AI in customer complaint management: Use cases, benefits and solution
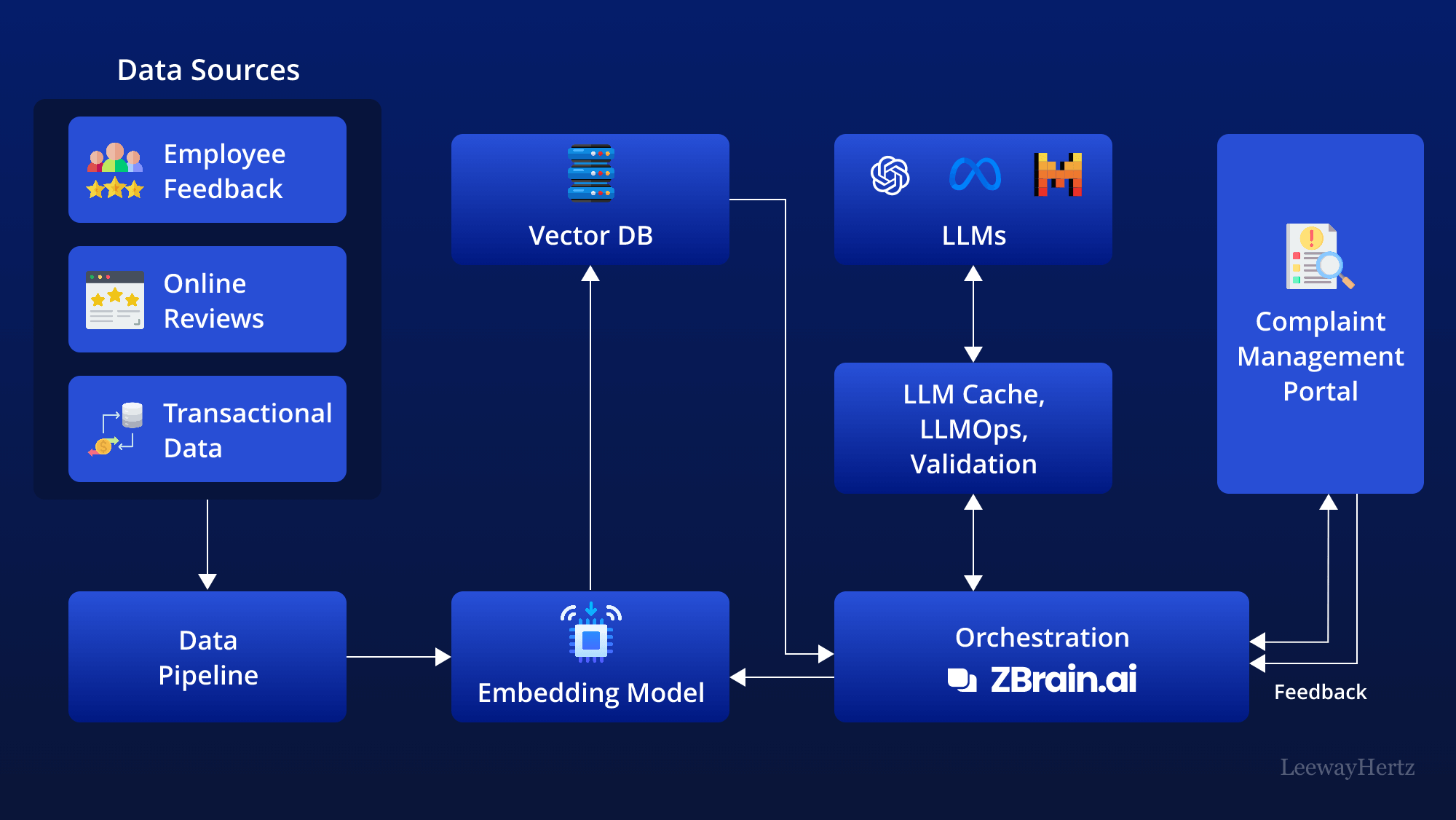
In today’s fast-paced and customer-centric business landscape, the effective management of customer complaints has evolved from being merely a necessity to a strategic imperative. As businesses strive to enhance customer satisfaction and stay ahead in fiercely competitive markets, they are turning to cutting-edge solutions. Among these, the integration of Artificial Intelligence (AI) stands out as a transformative force, redefining how organizations approach and resolve customer grievances. This integration is not just a technological upgrade; it represents a paradigm shift that empowers businesses to deliver more efficient, personalized, and ultimately, more satisfying customer complaint resolution experiences. Companies strive for market share and cultivate enduring customer trust and loyalty. This underscores the pivotal role of AI in complaint management systems. According to Digital Journal, the global market for AI complaint management is projected to demonstrate a remarkable valuation of approximately USD 4.5 billion, spanning the period from 2023 to 2030, with a compound annual growth rate (CAGR) of 23.2%.
It’s worth highlighting that conventional manual methods are proving inadequate, exposing businesses to a broad spectrum of risks encompassing regulatory penalties, reputational damage, eroding customer loyalty, and overlooked opportunities for service optimization. Within this landscape, organizations grapple with the critical task of precise complaint identification, meticulous classification, comprehensive reporting, and swift resolution. AI-powered complaint management, on the other hand, offers a multifaceted solution that transcends the limitations of manual approaches. By harnessing the capabilities of machine learning, natural language processing, and data analytics, AI empowers businesses to not only streamline complaint handling but also extract valuable insights from customer feedback. Consider a scenario in which a prominent e-commerce platform receives a high influx of customer complaints daily. Harnessing AI for customer complaint management empowers the system to discern urgent concerns swiftly, warranting immediate attention. This particularly aids in addressing grievances such as incorrect orders or missing packages, augmenting customer satisfaction and facilitating prompt issue resolution.
This article explores AI’s role in managing customer complaints, covering its specific business use cases, benefits, ethical considerations and more.
