AI for claims processing: Use cases, architecture, benefits and development
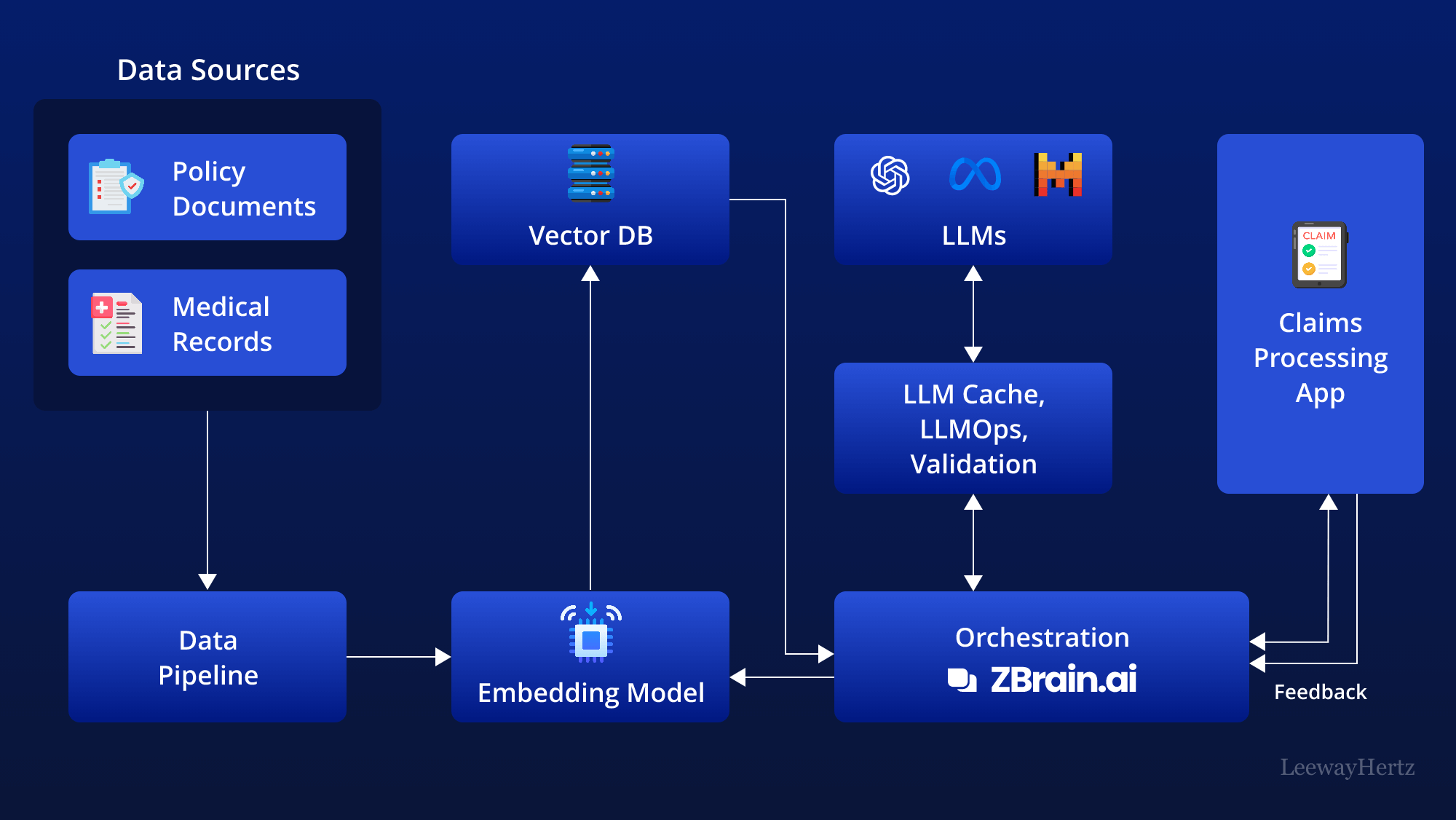
In the unpredictable journey of life, uncertainties often take center stage, demanding the reassurance that comes from a robust insurance shield. Amidst the twists and turns, insurance claims bridge vulnerability and resilience, offering a lifeline when the unexpected occurs. This process isn’t just for one type of insurance. It covers many areas, from simple claims to more complicated situations like negligence or professional indemnity claims. The steps might be similar, but how much thought and work goes into it can vary.
The role of AI emerges as a transformative force, reshaping the landscape of claim management. As the insurance industry navigates the complexities of claims processing, AI catalyzes streamlining and enhancing every stage of claim processing. AI’s involvement begins at the initial point of contact, where intelligent chatbots and virtual assistants efficiently gather information from policyholders. Furthermore, AI in claims processing is pivotal in thoroughly examining claims and utilizing advanced algorithms to detect patterns and anomalies that might signal potential fraud. By analyzing vast datasets and identifying irregularities, AI claim processing contributes significantly to the security and integrity of the insurance ecosystem, safeguarding against fraudulent activities.
AI claims management software transcends mere automation; it represents a paradigm shift towards a more intelligent, data-driven, and customer-centric approach. As the insurance industry embraces the potential of AI, it fortifies its capacity to navigate the unpredictable journey of life, providing a resilient shield against uncertainties. As per Allied Market Research, the worldwide market size for claims processing software reached $33.9 billion in 2020, with projections indicating substantial growth. Anticipated to surge at a Compound Annual Growth Rate (CAGR) of 8.3% from 2021 to 2030, the market is expected to achieve a valuation of $73.0 billion by the end of this decade.
This article delves into the ways in which AI plays a crucial role in addressing challenges in claims processing. It further explores the diverse applications of AI in claims processing, examining how it contributes to overcoming various hurdles in the insurance industry.
- What is claims processing?
- Challenges in claims processing and how AI addresses these challenges
- How does AI for claims processing work?
- Use cases of AI in claims processing
- Claims intake and triage
- Document image processing
- Fraud detection and prevention
- Predictive analytics for risk assessment
- Automated claims settlement
- Telematics in auto insurance claims
- Subrogation optimization
- Image and photo analysis
- Chatbots for initial customer interaction
- Litigation prediction
- Regulatory compliance monitoring
- Claims lifecycle monitoring
- Settlement optimization
- Data analytics for claims trend analysis
- Claims leakage detection
- Optimizing claims processing: How does generative AI enhance each step?
