- Alternative data sources: Alternative data sources, including social media sentiment analysis, web scraping etc., offer unconventional insights into market trends and consumer behavior. Integrating alternative data into financial models enables businesses to uncover hidden opportunities and mitigate risks not captured by traditional data sources.
- Data pipelines: Information sourced from the specified references undergoes processing through data pipelines. These pipelines are tasked with collecting, refining, and organizing data, preparing it for subsequent analysis.
- Embedding model: The prepped data undergoes processing through an embedding model. This model converts the textual data into numerical representations called vectors, enabling AI models to comprehend it. Prominent models include those developed by OpenAI, Google, and Cohere.
- Vector database: The resultant vectors are stored in a vector database, facilitating efficient querying and retrieval. Leading vector databases include Pinecone, Weaviate, and PGvector.
- APIs and plugins: APIs and plugins like Serp, Zapier, and Wolfram play a pivotal role by linking various components and facilitating additional functionalities, such as accessing extra data or effortlessly executing specific tasks.
- Orchestration layer: The orchestrating layer is vital in overseeing the workflow. One example is ZBrain, which exemplifies this layer by streamlining prompt chaining, overseeing interactions with external APIs to ascertain when API calls are necessary, fetching contextual data from vector databases, and preserving memory throughout multiple LLM calls. Ultimately, this layer crafts a prompt or sequence of prompts for submission to a language model for processing. It orchestrates the data and task flow, ensuring smooth coordination across all components in the financial modeling architecture.
- Query execution: The data retrieval and generation process commences upon the user submitting a query to the financial modeling app. This query can encompass various aspects pertinent to financial modeling, such as a company’s financial health, regulatory compliance, or operational risks.
- LLM processing: Upon receiving the query, the app forwards it to the orchestration layer. This layer then retrieves pertinent data from the vector database and LLM cache and dispatches it to the suitable LLM for processing. The selection of LLM depends on the query’s nature.
- Output: The LLM generates an output based on the query and the data it receives. This output can take various forms, including financial forecasts, sensitivity analysis reports, and risk assessments.
- Financial modeling app: The validated output is then presented to the user through the financial modeling app, serving as the core application where all data, analyses, and insights converge. Specifically tailored for financial modeling, this app offers findings in a user-friendly format tailored for decision-makers.
- Feedback loop: User feedback on the LLM’s output constitutes another crucial aspect of this architecture. This feedback loop is instrumental in progressively refining the accuracy and relevance of the LLM’s output over time.
- Agent: AI agents are crucial in addressing complex problems, interacting with the external environment, and enhancing learning through post-deployment experiences. They achieve this by employing advanced reasoning and planning techniques, utilizing strategic tools, and leveraging memory, recursion, and self-reflection capabilities.
- LLM cache: Tools such as Redis, SQLite, or GPTCache are used to cache frequently accessed information, thereby accelerating the AI system’s response time.
- Logging/LLMOps: Throughout this process, LLM operations (LLMOps) tools like Weights & Biases, MLflow, Helicone, and Prompt Layer aid in logging actions and monitoring performance. This ensures that the LLMs operate optimally and continuously enhance performance through feedback loops.
- Validation: A validation layer is implemented to validate the LLM’s output. Tools such as Guardrails, Rebuff, Guidance, and LMQL are utilized for this purpose, ensuring the accuracy and reliability of the output generated by the LLMs.
- LLM APIs and hosting platforms: LLM APIs and hosting platforms are pivotal in executing financial modeling tasks and hosting the application. Developers can choose from various LLM APIs, such as those offered by OpenAI and Anthropic, or opt for open-source models. Similarly, they can select hosting platforms from major cloud providers like AWS, GCP, Azure, and Coreweave, or they can choose opinionated clouds like Databricks, Mosaic, and Anyscale. The choice of LLM APIs and cloud hosting platforms depends on the unique requirements and preferences of the project.
