How to build an AI-powered chatbot?
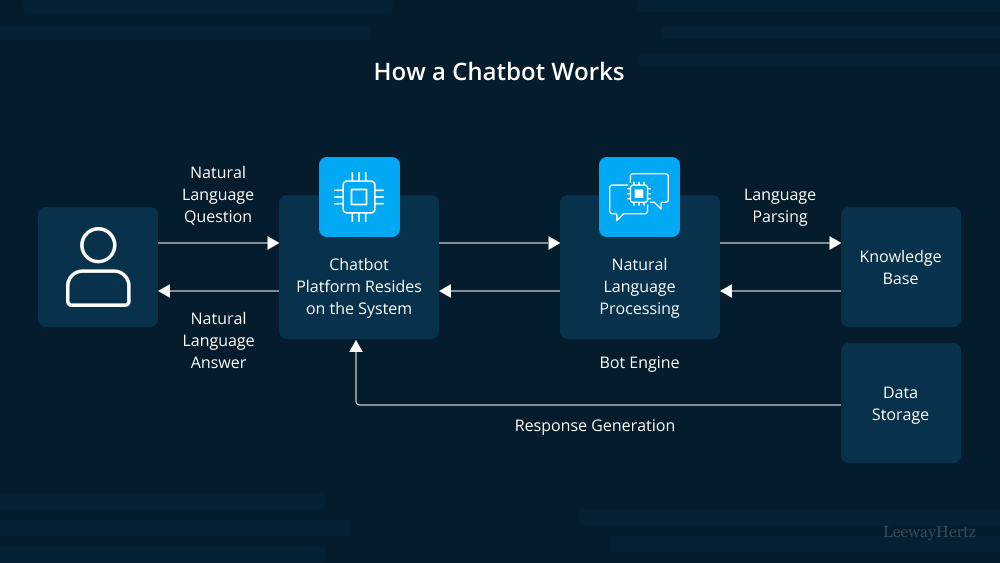
Listen to the article
In today’s fast-paced world, where time is a precious commodity, texting has emerged as one of the most common forms of communication. Hence, chatbots are becoming a crucial part of businesses’ operations, regardless of their size or domain. The concept of chatbots can be traced back to the idea of intelligent robots introduced by Alan Turing in the 1950s. And ELIZA was the first chatbot developed by MIT professor Joseph Weizenbaum in the 1960s. Since then, AI-based chatbots have been a major talking point and a valuable tool for businesses to ensure effective customer interactions. According to Demand Sage, the chatbot market is expected to earn about $137.6 million in revenue by 2023. Moreover, it is projected that chatbot sales will reach approximately $454.8 million by the year 2027.
With all the hype surrounding chatbots, it’s essential to understand their fundamental nature. So, what are chatbots? Chatbots are computer programs designed to simulate human conversation. They achieve this by generating automated responses and engaging in interactions, typically through text or voice interfaces. They are programmed to carry out a wide range of functions, from responding to frequently asked questions and offering customer service to automating sales and marketing procedures, and may be deployed on various platforms, including websites, social media, and messaging apps.
Businesses use these virtual assistants to perform simple tasks in business-to-business (B2B) and business-to-consumer (B2C) situations. Chatbot assistants allow businesses to provide customer care when live agents aren’t available, cut overhead costs, and use staff time better. According to the Demand Sage report cited above, an average customer service agent deals with 17 interactions a day, which means adopting chatbots in enterprises can prevent up to 2.5 billion labor hours.
Clearly, chatbots are one of the most valuable and well-known use cases of artificial intelligence becoming increasingly popular across industries.
Let’s delve deeper into chatbots and gain insights into their types, key components, benefits, and a comprehensive guide on the process of constructing one from scratch.
- What is a chatbot?
- Types of chatbots
- Architectural components of AI-powered chatbots and their operational mechanics
- What are the advantages of using AI-powered chatbots?
- How to build an AI chatbot using LLM?
- What are enterprise chatbots?
- Key functions of enterprise chatbots
- Types of enterprise chatbots
- Benefits of enterprise chatbots
- Use cases of enterprise chatbots
- How can LeewayHertz’s enterprise AI platform help build enterprise chatbots?
What is a chatbot?
A chatbot is an Artificial Intelligence (AI) program that simulates human conversation by interacting with people via text or speech. Chatbots use Natural Language Processing (NLP) and machine learning algorithms to comprehend user input and deliver pertinent responses. Chatbots can be integrated into various platforms, including messaging programs, websites, and mobile applications, to provide immediate responses to user queries, automate tedious processes, and increase user engagement. While some chatbots are task-oriented and offer particular responses to predefined questions, others closely mimic human communication. Computer scientist Michael Mauldin first used the term “chatterbot” in 1994 to to describe what later became recognized as the chatbot. The biggest reason chatbots are gaining popularity is that they give organizations a practical approach to enhancing customer service and streamlining processes without making huge investments.