- How does LeewayHertz’s enterprise AI solution optimize claims processing workflows?
- LeewayHertz’s AI development services for claims processing
- Benefits of AI in claims processing
- How to build an AI-powered claims processing system?
- Considerations for building an AI-powered claims processing system
- AI in claims processing: Innovative trends
What is claims processing?
Claims processing is the comprehensive procedure for handling policyholder claims, encompassing all stages from the initial contact to the case resolution. It involves triaging claims, conducting reviews, investigating potential fraud, making adjustments as needed, and ultimately deciding whether to accept or reject the claim.
This process applies to claims from various insurance domains, covering a spectrum of complexities. Whether dealing with claims for low-value items or navigating more intricate situations like negligence or professional indemnity claims, the pathways followed involve similar steps, though the level of judgment and involvement may vary.
Claims processing entails intricate and nuanced tasks, including legal and technical checks that must be completed before a claim can be approved. Additionally, substantial administrative duties are integral to the process, prompting many insurers to consider outsourcing these aspects of claims processing. Despite its complexities, the fundamental checks and procedures involved in claims processing are not inherently difficult.
Challenges in claims processing and how AI addresses these challenges
AI plays a significant role in overcoming challenges in claims processing by introducing automation, data analysis, and advanced decision-making capabilities. Here’s how AI can address the claim challenges mentioned:
AI-powered claim processing significantly improves various aspects of the insurance industry, enhancing efficiency and accuracy in the claims processing journey.
Efficiencies in the claims registration process
Challenge: The claims registration process often involves substantial data-intensive and repetitive tasks, leading to operational inefficiencies. The manual handling of this process can result in delays, errors, and increased workload.
Solution: AI in claims processing automates repetitive tasks in claims registration, minimizing errors and accelerating the entire process. Natural Language Processing (NLP) further simplifies things by extracting relevant information from documents, making the registration process smoother.
Streamlining claims adjuster’s allocation
Challenge: Assigning claims adjusters based on availability, workload, and location data can be complex. Inefficiencies may arise when these assignments are not dynamically optimized in real-time, leading to delays and potential resource mismatches.
Solution: Machine learning algorithms analyze historical data to allocate claims adjusters based on expertise and workload smartly. Real-time analytics provide insights into adjuster availability, ensuring a more dynamic and efficient assignment process.
Real-time claims estimation
Challenge: Non-life insurers spend much time on data preparation and modeling rather than claims reserve reporting. This data-intensive process can hinder real-time claims estimation and decision-making.
Solution: AI swiftly estimates claims through predictive analytics by considering historical data and current market trends. This empowers insurers to make quicker and more accurate decisions, expediting the claims estimation.
Fraud detection
Challenge: Fraudulent claims pose a significant challenge for insurers, with approximately 10% of claims in the US being deemed fraudulent from property-casualty insurance losses. Detecting fraud early is crucial for maintaining key metrics, such as the loss ratio, and preventing financial losses.
Solutions: AI employs pattern recognition to analyze claims data for potential fraud indicators. Continuous learning through machine learning models improves detection accuracy over time. Predictive modeling assesses the likelihood of fraud, helping insurers prioritize investigations and reduce false positives.
Inspection of hazardous locations
Challenge: Human inspectors face safety risks in hazardous or dangerous locations where inspections are necessary for damage assessment. False compensation claims can complicate the evaluation process, requiring careful and secure inspection procedures.
Solutions: AI-powered drones with cameras and sensors conduct inspections in hazardous locations, capturing high-quality images and data while minimizing risks to human inspectors. Computer vision systems analyze these visuals, flagging potential issues or fraudulent claims and adding an extra layer of security to the inspection process.
Addressing these challenges is crucial for insurers to optimize their claims operations, improve efficiency, and enhance the overall effectiveness of their risk management processes. Integrating advanced technologies and innovative solutions is key to overcoming these hurdles in claims processing.
How does AI for claims processing work?
Incorporating AI into claims processing involves a comprehensive approach to streamline the entire procedure, making it more efficient, accurate, and cost-effective. It goes beyond traditional claims processing by incorporating powerful Large Language Models (LLMs) and connecting them with an organization’s unique knowledge base. This approach unlocks a new level of insight generation and empowers businesses to make data-driven decisions in real time.
This architecture leverages various components to streamline the claims processing workflow. Here’s a step-by-step breakdown of how it works:
Data sources: The process begins by gathering data from various sources relevant to the claims process. This data can include:
- Claim forms: Information provided by the policyholder regarding the claim.
- Policy documents: Details of the insurance policy covering the claim.
- Medical records: For health-related claims, medical documentation is retrieved.
- Damage assessments: Reports from adjusters or third-party assessments for property or auto claims.
Data pipelines: Data from the sources listed above are then routed through data pipelines. These pipelines are responsible for the ingestion, cleaning, and structuring of data, making it ready for further analysis.
Embedding model: The prepared data is then processed by an embedding model. This model transforms the textual data into numerical representations called vectors that AI models can understand. Popular embedding models include those from OpenAI, Google, and Cohere.
Vector database: The generated vectors are stored in a vector database, which allows for efficient querying and retrieval. Examples of prominent vector databases include Pinecone, Weaviate, and PGvector.
APIs and plugins: APIs and plugins like Serp, Zapier, and Wolfram play a key role by connecting different components and enabling additional functionalities, such as accessing extra data or performing specific tasks with ease.
Orchestration layer: The orchestration layer is critical in managing the workflow. ZBrain is an example of this layer that simplifies prompt chaining, manages interactions with external APIs by determining when API calls are required, retrieves contextual data from vector databases, and maintains memory across multiple LLM calls. Ultimately, this layer generates a prompt or series of prompts that are submitted to a language model for processing. The role of this layer is to orchestrate the flow of data and tasks, ensuring seamless coordination across all components within the architecture.
Query execution: The data retrieval and generation process begins when the user submits a query to the claims processing app. This query can be about anything relevant to the claim, such as its status, required documentation, or potential issues.