This structured flow offers a comprehensive overview of how AI enhances financial modeling, leveraging diverse data sources and technological tools to generate precise and actionable insights. Through automation, AI enhances efficiency, enabling a more thorough analysis of financial models and empowering decision-makers with valuable information for informed financial strategies.
Optimize Your Operations With AI Agents
Optimize your workflows with ZBrain AI agents that automate tasks and empower smarter, data-driven decisions.
Applications of AI in financial modeling
Data processing and analysis
AI significantly enhances data processing and analysis in financial modeling. It excels in handling vast volumes of financial data, such as historical records, market data, and news sentiment. Machine learning algorithms, including supervised and unsupervised learning, perform in-depth data analysis to identify trends, patterns, and anomalies. For instance, supervised learning can forecast future financial metrics based on historical data, while unsupervised learning can detect unusual patterns in financial behavior that might signal emerging risks. This comprehensive analysis improves the accuracy of financial models, leading to more informed, data-driven decision-making.
Automation of routine tasks
One of the fundamental strengths of AI lies in its ability to automate routine and time-consuming tasks that are inherent in financial modeling. These tasks include data entry, data cleansing, and report generation, among others. By automating these repetitive processes, AI not only reduces the risk of human errors in financial models but also allows finance professionals to allocate their time and expertise to more strategic aspects of financial analysis.
Predictive analytics
AI’s predictive capabilities are crucial in financial modeling, providing accurate forecasts for various financial metrics. Techniques such as time series forecasting and regression analysis enable AI to generate reliable predictions. For example, time series models can forecast future revenue, expenses, and cash flow based on historical financial data. Regression analysis can predict the impact of economic variables, such as interest rates or inflation, on company performance. These enhanced predictions facilitate more informed decision-making and strategic financial planning.
Natural Language Processing (NLP)
NLP is a key driver of innovation in finance, as highlighted by a survey conducted by Nvidia, which included over 200 finance-based firms in America and Europe. The survey revealed that natural language processing and large language models were the leading AI use cases, representing 26% of the responses. NLP technology allows AI to process and understand textual data from sources like financial news, reports, and earnings call transcripts. Techniques such as sentiment analysis and entity recognition extract valuable insights, such as market sentiment and company performance indicators. This textual analysis helps financial professionals gauge market sentiment and incorporate it into their financial models, enhancing decision-making processes.
Risk assessment and management
AI significantly enhances real-time risk assessment and management by swiftly identifying and evaluating various financial risks, including credit, market, and operational risks. AI models utilize advanced techniques such as anomaly detection and predictive modeling to continuously monitor risk factors and adapt to new data. For example, anomaly detection can identify unusual patterns in transaction data, signaling potential fraud, while predictive modeling can forecast economic downturns based on historical trends and current indicators. This dynamic approach enables proactive risk management and timely decision-making, effectively mitigating potential losses. Additionally, AI integrates seamlessly with existing risk management systems, augmenting their capabilities and improving overall efficiency. By providing actionable insights and facilitating compliance with evolving regulations, AI helps financial institutions navigate complex risk landscapes, ultimately leading to enhanced financial stability and resilience.
Scenario analysis and sensitivity analysis
AI streamlines scenario and sensitivity analysis by automating the process of testing various scenarios. AI-driven models can rapidly adjust key variables in financial models to assess potential outcomes under different conditions. This automation simplifies the evaluation of the impact of changes in specific variables on financial results, allowing for a more comprehensive analysis. For instance, AI can simulate the financial impact of extreme economic scenarios, such as a recession or a sudden market crash, providing a comprehensive analysis of potential risks and opportunities.
Fraud detection and security
AI enhances financial security through advanced fraud detection systems. These systems use machine learning algorithms, such as anomaly detection and classification, to analyze transaction data and identify suspicious patterns. For example, AI can detect unusual spending behavior that may indicate fraudulent activity, such as account takeover or credit card fraud. These systems bolster security measures and protect financial institutions and their clients from fraud.
