AI in debt collection: Use cases, benefits, development and future trends
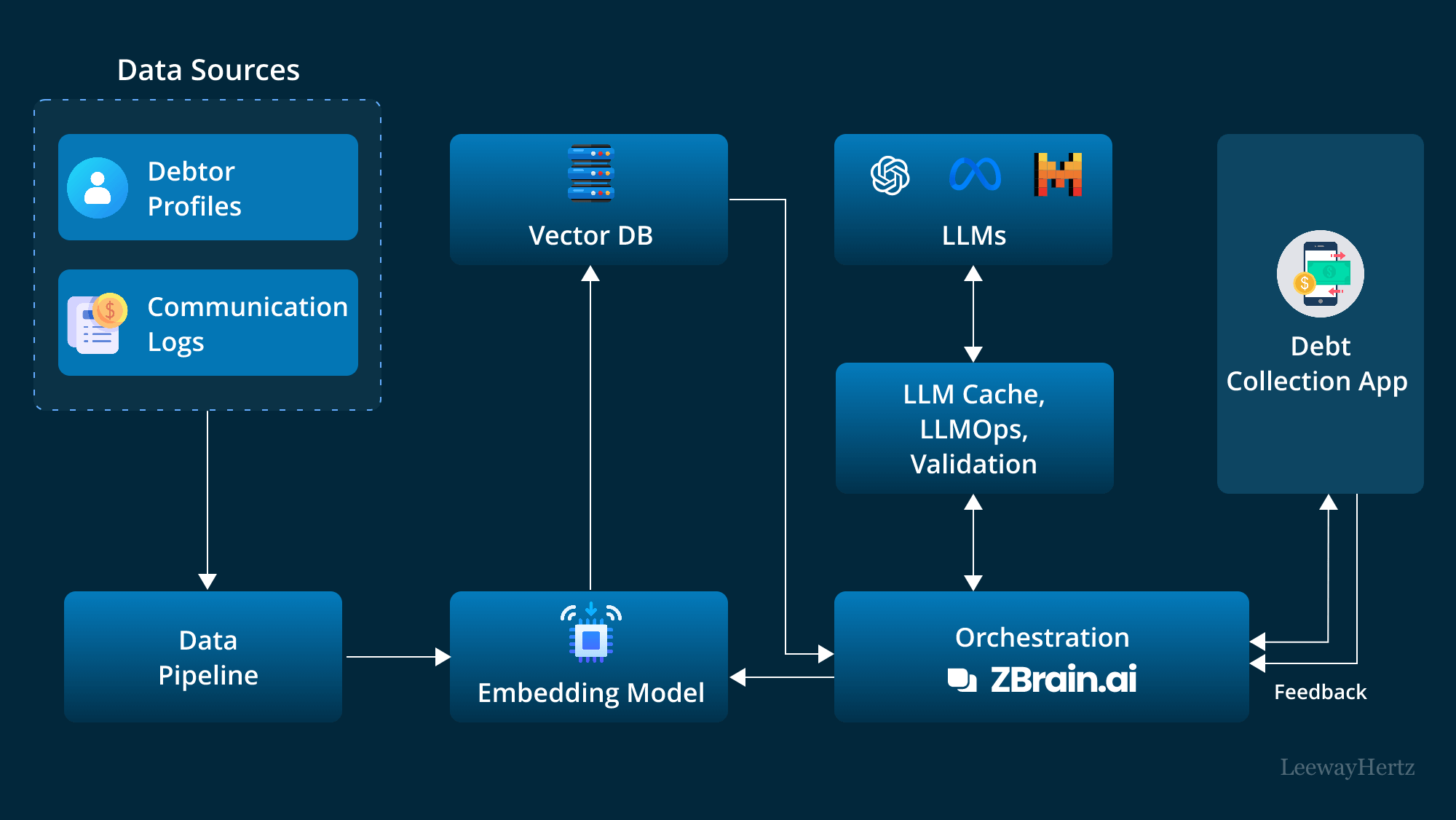
Debt collection, a fundamental aspect of financial services, has traditionally relied on manual processes, often leading to inefficiencies, errors, and strained customer relationships. However, the emergence of artificial intelligence (AI) promises to redefine debt collection practices, offering a paradigm shift that combines data-driven insights, automation, and personalized communication.
In the complex and often contentious world of debt collection, agencies increasingly turn to artificial intelligence to streamline operations, enhance customer interactions, and ensure compliance with regulatory standards. As businesses seek more efficient and less intrusive methods of recovering debts, AI emerges as a powerful tool that transforms traditional practices into more sophisticated, precise, and user-friendly processes.
According to the TransUnion report in 2023, 11% of debt collection companies, also known as third-party collection (3-PC) companies, already use AI in their tasks. The report further details that:
- 58% of these companies use AI to predict payment outcomes, such as a person’s ability or willingness to pay a debt.
- 56% apply AI to segment customers and profile them for various workflows.
- 46% leverage AI to anticipate consumer behavior.
- 47% utilize AI to recommend communication strategies.
These statistics not only demonstrate the increasing integration of AI in debt collection but also provide a crucial context, showcasing the technology’s extensive capacity to improve efficiency and humanize interactions within the industry. In this article, we will discuss the role of AI in debt collection, exploring its applications, architecture, benefits, AI-powered debt collection process and future trends in detail.
- What is debt collection?
- Challenges involved in traditional debt collection processes
- The role of AI in enhancing debt collection efficiency
- How does AI for debt collection work?