- What is customer complaint management?
- Why is complaint analysis significant within a complaint management system?
- What advantages does AI-powered complaint analysis bring to a business in managing complaints?
- Use cases of AI in complaint management system
- How can GenAI automate customer complaint management processes across sub-departments?
- Streamlining customer complaint management workflow with GenAI
- How LeewayHertz’s generative AI platform transforms customer complaint management operations
- How does AI in complaint management work?
- How do AI-powered customer complaint management systems work?
- Advantages of AI in complaint management systems
- Ensuring data privacy and ethical use of AI in complaint management
What is customer complaint management?
Customer complaint management refers to the systematic process of handling and addressing customer complaints and concerns in a way that maintains their satisfaction and loyalty. It involves identifying complaints, recording details, categorizing and prioritizing issues, responding empathetically, investigating root causes, providing resolutions, and ensuring follow-up.
Analyzing customer complaints empowers businesses to leverage data from diverse online sources to gain insights into customers’ preferences and criticisms regarding their offerings. This information can complement data obtained from internal channels like customer service interactions and surveys.
Why is complaint analysis significant within a complaint management system?
Complaint analysis is a critical component within a complaint management system for several key reasons that contribute to the overall efficiency, effectiveness, and success of customer service and business operations. Here are the main reasons why complaint analysis is significant:
Root cause analysis: Complaint analysis helps in identifying the underlying causes of complaints. This allows organizations to dig deeper into the reasons behind customer dissatisfaction and take corrective actions to prevent similar issues in the future.
Improving products and services: By understanding the nature of complaints and the areas where customers are dissatisfied, organizations can make informed decisions to improve their products and services. This feedback loop is crucial for enhancing the overall customer experience.
Identifying patterns and trends: Analyzing complaints allows organizations to identify recurring patterns or trends in customer feedback. This information is invaluable for understanding common pain points and recurring issues that need to be addressed systematically.
Data-driven decision-making: Analyzing complaints provides organizations with concrete, data-driven insights. These insights can guide decision-making at various levels, from improving processes to reallocating resources effectively.
Competitive advantage: Organizations that excel in complaint analysis gain a competitive advantage. They can quickly adapt to changing customer needs, stand out in the market for their commitment to resolving issues, and build a positive brand image that attracts and retains customers.
Complaint analysis is fundamental for driving proactive improvements, resolving issues efficiently, enhancing customer satisfaction, and fostering a customer-centric culture within an organization. It empowers organizations to learn from customer feedback and implement changes that contribute to long-term business success and customer loyalty.
What advantages does AI-powered complaint analysis bring to a business in managing complaints?
Highlights problems with your product or service
Incorporating AI into customer complaint management unveils potential shortcomings within your product or service. Complaints often extend beyond direct communication with your company, frequently manifesting as comments or reviews on various platforms like social media or review pages. These insights emerge from user discussions about your offering, providing unique perspectives that might not surface through traditional customer service channels alone. Dissatisfied users tend to voice their concerns on social media platforms, supplementing or even bypassing direct support interactions. By scrutinizing customers’ online grievances, you can pinpoint various issues, regardless of their magnitude, encountered by users while utilizing your product. This analysis and direct customer feedback offer a comprehensive panorama of prevailing customer challenges.
Facilitates quick complaint handling
An instrumental advantage of AI-enabled customer complaint analysis lies in its agility. Customers seek rapid solutions to their problems, and swift action is pivotal. The AI-driven analysis automatically aggregates, assesses, and categorizes customer complaints, promptly directing them to the appropriate team. For instance, if an issue pertains to your product’s functionality, it reaches the product development team; if dissatisfaction centers on customer service, the concern is routed to the dedicated customer service team. This streamlined resolution process significantly boosts customer retention rates by allowing proactive issue resolution. Effortlessly accessible support options contribute to maintaining customer satisfaction, ensuring their needs are promptly met.
Improves customer experience
The integration of AI in complaint management contributes to enhancing the overall customer experience. The insights derived through complaint analysis illuminate areas for enhancement and future growth possibilities. The scope of customer complaint analysis transcends immediate issue resolution, encompassing the identification of trends and opportunities for enriching the holistic customer journey. By harnessing AI-driven insights, you gain a competitive edge in addressing existing concerns and elevating the entire customer experience.
Optimize Your Operations With AI Agents
Optimize your workflows with ZBrain AI agents that automate tasks and empower smarter, data-driven decisions.
Use cases of AI in complaint management
Voice analysis
AI in customer complaint management systems involves voice analysis, which deciphers emotional tones in conversations between service representatives and customers. AI technology triggers alerts for agents regarding optimal moments to infuse positivity, escalate a grievance to a manager, or address a customer experiencing high emotional intensity. By aiding agents in adapting their tone to match the emotional context of the customer, this approach substantially heightens the likelihood of a favorable resolution.
One such example is IBM Watson’s Tone Analyzer, which employs linguistic analysis to identify emotional and language tones within written text. The technology scrutinizes audio input for one of seven distinct tones: sadness, frustration, satisfaction, excitement, sympathy, politeness, and impoliteness. Subsequently, the algorithm predicts the speaker’s emotional state based on the presence of tones and enhances communication.
Self-service chatbots
As customers remain perpetually connected to the internet, there’s a growing preference for rapid, self-serve customer service alternatives. While certain individuals refer to FAQ pages or external forums, others seek efficient solutions devoid of human involvement. This is where AI-powered chatbots come into play.
An AI chatbot strikes a harmonious balance, catering to younger customer demographics’ preferences while streamlining time and cost expenditures associated with customer service. Many companies present chatbots as an initial resolution avenue for managing customer complaints, allowing users to engage with a human representative if necessary during the interaction. This approach serves the dual purpose of meeting the needs of those seeking automated assistance and those with more intricate issues who opt to escalate their concerns to a human agent.