Compliance and regulatory reporting
AI-driven automation plays a critical role in facilitating compliance with complex financial regulations and reporting requirements. By continuously updating models with the latest regulatory changes and automating compliance tasks, AI reduces the risk of violations and penalties. For instance, AI systems can automatically generate reports that adhere to new regulatory standards, ensuring that reports are accurate and timely. AI also helps financial institutions stay up-to-date with evolving regulations, such as SOX, or Basel III, by integrating these changes into compliance models and processes. This automation not only enhances accuracy but also improves efficiency, allowing institutions to handle large volumes of data with fewer errors. Additionally, AI provides detailed audit trails, improving transparency and accountability in financial reporting. Overall, AI-driven solutions support more effective compliance management and help financial institutions navigate complex regulatory situations.
Personalized financial advice
AI-powered robo-advisors offer personalized investment advice based on individual goals and risk profiles. These systems use algorithms to analyze client data and provide tailored recommendations, such as asset allocation strategies and investment choices. This personalization makes high-quality financial advice more accessible to a broader range of investors compared to traditional wealth management services.
Valuation
AI enhances valuation techniques like Discounted Cash Flow (DCF) analysis, Comparable Company Analysis (CCA), and precedent transactions analysis. By integrating alternative data sources and real-time market information, AI improves the accuracy and speed of valuation processes. For example, AI can adjust DCF models based on the latest market trends, providing more precise value estimates for companies and assets.
Investment analysis
AI significantly enhances investment analysis by processing and evaluating large datasets to predict potential returns and risks associated with various investment opportunities. Machine learning techniques, such as classifiers and clustering algorithms, are employed to analyze diverse data sources, including information on stocks, bonds, real estate, and startups. For example, machine learning classifiers can assess historical stock performance to identify patterns indicative of future returns, while clustering algorithms can group similar real estate properties to uncover emerging market trends. AI-driven analysis supports investors by providing more accurate predictions and identifying promising opportunities, thereby facilitating more informed and timely investment decisions. Additionally, AI integrates with existing investment analysis tools, offering enhanced capabilities and insights. The use of AI in investment analysis can lead to improved decision-making accuracy, optimized portfolio management, and a more comprehensive understanding of market dynamics. However, it is important to address challenges such as data quality and the need for continuous model updates to ensure reliable outcomes.
Mergers and acquisitions (M&A)
In M&A transactions, AI assists by evaluating financial implications, determining fair purchase prices, and assessing potential synergies. AI models can analyze historical M&A data and simulate integration scenarios to support more accurate deal-making. For example, AI can identify potential cost savings and revenue enhancements from proposed mergers or acquisitions.
Budgeting and forecasting
AI can enhance the accuracy of budgeting and forecasting by analyzing historical data, market trends, and other pertinent variables, thereby generating more precise predictions. AI can identify patterns and predict future financial performance more precisely, allowing for better revenue, expenses, and cash flow planning. This improved accuracy helps companies allocate resources more effectively, anticipate financial needs, and proactively adjust strategies.
Loan and credit analysis
AI-driven financial models enable financial institutions to assess the creditworthiness of borrowers with greater accuracy by analyzing extensive datasets, including credit scores, transaction histories, and financial behaviors. These models identify patterns and anomalies that traditional methods might overlook, creating more detailed risk profiles. This enhanced precision allows lenders to effectively tailor loan terms and credit lines, reducing default rates and optimizing credit portfolios. Moreover, AI streamlines the approval process, making it faster and more efficient for lenders and borrowers, improving overall financial decision-making and customer experience.
Financial reporting
AI-powered financial modeling streamlines the preparation of financial statements by automating data collection, processing, and analysis. This automation reduces manual entry, minimizes errors, and ensures consistency in financial data. AI also continuously monitors and updates financial reporting standards to maintain regulatory compliance. Additionally, AI enhances shareholder reporting by generating timely, detailed insights and facilitating transparent and informed communication with stakeholders. This improves overall efficiency and accuracy in financial reporting processes.