- Applications of AI in debt collection
- Generative AI applications in debt collection
- Optimizing debt collection workflows with generative AI
- Why is LeewayHertz the right choice for building AI debt collection solutions?
- LeewayHertz’s AI development services for debt collection
- Benefits of AI in the debt collection process
- AI in debt collection: future trends
What is debt collection?
Debt collection is a crucial process in the financial landscape, initiated when borrowers default on their obligations, such as credit card balances or loans. This typically begins when payments are at least 30 days overdue, prompting lenders or specialized third-party agencies to step in and recover the owed amounts. The practice encompasses various debt types, including medical bills, auto loans, personal loans, credit card debts, and unpaid utilities or phone bills.
The methods and strategies of debt collection can vary significantly depending on the company managing the debt. Often, third-party debt collectors are either hired by the original creditor on a commission basis or purchase the debt at a fraction of its value, aiming to recover as much as possible. During the collection process, agencies employ multiple tactics—from sending written notices to making phone calls—to ensure compliance and settlement of outstanding debts.
Challenges involved in traditional debt collection processes
Traditional debt collection processes face several challenges that can affect both the efficiency of collections and the relationships between creditors and debtors. Here are some key difficulties associated with conventional methods:
- Inefficiency and high operational costs: Traditional debt collection is often labor-intensive, relying heavily on manual tasks such as phone calls, sending letters, and managing large records. These methods consume considerable time and resources and can increase the likelihood of human error, leading to additional costs and inefficiencies.
- Poor debtor engagement: The conventional approach to debt collection, characterized by frequent phone calls and standard demand letters, can be intrusive and stressful for debtors. Such practices can strain debtor relations and result in low engagement rates. Debtors often feel harassed, leading to a refusal to cooperate, further complicating the collection process.
- Regulatory compliance challenges: Debt collection is heavily regulated, with laws that vary significantly by region and are subject to change. Staying compliant with these regulations can be daunting for collection agencies, as non-compliance can result in hefty fines and legal issues. Traditional methods may struggle to adapt quickly to new or amended regulations, putting the agencies at risk.
- High default and dispute rates: Traditional methods may not effectively address the underlying reasons for non-payment, such as financial hardship or disputes over the debt validity. This can lead to high default rates and increased disputes, which are time-consuming and costly to resolve.
- Data management and privacy issues: Managing and securing the vast amount of personal and financial data in debt collection poses significant challenges. Traditional systems can be susceptible to security vulnerabilities, compromising debtor privacy and exposing the agency to legal and reputational hazards.
- Limited customization and scalability: Traditional debt collection practices often lack the flexibility to tailor strategies to individual debtor situations, leading to a one-size-fits-all approach that may not be effective for all debtors. Additionally, scaling these methods to handle larger volumes or diverse types of debt without increasing costs or compromising quality can be difficult.
- Resource-intensive practices: The deployment of multiple resources in terms of employees, remuneration, calling expenses, and physical mediums for information storage make traditional debt collection an expensive venture. These resource-intensive practices are inefficient and unsustainable in the long run.
- Administrative burdens: Traditional debt collection requires collectors to spend substantial time on administrative tasks such as processing documentation, research, reports, and archiving information. These tasks, inherently time-consuming, often distract from more strategic engagement efforts.
- Achieving impartiality and fair treatment: Ensuring impartial treatment in repayments and maintaining an ethical approach in collections are significant challenges. Traditional methods, which might lack flexibility and transparency, can lead to perceived or real bias in handling different debtors.
Addressing these challenges is crucial for improving the effectiveness and humaneness of debt collection practices. With the advent of AI innovations, many of these issues are being addressed, leading to more sophisticated, efficient, and fair debt recovery strategies.
The role of AI in enhancing debt collection efficiency
AI transforms the debt collection industry by mitigating traditional challenges and streamlining operations. Here’s how AI reshapes debt collection processes at both the administrative and client-side operations front.
At the back end, AI-powered solutions are transforming administrative and processing tasks. AI debt collection systems can read, interpret, and analyze inquiries related to invoices, payment delays, objections, outstanding debts, payment receipts, address changes, and more. They can also validate and archive essential documents like customer information, account statements, and credit reports. By automating these tasks, AI speeds up processes and enhances accuracy, boosting employee confidence and satisfaction. Furthermore, AI utilizes income, credit scores, and net worth data to make informed business decisions, optimizing the collection strategy.
In client-side operations, AI technology excels in detecting and addressing customer needs. It facilitates sending notices for repayment postponements and deferrals tailored to individual circumstances. AI’s ability to identify and analyze patterns in debtors’ financial behaviors allows agencies to personalize communications, making them more effective and less intrusive. AI also serves as a virtual customer assistant, handling simpler inquiries and escalating more complex cases to human agents. This saves time for both employees and customers and increases overall productivity and efficiency.
AI is a transformative solution for the debt collection process, enhancing the entire ecosystem by driving efficiencies, reducing risks, and fostering better relationships between creditors and debtors.
Optimize Your Operations With AI Agents
Optimize your workflows with ZBrain AI agents that automate tasks and empower smarter, data-driven decisions.
How does AI for debt collection work?
AI transforms debt recovery by automating routine tasks, predicting debtor behavior, and personalizing communication strategies to increase collection effectiveness. Incorporating AI into debt collection processes leverages advanced Large Language Models (LLMs) and connects them with an organization’s unique data repository. This innovative approach streamlines data analysis, enhances insight generation, and optimizes client interaction, transforming traditional debt collection methodologies.