LLM processing: Once received, the app transmits the query to the orchestration layer. This layer retrieves relevant data from the vector database and LLM cache and sends it to the appropriate LLM for processing. The choice of LLM depends on the nature of the query.
Output: The LLM generates an output based on the query and the data it receives. This output can take various forms, such as summaries of claim status, identification of potential fraud, or generation of communication drafts.
Claims processing app: The validated output is then presented to the user through the claims processing app. This is the core application where all the data, analysis, and insights converge. It presents the findings in a user-friendly format for claim handlers and decision-makers.
Feedback loop: User feedback on the LLM’s output is another important aspect of this architecture. The feedback is used to improve the accuracy and relevance of the LLM output over time.
Agent: AI agents step into this process to address complex problems, interact with the external environment, and enhance learning through post-deployment experiences. They achieve this by employing advanced reasoning/planning, strategic tool utilization, and leveraging memory, recursion, and self-reflection.
LLM cache: Tools like Redis, SQLite, or GPTCache are used to cache frequently accessed information, speeding up the response time of the AI system.
Logging/LLMOps: Throughout this process, LLM operations (LLMOps) tools like Weights & Biases, MLflow, Helicone, and Prompt Layer help log actions and monitor performance. This ensures the LLMs are functioning optimally and continuously improve through feedback loops.
Validation: A validation layer is employed to validate the LLM’s output. This is done through tools like Guardrails, Rebuff, Guidance, and LMQL to ensure the accuracy and reliability of the information provided.
LLM APIs and hosting: LLM APIs and hosting platforms are essential for executing claims processing tasks and hosting the application. Depending on the requirements, developers can select from LLM APIs offered by firms such as OpenAI and Anthropic or opt for open-source models. Similarly, they can choose hosting platforms from cloud providers like AWS, GCP, Azure, and Coreweave or opt for opinionated clouds like Databricks, Mosaic, and Anyscale. The choice of LLM APIs and cloud hosting platforms depends on the project’s needs.
This structured flow provides a detailed overview of how AI facilitates claims processing, leveraging various data sources and technological tools to generate accurate and actionable insights. Overall, AI automates various tasks involved in claims processing, improves efficiency, and enables a more comprehensive analysis of claims.
Optimize Your Operations With AI Agents
Optimize your workflows with ZBrain AI agents that automate tasks and empower smarter, data-driven decisions.
Use cases of AI in claims processing
AI has become a transformative force in claim processing across various industries. Several relevant use cases highlight the impact of AI in streamlining and enhancing the efficiency of the claims management process. The use cases of AI in claim processing are:
Claims intake and triage
AI algorithms play a pivotal role in automating the initial assessment of incoming claims. By leveraging advanced data analysis and pattern recognition, these algorithms swiftly categorize claims based on severity, complexity, and the specific actions required. This intelligent automation ensures a rapid and accurate sorting process, enabling insurers to prioritize high-priority claims that may demand immediate attention. Additionally, AI-driven triage optimizes resource allocation by directing complex cases to specialized adjusters and straightforward claims to automated processing, ultimately streamlining the entire claims management workflow for enhanced efficiency and customer satisfaction.
Document image processing
By leveraging advanced algorithms, AI technology can swiftly analyze and extract pertinent information from diverse document types, such as invoices, receipts, and policy documents. This expedites the verification and validation process and significantly reduces the need for manual intervention. Insurers can better assess claim details, ensure accuracy, and accelerate the overall claims adjudication timeline. Ultimately, this use case enhances operational productivity and contributes to a seamless and expedited claims experience for policyholders.
Fraud detection and prevention
AI employs historical data and advanced pattern recognition algorithms to meticulously analyze insurance claims, aiming to identify and prevent potential fraud effectively. By analyzing patterns and identifying anomalies within the data, the system assigns fraud scores to claims, quantifying the likelihood of fraudulent activity. Claims with high fraud scores automatically trigger further investigation by claims adjusters or special investigation units. This proactive approach enables insurers to swiftly intervene in high-risk cases, mitigating potential losses and safeguarding the integrity of the claims management process. Ultimately, AI-driven fraud detection enhances claims processing efficiency and contributes to a more secure and trustworthy insurance environment.
Predictive analytics for risk assessment
AI models analyze historical claims data using predictive analytics to assess the potential risk of a new claim. The AI system calculates the risk score by considering past claim patterns, severity, and outcomes, aiding insurers in accurate reserve setting. This predictive capability enables more informed decision-making, allowing insurers to allocate resources efficiently and proactively manage potential financial exposures. The result is a streamlined claims management process that optimizes resource utilization, enhances financial planning, and improves overall operational efficiency in the insurance industry.
Automated claims settlement
AI streamlines the claims settlement process by analyzing policy terms to determine coverage parameters. Next, it assesses damages by leveraging image analysis and data processing tools, extracting insights from photos or documents submitted with the claim. The AI system then applies predefined rules and algorithms to calculate settlements accurately, considering factors such as policy limits and deductibles. By automating these intricate tasks, insurers can achieve faster and more consistent claims settlements, reducing manual intervention and enhancing overall operational efficiency. This accelerates the resolution process and ensures adherence to policy guidelines, resulting in a more streamlined and customer-centric claims experience.
Telematics in auto insurance claims
In the auto insurance sector, AI-driven telematics leverage real-time driving behavior data from connected devices to reconstruct accident conditions. AI algorithms generate a detailed and accurate representation of the events surrounding the accident by analyzing factors such as speed, acceleration, braking, and vehicle trajectory leading up to the incident. This data-driven reconstruction expedites the claims process by providing insurers with immediate insights and enhancing accuracy in determining liability and assessing damages. Integrating AI in telematics transforms claims processing, enabling insurers to make faster settlements and reducing the administrative burden associated with accident assessments.
Subrogation optimization
Subrogation refers to the process of recovering losses from third parties responsible for an incident. AI plays a pivotal role by systematically analyzing claim data, policy details, and external information to pinpoint subrogation opportunities. By leveraging advanced algorithms, AI can identify liable parties, assess liability percentages, and streamline recovery. This ensures that insurers can efficiently and accurately hold those responsible for losses accountable, contributing to cost recovery and overall financial optimization in claims management. The automated nature of AI in subrogation enhances the speed and precision of this intricate process, allowing insurers to maximize their recovery efforts.