Tax planning
Companies use AI-driven financial modeling to analyze the tax implications of various business decisions, enabling efficient and strategic tax planning. AI’s advanced predictive capabilities provide precise forecasts of tax liabilities under different scenarios, helping businesses optimize their tax strategies. Additionally, AI ensures compliance by continuously updating models with the latest tax regulations and identifying potential tax-saving opportunities. This helps companies to make informed decisions that minimize tax burdens while adhering to legal requirements.
Pension fund management
AI enhances pension fund management using accurate and dynamic financial modeling to ensure sufficient funds are available to meet future liabilities. AI-driven models can predict future cash flows, investment returns, and demographic changes, allowing for more precise forecasting and strategic planning. By continuously analyzing market conditions and adjusting investment strategies, AI helps optimize asset allocation and risk management. This ensures the pension fund remains adequately funded and can meet its long-term obligations to beneficiaries.
Strategic planning and analysis
AI is crucial in long-term strategic planning by evaluating potential growth pathways and market expansion opportunities. Through comprehensive data analysis and machine learning algorithms, AI can identify trends, patterns, and correlations in large datasets that human analysts might overlook. This enables businesses to make more informed decisions about resource allocation, product development, and market positioning. By leveraging AI-powered predictive analytics, companies gain a competitive edge in anticipating market changes and adapting strategies for sustained growth and success.
How does AI for financial modeling benefit the private equity and investment sector?
Improved investment decisions
AI-powered predictive analytics can accurately estimate changes in stock prices, market activity. Investors may make more intelligent and strategic decisions by using these tools, which can quickly spot trends, patterns, and untapped possibilities. Better investing decisions may result in greater returns, lower risks, and a stronger position in the market by supplementing human judgment with data-driven insights.
Efficient due diligence
One distinguishing characteristic of AI integration in the investing sector is efficiency in the procedures of due diligence. AI speeds up the assessment of possible investments by automating the collection and analysis of financial data, company performance metrics, and regulatory compliance. In addition to saving a lot of time, this automation improves accuracy by lowering the possibility of human error. Additionally, AI is able to continuously monitor news, social media, and other outside influences, offering important updates that might influence investment choices. As a result, effective due diligence enables investors to decide quickly and with the best use of available resources.
Portfolio optimization
Financial models powered by AI take into consideration a wide range of important variables, such as investor risk tolerance, particular investment goals, and market circumstances. Investors may reach a degree of asset allocation precision that was previously unachievable by utilizing the enormous computational power and data analytic capabilities of AI. As a consequence, portfolios are not only tailored to each investor’s specific preferences and aims but also perfectly calibrated to respond to shifting market dynamics. Because of the consequently increased potential for greater returns and risk avoidance, portfolio optimization using AI has become an essential tool for investors looking to optimize their financial strategy in a dynamic and cutthroat investing environment.
Enhanced deal sourcing and origination
AI is transforming deal sourcing and origination by helping investors quickly identify promising investment opportunities. AI-powered market screening analyzes vast datasets of potential investment targets, identifying companies with specific characteristics that align with the investor’s criteria. AI can also predict future investment opportunities based on historical deal data and market trends, helping investors anticipate emerging sectors and identify companies likely to perform well. Network analysis can map out connections between companies, individuals, and investors to identify potential deal partners and uncover hidden opportunities.
Behavioral analysis
AI algorithms discover novel insights from market sentiments and investor behavior by closely examining investor sentiment, keeping track of news trends, and filtering through social media data. Making intelligent investing decisions is made much easier with this data-driven understanding. Investors can proactively modify their strategy by gauging the current market mood and spotting new trends or worries. This helps them to take advantage of possibilities or avoid potential dangers in addition to facilitating prompt actions. In the end, behavioral analysis with AI gives investors a potent tool to gauge the market mood in real-time, improving their capacity to make flexible and knowledgeable investment decisions in today’s quick-paced and data-rich financial scene.