The solution architecture leverages various components to streamline the debt collection process. Here’s a step-by-step breakdown of AI in debt collection solution architecture:
- Data sources: The process starts with compiling extensive data relevant to debt collection, which includes:
- Debtor financial profiles: Detailed information about debtors’ financial situations, including income, employment status, and credit history.
- Debt records: Data on the amount and type of debt, duration, and repayment history.
- Communication logs: Historical data of past debtor interactions, including phone calls, emails, and other correspondence.
- Behavioral data: Insights into debtor behaviors and payment patterns that can guide personalized collection strategies.
- Regulatory compliance data: Up-to-date information necessary to comply with collection laws and regulations.
- Data pipelines: Data from the sources listed above are funneled through data pipelines that manage the ingestion, cleaning, and structuring of data, preparing it for deeper analysis.
- Embedding model: The prepared data is then processed by an embedding model. This model transforms the textual data into numerical representations called vectors that AI models can understand. Popular models include those from OpenAI, Google, and Cohere.
- Vector database: The generated vectors are saved in a vector database that facilitates efficient querying and retrieval. Notable examples of vector databases include Pinecone, Weaviate, and PGvector.
- APIs and plugins: Essential APIs and plugins like Serp, Zapier, and Wolfram integrate various system components, enabling tasks such as additional data access or specific operational functions.
- Orchestration layer: The orchestrating layer is critical in managing the workflow. ZBrain is an example of this layer that simplifies prompt chaining, manages interactions with external APIs by determining when API calls are required, retrieves contextual data from vector databases, and maintains memory across multiple LLM calls. Ultimately, this layer generates a prompt or series of prompts that are submitted to a language model for processing. The role of this layer is to orchestrate the flow of data and tasks, ensuring seamless coordination across all components in the debt recovery architecture.
- Query execution: The data retrieval and generation process begins when the user submits a query to the debt collection app. This query concerns anything relevant to debt recovery, payment arrangements, or debtor profiles.
- LLM processing: Once received, the app transmits the query to the orchestration layer. This layer retrieves relevant data from the vector database and LLM cache and sends it to the appropriate LLM for processing. The choice of LLM depends on the nature of the query.
- Output: The LLM generates an output based on the query and the data it receives. The output can take various forms, such as communication strategies, repayment plans, or risk assessments.
- Debt collection app: AI-generated insights and recommendations are delivered through the dedicated app designed for debt collection, ensuring ease of access and usability for collection agents.
- Feedback loop: User feedback on the LLM’s output is another important aspect of this architecture. The feedback is used to improve the accuracy and relevance of the LLM output over time.
- Agent: AI agents step into this process to address complex problems, interact with the external environment, and enhance learning through post-deployment experiences. They achieve this by employing advanced reasoning/planning, strategic tool utilization, and leveraging memory, recursion, and self-reflection.
- LLM cache: Tools like Redis, SQLite, or GPTCache are used to cache frequently accessed information, accelerating the response time of the AI system.
- Logging/LLMOps: Throughout this process, LLM operations (LLMOps) tools like Weights & Biases, MLflow, Helicone and Prompt Layer help log actions and monitor performance. This ensures the LLMs operate at peak efficiency and evolve consistently through ongoing feedback mechanisms.
- Validation: A validation layer is employed to validate the LLM’s output. This is done through tools like Guardrails, Rebuff, Guidance, and LMQL to ensure the accuracy and reliability of the information provided by the LLM.
- LLM APIs and hosting: LLM APIs and hosting platforms are essential for executing debt recovery tasks and hosting the application. Depending on the requirements, developers can select from LLM APIs, such as those offered by OpenAI and Anthropic or opt for open-source models. Similarly, they can choose hosting platforms from cloud providers like AWS, GCP, Azure, and Coreweave or opt for opinionated clouds like Databricks, Mosaic, and Anyscale. The choice of LLM APIs and cloud hosting platforms depends on the project’s needs.
This structured architecture solution for AI in debt collection provides a detailed overview of how AI facilitates debt recovery, leveraging various data sources and technological tools to generate accurate and actionable insights. AI automates many tasks associated with debt recovery, boosts operational efficiency, and enhances the effectiveness of collection strategies.
Applications of AI in debt collection
In this section, we will explore the detailed applications of AI in debt collection.
Automated default risk analytics
Federal Reserve data indicates that credit card balances surged by $50 billion in the fourth quarter of 2023 and reached a new peak of $1.13 trillion. In this financial landscape, AI plays a pivotal role in transforming debt collection processes. AI systems monitor real-time payment transactions to identify overdue debts and notify collectors about collectible accounts, thereby streamlining the initial debt recovery steps.
AI significantly enhances the ability to predict payment defaults by analyzing extensive data sets, including borrower credit history, delinquency records, payment behavior, and social media interactions. By creating detailed risk profiles for each debtor, AI identifies high-risk accounts and flags them for prioritized attention. This targeted approach concentrates efforts on areas most likely to yield results, boosting overall efficiency and fostering a proactive risk management strategy.
Debt surveillance and prioritization
AI in debt collection helps prioritize debts based on multiple factors such as the debtor’s risk profile, the value of the debt, how long the debt has been outstanding, and the total amount due. Debts are then intelligently assigned to collectors based on these priorities, optimizing the allocation of resources. This enables collectors to focus their efforts more effectively on accounts that are most likely to yield returns.