Image and photo analysis
AI image analysis transforms claims processing by automatically evaluating photos submitted with claims. The technology employs advanced algorithms to detect and assess damages, offering adjusters a swift and precise overview of the situation. By rapidly identifying and quantifying the extent of damages through image analysis, adjusters can expedite their decision-making process, leading to quicker claims settlements. This enhances operational efficiency and minimizes delays, providing policyholders with a faster and more transparent claims experience. The AI’s ability to objectively analyze visual evidence improves accuracy in claim assessments, reducing the potential for human error.
Chatbots for initial customer interaction
AI-powered chatbots in claims reporting serve as virtual assistants for policyholders, streamlining the initial stages of the claims process. These chatbots conversationally interact with customers, guiding them through the necessary steps to report a claim. Through natural language processing, AI chatbots efficiently collect essential information such as incident details and relevant documentation. This enhances the customer experience by providing immediate assistance and ensures the accurate and timely gathering of crucial data for subsequent claims processing. In this context, chatbots demonstrate the fusion of automation and customer engagement, optimizing the efficiency of claims reporting while maintaining a user-friendly interface.
Litigation prediction
AI-driven predictive analytics harness historical claims data to assess the probability of a claim escalating to litigation. The model can identify high-risk claims early by evaluating patterns and key indicators. Insurers can allocate resources strategically, directing specialized personnel and legal support to cases with a heightened likelihood of litigation. This proactive approach enables insurers to manage legal expenses efficiently, streamline the claims resolution process, and mitigate potential legal challenges, ultimately contributing to cost savings and improved overall claims management.
Regulatory compliance monitoring
AI is pivotal in ensuring that insurance claims processes adhere to dynamic and evolving regulatory frameworks. AI algorithms can swiftly identify deviations or non-compliance with established regulations by continuously monitoring and analyzing data throughout the claims lifecycle. This proactive approach mitigates the risk of penalties and legal complications and streamlines the overall claims management process. AI’s ability to adapt to regulatory changes in real-time ensures that insurers maintain compliance, fostering a secure and transparent claims environment while saving valuable time and resources previously spent on manual compliance checks.
Claims lifecycle monitoring
AI-powered dashboards in claims processing leverage real-time data analytics to offer immediate insights into the status and progression of claims. These dashboards dynamically display key performance indicators, claim processing stages, and potential bottlenecks. This enables insurers to proactively identify issues, allocate resources efficiently, and intervene promptly when necessary. The system can automatically flag delays, outliers, or critical milestones, empowering decision-makers to take timely actions for a more responsive and streamlined claims management process. Using AI in dashboards enhances operational agility and responsiveness in managing the claims lifecycle.
Settlement optimization
AI-driven settlement optimization leverages historical claims data to determine the most suitable settlement amounts for individual cases, considering factors like the nature of the claim, its severity, and the policy coverage in place. By analyzing vast datasets, AI algorithms can discern patterns and trends that inform fair and reasonable settlement recommendations. This allows insurers to streamline negotiations, ensuring efficient resolution while mitigating potential losses. By employing AI-driven settlement optimization, insurers can achieve a balance between meeting policyholder needs and safeguarding their bottom line, ultimately enhancing operational efficiency and customer satisfaction within the claims processing workflow.
Data analytics for claims trend analysis
AI can analyze large volumes of claims data to identify trends and patterns over time. By examining factors such as claim frequency, severity, geographic distribution, and seasonality, AI algorithms can provide valuable insights to insurers for risk management and strategic decision-making. For example, insurers can use AI-powered analytics to anticipate emerging risks, adjust pricing models, and develop targeted loss prevention initiatives. Additionally, AI can help insurers identify opportunities for process optimization and cost reduction by highlighting inefficiencies or bottlenecks in the claims processing workflow. Overall, leveraging AI for data analytics enables insurers to gain a deeper understanding of their claims portfolio and make data-driven decisions to improve operational efficiency and profitability.
Claims leakage detection
Claims leakage detection involves the use of AI algorithms to identify instances where insurers are incurring unnecessary expenses or missing opportunities to recover costs. These leaks can occur due to various factors, such as errors in claims processing, fraudulent activities, or inefficiencies in the system. AI analyzes large volumes of claims data, comparing it against predefined patterns and historical trends to pinpoint discrepancies or anomalies. By detecting these instances early on, insurers can take corrective actions to plug the leaks, whether it involves recovering funds from responsible parties, improving internal processes, or enhancing fraud detection measures. Ultimately, leveraging AI for claims leakage detection not only improves profitability for insurers but also strengthens the overall integrity of the claims processing system.
These use cases showcase how AI technologies can be strategically implemented to address specific challenges in claim processing, leading to increased efficiency, accuracy, and overall improvements in the insurance industry.
Optimize Your Operations With AI Agents
Optimize your workflows with ZBrain AI agents that automate tasks and empower smarter, data-driven decisions.
Optimizing claims processing: How does generative AI enhance each step?
Generative AI is transforming the claims processing landscape by streamlining and automating each stage of the lifecycle. From capturing and evaluating claims to investigating and settling, GenAI-driven solutions offer exceptional efficiency and accuracy. By leveraging them, insurance companies can reduce processing times, minimize errors, and improve overall claim management. Here is how generative AI enhances each step of the claims process, ensuring a smoother experience for both insurers and policyholders.
Claim intake and screening
Steps | Action | Generative AI Application |
Gather information |
|
|
Evaluate claim |
|
|
Determine validity |
|
|
Investigation
Steps | Action | Generative AI application |
Gather evidence |
|
|
Analyzing and verifying evidence |
|
|
Determining causation |
|
|
Assessment
Steps | Action | Generative AI application |
Assess coverage |
|
|
Liability analysis |
|
|
Regulatory compliance |
|
|
Negotiation
Steps | Action | Generative AI application |
Evaluate claims |
|
|
Communicate offers |
|
|
Resolve disputes |
|
|
Finalize settlement |
|
|
Settlement
Steps | Action | Generative AI application |
Assess settlement |
|
|
Release funds |
|
|
Issue documentation |
|
|
Conclude settlement |
|
|
The above tables show how generative AI transforms claims processing by automating tasks, enhancing decision-making, and improving accuracy. GenAI streamlines workflows, reduces errors, and ensures compliance, enabling insurers to boost efficiency, lower costs, and deliver faster, more accurate service to policyholders. GenAI is the future of claims processing, transforming operations with smarter, data-driven solutions.