Benefits of AI in financial modeling
AI offers several benefits in financial modeling that enhance accuracy, efficiency, and decision-making:
- Improved accuracy: AI algorithms can process large volumes of data and identify patterns with a high degree of precision, leading to more accurate financial forecasts and risk assessments. By reducing human errors and incorporating real-time data, AI helps in generating reliable predictions.
- Enhanced efficiency: AI can automate repetitive tasks such as data collection, data cleaning, and model updating. This automation reduces the time and effort required for financial modeling, allowing professionals to focus on strategic analysis and decision-making.
- Advanced predictive analytics: AI-powered models can analyze historical data and current market conditions to predict future trends more effectively. Machine learning algorithms can uncover hidden patterns and correlations that traditional models might miss, providing deeper insights into market dynamics.
- Real-time analysis: AI enables real-time data processing and analysis, allowing for timely updates to financial models. This is particularly valuable in dynamic markets where timely information is crucial for making informed decisions.
- Risk management: AI enhances risk assessment by continuously learning from new data and adapting to changing market conditions. This helps in identifying potential risks earlier and more accurately, allowing for better risk mitigation strategies.
- Personalized insights: AI can tailor financial models to specific needs and scenarios, offering customized insights and recommendations based on individual business requirements or investment goals.
- Scalability: AI systems can handle vast amounts of data and complex calculations more efficiently than traditional methods. This scalability is crucial for large-scale financial modeling projects and allows for more comprehensive analysis.
- Enhanced decision-making: By providing more accurate and timely insights, AI supports better decision-making in finance. It helps in evaluating investment opportunities, valuing assets, and developing strategic plans with greater confidence.
- Cost savings: Automation and improved efficiency reduce the costs associated with manual data handling and model development. AI can also minimize the need for extensive manual oversight and intervention.
- Competitive advantage: Leveraging AI in financial modeling can provide a competitive edge by enabling quicker and more informed decision-making, improving financial performance, and identifying new opportunities for growth.
How to implement AI in financial modeling?
Implementing AI in financial modeling involves several key steps:
- Define objectives: Define the goals you aim to achieve with AI in financial modeling. Whether it’s optimizing investment strategies, risk management, or improving forecasting accuracy, having clear objectives will guide your implementation process.
- Data collection and preprocessing: Gather relevant financial data from various sources such as market data, financial statements, and alternative data sources. Preprocess the data to ensure consistency, accuracy, and compatibility for AI algorithms.
- Feature engineering: This stage, known as feature engineering, involves selecting, transforming, and creating features from raw data to enhance the AI model’s performance. Feature engineering is critical for capturing relevant information and patterns in the data that can enhance the predictive power of the models.
- Model selection: Choose appropriate AI models based on your objectives and the nature of financial data. Commonly used AI techniques in financial modeling include machine learning algorithms like regression, decision trees, neural networks, and natural language processing (NLP) for textual data analysis.
- Model training: Train your chosen AI models using historical financial data. This involves feeding the model with labeled data (supervised learning) or allowing the model to identify patterns in the data.
- Validation and testing: Validate the trained models using validation datasets to ensure their accuracy, reliability, and generalization capabilities. Test the models with unseen data to evaluate their performance and fine-tune them as necessary.
- Integration with workflow: Integrate the AI models into your financial modeling workflow. This may involve developing APIs or integrating AI algorithms into financial software platforms.
- Monitoring and maintenance: Monitor the performance of AI models in real-world financial scenarios. Regularly update and retrain the models with the latest data to ensure they remain relevant and effective.
- Regulatory compliance and risk management: Ensure compliance with regulatory requirements and address potential risks associated with AI implementation in financial modeling, such as data privacy, model interpretability, and algorithmic bias.
- Feedback and iteration: Gather feedback from users and stakeholders to identify areas for improvement and iterate on the AI models accordingly. Continuous improvement is essential for refining models and enhancing their effectiveness in financial decision-making.