Propensity modeling and analytics
According to the Commercial Collection Agency’s study, the chances of collecting a full payment on a delinquent account fall to 68.9% after three months, 51.3% after six months, and 21.4% after one year. AI-driven propensity models predict which customers are most likely to repay their debts. This involves analyzing customer data and using machine learning algorithms to identify patterns and predict future behaviors. By focusing on customers with a higher propensity to pay, collection agencies can allocate their resources more effectively, leading to increased efficiency and higher recovery rates.
Collection planning
AI-driven analytics, particularly through predictive analytics, enable the identification of potential defaulters in financial contexts. Through data-driven approaches, collection efforts can be prioritized focusing on high-value or high-risk accounts, making the collection process more efficient and effective. Additionally, AI can provide personalized strategy suggestions tailored to individual borrower behaviors and circumstances, significantly improving the chances of successful debt recovery. The value delivered through these innovations includes streamlined and more accurate collection planning, minimized risk of non-performing loans, and an enhanced customer experience.
Collection execution
The automation of payment reminder generation and distribution is transformed by AI, which can craft and send personalized textual and voice reminders, drastically reducing the need for manual intervention. AI’s capability to instantly process debtor responses from multiple channels and automate subsequent communications maintains consistent engagement with borrowers. Moreover, AI systems are instrumental in monitoring compliance with internal and legal debt collection requirements, ensuring all activities are compliant and mitigating associated risks. This level of automation and compliance monitoring eliminates manual collection efforts and significantly reduces loan payment defaults.
Collection optimization
AI can continuously monitor and analyze key metrics such as response rates, delinquency, and recovery rates. This real-time analysis helps assess the effectiveness of existing collection strategies and offers intelligent recommendations for improvements. AI also enables dynamic adjustments to planned collection activities based on real-time data and emerging trends, enhancing the collection efforts’ efficiency and effectiveness. The resultant value is an improved planning of personalized collections, leading to increased borrower response rates and a higher probability of timely debt repayment.
AI-enhanced financial scoring
AI-enhanced financial scoring transforms debt collection by streamlining decision processes and tailoring strategies to individual debtor profiles. These systems analyze a broad spectrum of data to evaluate debt repayment capabilities and optimize collection efforts.
- Enhanced credit scoring: AI analyzes credit history alongside real-time behavioral data to comprehensively assess a debtor’s ability to repay. This rapid evaluation helps make informed decisions about whether to extend credit.
- Strategic decision-making: AI-driven models predict the likelihood of payment defaults, enabling collectors to prioritize cases and allocate resources where they are most effective, thus improving recovery rates.
- Tailored collection strategies: AI insights enable collectors to customize their outreach, selecting the most effective communication methods and timing, whether through phone calls, texts, or digital platforms, to maximize debtor engagement and response.
- Seamless system integration: AI seamlessly integrates with existing collection systems, upgrading them with advanced capabilities to replace outdated procedures. This integration not only boosts efficiency but also enhances the accuracy of the debt collection operations.
By implementing AI in financial scoring, debt collection agencies can make quicker, more accurate decisions that align with dynamic market conditions and debtor situations, significantly enhancing the effectiveness of their collection strategies.
Automated debtor outreach
AI-powered chatbots and Interactive Voice Response (IVR) bots distribute personalized textual and voice payment reminders. These are sent across various channels, including email, messaging apps, SMS/MMS, customer portals, and VoIP systems, ensuring extensive coverage and convenience for the debtor.
Personalized communications
AI-powered tools enhance customer communication personalization. By analyzing individual debtor data, AI enables the creation of customized messages that resonate more effectively with each debtor. This approach increases the chances of successful engagement and contributes to a better overall customer experience. NLP techniques analyze written and spoken communications to extract meaningful insights about debtor sentiment, intent, and reliability. This information is crucial in determining the approach and tone of subsequent communications.
Automated debtor interaction
In the debt collection process, several AI-powered tools such as chatbots, Intelligent Virtual Assistants (IVAs), and automated phone systems work collaboratively to enhance debtor interactions. These tools are integral in handling various aspects of communication and engagement with debtors:
- Chatbots and Intelligent Virtual Assistants (IVAs): These tools are crucial in modernizing the debt collection process. They engage debtors through digital platforms, efficiently managing inquiries and providing personalized responses based on detailed debtor data and previous interactions. They are designed to handle multiple inquiries simultaneously, significantly reducing operational costs and providing a non-confrontational platform for debtors.
- Handles multiple debtor inquiries simultaneously, increasing operational efficiency.
- Programmed to comply with industry regulations, minimizing legal risks.
- Tailors communications based on debtor profiles, enhancing engagement and recovery success.
- Operates seamlessly across multiple digital channels such as text/SMS, Facebook Messenger, WhatsApp, etc.
- Adapts to fluctuating demand levels without the need for additional staff.
- Reduces operational costs by automating routine debtor interactions.
- Automated phone systems: AI-enhanced phone systems transform debt collection by leveraging voice recognition and machine learning to handle calls autonomously. They deliver personalized payment reminders and guide debtors through payment processes, adapting interactions based on debtor history and behavior. This results in more effective communication, encouraging timely payments and improving customer experience.
- Data-driven personalization: Across chatbots, IVAs, and phone systems, AI analyzes debtor profiles to customize communication. This involves adjusting the dialogue based on the debtor’s history, preferences, and optimal engagement times, ensuring relevance and effectiveness in each interaction.
- Multi-channel coordination: AI integrates interactions across various channels—text, voice, or online portals—to provide a consistent and unified communication experience. This ensures that debtors receive the same level of service and information across all points of contact.