Automated responses
Automated responses driven by AI, particularly through chatbot technology, offer an efficient and timely solution for addressing common and straightforward customer complaints. These AI-powered chatbots are adept at swiftly identifying the nature of the complaint and generating instant responses that provide solutions to frequently encountered issues. They offer resolutions and excel at addressing frequently asked questions with accurate information, offering customers a rapid way to find answers.
Furthermore, these chatbots can direct customers to relevant resources such as knowledge bases, tutorials, or self-help guides. Chatbots streamline the complaint resolution process by quickly disseminating information and guiding users toward self-service options, enabling human agents to concentrate on intricate matters demanding personalized attention.
The benefits of automated responses are twofold: customers receive immediate assistance, improving their overall experience, while businesses benefit from enhanced operational efficiency and reduced workload for human agents.
Real-time monitoring
Through AI-powered real-time monitoring, businesses can promptly detect and address complaints and mentions across various online platforms, including social media and review sites. By scrutinizing website or app data, AI systems can pinpoint “distress indicators,” effectively spotting customers facing problems and discerning the nature of those issues. By instantly detecting these interactions and analyzing sentiment, companies can take immediate action to address concerns, prevent potential reputation damage, and demonstrate their commitment to customer satisfaction.
The proactive resolution of customer service matters not only ensures customer satisfaction but also optimizes agent efficiency. Additionally, this approach could potentially mitigate abandonment rates, as customers won’t be confronted with unresolved queries that might prompt them to opt for a more straightforward purchase elsewhere.
Performance metrics
AI analytics play a pivotal role in enhancing customer complaint management by providing comprehensive insights into various performance metrics. These metrics encompass crucial aspects such as the time it takes to address and resolve customer complaints, gauging the satisfaction levels of customers based on the resolutions provided, and evaluating the overall effectiveness of the complaint management process.
By closely monitoring complaint resolution times, AI analytics can pinpoint bottlenecks or delays in the workflow, allowing organizations to optimize their processes and ensure swift responses. AI-driven performance metrics provide organizations with actionable insights that guide ongoing improvements in their complaint management strategies. By leveraging this data, companies can enhance their customer service, streamline internal processes, and foster a culture of continuous learning and refinement. This, in turn, leads to greater customer satisfaction, enhanced business outcomes, and a stronger competitive edge in the market.
Sentiment analysis
AI-enabled sentiment analysis offers a valuable tool for deciphering the emotions and attitudes embedded within customer complaints. By analyzing the sentiment—whether it’s positive, negative, or neutral—businesses can gain a deeper understanding of the customer’s perception and emotional state. This analysis enables companies to promptly prioritize and address urgent and emotionally charged issues, enhancing customer satisfaction by showing empathy and responsiveness.
Moreover, sentiment analysis provides a data-driven approach to uncovering trends and patterns within customer complaints. By identifying recurring sentiments and themes, businesses can discern underlying issues that might require process improvements or systemic changes. This proactive approach ensures that immediate concerns are being addressed and the root causes of negative sentiment are tackled to prevent similar complaints.
Personalized responses
AI-enabled personalized responses leverage customer data and purchase history to craft tailored solutions for individual complaints. AI can understand their unique context and needs by analyzing a customer’s past interactions, preferences, and buying behavior. When a complaint is received, the AI system can respond directly to the customer’s concerns and provide relevant information or solutions.
This level of personalization enhances the customer experience by showing that the company values and understands their individual situation and significantly increases the likelihood of resolving the issue in a single interaction. Customers feel heard and appreciated when their specific circumstances are acknowledged, leading to higher satisfaction levels and improved brand loyalty. AI’s ability to swiftly process and apply personalized data ensures that each interaction is efficient, effective, and tailored to the customer’s requirements, ultimately fostering stronger customer relationships and a positive reputation for the company.
Language translation
AI-powered language translation significantly aids in overcoming language barriers, fostering seamless communication between businesses and global customers. This technology enables seamless interactions for global companies catering to a diverse clientele regardless of language differences.
When a customer submits a complaint in their native language, AI-driven translation algorithms swiftly and accurately translate the content into the company’s preferred language. This allows customer service teams to understand the complaint’s details and nuances, ensuring that the response provided is accurate and relevant. AI can translate the company’s response into the customer’s language, creating a smooth and meaningful dialogue. By eliminating language obstacles, AI-driven language translation ensures that international customers receive the same level of attention and support as those who communicate in the company’s primary language.
Escalation management
AI-driven escalation management streamlines the process of identifying and addressing complex or sensitive customer complaints. When a complaint is received, AI algorithms analyze the content, sentiment, and contextual information to determine its complexity and severity. If the complaint surpasses a predefined threshold of complexity, the AI system recognizes the need for human intervention and escalates it to the appropriate skilled professionals within the organization.
By automating this process, businesses ensure that intricate issues receive the attention they require from experienced personnel, minimizing the risk of mishandling and ensuring effective resolutions. This enhances customer satisfaction and optimizes resource allocation by directing complex cases to specialists who are best equipped to address them. Ultimately, AI-driven escalation management enhances the quality of customer interactions, reinforces the company’s reputation for effective problem-solving, and contributes to the overall success of the complaint management process.
Feedback analysis
AI-powered feedback analysis involves the systematic examination of customer feedback and complaints to extract valuable insights that drive improvements across various aspects of a business. AI algorithms analyze large volumes of feedback, identifying recurring themes, sentiment trends, and specific pain points that customers are encountering. These insights serve as a goldmine of information that can guide crucial decisions.
By dissecting customer feedback, businesses can deeply understand their customers’ needs, preferences, and pain points. This data can influence product development by highlighting features that need enhancement or identifying new opportunities. Service improvements can be informed by pinpointing recurring issues and addressing them proactively. Additionally, these insights can guide broader business strategies, helping companies align their offerings with customer expectations and market trends.