- Ethical considerations: Consider the ethical implications of AI implementation in financial modeling, such as transparency, fairness, and accountability. Implement appropriate measures to mitigate biases and ensure the ethical use of AI in financial decision-making.
LeewayHertz’s AI development services for financial modeling
At LeewayHertz, we specialize in developing custom AI solutions tailored to the intricate demands of financial modeling. Our strategic AI/ML consulting empowers financial institutions to leverage cutting-edge technology for refined decision-making, streamlined processes, and optimized investment strategies.
We excel in developing Proof of Concepts (PoCs) and Minimum Viable Products (MVPs) that offer a glimpse into the potential impacts of AI tools in real-world financial modeling scenarios. This ensures that our solutions are not only effective but also perfectly aligned with the intricate needs of the financial modeling landscape.
Our expertise extends to generative AI, transforming routine tasks such as report generation and data management. By automating these processes, we liberate financial analysts to focus on more strategic endeavors, enhancing overall productivity and efficiency.
By fine-tuning large language models on financial lexicons and communication nuances, we elevate the accuracy and relevance of AI-driven analyses and communications within financial modeling frameworks.
Moreover, we specialize in seamlessly integrating these AI systems into existing technological infrastructures, optimizing operational efficiency and decision-making processes across financial modeling endeavors.
Our AI solutions development expertise
AI solutions development for financial modeling typically involves creating systems that enhance decision-making, automate complex tasks, and provide personalized insights. These solutions integrate key components such as data aggregation technologies, which compile and analyze financial data from diverse sources, including market trends, company financial statements, and industry reports. This comprehensive data foundation supports predictive analytics capabilities, allowing for the forecasting of trends, market movements, and financial performance that inform strategic decisions. Additionally, machine learning algorithms are employed to simulate various scenarios by adjusting input variables and constraints, enabling the exploration of different possible outcomes and the optimization of financial models based on specific objectives or risk tolerance levels. These solutions often cover areas like valuation modeling, forecasting, risk analysis, portfolio optimization, and financial planning.
Overall, AI solutions in financial modeling aim to enhance the accuracy and reliability of financial projections, facilitate informed decision-making, and drive better financial outcomes.
AI agent/copilot development for financial modeling
At LeewayHertz, we specialize in constructing AI agents and co-pilots designed to elevate diverse financial modeling operations. Our tailored solutions empower companies to conserve valuable time and resources, expediting decision-making processes. Here’s how they contribute:
Data acquisition and preparation:
- Extract relevant data from websites, databases, and financial news sources, eliminating manual data entry and ensuring real-time data updates.
- Identify and correct data inconsistencies, errors, and missing values, ensuring data quality and accuracy for analysis.
- Perform complex data transformations, such as converting currencies, adjusting for inflation, and creating derived variables, simplifying data preparation for modeling.
Reporting and communication:
- Create dynamic dashboards presenting real-time complex financial data, enabling stakeholders to make informed decisions through interactive insights and clear visualizations.
- Generate comprehensive financial reports, including key performance indicators, risk assessments, and investment recommendations, saving time and effort.
- Translate complex financial data and analysis into clear, concise reports, making them accessible to a wider audience.
Scenario analysis and risk management:
- Generate multiple financial scenarios based on various assumptions, allowing analysts to assess potential outcomes and assess risk under different market conditions.
- Identify key variables impacting model results, enabling analysts to understand the drivers of financial performance and assess the impact of uncertainties.
- Analyze historical data and market trends to identify potential risks and generate mitigation strategies, improving financial planning and portfolio management.
Portfolio management and optimization:
- Identify optimal asset allocations based on investor risk profiles and financial goals, optimizing portfolio performance.
- Provide tailored investment recommendations based on individual financial situations and goals, enhancing personalized financial planning.
Regulatory compliance and audit:
- Analyze financial data and identify potential compliance violations, ensuring adherence to regulatory requirements.
- Analyze transaction data and identify potential fraudulent activities, mitigating risk and reducing financial losses.