- Self-service portals: AI enhances self-service options by acting as a digital assistant within portals. These AI tools guide customers through the process, answering queries and facilitating payment arrangements autonomously, which enhances user experience and operational efficiency.
These functionalities are crucial in streamlining the process of engaging with debtors and ensuring efficient management of interactions across various communication channels. By utilizing these AI-powered tools, debt collection agencies can maintain a high standard of interaction tailored to meet the needs and circumstances of each debtor.
Automated processing of debtor responses
AI-powered systems integrate Optical Character Recognition (OCR) and Natural Language Processing (NLP) to automate the capture and processing of debtor responses across various communication channels. By seaml+9essly handling inputs from emails, text messages, and documents, this technology ensures efficient tracking and management of payment agreements and other debtor communications. This robust automation enhances the accuracy and speed of data handling, enabling more effective follow-up actions and reducing the administrative burden on collection teams.
Intelligent document processing
AI in Intelligent Document Processing (IDP) helps automate the extraction, understanding, and processing of data from various documents. This powerful technology enhances accuracy and efficiency across numerous applications, transforming how data-heavy tasks are managed in debt collection.
- Full-text search for skip tracing: Skip tracing is a process used primarily by debt collectors, private investigators, and other professionals who need to locate a person whose contact details are not immediately known. AI in document processing simplifies the skip tracing process by allowing debt collectors to efficiently search through vast numbers of documents to locate debtor information quickly. This process uses AI to scan and read documents, identifying keywords and data points essential for tracing individuals who have moved or changed their contact details.
- Automated data extraction: AI-powered OCR (Optical Character Recognition) and data extraction technologies streamline processing documents such as court orders, credit reports, and financial statements. By automating the extraction of critical information, IDP minimizes manual data entry errors and speeds up the decision-making process.
- Document classification and parsing: This function categorizes and organizes documents automatically, saving time and reducing the cognitive load on agents. By classifying documents based on their content and layout, AI helps quickly direct them to the appropriate processing channels.
Automated compliance adherence
AI supports debt collection activities by verifying all actions against internal policies and legal regulations such as the FDCPA, CFPB, and TCPA. The system immediately identifies any areas of non-compliance and reports them to the responsible parties, enabling swift corrective actions.
Speech analytics
AI is also used to analyze recorded calls between agents and customers. This technology identifies keywords and phrases, emotions, tone, and compliance with legal regulations. Insights gained from speech analytics are used to improve agent performance, ensure compliance, and refine collection strategies.
Automated decisioning systems
Automated decisioning systems powered by AI have transformed debt collection practices by enhancing both the efficiency and effectiveness of interactions with debtors. These systems analyze extensive data to streamline numerous collection processes:
- Optimal contact timing: AI determines the most effective times to initiate contact based on debtor activity patterns and past responsiveness, improving the likelihood of successful communication.
- Channel selection: The system selects the most appropriate communication channels, such as email, SMS, or phone, according to individual debtor preferences, thereby boosting engagement and response rates.
- Optimization of the dunning process: AI expedites the dunning process by identifying overdue invoices and initiating recovery procedures. It employs predictive analytics to refine the timing and methods of communication, shortening the overall debt collection cycle.
- Personalized payment solutions: AI proposes customized payment arrangements tailored to each debtor’s financial situation and payment history, enhancing the feasibility of repayment plans.
- Debt recovery decisions: The system continuously monitors debtor behavior to detect signs of potential payment defaults or shifts in financial status, facilitating timely and appropriate interventions such as loan restructuring or proactive debt enforcement.
- Regulatory compliance and ethical practices: AI ensures that all procedures and interactions adhere to regulatory standards like the FDCPA, promoting ethical practices and maintaining positive debtor relations.
Integrating these AI-driven capabilities enhances the strategic and humane approach to debt collection, ensuring that operations are not only efficient but also aligned with the best interests of both creditors and debtors.
Optimize Your Operations With AI Agents
Optimize your workflows with ZBrain AI agents that automate tasks and empower smarter, data-driven decisions.
Generative AI applications in debt collection
Generative AI redefines the debt collection landscape with various capabilities that enhance efficiency, accuracy, and customer engagement. Here’s how generative AI is making a significant impact in debt recovery:
- Real-time insights: Generative AI offers immediate data-driven insights during debtor interactions, helping agents provide accurate recommendations and strategies.
- Identifying behavioral patterns: Generative AI predicts debtor behaviors by analyzing past interactions and current financial data. It can analyze historical data to identify trends or anomalies in debtor behavior, such as financial hardships or recurring billing errors.
- Profiling and segmentation: Generative AI enhances debtor profiling and segmentation by analyzing risk levels, payment histories, and demographics. This targeted approach allows debt collection agencies to tailor strategies for individual debtor groups, improving efficiency and recovery rates. By fine-tuning interactions based on debtor profiles, agencies can optimize resource allocation and enhance customer experience, making collection efforts both effective and considerate.
- Content generation: This application of generative AI produces communication material such as emails, negotiation scripts, and payment reminder texts tailored to the debtor’s profile, increasing the effectiveness of such interactions.
- Synthetic data generation: Generative AI is crucial in synthesizing data to enhance training models without compromising real customer privacy. This process generates realistic, anonymized data that mimics actual debtor behaviors and patterns, which is essential for testing and improving debt collection strategies. By expanding the dataset, AI systems can learn and adapt more effectively, leading to more refined risk assessments and segmentation techniques.
- Empathetic communication: Generative AI guides the tone and style of communications to be more debtor-friendly, focusing on empathy and understanding.