Automated case updates
Automated case updates, powered by AI, offer a seamless and proactive way to keep customers informed about the progress of their complaints. Once a complaint is lodged, AI systems can track its status and automatically generate updates that reflect real-time developments. These updates are then sent to customers via their preferred communication channels, providing them with timely and accurate information without requiring them to reach out for updates.
This automated communication keeps customers engaged and reassured throughout the resolution process and enhances transparency and customer satisfaction. Customers appreciate being kept in the loop, as it demonstrates the company’s commitment to resolving their concerns. Furthermore, by reducing the need for customers to inquire about their case status, AI-facilitated updates free customer service agents, enabling them to tackle more intricate responsibilities.
Automated ticket classification
AI-driven automated ticket classification involves using advanced algorithms to analyze the content of incoming customer complaints and categorize them based on their nature and severity. AI can accurately determine which department or team should handle each issue by understanding the underlying themes and context within the complaints. This streamlined process ensures that complaints are directed to the right specialists who possess the expertise to address them effectively.
As a result, response times are significantly reduced since complaints no longer need to go through multiple levels of manual sorting. This swift and accurate allocation of complaints enhances the overall efficiency of the customer complaint management system. Moreover, by directing each issue to the most appropriate team, the chances of resolutions being achieved in a single interaction are heightened, which in turn boosts customer satisfaction levels. AI-driven ticket classification optimizes the complaint-routing process, expedites problem-solving, and enhances the overall customer experience.
Predictive analytics
AI-enabled predictive analytics involves analyzing historical data and user behavior patterns to anticipate potential customer complaints before they escalate. By identifying trends, patterns, and early warning signs, AI systems can provide businesses with insights that enable them to take proactive measures to address issues and prevent customer frustrations.
For instance, AI can analyze data to identify common triggers or patterns that lead to complaints, such as product defects, service interruptions, or communication breakdowns. By detecting these patterns in real-time, businesses can implement corrective actions, allocate resources strategically, and enhance processes to avert the recurrence of similar issues in the future. This predictive approach reduces the occurrence of complaints and demonstrates a proactive commitment to customer satisfaction. Ultimately, AI-powered predictive analytics empowers businesses to be ahead of the curve, addressing potential problems before they escalate into major complaints.
Optimize Your Operations With AI Agents
Optimize your workflows with ZBrain AI agents that automate tasks and empower smarter, data-driven decisions.
How can GenAI automate customer complaint management processes across sub-departments?
This section outlines the sub-departments within customer complaint management and the associated processes that GenAI can transform. GenAI greatly improves efficiency, enhances decision-making, and boosts customer satisfaction across complaint-resolution efforts by harnessing automation and sophisticated data analytics.
Customer service department
Handles customer inquiries and resolves complaints through multiple communication channels.
Sub-processes and GenAI automation:
- Automated customer interaction: GenAI-powered chatbots manage initial complaint intake, providing instant responses and routing complex issues to agents.
- Sentiment analysis: Analyzes customer emotions in real-time to prioritize complaints requiring urgent attention.
- Issue classification: Automatically categorizes complaints by type and severity, reducing manual sorting.
- Personalized response generation: Uses customer data and complaint history to craft tailored responses, enhancing customer satisfaction.
Quality assurance department
Ensures service standards and addresses performance issues in complaint resolution.
Sub-processes and GenAI automation:
- Service quality monitoring: GenAI reviews customer-agent interactions and scores them based on quality metrics like empathy and resolution accuracy.
- Feedback analysis: Automatically processes customer surveys and reviews to identify common issues and improvement areas.
- Real-time coaching: Provides live guidance to agents during customer interactions, helping improve complaint handling.
- Comprehensive reporting: Generates detailed reports on quality metrics and agent performance, providing insights for management on areas needing attention.
- Analysis of escalations: Analyzes escalated complaints to identify recurring issues and training needs, allowing for targeted improvements.
Operations department
Manages logistics and processes, ensuring efficient complaint resolution.
Sub-processes and GenAI automation:
- Root cause analysis: GenAI detects patterns in operational failures and suggests process adjustments to minimize complaints.
- Workload optimization: Allocates resources dynamically based on real-time complaint volume forecasts, ensuring quicker resolutions.
- Automated escalation management: Automatically routes unresolved complaints to appropriate teams for faster resolution.
- Process performance tracking: Monitors key performance indicators (KPIs) related to complaint resolution, providing insights for continuous improvement.
- Workflow automation: Streamlines complaint resolution processes by automating repetitive tasks, allowing team members to focus on more complex issues.
Technical support department
Provides technical expertise for resolving product-related complaints.
Sub-processes and GenAI automation:
- Automated troubleshooting: GenAI diagnoses common product issues using knowledge bases and offers instant solutions.
- Predictive maintenance alerts: Notifies technical teams of potential product failures based on customer reports and usage data.
- Tech support ticketing: Automatically generates and prioritizes technical support tickets based on the complexity and urgency of the issue.
- Virtual support assistants: Deploys chatbots and virtual agents to assist customers in real-time, guiding them through troubleshooting steps.
Product management department
Uses customer feedback and complaints to improve product design and functionality.
Sub-processes and GenAI automation:
- Complaint-driven product enhancement: Analyzes complaint data to identify design flaws or product inefficiencies and recommends improvements.
- Customer-driven feature suggestion: GenAI identifies common feature requests or pain points, informing future product updates.
- Trend analysis: Predicts emerging product trends based on recurring complaints, enabling proactive product changes.
- A/B testing optimization: Uses GenAI to analyze the performance of different product features or designs and recommend the most effective options based on customer feedback.
Data analytics department
Analyzes complaint data to uncover trends, improve processes, and predict future issues.
Sub-processes and GenAI automation:
- Complaint pattern detection: GenAI analyzes large volumes of complaint data, identifying trends and underlying causes.