Chatbots use dialogue systems to efficiently handle tasks related to retrieving information, directing inquiries to the appropriate channels, and delivering customer support services. Some chatbots utilize advanced natural language processing and word categorization techniques to understand and interpret user inputs. These chatbots can comprehend the context and nuances of the conversation, allowing for more accurate and detailed responses. On the other hand, some chatbots rely on a simpler method of scanning for general keywords and constructing responses based on pre-defined expressions stored in a library or database. The primary methods through which chatbots can be accessed online are virtual assistants and website popups. Virtual assistants, such as voice-activated chatbots, provide interactive conversational experiences through devices like smartphones or smart speakers. Website popups, on the other hand, are chatbot interfaces that appear on websites, allowing users to engage in text-based conversations. These two contact methods cater to various utilization areas, including business (such as e-commerce support), learning, entertainment, finance, health, news, and productivity.
Types of chatbots
Chatbots can be classified in various ways based on different criteria. Here are some typical chatbot classifications:
Rule-based chatbots: They operate according to a set of rules or scripts and hence, are known as “rule-based” chatbots. They use predetermined answers based on phrases or patterns found in user inputs. Rule-based chatbots are straightforward and have limited functionality because they can only answer a limited set of predefined questions.
Machine learning-based chatbots: Artificial intelligence (AI) and natural language processing (NLP) techniques are used by machine learning-based chatbots to understand and respond to user input. Based on user interactions, they can gradually improve their responses as they learn from the data. Machine learning-based chatbots are more sophisticated and capable of handling a larger range of questions than rule-based chatbots.
Retrieval-based chatbots: Chatbots that rely on retrieval create responses based on predefined responses kept in their database. They don’t produce new responses but choose the most appropriate response based on user input. Retrieval-based chatbots are frequently employed for particular tasks or domains where predefined responses are possible.
Generative chatbots: Chatbots that develop responses on their own, rather than relying on predefined ones, are called generative chatbots. They can produce fresh, interactive replies in response to user input. Compared to retrieval-based chatbots, generative chatbots are more adaptable and imaginative in their responses, but they can also be more difficult to build and train.
Task-oriented chatbots: Chatbots built to do certain jobs or duties, such as scheduling appointments, offering customer assistance, or making reservations, are known as task-oriented chatbots. They have a limited functional range and are concentrated on carrying out a specific activity.
Conversational Chatbots: Chatbots built to have open-ended interactions with users are called conversational chatbots. They may not have clear tasks or responsibilities because they are primarily concerned with facilitating conversational interactions. Conversational chatbots are frequently employed for amusement, customer engagement, and social connections.
Architectural components of AI-powered chatbots and their operational mechanics
Chatbots are similar to a messaging interface where bots respond to users’ queries instead of human beings. They look like other apps. But their UI layer works differently. Machine learning algorithms power the conversation between a human being and a chatbot.
ML algorithms break down your queries or messages into human-understandable natural languages with NLP techniques and send a response similar to what you expect from the other side. Let us share an example of how a chatbot works.
Suppose you have a smart AI-based conversational chatbot app on your phone or computer and want to travel from LA to New York. You can open the chatbot app and write a message:
“Book a flight from LA to New York.”
You may get a response like this:
“How many people are traveling with you?
Once you send a response, the bot will respond with all relevant flight details in seconds. Sounds amazing. Right?
The generated response from the chatbot exhibits a remarkable level of naturalness, resembling that of genuine human interaction. However, it is essential to recognize the extensive efforts undertaken to deliver such an immersive experience.
First of all, a bot has to understand what input has been provided by a human being. Chatbots achieve this understanding via architectural components like artificial neural networks, text classifiers, and natural language understanding.
Let’s understand the architectural components in depth.
Optimize Your Operations With AI Agents
Optimize your workflows with ZBrain AI agents that automate tasks and empower smarter, data-driven decisions.
Text classifiers
In chatbot development, text classification is a typical technique where the chatbot is educated to comprehend the intent of the user’s input and reply appropriately. Text classifiers examine the incoming text and group it into intended categories after analysis. Certain intentions may be predefined based on the chatbot’s use case or domain. Text classification can be accomplished using deep learning models like Convolutional Neural Networks (CNN) or Recurrent Neural Networks (RNN), as well as machine learning methods like naive bayes and Support Vector Machines (SVM). Since the input text is linked to specified categories or intentions, these models are trained on labeled data, and to forecast the intent of the incoming text input, the model learns the patterns and features in the text data.
Once the intent of the text input has been determined, the chatbot can produce a response or carry out the appropriate activities in accordance with the programmed responses or actions related to that intent. For instance, if the user wants to book a flight, the chatbot can request essential details, such as the destination, time of travel, and the number of passengers, before booking the flight as necessary.
Text classifiers are a crucial part of chatbot architecture because they allow the chatbot to comprehend user input and react accordingly based on the user’s intent, resulting in a more tailored and meaningful conversation experience.
Natural Language Processing (NLP)
Natural Language Processing (NLP) is a subfield of artificial intelligence that enable computers to understand, interpret, and respond to human language. Applications for NLP include chatbots, virtual assistants, sentiment analysis, language translation, and many more.
The processing of human language by NLP engines frequently relies on libraries and frameworks that offer pre-built tools and algorithms. Popular libraries like NLTK (Natural Language Toolkit), spaCy, and Stanford NLP may be among them. These libraries assist with tokenization, part-of-speech tagging, named entity recognition, and sentiment analysis, which are crucial for obtaining relevant data from user input.