How does LeewayHertz’s enterprise AI solution optimize claims processing workflows?
LeewayHertz’s generative AI platform, ZBrain, plays a transformative role in optimizing claims processing workflows across financial institutions by elevating risk assessment processes, enhancing accuracy, and expediting decision-making for unparalleled efficiency. As a comprehensive, enterprise-ready platform, ZBrain empowers businesses to design and implement applications tailored to their specific operational requirements. The platform uses clients’ data, whether in the form of text, images, or documents, to train advanced LLMs like GPT-4, Vicuna, Llama 2, or GPT-NeoX for developing contextually aware applications capable of performing diverse tasks.
Enterprises grapple with challenges in claims processing, navigating through issues such as manual data entry errors, fraud detection intricacies, regulatory compliance, inconsistent data formats, communication hurdles, high claim volumes, human resource dependencies, and the intricate nature of policy details. ZBrain effectively addresses these challenges through its distinctive feature called “Flow,” which provides an intuitive interface that allows users to create intricate business logic for their apps without the need for coding. Flow’s easy-to-use drag-and-drop interface enables the seamless integration of large language models, prompt templates, and media models into your app’s logic for its easy conceptualization, creation, or modification.
To comprehensively understand how ZBrain Flow works, explore this resource that outlines a range of industry-specific Flow processes. This compilation highlights ZBrain’s adaptability and resilience, showcasing how the platform effectively meets the diverse needs of various industries, ensuring enterprises stay ahead in today’s rapidly evolving business landscape.
ZBrain’s robust applications enhance claims processing by translating intricate data into actionable insights, leading to heightened efficiency, expediting claims settlements, minimizing losses from fraudulent activities, and achieving efficiency gains that ultimately contribute to substantial operational cost reductions. ZBrain adeptly addresses inherent challenges in claims processing, offering effective and strategic resolutions to streamline the entire claims management workflow. Delve into the following section, elucidating how ZBrain executes insurance claims processing with remarkable speed and accuracy.
AI-powered insurance claims processing
ZBrain simplifies and automates insurance claim processing by efficiently collecting essential data, including customer records, external data sources, medical records, policyholder information, and government data. Through automated Exploratory Data Analysis (EDA), ZBrain reveals valuable insights, identifying patterns, anomalies, and historical trends to enhance the efficiency of the insurance claim processing procedure significantly. Using advanced techniques, ZBrain converts textual data into numerical embeddings, capturing semantic relationships for efficient retrieval and analysis. These embeddings facilitate assessing claim information against policy terms, medical records, and external data to determine claim validity and calculate settlement amounts. Leveraging the chosen Large Language Model (LLM), ZBrain assesses the insurance claim status and promptly generates detailed reports in response to user queries, providing crucial information about claim assessment and proposed settlements with high efficiency and consistency. With the aid of embeddings, the LLM conducts a comprehensive review to detect potential signs of fraud and offers actionable recommendations for the claim. Following LLM-generated reports, ZBrain utilizes parsing techniques to refine and extract insights, delivering well-organized data that expedites the approval process and reduces the time required for claim settlement.
ZBrain applications enable organizations in claims processing to achieve enhanced efficiency, accuracy, fortified fraud detection, access to data-driven insights, policy customization, adaptability to emerging risks, streamlined underwriting processes, and heightened compliance. This facilitates a more agile claims processing landscape, driving operational enhancements, cost efficiencies, and elevated service quality, ultimately benefiting insurance institutions and their customers. Delve into this detailed Flow to understand how ZBrain transforms insurance claims processing, providing an efficient approach to evaluating claim validity and optimizing the overall decision-making process in settlements.
LeewayHertz’s AI development services for claims processing
At LeewayHertz, we craft tailored AI solutions that cater to the unique requirements of insurance firms focusing on claims processing. We provide strategic AI/ML consulting that enables these firms to harness AI for enhanced decision-making, improved client engagement, and optimized claims-handling strategies.
Our expertise in developing Proof of Concepts (PoCs) and Minimum Viable Products (MVPs) allows insurance companies to preview the potential impacts of AI tools in real scenarios, ensuring that the solutions are both effective and tailored to the insurance sector’s claims processing related needs.
Our work in generative AI transforms routine tasks like claims documentation and data management, automating these processes to free up claims adjusters for more strategic roles.
By fine-tuning large language models to the nuances of insurance terminology and client interactions, LeewayHertz enhances the accuracy and relevance of AI-driven communications and analyses.
Additionally, we ensure these AI systems integrate seamlessly with existing technological infrastructures, enhancing operational efficiency and decision-making in claims processing departments.
Our AI solutions development expertise
AI solutions development for claims processing typically involves creating systems that enhance decision-making, automate routine tasks, and personalize client services. These solutions integrate key components such as data aggregation technologies, which compile and analyze claim-related information from diverse sources. This comprehensive data foundation supports predictive analytics capabilities, enabling informed strategic decisions in insurance claims processing. Additionally, machine learning algorithms are employed to tailor claims handling procedures to individual claim scenarios, ensuring that each case is processed efficiently and accurately. These solutions often cover areas like fraud detection, risk assessment, regulatory compliance, and customer relationship management.
Overall, AI solutions in claims processing aim to optimize operational outcomes, reduce processing times, and enhance the client experience.
AI agent/copilot development for claims processing
LeewayHertz builds custom AI agents and copilots that enhance various claims processing operations, enabling companies to save time and resources while facilitating faster and more accurate decision-making. Here is how they help:
Claim analysis:
- Analyzing claim data to quickly assess claim validity and estimate payouts.
- Employing pattern recognition to identify potentially fraudulent claims based on anomalies in the data.