- Predictive analytics: Predicts potential areas of customer dissatisfaction based on historical data and proactively suggests solutions.
- Real-time data visualization: Provides up-to-the-minute insights on complaint metrics, enabling rapid decision-making and adjustments.
- Sentiment analysis: Applies natural language processing (NLP) to gauge customer sentiment from feedback and complaints, identifying areas for improvement in customer interactions.
- Anomaly detection: Automatically identifies unusual spikes or drops in complaint volume, alerting teams to potential underlying issues or emerging trends.
- Data integration: Combines data from various sources (e.g., CRM, support tickets, social media) to provide a comprehensive view of customer feedback and complaints.
Escalation management department
Handles complaints requiring immediate attention or involving high-priority customers or critical issues.
Sub-processes and GenAI automation:
- Critical issue detection: GenAI automatically flags and prioritizes complaints based on severity or customer status.
- Escalation routing: Automatically routes high-priority cases to senior agents based on predefined escalation rules.
- Resolution timeline prediction: Uses predictive analysis to estimate the time required for resolving complex issues.
- Sentiment-based escalation: Detects customer frustration through sentiment analysis and triggers escalations accordingly.
Customer experience (CX) department
Focuses on improving the customer journey, aligning complaint management with customer satisfaction goals.
Sub-processes and GenAI automation:
- Journey mapping: GenAI analyzes customer touchpoints to identify pain points in the complaint process.
- Satisfaction score prediction: Predicts customer satisfaction levels based on interactions and past feedback.
- Personalized experience suggestions: Recommends improvements in the complaint resolution process based on customer profiles.
- Customer journey analytics: Provides insights into the most common issues affecting the overall experience and suggests improvements.
Customer retention department
Specializes in resolving complaints to retain customers, often offering compensation, discounts, or loyalty programs.
Sub-processes and GenAI automation:
- Churn prediction: GenAI identifies customers at risk of leaving based on complaint history and behavior.
- Compensation recommendation: Suggests personalized compensation or loyalty rewards to retain dissatisfied customers.
- Retention offer automation: Automates sending retention offers, such as discounts or exclusive deals, to customers who lodged complaints.
- Customer loyalty analysis: Analyzes the impact of complaint resolution on long-term customer loyalty and suggests retention strategies.
Social media and public relations department
Monitors and manages complaints on social media and public forums to prevent reputational damage.
Sub-processes and GenAI automation:
- Social sentiment monitoring: GenAI tracks social media platforms for negative sentiment related to the brand.
- Crisis response automation: Automatically generates real-time responses to complaints on public platforms to contain reputational damage.
- Public relations insights: Provides insights into public opinion trends and suggests proactive engagement strategies.
- Influencer outreach automation: Identifies key influencers discussing complaints and automates outreach for damage control.
Training and development department
Trains staff to effectively handle complaints and improve communication and conflict resolution skills.
Sub-processes and GenAI automation:
- Training material generation: GenAI creates training modules based on the latest complaint-handling techniques and best practices.
- Skill gap analysis: Identifies areas where staff need additional training by analyzing complaint resolution patterns.
- Real-time coaching: Offers live feedback and coaching to agents during complaint handling based on interaction quality.
- Performance tracking: Analyzes agent performance over time to optimize training programs.
Feedback and survey management department
Collects and analyzes feedback related to complaints, identifying trends and areas for improvement.
Sub-processes and GenAI automation:
- Automated feedback collection: GenAI automates the process of soliciting and collecting customer feedback after a complaint is resolved, using tools like triggered emails and chatbot follow-ups.
- Trend analysis: Analyzes feedback data to identify common root causes of complaints and areas needing improvement.
- Real-time sentiment analysis: Assesses customer sentiment in feedback to prioritize future actions.
- Improvement recommendation: Provides actionable suggestions for improving complaint resolution based on survey and feedback trends.
GenAI can significantly streamline complaint management processes by automating data analysis, categorization, and response generation across sub-departments. This can lead to faster resolution times and improved customer satisfaction. By leveraging real-time insights and predictive analytics, organizations can proactively address issues and enhance overall service quality.
Optimize Your Operations With AI Agents
Optimize your workflows with ZBrain AI agents that automate tasks and empower smarter, data-driven decisions.