The preprocessing of the incoming text is the initial stage in NLP. Tokenization separates the text into individual words or phrases (tokens), eliminating superfluous features like punctuation, special characters, and additional whitespace. To reduce noise in the text data, stopwords, which are frequent words like “and,” “the,” and “is,” can be safely eliminated.
Further, lemmatization and stemming are methods for condensing words to their root or fundamental form. While stemming entails truncating words to their root form, lemmatization reduces words to their basic form (lemma). For instance, “running” would be stemmed and lemmatized to “run.” Part of Speech (POS) tagging is then employed, which entails classifying each word in the text according to its grammatical type, such as a noun, verb, adjective, etc. Understanding the grammatical structure of the text and gleaning relevant data is made easier with this information.
Named Entity Recognition (NER) is a crucial NLP task that involves locating and extracting specified data from user input, including names of individuals, groups, places, dates, and other pertinent entities. The chatbot or other NLP programs can use this information to interpret the user’s purpose, deliver suitable responses, and take pertinent actions.
The chatbot responds based on the input message, intent, entities, sentiment, and dialogue context. This can involve selecting pre-defined responses from a set of templates, dynamically producing responses using text generation techniques like language models, or combining pre-defined templates with dynamic text generation to provide tailored responses. Natural language generation is the next step for converting the generated response into grammatical and human-readable natural language prose. This process may include putting together pre-defined text snippets, replacing dynamic material with entity values or system-generated data, and assuring the resultant text is cohesive. The chatbot replies with the produced response, displayed on the chat interface for the user to read and respond to.
The chatbot may continue to converse with the user back and forth, going through the above-said steps for each input and producing pertinent responses based on the context of the current conversation.
Knowledge base
A knowledge base is a collection of data that a chatbot utilizes to generate answers to user questions. It acts as a repository of knowledge and data for the chatbot to deliver precise and accurate answers to user inquiries.
Installing the chatbot will determine the exact format of the knowledge base. It could be a database with organized data like facts, Frequently Asked Questions (FAQs), product details, or other pertinent data. The chatbot can run a query against this database to get the relevant data and utilize it to generate responses. A knowledge base could also include a library of pre-written responses or templates that the chatbot can utilize to create responses on the go. These pre-written replies can be created to address frequent user scenarios and queries. The following steps are often required for a chatbot’s knowledge base to function:
Collecting essential data is the first stage in creating a knowledge base. Text files, databases, webpages, or other information sources create the knowledge base for the chatbot. After the data has been gathered, it must be transformed into a form the chatbot can understand. Tasks like cleaning, normalizing, and structuring may be necessary to ensure the data is searchable and retrievable.
The knowledge base’s content must be structured so the chatbot can easily access it to obtain information. To do this, it may be necessary to organize the data using techniques like taxonomies or ontologies, natural language processing (NLP), text mining, or data mining.
The knowledge base must be indexed to facilitate a speedy and effective search. Various methods, including keyword-based, semantic, and vector-based indexing, are employed to improve search performance. The collected data may subsequently be graded according to relevance, accuracy, or other factors to give the user the most pertinent information. The chatbot explores the knowledge base to find relevant information when it receives a user inquiry. After retrieving the required data, the chatbot creates an answer based on the information found.
A knowledge base must be updated frequently to stay informed because it is not static. Chatbots can continuously increase the knowledge base by utilizing machine learning, data analytics, and user feedback. To keep the knowledge base updated and accurate, new data can be added, and old data can be removed. The knowledge base is connected with the chatbot’s dialogue management module to facilitate seamless user engagement. The dialogue management component can direct questions to the knowledge base, retrieve data, and provide answers using the data.
A chatbot knowledge base generally functions by gathering, processing, organizing, and expressing information to facilitate effective search, retrieval, and response creation. It is an essential element that allows chatbots to offer users accurate and relevant information and continuously enhance their performance through continuous learning.
Dialogue management
A crucial part of a chatbot is dialogue management which controls the direction and context of the user’s interaction. Dialogue management is responsible for managing the conversation flow and context of the conversation. It keeps track of the conversation history, manages user requests, and maintains the state of the conversation. Dialogue management determines which responses to generate based on the conversation context and user input. Let’s explore the technicalities of how dialogue management functions in a chatbot.
Input processing: The chatbot accepts inputs from the user, which may be speech or text. The task of retrieving pertinent data from the input, including intent, entities, and context, falls into the input processing module. Variables, such as dates, names, or locations, are the specific bits of information provided as the input, whereas intent represents the user’s intention or purpose behind the input. Context is used to describe pertinent details from the conversation’s earlier turns, which aids in preserving the dialogue’s flow.
Intent recognition: Inferring the user’s intention based on their input is a crucial step in dialogue management for chatbots. Various methods can be employed to achieve this, including rule-based strategies, statistical approaches, and machine learning algorithms such as natural language understanding (NLU) models. NLU models utilize techniques like deep learning, recurrent neural networks, or transformers to process and understand the input text, enabling them to identify the intent behind the user’s message.