- Consistent communication across platforms: Generative AI synchronizes debtor interactions across all communication channels, ensuring a seamless debtor experience.
Generative AI not only optimizes the operational aspects of debt collection but also significantly improves the interaction with debtors, ensuring a more humane and effective debt recovery process.
Optimizing debt collection workflows with generative AI
Generative AI is transforming the debt collection process by automating key tasks, enhancing decision-making, and streamlining workflows. From gathering debtor information to negotiating payment plans, GenAI technology improves efficiency, accuracy, and compliance across all stages of the debt collection lifecycle. Below are key stages of the debt collection process, where generative AI plays a pivotal role in automating substeps and improving operational effectiveness.
1. Debt assessment and segmentation
Steps involved | Sub-steps | Role of generative AI |
---|---|---|
Gather debtor information |
|
|
Debt verification and validation |
|
|
Segmentation of debtors |
|
|
Case assignment and prioritization |
|
|
Collection strategy |
|
|
2. Initial communication
Steps involved | Sub-steps | Role of generative AI |
---|---|---|
Customer communication and engagement |
|
|
Monitor engagement |
|
|
Payment plan proposal |
|
|
3. Repayment negotiation and agreement
Steps involved | Sub-steps | Role of generative AI |
---|---|---|
Assess financial capability |
|
|
Negotiation and agreement |
|
|
Payment plan approval and monitoring |
|
|
4. Payment tracking
Steps involved | Sub-steps | Role of generative AI |
---|---|---|
Payment tracking and allocation |
|
|
Agreement monitoring |
|
|
5. Resolution and closure
Steps involved | Sub-steps | Role of generative AI |
---|---|---|
Debt write-off |
|
|
Review write-off criteria |
|
|
Debt closure |
|
|
Generative AI optimizes debt collection by automating payment tracking, write-offs, and resolutions while analyzing debtor behavior and ensuring legal compliance. This enhances efficiency and accuracy and improves debtor communication and negotiation outcomes, allowing teams to focus on strategic tasks.
Why is LeewayHertz the right choice for building AI debt collection solutions?
LeewayHertz utilizes its expertise in technology consulting, AI, and custom software development to transform traditional debt collection practices. Our approach integrates advanced technologies, enhancing both the efficiency and effectiveness of AI debt collection strategies. Our expert team, proficient in machine learning and natural language processing, focuses on enhancing efficiency and security in financial operations. Here’s why LeewayHertz is a preferred partner for integrating AI into debt collection:
- Consulting and strategy development: LeewayHertz is an AI development company providing solutions for debt collection. We offer expert consulting to tailor AI solutions according to each client’s specific needs, thereby optimizing debt collection strategies. This approach likely improves operational efficiency and effectiveness in debt collection processes.
- Custom AI solutions: With a deep understanding of the financial sector’s unique challenges, LeewayHertz designs custom AI tools that enhance debtor profiling, risk assessment, and collection prioritization. Our solutions include advanced analytics for predicting debtor behavior, and interactive systems for personalized debtor communication. These solutions enable agencies to make faster, data-driven decisions to improve recovery rates.
- Application development: LeewayHertz specializes in designing intuitive applications that provide debt collection agents with real-time insights, predictive analytics, and actionable debt recovery strategies. These applications improve operational efficiency and are integrated smoothly with clients’ existing systems.
- Security-first approach: Recognizing the critical importance of data security in finance, LeewayHertz integrates robust security measures into every AI solution. This ensures that all data handling and processing comply with the highest security standards, protecting sensitive debtor information and maintaining trust.
- Client-centric development: LeewayHertz begins each project with an in-depth consultation to understand specific client needs and objectives in debt collection. This approach ensures that every AI solution precisely aligns with client goals, enhancing targeted collection efforts and improving recovery rates.
- End-to-end AI implementation: LeewayHertz offers a complete AI development service that covers everything from initial consulting to full integration within existing debt collection systems. Our implementation process integrates seamlessly, ensuring smooth incorporation of all aspects, from data handling to security and governance. This holistic approach ensures that AI solutions are not only seamlessly integrated but also rigorously tested and continuously refined to effectively address the evolving needs of the debt collection industry.
By leveraging LeewayHertz’s AI expertise, debt collection agencies can not only improve operational efficiencies but also foster a more strategic and humane approach to debt recovery. Our innovative solutions are tailored to meet the complex demands of modern debt collection, making LeewayHertz a vital partner in transforming financial practices with modern technology.
LeewayHertz’s AI development services for debt collection
At LeewayHertz, we design customized AI solutions specifically for debt collection organizations. Our strategic AI/ML consulting empowers debt collectors to leverage AI for more effective decision-making, improved debtor engagement, and optimized recovery strategies.
Our expertise in developing Proof of Concepts (PoCs) and Minimum Viable Products (MVPs) enables debt collection firms to experience the tangible benefits of AI tools in real-world scenarios. This ensures that our solutions are effective and tailored to meet the unique demands of the debt collection industry.
Our work in generative AI also transforms routine tasks such as report generation and data management, automating these processes to free up debt collectors for more strategic and high-value activities.
By fine-tuning large language models to the nuances of debt collection terminology and debtor interactions, LeewayHertz enhances the accuracy and relevance of AI-driven communications and analyses.
Additionally, we ensure these AI systems integrate seamlessly with existing technological infrastructures, enhancing operational efficiency and decision-making in debt collection firms.