Streamlining customer complaint management workflow with GenAI
Generative AI is no longer a futuristic concept; diverse industries and processes including customer complaint management, driving efficiency, empathy, and resolution at scale. Here’s a breakdown of how GenAI is making a significant impact across key complaint-handling processes:
- Complaint capture and logging
Steps involved | Sub-steps | Role of generative AI |
Capture complaint |
|
Auto-populates fields using existing customer data, extracts details from emails/chats. Analyzes complaint text to suggest the appropriate category. Unique case ID assignment is handled by system logic, but AI could help ensure uniqueness in complex cases. |
Validate complaint |
|
Identifies potential typos in details and suggests corrections based on existing records. Flags inconsistencies or missing information and suggests follow-up questions for the customer. |
Acknowledgement and initial response | N/A | Crafts tailored acknowledgments with customer names, complaint summaries, and accurate response timeframes. |
- Investigation and analysis
Steps involved | Sub-steps | Role of generative AI |
Case intake and assignment |
|
Auto-summarizes lengthy complaints, highlight key issues. Recommends investigators based on expertise, caseload, and availability. |
Evidence-gathering and validation |
|
Analyzes text, images, or audio/video evidence to extract relevant information and flag potential inconsistencies. Surfaces past interactions from various sources (e.g., CRM, support tickets) that might provide context to the complaint. |
Findings of documentation and reporting | Record investigation findings | Generates clear investigation reports, summarizing evidence, findings, and recommendations. |
Resolution implementation and closure | Assess complaint resolution | Analyzes post-resolution communication to gauge satisfaction and identify follow-up needs. |
- Solution and resolution
Steps involved | Sub-steps | Role of Generative AI |
Evaluate resolution |
|
Direct retrieval from the system is more efficient. Validates resolutions against policies, cases, and rules for fairness and compliance. |
Determine and calculate compensation |
|
Summarizes the complaint’s key points for quick review during compensation assessment. Surfaces policies as per the complaint type and history to ensure accurate compensation. Suggests a compensation amount based on policy guidelines, complaint severity, and customer value. |
Disbursement/Resolution actions | N/A | Primarily involves financial transactions and system updates; AI’s role would be limited. |
Case resolution and closure |
|
Analyzes data (resolution actions taken, customer communication) to automate case closure. Generates insightful reports on resolution trends and agent performance for improvement. |
- Follow-up and closure
Steps involved | Sub-steps | Role of generative AI |
Resolution finalization and communication |
|
Analyzes resolution details against predefined criteria to flag potential issues for review. Generate personalized closure notifications summarizing the resolution and next steps. |
Final approval and task completion | Approve case closure | (Human approval might be preferable here due to the sensitive nature of case closure). |
Further review assessment | Check whether further review is needed | Analyzes case details to assess the need for further review or investigation. |
Case data archival | Archive case data | Anonymizes sensitive customer data within archived cases to comply with privacy regulations. |
- Trend analysis and process improvement
Steps involved | Sub-steps | Role of generative AI |
Feedback collection and analysis |
|
Aggregates feedback from channels (surveys, reviews, social media) for a comprehensive view. Identifies key themes, sentiment trends, and common pain points from customer feedback data. |
Quality review and investigation |
|
Highlights inconsistencies, missing information, or potential red flags for quality reviewers. Analyzes complaints and customer history to assess the severity and impact of the issue. |
Resolution and improvement |
|
Predict the likelihood of a resolution being needed based on historical data and case similarities. If escalation is required, automatically create a report summarizing the issue, relevant data, and suggested actions. |
Generative AI empowers a smarter, more efficient approach to customer complaint management – driving faster resolutions, proactive improvements, and ultimately, stronger customer relationships.
How LeewayHertz’s generative AI platform transforms customer complaint management operations
LeewayHertz’s generative AI platform, ZBrain, is a crucial tool enhancing and streamlining various aspects of customer complaint management within businesses across industries. By creating custom LLM-based applications tailored to clients’ proprietary complaint data, ZBrain optimizes complaint management workflows, ensuring operational efficiency and an improved customer service experience. The platform processes diverse complaint data types, including customer feedback, support tickets, and service records, and utilizes advanced large language models like GPT-4, Vicuna, Llama 2, and GPT-NeoX to build context-aware applications that can improve decision-making, deepen insights, and boost overall productivity, all while maintaining strict data privacy standards, making it indispensable for modern customer complaint management operations.
In customer complaint management, challenges like handling diverse customer grievances, ensuring timely resolution, adapting to evolving customer expectations, managing the influx of complaints across multiple channels seamlessly, implementing personalized resolution strategies, ensuring consistent communication throughout the resolution process, and leveraging data insights for continuous improvement are prevalent. ZBrain offers a solution to these challenges through its distinctive feature called “Flow,” which provides an intuitive interface that allows users to create intricate business logic for their apps without the need for coding. Flow’s easy-to-use drag-and-drop interface enables the seamless integration of prompt templates, large language models, and other generative AI models into your app’s logic for its easy conceptualization, creation, or modification.
With sophisticated apps built on ZBrain, businesses can convert complex complaint data into actionable insights, enhancing operational efficiency, minimizing inaccuracies, and enhancing the overall quality of customer service. For an intricate overview of ZBrain’s capabilities, explore this resource showcasing a range of industry-specific Flow processes. This compilation highlights the platform’s strength and adaptability, demonstrating how ZBrain caters to various industry use cases.
Optimize Your Operations With AI Agents
Optimize your workflows with ZBrain AI agents that automate tasks and empower smarter, data-driven decisions.
How does AI in complaint management work?
Integrating AI into complaint management processes entails deploying multiple components to optimize query handling, extract valuable insights from customer feedback, and facilitate informed decision-making to address issues effectively. It transcends conventional complaint management methods by harnessing the capabilities of robust large language models (LLMs) and integrating them with an organization’s unique knowledge repository. This architecture marks a new level of insight generation, empowering businesses to promptly and efficiently handle customer complaints.
The architecture employs a range of components to streamline the complaint management process, thereby enhancing its capacity to deliver real-time solutions. Here’s a detailed breakdown of how it operates:
- Data sources: The initial step entails gathering data from various sources relevant to the complaint management process. This data may encompass:
- Direct customer feedback: This involves data collected from various direct channels where customers express their complaints, such as emails, phone calls, live chat transcripts, social media comments, and online complaint forms.
- Customer service interactions: Data from interactions with customer service representatives, including call logs, case notes, and transcripts from chat sessions, provide valuable insights into customer complaints and resolutions.
- Surveys and feedback forms: Surveys, feedback forms, and Net Promoter Score (NPS) responses can offer structured feedback about customer experiences and areas for improvement.
- Online reviews and ratings: Monitoring online review platforms, forums, and social media channels allows organizations to capture complaints and feedback shared publicly by customers.
- Transactional data: Transactional data, such as purchase history and order details, can provide context for understanding customer complaints, especially concerning product defects, shipping issues, or billing discrepancies.
- Employee feedback: Input from frontline employees who interact directly with customers can provide valuable insights into recurring complaints, customer sentiments, and areas where support or training may be needed.
- Data pipelines: The data gathered from the previous sources is subsequently channeled through data pipelines. These pipelines handle tasks such as data ingestion, cleaning, processing (including data transformations like filtering, masking, and aggregations), and structuring, thereby preparing it for subsequent analysis.