Client engagement:
- Automating routine communication tasks like claim updates and reminders tailored to individual client preferences.
- Offering round-the-clock virtual support to answer client queries and provide information on claim status.
Compliance and risk monitoring:
- Automating the analysis of claims documentation to ensure adherence to industry regulations and standards.
- Monitoring claims for unusual patterns that might indicate higher risks or potential fraud.
Process automation:
- Automating tasks such as data entry, document scanning, and initial claim reviews to speed up the claims process.
- Automating the retrieval and management of claim-related documents, reducing manual errors and saving time.
Strategic decision making:
- Forecasting claim trends involves analyzing past data to predict future volumes and identify bottlenecks, focusing on seasonal variations, claims frequency and severity, and processing efficiencies to optimize resource allocation.
- Generating instant reports on claims statistics and operations to help managers make informed decisions quickly.
AI agents and copilots developed by LeewayHertz not only increase the efficiency of claims processing but also significantly enhance the quality of customer service and strategic decision-making. By integrating these advanced AI solutions into their existing infrastructure, insurance companies can achieve a significant competitive advantage, navigating the complex landscape of claims management with innovative, efficient, and reliable AI-driven tools and strategies. This leads to faster claim resolutions, reduced operational costs, and improved customer satisfaction.
Benefits of AI in claims processing
The benefits of AI in claims processing are:
Efficiency and speed: AI streamlines claim processing by automating repetitive tasks, reducing processing times, and enabling swift decision-making. This expedites the claims lifecycle and ensures timely resolution, improving overall operational efficiency.
Cost savings: Automation and predictive analytics significantly cut operational costs by minimizing the need for manual labor in routine tasks. The optimized resource allocation ensures that human expertise is utilized where needed most, maximizing cost-effectiveness throughout the claims management process.
Accuracy and precision: AI algorithms enhance data extraction, fraud detection, and risk assessment accuracy. By minimizing errors, insurers can rely on more precise information, leading to better-informed decisions and higher trust in the claims processing system.
Customer experience improvement: Faster claims processing, personalized communication based on AI insights, and proactive issue resolution contribute to an improved customer experience. The timely and efficient handling of claims enhances customer satisfaction, fostering loyalty and positive brand perception.
Data-driven decision-making: AI provides actionable insights through data analysis. Insurers can make informed decisions, optimize processes, and align business strategies with market trends. This data-driven approach enhances strategic planning and adaptability to changing market dynamics.
Resource optimization: AI algorithms intelligently allocate resources, ensuring that claims are assigned to the right personnel based on their expertise and current workload. This not only optimizes workforce productivity but also enhances the overall efficiency of the claims management team, leading to improved outcomes and customer satisfaction.
How to build an AI-powered claims processing system?
Building an AI-driven automated claims processing system involves several key steps, each of which is critical for ensuring accuracy, efficiency, and reliability in handling insurance claims. Here’s a detailed breakdown of the process:
Problem definition and planning
In defining the requirements and scope of an AI-driven claims processing system, it’s crucial to thoroughly grasp the system’s intended functionality and objectives. This involves identifying the types of claims to be handled, whether they pertain to insurance, healthcare, or other domains, and understanding the data sources accessible for analysis. Additionally, outlining the desired outcomes, such as increased efficiency, accuracy, or fraud detection, helps shape the development process. By clarifying these parameters early on, developers can streamline the subsequent stages of data collection, model development, and deployment, ensuring alignment with the system’s overarching goals.
Data collection
In the process of developing an AI-driven automated claims processing system, data collection serves as a fundamental step where historical claims data is gathered from diverse sources and structured appropriately. This data aggregation encompasses extracting relevant information from existing databases within the organization or external partners, as well as obtaining data from filled-out claim forms submitted by customers. Techniques such as natural language processing (NLP) are utilized to parse relevant information from emails where claims are submitted digitally. Additionally, in cases where claims are submitted as scanned documents, optical character recognition (OCR) technology is employed to convert scanned text into a machine-readable format, facilitating further processing.
Ensuring that collected data is structured and labeled correctly is essential for effective analysis and modeling within the claims processing system. Data structuring involves organizing the collected information into a structured format, such as databases or spreadsheets, where each entry represents a single claim, and columns denote different attributes or features of the claim. Concurrently, appropriate labels are assigned to the claims data based on predefined categories or outcomes, ensuring consistency and accuracy in labeling to prevent errors during model training. Including relevant metadata such as claim type, date of submission, claim amount, and outcome further enriches the dataset, providing valuable context and insights for analysis and decision-making throughout the claims processing workflow.
Data preprocessing
Data preprocessing is a crucial stage in building an AI-driven claims processing system as it involves preparing the collected data to be effectively utilized for training machine learning models. This process begins with data cleaning, which entails identifying and rectifying inconsistencies, errors, or outliers in the dataset that could potentially skew the model’s performance. Following data cleaning, normalization techniques are applied to ensure that data across different features or attributes are scaled to a consistent range, preventing certain features from dominating others during model training. Moreover, feature engineering is employed to extract relevant information and create new features that enhance the predictive power of the models. This might involve transforming categorical variables into numerical representations or deriving new features from existing ones to capture additional insights.
In addition to cleaning and feature engineering, handling missing values is another critical aspect of data preprocessing. Missing data can significantly impact the performance of machine learning models if not addressed properly. Techniques such as imputation, where missing values are replaced with estimated values based on the available data, or deletion of incomplete records may be employed to manage missing data effectively. However, the choice of approach depends on the nature and extent of missingness in the dataset, as well as the requirements of the claims processing system.
Model development
Model development is a pivotal phase in developing an AI-driven claims processing system, focusing on crafting machine learning models that automate different facets of the claims processing workflow. Natural Language Processing (NLP) models play a vital role in extracting valuable information from unstructured text, such as claim descriptions or medical reports. These models employ techniques like tokenization, entity recognition, and sentiment analysis to parse and understand textual data, enabling efficient processing and decision-making. Furthermore, computer vision models are utilized to analyze images or scanned documents associated with claims, aiding in tasks such as damage assessment, document classification, or verification of supporting evidence. Leveraging deep learning architectures like convolutional neural networks (CNNs), these models can accurately interpret visual content and extract relevant features for downstream processing.