Dialogue state tracking: The chatbot updates its internal dialogue state, which depicts the current context and stage of the interaction, as soon as the intent is identified. The dialogue state contains any important context that must be retained to preserve discussion consistency, such as user choices, system answers, and other information. Dialogue state tracking is essential for managing the dynamic nature of discussions and delivering suitable responses based on the current state of the dialogue.
Conversation policy: The dialogue policy decides the chatbot’s action or response based on the current dialogue state. The conversation policy may be rule-based, in which case previously established rules determine the response, or it may be machine-learned using deep learning or reinforcement learning. The chatbot is trained using reinforcement learning to discover the best course of action based on a reward signal that denotes the effectiveness of the action taken. Large amounts of training data can be utilized to learn the dialogue policy using deep learning techniques like neural networks.
Response generation: The chatbot generates a response to the user once the dialogue policy decides the proper action or reaction. Natural Language Generation (NLG) techniques or predefined templates can generate the response, with placeholders filled in with the relevant data from the dialogue state. The goal of NLG techniques is to produce text that sounds like human speech based on the state of the conversation and the desired response. These techniques can use template-based generation, rule-based generation, or machine learning-based generation using tools like recurrent neural networks or transformers.
System response: The system responds by sending the generated response back to the user. Depending on the chatbot’s capabilities and the user’s desires, the system’s answer may be in text, speech, or other modalities.
User interaction and feedback: The chatbot keeps up the conversation with the user, taking in new information and changing the dialogue’s status in response to the user’s responses. The chatbot may also gather user feedback to improve over time by enhancing responses.
Error handling and fall-back mechanisms: Conversation management also entails handling mistakes and fall-back procedures if the chatbot cannot discern intent, extract entities, or produce suitable responses. The error handling strategies may include error correction, re-prompting, or clarifying inquiries. Fall-back techniques can involve redistributing the workload or offering substitute replies.
Machine learning models
The components of the chatbot architecture heavily rely on machine learning models to comprehend user input, retrieve pertinent data, produce responses, and enhance the user experience.
Collecting data is the initial step in creating an ML-based chatbot. A wide variety of inputs and outputs, including text dialogues, user questions, and related answers, can be included in this data. The ML algorithms are trained using this data to recognize and respond to various inputs and query types.
After data collection is preprocessed to eliminate noise and irrelevant information, the data is extracted with the required features or patterns from the text data. Tokenization, a technique that separates text into individual words or phrases and generates numerical representations of these words or phrases, such as word embeddings or bag-of-words representations, may be used in this situation. These features operate as inputs to the ML algorithms, assisting them in interpreting the meaning of the text.
The ML model needs to be trained after the data has been preprocessed and the features are extracted. Chatbots can use a variety of machine learning algorithms, including rule-based algorithms, traditional ML algorithms like naive bayes and decision trees, and more sophisticated ML algorithms like recurrent neural networks, convolutional neural networks, and transformer models. The model builds a mapping between user queries and responses during training by learning from the input attributes and associated outputs.
The ML model must be tested after training to gauge its effectiveness. A valid set of data—which was not used during training—is often used to accomplish this. The model’s performance can be assessed using various criteria, including accuracy, precision, and recall. Additional tuning or retraining may be necessary if the model is not up to the mark. Once trained and assessed, the ML model can be used in a production context as a chatbot. Based on the trained ML model, the chatbot can converse with people, comprehend their questions, and produce pertinent responses. For a more engaging and dynamic conversation experience, the chatbot can contain extra functions like natural language processing for intent identification, sentiment analysis, and dialogue management.
After deployment, an iterative procedure can continuously enhance the chatbot. The ML model can be further trained and improved by gathering user input and interactions. The chatbot can continuously learn from and adjust to human behavior thanks to this feedback loop, which helps it perform better over time.
Machine learning plays a crucial role in the technical operation of chatbots by enabling them to comprehend user inputs, produce suitable responses, and enhance their performance over time through continuous learning and adaptation.
Backend services
A chatbot’s integration and functionality depend heavily on backend services. The backend handles the underlying business logic, manages data storage, and interacts with external systems to ensure the chatbot runs smoothly. In a chatbot architecture, backend services have the following critical functions:
Business logic: The underlying code or algorithms that implement the particular functionality or features of the chatbot are referred to as the chatbot’s business logic. Based on specified rules, scripts, or machine learning models, it dictates how the chatbot understands user inputs, creates appropriate responses, and handles different jobs or activities.
Integration with external systems: To retrieve or store data, backend services frequently need to integrate with external systems like databases, APIs, or other services. This can involve getting user data and information from external APIs or updating databases with relevant data from user interactions.
API integrations: Backend services can integrate with external APIs to obtain information or features from other services, such as payment processors, third-party databases, weather APIs, or weather forecasting tools. As a result, the chatbot can utilize other resources to give users access to more thorough and accurate information and services.
Backend services are essential for the overall operation and integration of a chatbot. They manage the underlying processes and interactions that power the chatbot’s functioning and ensure efficiency.