- Embedding model: The processed data is segmented into chunks and fed into an embedding model. This model converts textual data into numerical representations called vectors, enabling AI models to comprehend it effectively. Prominent models used for this purpose are developed by OpenAI, Google, and Cohere.
- Vector database: The resulting vectors are stored in a vector database, facilitating streamlined querying and retrieval processes. This database efficiently manages the storage, comparison, and retrieval of potentially billions of embeddings (i.e., vectors). Prominent examples of such vector databases include Pinecone, Weaviate, and PGvector.
- APIs and plugins: APIs and plugins like Serp, Zapier, and Wolfram play a key role by connecting different components and enabling additional functionalities, such as accessing extra data or performing specific tasks easily.
- Orchestration layer: The orchestrating layer plays a pivotal role in managing the workflow. For instance, ZBrain exemplifies this layer by streamlining prompt chaining, handling interactions with external APIs to determine when API calls are necessary, fetching contextual data from vector databases, and preserving memory across multiple LLM calls. It offers templates for various applications mentioned earlier. This layer generates a prompt or series of prompts for submission to a language model for processing. Its responsibility lies in orchestrating data flow and tasks, ensuring smooth coordination among all components of the AI-powered complaint management architecture.
- Query execution: The data retrieval and generation process begins when a user submits a complaint through the customer service portal or any designated platform/channel. This query can cover any aspect pertinent to the complaint, including factors such as the customer’s previous interactions, the validity of their complaint, their satisfaction level, and any relevant details regarding the product or service in question.
- LLM processing: Upon receiving the query, the customer service portal forwards it to the orchestration layer. This layer retrieves pertinent data from the vector database and the LLM cache and then sends it to the suitable large language model for further processing. The selection of the appropriate LLM is based on the query’s nature.
- Output: The LLM generates an output based on the query and the data it receives. This output can be in various forms pertinent to complaint management, such as identifying the root cause of the complaint, suggesting potential solutions or actions to address the issue, drafting response messages for customer communication, or summarizing patterns and trends in customer feedback.
- Complaint management portal: The verified output is then presented to the user through the complaint management system. This central platform aggregates all data, analysis, and insights, presenting them in a user-friendly format. This empowers decision-makers to efficiently review and respond to customer complaints, facilitating prompt resolution and continuous improvement in customer satisfaction.
- Feedback loop: User feedback on the LLM’s output is another important aspect of this architecture. The feedback is used to improve the accuracy and relevance of the LLM output over time.
- Agent: AI agents play a vital role in this process by solving complex problems, interacting with the external environment, and enhancing learning through post-deployment experiences. They accomplish this by employing advanced reasoning and planning, strategically utilizing tools, memory, recursion, and self-reflection.
- LLM cache: To enhance the AI system’s responsiveness, commonly accessed information is cached using tools such as Redis, SQLite, or GPTCache. This caching mechanism accelerates data retrieval, optimizing the system’s performance and reducing response times.
- Logging/LLMOps: Throughout this process, LLM operations (LLMOps) tools like Weights & Biases, MLflow, Helicone and Prompt Layer help log actions and monitor performance. This ensures the LLMs are functioning optimally and continuously improve through feedback loops.
- Validation: A validation layer is employed to validate the LLM’s output. This is done through tools like Guardrails, Rebuff, Guidance, and LMQL to ensure the accuracy and reliability of the information provided by the LLM.
- LLM APIs and hosting: LLM APIs and hosting platforms are essential for performing complaint management tasks and hosting the application. Developers can tailor their choices based on project specifications. For LLM APIs, companies like OpenAI and Anthropic provide versatile solutions, while open-source models offer alternative avenues. Similarly, a range of hosting platforms is available, from major cloud providers like AWS, GCP, Azure, and Coreweave to specialized options such as Databricks, Mosaic, and Anyscale. The selection of LLM APIs and cloud hosting platforms depends on the distinct requirements and preferences of each project.
This structured flow provides a comprehensive insight into how AI enhances complaint management, leveraging diverse data sources and technological tools to generate precise and actionable solutions. AI streamlines various processes within complaint management, enhancing efficiency, enabling organizations to conduct thorough analyses of customer feedback, identify recurring issues, and implement effective strategies for complaint resolution process and continuous enhancement in customer satisfaction.
How do AI-powered customer complaint management systems work?
AI-powered customer complaint management systems operate by harnessing the capabilities of Natural Language Processing (NLP) to streamline and enhance the entire complaint-handling process. This system is powerful enough to automate responses, swiftly address issues, and identify patterns for product or service enhancements. The integration of AI into customer complaint management systems has enabled businesses to enhance customer satisfaction and cut operational costs. Let’s understand how the customer complaint management system works:
Data collection and entry
AI-powered systems are set up to monitor multiple channels where customers can submit complaints, such as emails, social media, chatbots, and web forms. These systems are programmed to continuously scan these channels for new incoming messages and complaints in real time. When a customer submits a complaint through any of these channels, the AI system first extracts the text from the incoming message or post. For example, if a complaint comes in as an email, the AI system would extract the text content of the email.
Content and context analysis
Once the text is extracted, NLP algorithms come into play to analyze the complaint’s content and context. NLP algorithms can identify keywords and phrases within the text that are relevant to the nature of the complaint. For instance, if a customer mentions “product defect” or “billing issue,” the NLP algorithms can recognize these key terms.
Categorization
After identifying key terms and phrases, the AI system categorizes the complaint based on predefined categories or tags. These categories can include “product-related issues,” “billing problems,” “shipping delays,” and more. NLP helps the system understand the context of the complaint, allowing it to assign the appropriate category accurately.
Sentiment analysis
AI can perform sentiment analysis on the complaint text to gauge the emotional tone of the customer. It analyzes words and phrases to determine whether the customer’s sentiment is positive, negative, or neutral. For example, if a customer uses words like “frustrated” or “disappointed,” the sentiment analysis would likely categorize the sentiment as negative.