In addition to NLP and computer vision, predictive modeling techniques are deployed for tasks like fraud detection or claim approval, where historical data is utilized to forecast outcomes or identify patterns indicative of fraudulent activity. These models employ algorithms such as logistic regression, decision trees, or ensemble methods to classify claims based on risk or likelihood of fraud, facilitating timely intervention and mitigation of potential losses. By leveraging diverse machine learning approaches tailored to specific aspects of the claims processing workflow, organizations can streamline operations, enhance accuracy, and expedite decision-making, ultimately improving the efficiency and effectiveness of their claims processing systems. Moreover, continual refinement and optimization of these models through iterative testing and validation ensure adaptability and robustness in handling evolving challenges and scenarios in claims processing.
Model training
In this step, preprocessed data is used to train models to recognize patterns and make predictions. Typically, the dataset is divided into three subsets: training, validation, and test sets. The training set is used to train the model to understand the underlying patterns in the data, while the validation set is employed to fine-tune model parameters and assess performance during training. The test set, held out from the training process, serves as an independent benchmark to evaluate the model’s generalization and performance on unseen data.
During training, various machine learning algorithms are applied depending on the nature of the problem and the data characteristics. Deep learning models, such as convolutional neural networks (CNNs) or recurrent neural networks (RNNs), excel at learning intricate patterns from complex data sources like images or sequential data. Random forests, on the other hand, are well-suited for tasks requiring feature importance analysis and handling non-linear relationships in structured data. Support vector machines (SVMs) are effective for binary classification tasks, offering robust performance even in high-dimensional feature spaces. By iteratively adjusting model parameters based on feedback from the validation set, the training process aims to optimize model performance and enhance its ability to accurately predict outcomes in real-world scenarios.
Testing and evaluation
Testing and evaluation are crucial stages in the development of an AI-driven claims processing system, where the performance of trained models is rigorously assessed using relevant metrics tailored to the specific use case. Metrics such as accuracy, precision, recall, F1-score, and area under the ROC curve (AUC) provide insights into different aspects of model performance and help gauge its effectiveness in making accurate predictions. Accuracy measures the proportion of correctly predicted outcomes over the total number of predictions, providing an overall assessment of model correctness. Precision quantifies the fraction of true positive predictions among all positive predictions, reflecting the model’s ability to avoid false positives. Recall, also known as sensitivity, calculates the proportion of true positive predictions among all actual positive instances, indicating the model’s ability to capture all relevant cases. F1-score harmonizes precision and recall, providing a balanced assessment of model performance, particularly in imbalanced datasets. Additionally, the area under the ROC curve (AUC) evaluates the model’s ability to distinguish between classes across different thresholds, with higher values indicating better discrimination.
Following evaluation, iterative refinement of models and preprocessing techniques may be necessary to improve performance and address any shortcomings identified during testing. This iterative process involves adjusting model parameters, exploring alternative algorithms, or revisiting data preprocessing steps to enhance model robustness and generalization. Moreover, the selection of evaluation metrics should align with the specific objectives and requirements of the claims processing system, prioritizing metrics that directly impact operational efficiency, accuracy, and user satisfaction. By iteratively testing, evaluating, and refining models, organizations can iteratively enhance the effectiveness and reliability of their AI-driven claims processing systems, ensuring alignment with business goals and delivering tangible benefits to stakeholders.
Integration and deployment
Integration and deployment mark the culmination of efforts in building an AI-driven claims processing system, involving the seamless incorporation of trained models into the existing infrastructure and transitioning them to a production environment. This process typically entails developing application programming interfaces (APIs) or other interfaces to facilitate communication between the trained models and other components of the claims processing system. APIs serve as a gateway for requesting predictions or insights from the models, enabling easy integration with various applications, services, or user interfaces. Moreover, integration efforts may involve configuring the models to interact with existing workflows and systems, ensuring compatibility and interoperability with the broader ecosystem of tools and processes within the organization.
Deploying trained models into a production environment requires careful consideration of scalability, reliability, and performance to meet the demands of real-world usage. Organizations must ensure that the deployed models can handle incoming requests efficiently, scale seamlessly to accommodate increasing workloads, and maintain high availability to minimize disruptions to business operations. Additionally, robust monitoring and logging mechanisms are essential to track model performance, detect anomalies, and troubleshoot issues in real-time. Continuous monitoring and maintenance of deployed models enable organizations to proactively address any deviations from expected behavior and optimize model performance over time, ensuring consistent and reliable operation of the AI-driven claims processing system. By effectively integrating and deploying trained models, organizations can realize the benefits of automation, efficiency, and accuracy in claims processing, driving tangible value and competitive advantage in the marketplace.
Monitoring and maintenance
Monitoring and maintenance are essential aspects of managing an AI-driven claims processing system in production. Continuous monitoring involves tracking the performance of the deployed models, including metrics such as accuracy, throughput, latency, and resource utilization. Monitoring allows organizations to detect deviations from expected behavior, identify performance bottlenecks, and address issues promptly to ensure the system operates smoothly. Additionally, monitoring helps organizations assess the impact of model changes or updates on system performance and user experience, enabling informed decision-making regarding maintenance activities.
Maintenance of an AI-driven claims processing system involves various tasks to ensure optimal performance and adaptability to evolving requirements. One crucial aspect of maintenance is retraining models on new data to keep them up-to-date and maintain their effectiveness over time. This may involve periodically retraining models using fresh data to capture changing patterns, trends, or anomalies in claims processing. Furthermore, fine-tuning model parameters or architecture based on performance feedback or emerging insights can help enhance model accuracy and robustness. Incorporating feedback from users and stakeholders is also integral to maintenance, as it provides valuable insights into usability, functionality, and areas for improvement, guiding future development efforts and ensuring alignment with user needs and expectations.