Our AI solutions development expertise
AI solutions development for debt collection typically involves creating systems that enhance decision-making, automate routine tasks, and optimize debtor engagement. These solutions integrate key components such as data aggregation technologies, which compile and analyze debtor information from diverse sources. This comprehensive data foundation supports predictive analytics capabilities, allowing for forecasting debtor behavior and improving recovery strategies.
Additionally, machine learning algorithms tailor communication strategies to individual debtor profiles, ensuring that each interaction considers the debtor’s unique circumstances and payment likelihood. These solutions often cover debt recovery management, risk assessment, regulatory compliance, and debtor relationship management.
AI solutions in debt collection aim to maximize recovery rates, improve operational efficiency, and enhance the debtor experience.
AI agent/copilot development for debt collection
LeewayHertz builds custom AI agents and copilots that enhance various debt collection operations, enabling agencies to save time and resources while facilitating faster decision-making. Here is how they help:
Client engagement:
- Analyze debtor data and past interactions to provide personalized repayment advice and strategies.
- Automate routine communication tasks like payment reminders and negotiation follow-ups.
- Offer 24/7 virtual assistance to address debtor queries regarding payment options and debt resolution.
Compliance and risk monitoring:
- Automate regulatory document analysis, ensuring agencies comply with evolving debt collection regulations.
- Monitor debt portfolios for compliance with predefined collection rules and policies.
- Automate documentation and reporting processes, ensuring accurate record-keeping and audit trails.
Fraud detection:
- Monitor debtor transactions for predefined patterns or rules associated with potential fraudulent activities, such as identity theft or payment evasion.
- Flag suspicious debtor behaviors based on predefined criteria or models, aiding in early fraud detection and prevention.
Customer segmentation and targeting:
- Analyze debtor data to segment customers based on predefined criteria (e.g., payment history, debt amount, responsiveness).
- Identify potential debt recovery opportunities based on debtor segments, optimizing collection strategies for higher success rates.
AI agents/copilots don’t just increase the efficiency of operational processes but also significantly enhance the quality of debt collection service and strategic decision-making. By integrating these advanced AI solutions into their infrastructure, debt collection agencies can achieve a significant competitive advantage, navigating the complex debt recovery landscape with innovative, efficient, and reliable AI-driven tools and strategies.
Benefits of AI in the debt collection process
The table summarizes the contribution of different AI-powered debt collection practices.
Debt collection practice | Function | Impact | Technology used | Benefit to agencies |
---|---|---|---|---|
Behavioral Segmentation | Predicting customer reactions to specific contact strategies | Refines customer outreach and increases engagement | Machine Learning, Data Analytics | Higher engagement and conversion rates |
Skip Tracing | Locating elusive debtors by integrating various data sources and employing advanced search algorithms | Improves the effectiveness of locating debtors and reduces time spent on tracing | Data Integration, Machine Learning | Increased recovery rates and cost savings through automated debt collections |
Contact Strategies Optimization | Determining the most effective method and timing for contacting each individual debtor | Enhances communication efficiency and debtor responsiveness | Machine Learning, Predictive Analytics | Improved customer reach and response rates |
Treatment Options Customization | Creating flexible repayment solutions tailored to individual financial situations | Increases likelihood of debt repayment and customer satisfaction | Artificial Intelligence, Custom Software | Higher repayment success and customer retention |
Frontline Capabilities Enhancement | Providing decision support tools and customer interaction guidance for effective negotiation | Helps build stronger rapport with debtors | Decision Support Systems, Interactive Guides | More effective debtor negotiations and solutions |
Performance Management | Providing real-time performance insights for better coaching and team management | Enhances staff performance and streamlines management processes | Analytics, Performance Monitoring Tools | Better team performance and efficient operations |
Organizational Structure Adaptation | Reorganizing collection operations to align with value-at-risk segmentation | Aligns resources more strategically and focuses on high-value collections | AI Planning, Resource Management | Optimized allocation of resources and improved ROI |
Optimize Your Operations With AI Agents
Optimize your workflows with ZBrain AI agents that automate tasks and empower smarter, data-driven decisions.
- Improved pay-back rates: By leveraging predictive analytics and risk assessment, AI helps financial institutions enhance their debt recovery rates. This technology enables a deeper understanding of customer behavior, leading to tailored outreach strategies that can significantly increase pay-back rates and boost profits.
- Reduction in insolvencies: AI preemptively identifies at-risk profiles, allowing banks to assess creditworthiness before issuing loans. This proactive approach prevents lending to high-risk individuals, reducing the incidence of insolvencies and enabling more stable financial operations.
- Automated communication: AI optimizes communication by automating outreach and ensuring messages reach customers through the most effective channels and times. For instance, AI can analyze past customer interactions to determine the optimal time and content for sending emails. This approach ensures that promotional or reminder emails are sent when customers are most likely to read and respond, thus improving engagement rates and potentially increasing sales or prompt payments.
- Automated customer coverage: AI transforms debt collection by automating significant portions of customer interactions. AI assistants efficiently handle routine tasks like identification and payment notifications and manage the entire collection process through custom call flows. This automation streamlines operations and enhances debtor engagement and recovery rates through empathetic and tailored communication.
- Personalized communication strategies: AI in debt collection customizes communication strategies to match individual borrower profiles, enhancing engagement through tailored messages. This personalization increases the effectiveness of interactions, boosting the likelihood of successful debt recovery.
- Increased efficiency and productivity: AI automates routine tasks such as data entry and document processing, freeing human agents to focus on more complex cases. This automation enhances operational speed and boosts productivity within collections teams, streamlining overall processes.