- Automate repetitive audit tasks, such as data extraction and reconciliation, freeing up auditors for more complex analysis.
AI agents and copilots not only enhance the efficiency of operational processes but also notably elevate the quality of customer service and strategic decision-making in financial modeling. By integrating LeewayHertz’s advanced AI agents into their existing infrastructure, companies can gain a substantial competitive edge, navigating the intricate financial landscape with innovative, efficient, and dependable AI-driven tools and strategies.
Optimize Your Operations With AI Agents
Optimize your workflows with ZBrain AI agents that automate tasks and empower smarter, data-driven decisions.
Ethical considerations for implementing AI in financial modeling
Implementing AI in financial modeling introduces numerous benefits but also raises significant ethical considerations that must be addressed to ensure responsible use and maintain stakeholder trust.
Bias and fairness: AI systems can unintentionally amplify biases present in the data they are trained on. For example, if a model is trained on data that reflects historical inequalities, it may continue to make biased predictions, affecting the fairness of financial decisions. To mitigate this risk, it is crucial to regularly audit AI models for bias, use diverse datasets, and implement fairness-aware algorithms to ensure equitable outcomes across different demographic groups.
Transparency: Transparency in AI models is essential for building trust and accountability. Stakeholders need to understand how decisions are made, especially in high-stakes areas like financial modeling. Developing explainable AI (XAI) techniques, which provide clear insights into how models arrive at their conclusions, can help demystify AI processes and facilitate better decision-making. Ensuring that AI systems are transparent can also help in meeting regulatory requirements and addressing stakeholder concerns.
Data privacy: Financial data is highly sensitive, and protecting this information is paramount. AI systems must comply with data protection regulations such as GDPR (General Data Protection Regulation) and CCPA (California Consumer Privacy Act). Implementing robust data encryption, access controls, and anonymization techniques can safeguard personal and financial information from unauthorized access or breaches. Ensuring data privacy helps maintain user trust and compliance with legal standards.
Regulatory compliance: AI in financial modeling must adhere to existing financial regulations and standards. Non-compliance can result in legal consequences and damage to an organization’s reputation. It is important to stay updated with regulatory changes and ensure that AI systems are designed to meet these requirements. Engaging with legal experts and regulatory bodies can help ensure that AI implementations align with current regulations and best practices.
Security risks: AI systems are susceptible to security threats, including cyber-attacks and misuse. Protecting AI systems from such risks is crucial to maintaining their integrity and reliability. Implementing strong security measures, such as regular security assessments, intrusion detection systems, and incident response plans, can help safeguard AI systems from potential threats. Additionally, ensuring ethical use of AI includes preventing its misuse for financial manipulation or fraudulent activities.
Ethical use of AI: The ethical use of AI in financial modeling involves aligning AI applications with societal values and ethical standards. Organizations should establish clear ethical guidelines for AI usage, promote responsible AI development, and engage in open discussions about the ethical implications of their AI strategies. Encouraging ethical practices helps ensure that AI contributes positively to society and avoids harmful consequences.
Addressing these ethical considerations is essential for the responsible implementation of AI in financial modeling. By focusing on bias mitigation, transparency, data privacy, regulatory compliance, employment impacts, security, and ethical use, organizations can harness the benefits of AI while upholding high ethical standards and maintaining stakeholder trust.
Future trends and innovations in AI for financial modeling
The landscape of financial modeling is rapidly evolving with advancements in AI technology, promising transformative changes and new possibilities. As AI continues to develop, several future trends and innovations are expected to shape the field:
Explainable AI (XAI): While AI models can produce impressive results, understanding why they make certain predictions is crucial for building trust and transparency. XAI aims to make AI models more interpretable and explainable, allowing investors to understand the reasoning behind AI-driven decisions and better assess the reliability of the models. This will be particularly important in a regulated industry where accountability is paramount.