By prioritizing monitoring and maintenance activities, organizations can proactively address issues, optimize performance, and maximize the value derived from their AI-driven claims processing system. Continuous monitoring allows organizations to identify and mitigate potential risks or issues before they escalate, ensuring uninterrupted operation and minimizing the impact on business operations. Moreover, ongoing maintenance activities enable organizations to adapt to changing requirements, address emerging challenges, and leverage opportunities for innovation and improvement, ultimately driving greater efficiency, accuracy, and effectiveness in claims processing operations.
By following these steps, organizations can build an AI-driven automated claims processing system that enhances efficiency, accuracy, and customer satisfaction while reducing operational costs and mitigating fraud risks.
Optimize Your Operations With AI Agents
Optimize your workflows with ZBrain AI agents that automate tasks and empower smarter, data-driven decisions.
Considerations for building an AI-powered claims processing system
Building an AI-driven claims processing system involves several key considerations to ensure its effectiveness, efficiency, and compliance. Here are some of the main considerations:
- Data quality and accessibility: Ensure access to high-quality and relevant data sources for training AI models and making informed decisions. Data should be accurate, complete, and representative of the claims processing domain. Take into account data privacy, security, and compliance requirements when accessing and handling sensitive claim data.
- Regulatory compliance: Adhere to regulatory requirements and industry standards governing claims processing, such as GDPR, HIPAA, PCI-DSS, and relevant insurance regulations. Ensure that the AI-driven system complies with legal and ethical guidelines for data privacy, fairness, transparency, and non-discrimination.
- Interpretability and explainability: Design AI models and decision-making algorithms that are interpretable and explainable to stakeholders. Provide transparency into how decisions are made, enabling users to understand the rationale behind automated decisions and detect biases or errors in the system.
- Scalability and performance: Build a scalable and high-performance infrastructure capable of handling large volumes of claims data and processing tasks efficiently. Design the system to accommodate growth in data volume, user traffic, and computational demand over time without compromising performance or reliability.
- Integration with existing systems: Integrate the AI-driven claims processing system seamlessly with existing IT infrastructure, legacy systems, and third-party applications. Ensure compatibility and interoperability with other systems used in the claims management lifecycle, such as policy administration systems, customer relationship management (CRM) platforms, and document management systems.
- User experience and usability: Prioritize user experience and usability in the design of the claims processing system’s interfaces and workflows. Ensure that the system is intuitive, user-friendly, and accessible to claims processors, administrators, and other stakeholders involved in the claims management process. Provide training and support to users to facilitate adoption and proficiency with the system.
- Robustness and reliability: Build robust and reliable AI models and algorithms that can handle noisy, incomplete, or inconsistent data gracefully. Implement error-handling mechanisms, fallback strategies, and resilience measures to mitigate the impact of system failures, data errors, or adversarial attacks on claims processing operations.
By addressing these considerations thoughtfully, organizations can build an AI-driven claims processing system that delivers value, improves efficiency, and enhances the overall claims management process. Simultaneously, ensuring compliance with regulatory requirements and meeting stakeholder expectations remains a priority.
AI in claims processing: Innovative trends
The current and imminent trends in claim processing automation showcase the most innovative developments shaping the industry’s landscape:
Digital customer experience: Insurers find themselves at a pivotal moment to cultivate trust with customers through proactive enhancements in engagement, providing a seamless, personalized experience within the dynamic digital landscape. This shift is intricately tied to the escalating integration of AI, data analytics, and artificial intelligence across various facets of insurance.
Health and well-being convergence: AI plays a pivotal role in health and well-being convergence by enabling personalized care through data-driven insights. It facilitates predictive analytics, allowing healthcare providers to anticipate individual needs and recommend tailored wellness treatment plans. Additionally, AI-driven platforms contribute to cost reduction by optimizing operational efficiencies and enhancing the overall quality of care, aligning with the industry shift towards preventive healthcare.
Advanced analytics and personalization: The utilization of advanced analytics and personalization is on the rise, empowering insurers to elevate customer engagement, streamline processes, and stimulate revenue growth. This momentum is fueled by insurers leveraging customer behavior and preference data to deliver tailored solutions.
Inclusive insurance: AI plays a pivotal role in expanding insurance accessibility to marginalized segments by automating underwriting processes and enabling personalized and affordable coverage. Machine learning algorithms help assess risk more accurately, allowing insurers to offer tailored products that cater to the specific needs of low-income households, migrants, and small businesses. This technological advancement contributes to closing the protection gap and fostering financial stability in underserved markets.
Ecosystem collaboration: This has become imperative in response to the rapidly changing insurance industry landscape. Insurers are increasingly seeking agility, customer-centricity, and operational efficiency, leading to a surge in strategic partnerships, cross-industry collaborations, and new ecosystems. These endeavors leverage emerging technologies and data-driven insights by AI to deliver superior outcomes.
Endnote
The potential of AI in claims processing is paramount for insurers striving to stay ahead in an ever-evolving industry landscape. As AI technology advances, its ability to streamline claims processing, fortify fraud detection, and refine risk assessment becomes increasingly indispensable. The imperative lies in capitalizing on AI’s current capabilities and recognizing its ongoing evolution, which promises higher value with each passing day.
Insurers must acknowledge that the claims function is pivotal in ensuring customer-centricity and long-term sustenance. Embracing AI-driven solutions in claims management is not merely a technological advancement but a strategic imperative to meet the changing demands of policyholders. Integrating AI promises efficiency gains and is key to delivering a superior and faster claims experience, enhancing overall customer satisfaction. Building an AI-powered claims processing system requires careful consideration of factors such as data privacy, security, regulatory compliance, and ethical implications. Organizations must ensure that AI systems are transparent, accountable, and aligned with legal and ethical guidelines to foster trust and mitigate risks associated with algorithmic decision-making. Additionally, collaboration between data scientists, domain experts, and stakeholders is crucial to designing AI solutions that address specific business needs, enhance operational efficiency, and deliver tangible value in claims processing operations. As the insurance landscape navigates the waves of innovation, those who adeptly harness the potential of AI in claims processing will find themselves better equipped to navigate the complexities of the modern market.
Experience the future of insurance with AI-powered claims management. Contact LeewayHertz’s experts for robust AI solutions and consulting that will help you optimize operational efficiency, reduce costs, and elevate customer satisfaction.
Start a conversation by filling the form
All information will be kept confidential.