- Compliance and regulation adherence: AI-driven solutions ensure adherence to the latest regulatory standards, helping financial institutions maintain compliance with legal requirements. This reduces non-compliance risk and potential fines, safeguarding the institution’s reputation and financial health.
- Cost reduction: AI significantly cuts operational costs in debt collection by automating processes and enhancing recovery rates. These savings stem from reduced staffing requirements and lower expenses on communications and administrative tasks.
- Enhanced customer experience: AI systems foster a more empathetic approach to debt collection, improving customer satisfaction and loyalty. This positive interaction helps maintain a favorable brand image and strengthens customer relationships, which are crucial for long-term business success.
- Enhanced risk management and analysis: AI tools analyze large datasets to uncover broader financial trends and patterns, enabling better risk management strategies. These insights help institutions adjust their credit policies and portfolio management practices to minimize risks and optimize financial outcomes.
AI in debt collection: future trends
The future of AI in debt collection is rapidly evolving, propelled by the innovative applications of the technology. Let’s explore the upcoming trends that are set to transform this sector:
- Behavioral insights for personalization: Merging AI with behavioral science will give rise to more nuanced debtor profiles. Debt collection strategies will become highly personalized, utilizing these insights to craft communication that resonates on an individual level, thereby improving the chances of repayment.
- Focus on ethical AI: As AI becomes more prevalent, the emphasis on ethical practices and algorithmic transparency will grow. AI systems used in debt collection should be designed to be fair, impartial, and understandable, promoting trust and ethical treatment of debtors.
- Fintech synergy: AI’s role will expand by integrating with emerging financial technologies like blockchain and smart contracts. This synergy will not only heighten the security of debt collection processes but will also introduce new, innovative methods for debt recovery.
- Managing commercial debt volume: AI tools can help manage and prioritize increasing volumes of commercial debt, using data-driven insights to inform collection strategies.
- Customer-centric collections: AI facilitates a customer-centric approach in collections, using sentiment analysis and personalized communication to deliver positive customer outcomes and adhere to changing relationship landscapes.
- Automation of outdated processes: By digitizing and automating outdated collection methods, AI offers customers a more efficient and less frustrating experience. Simple solutions like smart SMS with payment links or QR codes in emails modernize the payment process, allowing customers to easily pay on the go. This streamlining leads to more efficient company operations, as it reduces manual workload and speeds up transaction processing, ultimately boosting overall operational efficiency.
- Enhanced A/B testing with AI: Artificial intelligence transforms A/B testing by quickly learning and analyzing customer behavior. It goes beyond comparing open rates, examining pre and post-interaction behaviors and optimizing communication strategies. AI accelerates testing cycles, providing precise insights for timely strategy adjustments and personalizing customer engagement.
- Optimizing collection rates with data analytics: AI sifts through vast amounts of data to identify trends and inform strategies, moving beyond outdated methods that rely mainly on human judgment. Companies can use minimax strategies enhanced by AI to prepare for worst-case scenarios and adapt their approaches effectively. This predictive approach enables proactive measures, such as offering incentives for early payments, to prevent future defaults. This not only boosts collection rates but also enhances the customer experience.
Endnote
In navigating the complex and often sensitive task of debt collection, artificial intelligence stands as a foundation for innovation, guiding the industry toward a future where efficiency and empathy go hand in hand. As we have explored throughout this article, AI’s integration into debt recovery processes promises a more strategic, personalized, and humane approach to collections. From predictive analytics to advanced communication strategies, AI’s transformative power enables collectors to engage with debtors more effectively while maintaining a strong ethical framework.
This dynamic landscape of AI-driven debt collection is an ongoing journey of advancement and adaptation. We must continue to observe, learn, and refine our approaches, ensuring that as the technology evolves, so too does our commitment to responsible use and continuous improvement. The ultimate goal remains steadfast: fostering a fair, transparent, mutually beneficial relationship between creditors and debtors.
As we conclude, let us remember that at the core of debt collection lies the human element—the debtors with their unique stories and the collectors with their vital roles. It is through the lens of technology that we can bring clarity, compassion, and efficiency to this intricate interaction, shaping a future that respects both the financial imperative and the human dignity involved in every transaction.
Ready to redefine how you manage debt collection? Discover how AI can streamline your processes, improve recovery rates, and enhance customer relations with LeewayHertz’s AI development services.
Start a conversation by filling the form
All information will be kept confidential.
Insights
AI for startups: Its use cases and role in steering success for early-stage businesses
AI empowers startups to overcome resource constraints, outperform competitors, enhance customer experiences, and make data-driven decisions.
AI in loan underwriting: Paving the way for smarter lending
AI is transforming the loan underwriting process by harnessing advanced machine learning algorithms and data analytics, facilitating more informed and efficient credit decisions.
The role of AI in logistics and supply chain
By harnessing the capabilities of AI, companies can refine their operations and elevate their business performance, leading to enhanced profitability, operational efficiency, and increased customer satisfaction.
- What is debt collection?
- Challenges involved in traditional debt collection processes
- The role of AI in enhancing debt collection efficiency
- How does AI for debt collection work?
- Applications of AI in debt collection
- Generative AI applications in debt collection
- Optimizing debt collection workflows with generative AI
- Why is LeewayHertz the right choice for building AI debt collection solutions?
- LeewayHertz's AI development services for debt collection
- Benefits of AI in the debt collection process
- AI in debt collection: future trends
- Contact us