Response generation
AI-powered chatbots excel at handling common and straightforward complaints by instantly retrieving predefined responses or information from a knowledge base. These responses are based on frequently encountered issues and are designed to provide quick and accurate solutions to customers. On the other hand, NLP models analyze the complaint content, context, and historical data for more complex complaints to generate personalized responses. This ensures customers feel heard and understood and receive appropriate, context-based solutions to their problems.
Automated triage and routing
The AI system automatically routes the complaint to the appropriate department or customer service agent based on the severity, urgency, and categorization assessment. For instance, a high-severity product defect complaint may be routed directly to the product support team, while a low-severity billing inquiry might go to the billing department.
Issue resolution assistance
AI can suggest solutions or troubleshooting steps to customer service agents based on historical data and knowledge bases. Machine learning algorithms can predict which resolutions are most likely to be effective based on past success rates. This guidance helps agents provide optimal solutions.
Analysis and reporting
AI continuously analyzes incoming complaints, categorization, sentiment, resolution outcomes, and customer feedback. This ongoing analysis helps organizations identify trends, emerging issues, and areas that require improvement.
Feedback loop and monitoring
AI can monitor customer satisfaction through post-interaction surveys and feedback analysis. Organizations can use this feedback to adjust their complaint management strategies and ensure a positive customer experience.
AI-powered customer complaint management systems offer efficiency, scalability, and the ability to provide customers with more personalized and timely support. They enable organizations to turn customer complaints into opportunities for improvement and demonstrate a commitment to customer satisfaction.
Advantages of AI in complaint management systems
AI offers numerous benefits compared to conventional complaint management techniques. The advantages of AI in complaint management systems are:
Faster response time: By harnessing the capabilities of an AI in a customer complaint management system, businesses can effectively analyze customer grievances and swiftly deploy automated responses. This dynamic approach translates to expedited problem resolution, as AI rapidly processes and addresses complaints, leading to shorter response times and ultimately fostering a higher degree of customer contentment.
Automation: Artificial Intelligence offers the capacity to automate mundane tasks and reduces the manual workload and administrative burden on staff, allowing them to direct their skills and attention toward more intricate and value-added tasks that require human insight and problem-solving. As a result, AI increases operational efficiency and empowers employees to engage in tasks that demand critical thinking and strategic decision-making, ultimately contributing to a more streamlined and productive work environment.
Accuracy: Artificial Intelligence possesses the remarkable ability to handle extensive datasets and discern complex patterns that may elude human perception. Its analytical prowess enables it to delve into intricate details within the data, revealing correlations, trends, and subtle relationships that might not be readily apparent to humans. By leveraging AI’s data processing capabilities, businesses can identify genuine sources of complaints with high precision. This empowers them to address issues at their core, preventing the recurrence of problems and demonstrating a proactive commitment to customer satisfaction.
Cost savings: Through the automation of monotonous tasks and the optimization of operations, AI expedites task completion, diminishes manual effort, and amplifies overall operational efficiency within enterprises. This results in considerable time and cost savings as employees can focus on higher-value activities, processes become more streamlined, and resources are allocated more effectively.
Ensuring data privacy and ethical use of AI in complaint management
When integrating AI into complaint management systems, maintaining ethical standards and safeguarding customer data privacy is paramount. Several key considerations ensure responsible AI deployment:
Transparency: Customers should be notified when AI is involved in their complaint resolution process. Transparency is crucial to building trust, and businesses should provide clear explanations of how AI-generated responses are formulated.
Data privacy: Protecting customer data is a priority. Firms must adhere to stringent data protection regulations, ensuring that data collection, storage, and usage comply with privacy laws. AI models should be designed with data anonymization and encryption to prevent unauthorized access.
Bias mitigation: Businesses must actively address biases in AI algorithms that can lead to unfair treatment. Regular audits and adjustments are necessary to counteract any inadvertent discrimination and ensure equitable treatment of all customers.
Human oversight: While AI aids efficiency, human oversight is indispensable to reviewing AI-generated responses for accuracy, relevance, and ethical considerations. This human touch guarantees that responses align with company values and customer expectations.
By addressing these challenges and embracing ethical AI practices, businesses can harness the benefits of AI for complaint management while preserving customer trust and safeguarding their sensitive information. This approach cultivates a responsible and ethical implementation of AI technology that benefits both businesses and their customers.
Endnote
Incorporating AI in complaint management has emerged as a strategic imperative in modern business operations. The deployment of AI within the complaint management system ushers in a paradigm shift that leverages advanced technologies to optimize customer interactions. By harnessing AI for customer complaint management, businesses can proactively streamline issue resolution, ensure data privacy, enhance personalized responses, and mitigate potential biases. This dynamic use of AI in complaint management systems enhances operational efficiency, fosters customer satisfaction, and solidifies an organization’s commitment to elevating the complaint management process. Through the astute utilization of AI in the complaint management system, enterprises can navigate the complexities of contemporary customer service, imbuing it with agility and effectiveness while aligning with evolving customer expectations.
Enhance customer experience with our custom AI-driven complaint management systems. Contact LeewayHertz’s AI experts for a solution fitting your needs.
Start a conversation by filling the form
All information will be kept confidential.
Insights
AI in predictive maintenance: Use cases, technologies, benefits, solution and implementation
AI offers numerous applications in predictive maintenance across a variety of industries, leveraging its capabilities in data analysis, machine learning, and predictive analytics to improve equipment reliability and operational efficiency.
Conversational AI: Use cases, types and solution
Conversational AI is a subset of artificial intelligence that enables human-like interactions between computers and humans using natural language.
AI for claims processing: Use cases, benefits and development
AI plays a significant role in resolving challenges in claims processing by introducing automation, data analysis, and advanced decision-making capabilities.