User Interface
The user interface in a chatbot serves as the bridge between the chatbot and consumers, enabling communication through a message interface like an online chat window or messaging app. This component plays a crucial role in delivering a seamless and intuitive experience. A well-designed UI incorporates various elements such as text input/output, buttons, menus, and visual cues that facilitate a smooth flow of conversation. The UI must be simple, ensuring users can easily understand and navigate the chatbot’s capabilities and available options. Users can effortlessly ask questions, receive responses, and accomplish their desired tasks through an intuitive interface, enhancing their overall engagement and satisfaction with the chatbot.
Analytics and monitoring
A chatbot architecture must have analytics and monitoring components since they allow tracking and analyzing the chatbot’s usage and performance. They allow for recording relevant data, offering insights into user interactions, response accuracy, and overall chatbot efficacy. The performance and capabilities of the chatbot enhance over time with the use of this data.
Monitoring performance metrics such as availability, response times, and error rates is one-way analytics, and monitoring components prove helpful. This information assists in locating any performance problems or bottlenecks that might affect the user experience. These insights can also help optimize and adjust the chatbot’s performance.
User interaction analysis is essential for comprehending user trends, preferences, and behavior. Analytics and monitoring components offer insights into how users interact with the chatbot by collecting data on user queries, intentions, entities, and responses. This data can be utilized to spot trends, frequently asked questions by users, and areas where the chatbot interpretations and response capabilities should be strengthened.
What are the advantages of using AI-powered chatbots?
Here are some key advantages of deploying AI chatbots in various business processes:
Improved end-user experience: Chatbots provide end-user support on a real-time basis in any setting, whether in a retail sales store, product support center/website or a business front or back office. Because these interfaces are readily available to end-users, there is no specific wait time. This means customers or end-users can readily have answers to their queries, which significantly enhances the user experience. Based on the query, chatbots can present users with rich content with documentation, videos, and so on to help resolve queries. Furthermore, chatbots can provide 24/7 assistance and support to end-users. They can be programmed to immediately provide automated answers to common queries and forward the request to a real person when a more comprehensive action is required. This has a significant positive impact on customer and user experience.
Increased face time with customers: Businesses can use chatbots to increase their face time. Modern consumers anticipate a personalized relationship with their preferred brands through longer interactions and more tailored communication channels. Chatbots facilitate this by facilitating quicker and easier access. Moreover, chatbots are easily integrated into well-known platforms like Facebook or Instagram, allowing for a seamless user experience.
Analytics and insights: Chatbots are a great communication channel and a medium to gather insights into customer preferences and behavior. Businesses can collect instant feedback from customers and end-users through chatbots and then analyze the data to gather insights into their habits and preferences. Besides, chatbots can also be leveraged to identify purchasing patterns and consumer behavior. It can help businesses make critical decisions around product marketing and launch strategies.
Lead generation and conversion: Chatbots can be developed to offer users personalized guidance and assistance throughout their shopping experience. Chatbots can recommend goods or services that are probably interesting to the user by gathering information on their preferences, behavior, and previous purchases. Moreover, chatbots can employ persuasive language and techniques to influence user judgment and persuade them to purchase.
For instance, a chatbot on an e-commerce website can inquire about the user’s tastes and spending limit before making product recommendations that match those parameters. To persuade the user to buy anything, the chatbot can also provide social evidence, such as testimonials and ratings from other consumers. Chatbots can occasionally offer users special discounts or promotions to entice them to buy. Businesses may boost conversion rates and customer satisfaction by introducing chatbots to help consumers through shopping. Chatbots can make users’ buying experiences more personalized and interesting, enhancing customer retention and brand loyalty.
Cost savings and scalability: Developing and implementing a fully functional chatbot is faster and cheaper than developing a cross-platform app or hiring employees to handle many incoming queries. Thus, businesses can significantly save hiring, training and payroll costs. A typical chatbot would only involve the initial development cost and a nominal runtime cost, potentially less than the costs spent on actual human resources.
Furthermore, multi-lingual chatbots can scale up businesses in new geographies and linguistic areas relatively faster.
Optimize Your Operations With AI Agents
Optimize your workflows with ZBrain AI agents that automate tasks and empower smarter, data-driven decisions.
How to build an AI chatbot using LLM?
Building an AI chatbot has become increasingly feasible with the advancement of large language models (LLMs) like GPT and LLaMA. These models, pre-trained on extensive datasets, can generate human-like text, making them ideal for creating interactive and responsive chatbots. Here’s a step-by-step guide to building an AI chatbot using LLMs.
1. Installation and setup
Start by preparing your development environment. This involves:
- Installing necessary libraries: Ensure you have the required libraries that provide access to pre-trained LLMs. These might include frameworks like TensorFlow and PyTorch or libraries such as Hugging Face’s transformers.
- Setting up a development environment: Use tools like virtualenv or conda to create a virtual environment, which helps manage dependencies and keeps your workspace organized.
2. Choosing the right model
Selecting the appropriate model is critical for your chatbot’s performance:
- Open-source LLMs: Opt for well-known models like GPT-4 or LLaMA 3. These models are extensively trained on diverse datasets and are known for their ability to generate human-like text and handle complex conversations.
- Consider needs: Choose a model based on the complexity of conversations your chatbot will handle. Simpler models may suffice for basic interactions, while more advanced models are necessary for complex dialogues.