Focus on responsible AI: As AI becomes more powerful in financial modeling, a growing emphasis on responsible AI is crucial. This involves developing AI models that are fair and unbiased, especially in areas like loan approvals and risk assessment, to mitigate potential discrimination. Furthermore, robust data privacy and security measures must be implemented to protect sensitive financial data and ensure its ethical use. Finally, maintaining human control and oversight of AI systems is essential to prevent unintended consequences and ensure that these powerful tools are used for the benefit of all stakeholders.
Endnote
The integration of AI into financial modeling represents a transformative leap forward in the domain of finance. AI tools for financial modeling have redefined the boundaries of what’s possible, offering professionals in finance and investment a powerful set of capabilities for data analysis, predictive modeling, risk management, and decision-making. These AI-powered tools and techniques enhance the accuracy and efficiency of financial forecasts, enabling more informed strategic decisions.
AI will play a bigger part in financial modeling as it develops and adapts to the changing financial scene. The advantages of AI, such as improved data processing, regular operations being automated, and personalized insights, enable finance professionals to better manage the complexity of the current financial world.
Looking to leverage AI’s potential in financial modeling? Contact LeewayHertz’s experienced AI developers today for tailored solutions that elevate your financial strategies.
Start a conversation by filling the form
All information will be kept confidential.
Frequently Asked Questions
How does AI enhance financial modeling?
AI enhances financial modeling by processing vast amounts of data rapidly, identifying complex patterns and correlations, and generating more accurate predictions for investment opportunities, risk assessment, and portfolio management.
Can AI be used for risk management in financial modeling?
Yes, AI techniques such as machine learning and deep learning are extensively used for risk management in financial modeling. These technologies help in identifying and assessing different types of risks, including market, credit, operational, and compliance risks, thereby enabling financial institutions to mitigate potential losses and optimize their risk-adjusted returns.
How does AI-powered financial modeling impact investment decisions?
AI-powered financial modeling equips investors with advanced, data-driven insights, significantly enhancing their decision-making capabilities. By integrating AI, investors can rapidly identify lucrative opportunities, respond to market changes, optimize their asset allocations based on predictive analytics, and manage risks more precisely. This leads to more strategic, informed, and timely investment decisions, potentially increasing returns while mitigating risks.
Which types of financial models are most commonly enhanced using AI?
Some examples include credit scoring models for lending institutions, stock price prediction models used by investment firms, and fraud detection systems employed by banks and other financial organizations.
How can LeewayHertz assist with AI in financial modeling?
LeewayHertz offers expertise in developing AI-driven financial modeling solutions customized to the specific needs of financial institutions, investment firms, and fintech startups. Our team specializes in building predictive models, risk management tools, and automated investment platforms using advanced AI techniques.
What sets LeewayHertz apart as an AI partner for financial modeling?
LeewayHertz distinguishes itself as a leading AI consulting and development company with a proven track record of delivering innovative solutions for financial modeling. Our team of data scientists, AI engineers, and domain experts collaborates closely with clients to understand their business objectives, develop robust AI models, and deploy scalable solutions that drive tangible business outcomes.
How does LeewayHertz ensure data security and compliance in AI-driven financial modeling projects?
LeewayHertz prioritizes data security and compliance with industry regulations in all AI-driven financial modeling projects. We implement robust encryption protocols, access controls, and compliance measures to safeguard sensitive financial data and ensure regulatory compliance throughout the project lifecycle.
What is the process for initiating a financial modeling project with LeewayHertz?
To begin a financial modeling project with LeewayHertz, clients can schedule a consultation with our team to discuss their requirements, objectives, and vision for the project. Our experts will then conduct a thorough analysis of the data and develop a customized AI solution tailored to the client’s specific needs and objectives.
Can LeewayHertz provide ongoing support and maintenance for AI-powered financial modeling solutions?
To begin a financial modeling project with LeewayHertz, clients can schedule a consultation with our team to discuss their requirements, objectives, and vision for the project. Our experts will then conduct a thorough analysis of the data and develop a customized AI solution tailored to the client’s specific needs and objectives.