3. Building the chatbot core
The core of the chatbot involves loading and preparing the selected model:
- Model initialization: Load the selected model and its tokenizer. The tokenizer breaks down the text into tokens that the model can understand.
- Response generation: The core process includes encoding user input, processing it through the LLM, and decoding the model’s output into a readable response. This ensures the chatbot can generate relevant and coherent replies.
4. Developing the chatbot backend
A robust backend is essential for deploying your chatbot:
- Web framework: Use frameworks like Flask or Django to create a server that handles user requests. The backend should be capable of receiving user inputs, processing them, and sending back the generated responses.
- API endpoints: Develop API endpoints that facilitate communication between the user interface and the LLM.
5. Creating the chat interface
The interface is where users interact with your chatbot:
- Platform-specific design: The chat interface should be tailored to your target platform, whether it’s a website, communication app, or customer service platform, focusing on user-friendliness and seamless integration.
- User-centric interface: Design the interface with an intuitive user journey in mind, ensuring clear input fields, readable text, and responsive elements that adapt to different devices.
6. Chatbot interaction process
Maintaining a coherent conversation requires managing the dialogue context:
- Context tracking: Track the conversation history to provide contextually relevant responses. This involves encoding the entire conversation along with the new user input.
- Dialogue flow: Ensure the chatbot updates the conversation history with each interaction, maintaining the flow and coherence of the conversation.
7. Integrate retrieval systems
For advanced functionality, consider integrating retrieval-augmented generation (RAG) systems:
- Information retrieval: RAG systems pull information from databases or corpora to provide contextually relevant responses. This enhances the chatbot’s ability to deliver accurate and informative answers.
- Knowledge integration: Use these systems to integrate domain-specific knowledge, making your chatbot more knowledgeable and useful.
8. Testing and debugging
Thorough testing is vital for ensuring your chatbot functions correctly:
- Simulate user inputs: Use tools to simulate various user interactions and verify the chatbot’s responses. Ensure it handles a wide range of scenarios effectively.
- Identify and fix issues: Continuously test and debug to resolve issues like incorrect responses or unexpected behavior. This refinement process is crucial for improving performance.
9. Deployment
Once the chatbot is tested and refined, it’s ready for deployment:
- Public access: Deploy the chatbot via a public URL, integrate it with customer-facing systems, or embed it in websites and apps.
- Scalability and security: Ensure that your deployment process includes considerations for scalability and security to handle user interactions efficiently.
Following these steps allows for the development of an advanced AI chatbot using LLMs. Each step is crucial to ensuring the chatbot is efficient, user-friendly, and capable of delivering meaningful interactions.
Transition to enterprise chatbots
While the process of building an AI chatbot with LLMs equips you with the foundational skills for creating advanced conversational agents, enterprise chatbots take these capabilities to a new level. These sophisticated systems are specifically designed for large-scale organizational use, offering deep integration with company-specific data and handling complex business functions.
What are enterprise chatbots?
Enterprise chatbots represent the cutting edge of conversational AI technology tailored for large-scale organizational use. These sophisticated systems go far beyond simple question-and-answer interfaces, functioning as intelligent virtual assistants capable of handling complex queries and tasks across various business functions.
At their core, enterprise chatbots leverage advanced Natural Language Processing (NLP) and Natural Language Understanding (NLU) to interpret user inputs and generate appropriate responses. This allows them to engage in more natural, context-aware conversations that can understand nuances, idioms, and even emotional undertones.
What sets enterprise chatbots apart is their deep integration with company-specific data and systems. They’re not just generic chatbots – they’re trained on vast amounts of organization-specific information, allowing them to provide highly accurate and relevant responses within the company’s unique context. This could include everything from internal policies and procedures to product specifications and customer history.
Moreover, enterprise chatbots are designed with scalability in mind. They can handle thousands of simultaneous interactions without compromising quality or speed, making them ideal for large organizations with high internal and external communications volumes.
Key functions of enterprise chatbots
Enterprise chatbots are designed to optimize and streamline various business processes, enhancing efficiency and user experience. Their advanced functionalities make them indispensable tools for large organizations. Here are some of the key functions of enterprise chatbots:
- Multi-request management: Enterprise chatbots excel at juggling multiple queries simultaneously. They can parse complex requests, break them into manageable parts, and efficiently address each component. This capability is crucial in high-volume environments where multiple users may be seeking assistance on various topics simultaneously.
- Seamless integration: These chatbots are designed to integrate smoothly with a wide array of enterprise software systems. These could include Customer Relationship Management (CRM) tools, Enterprise Resource Planning (ERP) systems, Human Resources Information Systems (HRIS), and more. This integration allows the chatbot to pull relevant information from multiple sources in real time, providing comprehensive and accurate responses.
- Multilingual support: In our increasingly globalized business world, the ability to communicate across language barriers is crucial. Enterprise chatbots often come equipped with multilingual capabilities, allowing them to engage with users in their preferred language. This feature not only enhances user experience but also expands the chatbot’s utility across different geographic regions and markets.
- Continuous learning: One of the most powerful features of enterprise chatbots is their ability to learn and improve over time. Through machine learning algorithms, these chatbots analyze each interaction, identifying patterns and refining their responses. This continuous learning process allows them to become more accurate and efficient over time, adapting to changing user needs and new information.
- Human handoff: While enterprise chatbots are highly capable, they’re also designed to recognize their limitations. When faced with a query or situation that requires human expertise or empathy, these chatbots can smoothly transfer the conversation to a human agent. Importantly, they provide the human agent with the full context of the conversation, ensuring a seamless transition for the user.
- Scalable conversations: Enterprise chatbots are built to handle vast volumes of interactions without compromising on quality. This scalability is crucial for large organizations that may need to manage thousands or even millions of customer or employee interactions daily. The chatbot can maintain consistent performance regardless of volume, ensuring that each user receives prompt and accurate assistance.
Types of enterprise chatbots
Enterprise chatbots come in various forms, each tailored to meet specific organizational needs and enhance different aspects of business operations. Here are some types of enterprise chatbots:
- FAQ chatbots: These are often the entry point for organizations implementing chatbot technology. They excel at quickly providing answers to common questions, reducing the load on human customer service representatives. FAQ chatbots can be particularly useful for handling routine inquiries about product features, pricing, or company policies.
- Conversational chatbots: These more advanced chatbots use sophisticated NLP and NLU to engage in more natural, context-aware dialogues. They can understand and respond to complex queries, maintain context over multiple exchanges, and even pick up on emotional cues in the user’s language. This makes them particularly effective for more nuanced customer service interactions or internal use where employees might have complex, multifaceted questions.
- RPA (Robotic Process Automation) chatbots: These chatbots specialize in automating repetitive, rule-based tasks. They can interact with multiple IT systems to perform actions like data entry, file transfers, or generating reports. In an enterprise context, RPA chatbots can significantly reduce the time spent on routine administrative tasks, freeing up employees to focus on more strategic work.
- AI customer service chatbots: These chatbots take customer service to the next level by not just answering questions but by understanding and responding to the customer’s emotional state. They can detect frustration, confusion, or satisfaction in a customer’s language and adjust their responses accordingly. This emotional intelligence allows them to provide a more empathetic and effective customer service experience.
Benefits of enterprise chatbots
Enterprise chatbots offer a range of benefits that significantly enhance organizational operations and user experiences. These advantages include:
- Increased organizational efficiency: By automating routine tasks and inquiries, enterprise chatbots free up human employees to focus on more complex, high-value work. This can lead to significant improvements in overall organizational productivity.
- Enhanced customer and employee engagement: The ability to provide instant, 24/7 responses can greatly improve both customer and employee satisfaction. Users appreciate the ability to get quick answers to their questions without having to wait for human assistance.
- Reduced support ticket resolution time: By handling a large volume of routine inquiries, chatbots can significantly reduce the workload on support teams. This allows human agents to focus on more complex issues, leading to faster resolution times across the board.
- Lower operating costs: While implementing chatbot technology requires an initial investment, the long-term cost savings can be substantial. Chatbots can handle a large volume of interactions at a fraction of the cost of human agents.
- Real-time customer insights: Every interaction with a chatbot generates data. This wealth of information can provide valuable insights into customer behavior, preferences, and pain points, informing business strategy and product development.
- Improved omnichannel strategy: Enterprise chatbots can be deployed across multiple platforms – website, mobile app, social media, etc. – ensuring a consistent user experience regardless of how customers choose to engage with the company.
- 24/7 availability: Unlike human agents, chatbots can provide round-the-clock service without additional cost, ensuring that customers or employees can get assistance whenever they need it.
- Scalability: During peak times or sudden spikes in demand, chatbots can handle increased volumes without any drop in service quality. This scalability ensures consistent service levels even during unpredictable demand fluctuations.
Use cases of enterprise chatbots
Enterprise chatbots offer versatile solutions across various business functions, enhancing efficiency and improving user experiences. Here are some key use cases:
- Customer service and support: This is perhaps the most common use case for enterprise chatbots. They can handle a wide range of customer inquiries, from product information requests to troubleshooting common issues. In more complex cases, they can collect initial information before transferring it to a human agent, streamlining the support process.
- Enterprise IT support: In large organizations, IT support can be a significant bottleneck. Chatbots can handle many common IT issues, such as password resets, software installations, or basic troubleshooting. This reduces wait times for employees and frees up IT staff to focus on more complex technical issues.
- Internal process automation: Chatbots can streamline many internal processes. For example, they can assist with employee onboarding by providing information about company policies, helping new hires complete necessary paperwork, and answering common questions. They can also assist with tasks like inventory management or data entry, reducing the administrative burden on employees.
- HR assistance: HR departments often deal with a high volume of repetitive queries. Chatbots can handle many of these, such as questions about benefits, vacation policies, or payroll. They can also assist with processes like performance reviews or training sign-ups.
- Appointment scheduling and booking: In industries like healthcare or professional services, chatbots can manage the entire appointment booking process. They can check availability, schedule appointments, send reminders, and even handle rescheduling or cancellations.
- Virtual assistants for enterprise applications: Integrated with enterprise software, chatbots can act as intelligent interfaces for complex systems. They can help employees navigate these systems, pull relevant data, generate reports, or perform specific actions, all through natural language commands.
- Knowledge base access: Large organizations often have extensive knowledge bases that can be difficult to navigate. Chatbots can act as intelligent search interfaces, helping users quickly find the information they need, whether it’s a company policy, a product specification, or a how-to guide.
How can LeewayHertz’s enterprise AI platform help build enterprise chatbots?
LeewayHertz’s enterprise AI platform, ZBrain, is an all-in-one orchestration solution that offers an innovative, low-code approach for building enterprise chatbots using proprietary data. This comprehensive platform enables organizations to develop, deploy, and manage enterprise chatbots securely and efficiently. With its robust architecture and flexible integration options, ZBrain empowers enterprises to leverage AI capabilities across various domains and applications. Here’s how it streamlines the development of enterprise chatbots:
- Advanced knowledge base: ZBrain ingests data from various sources and formats, creating a rich knowledge base for chatbots. This ensures that enterprise chatbots have access to comprehensive, up-to-date information, allowing them to provide accurate and contextually relevant responses.
- Low-code development: The Flow feature offers pre-built components for rapid chatbot development. This allows enterprises to create complex AI-powered chatbots without extensive coding, speeding up the development process.
- Customization: ZBrain enables tailoring AI solutions, including chatbots, to specific business needs. This means enterprises can create chatbots that align perfectly with their unique workflows and requirements.
- Integration capabilities: The platform seamlessly integrates with existing technology stacks, allowing chatbots to access and utilize data from various enterprise systems.
- Multi-model support: Being cloud- and model-agnostic, ZBrain can work with various AI models (like GPT-4, Claude, Gemini, LLaMA, etc.). This flexibility allows enterprises to choose or switch between different models for their chatbots based on specific requirements.
- Advanced prompting techniques: ZBrain supports various prompting techniques, ensuring high accuracy in chatbot responses and enabling more sophisticated conversational capabilities.
- Continuous improvement: The Human in the Loop feature allows for gathering feedback and making corrections, helping to refine the chatbot’s performance over time.
- Evaluation and monitoring: ZBrain’s evaluation suite and APPOps features enable continuous testing, monitoring, and improvement of chatbot performance, ensuring reliability and consistency.
- Security and privacy: Designed for secure, private deployment in enterprise environments, ZBrain ensures that sensitive data used in chatbots remains protected.
- Scalability: As an enterprise-grade platform, ZBrain is built to handle the scaling needs of large organizations, allowing chatbots to grow with the business.
- Natural Language Processing: ZBrain’s capabilities in NLP tasks like sentiment analysis, text classification, and summarization enhance the chatbot’s ability to understand and respond to user queries effectively.
By providing these features and capabilities, ZBrain enables enterprises to build sophisticated, knowledge-rich, and highly customized chatbots that can efficiently handle complex tasks and integrate seamlessly with existing business processes. This comprehensive approach allows for the creation of chatbots that not only answer queries but also contribute to improving operational efficiency and enhancing customer experience.
Endnote
Chatbots have emerged as a powerful technology that combines the strengths of artificial intelligence and natural language processing, enabling automated interactions and the simulation of human-like conversations. Their integration into various industries, such as e-commerce, healthcare, and banking, has proven to enhance operational efficiency, streamline processes, and improve overall customer experiences. As their adoption continues to grow rapidly, chatbots are set to transform our interactions with technology and reshape business operations. AI-powered chatbots offer a wider audience reach and greater efficiency compared to human counterparts, making them increasingly valuable for businesses.
Enterprise chatbots, in particular, stand out for their ability to handle complex organizational tasks and integrate deeply with company systems. These advanced chatbots are designed to manage large-scale interactions and provide real-time insights, contributing to significant improvements in productivity and cost efficiency. By automating routine inquiries, supporting internal processes, and providing 24/7 availability, enterprise chatbots help organizations maintain high service levels and respond to evolving demands effectively. Looking ahead, it is conceivable that both AI-powered and enterprise chatbots will evolve into indispensable tools for businesses across industries, driving further innovation and operational excellence.
Ready to build powerful AI-based chatbots? Consult our LeewayHertz AI experts to enhance internal operations and customer experiences with advanced chatbots that integrate seamlessly and handle complex tasks.
Listen to the article
Start a conversation by filling the form
All information will be kept confidential.
FAQs
What is a chatbot?
How do chatbots benefit businesses?
How do AI-powered chatbots work?
How can businesses use chatbots effectively?
Can chatbots be customized for specific business needs?
How do chatbots handle multilingual interactions?
How do businesses measure the success of their chatbots?
Can LeewayHertz customize chatbots for specific business needs?
How can businesses get started with LeewayHertz for custom chatbot development?
Insights
Generative AI for Regulatory Compliance: Benefits, integration approaches, use cases, best practices, and future trends
Generative AI is reshaping the field of regulatory compliance by enhancing risk management, boosting operational efficiency, and improving compliance monitoring.
Generative AI for marketing: Overview, use cases, integration strategies, and future outlook
Generative AI is transforming the marketing landscape by enhancing content creation, customer interaction, and data analysis.
Generative AI in due diligence: Integration approaches, use cases, challenges and future outlook
Generative AI is reshaping the due diligence landscape, establishing new data analysis and processing benchmarks.