AI agent for knowledge management: Key capabilities, use cases and applications, benefits and implementation
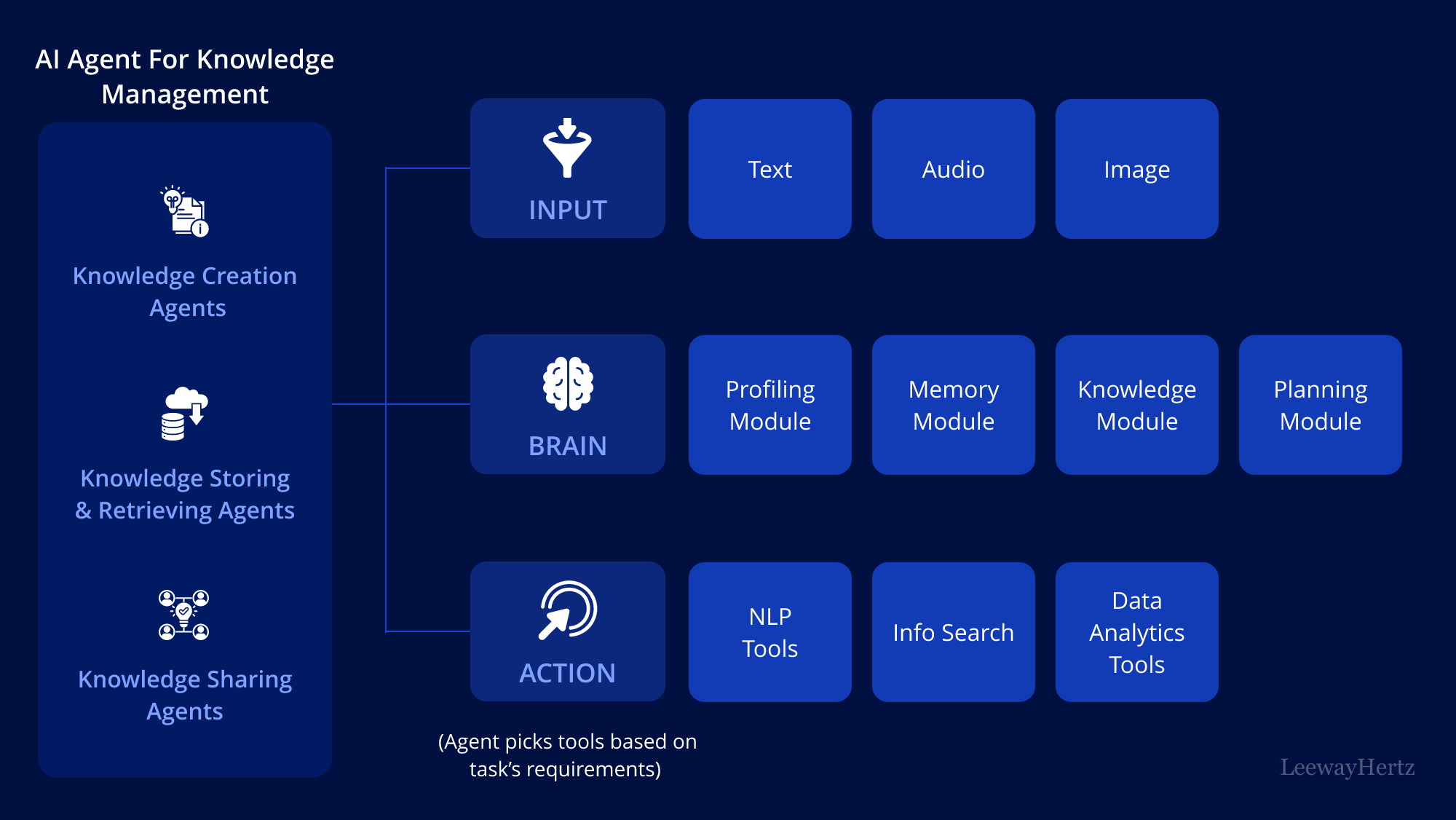
In the business landscape, organizations are struggling with vast amounts of data dispersed across various platforms and formats. Traditional knowledge management (KM) systems often struggle to keep pace, resulting in inefficiencies, information silos, and missed opportunities. This approach to knowledge management can significantly impede an organization’s agility and responsiveness to market changes, customer demands, and internal needs.
As AI evolves, AI agents become game-changing solutions designed to transform knowledge management. These intelligent agents leverage advanced language models to integrate, organize, and retrieve information seamlessly. AI agents excel at processing unstructured data, converting it into structured, easily accessible knowledge that can be utilized across the organization. AI agents liberate human resources by automating tasks such as data entry, document classification, and information retrieval, allowing employees to focus on more strategic, value-added activities.
Moreover, AI agents continuously learn from user interactions and feedback. This ongoing learning process allows them to improve their capabilities and improve performance. As they accumulate more data and insights, AI agents become increasingly proficient at anticipating future knowledge needs and trends, helping organizations stay ahead of the curve.
With AI agents leading the way, organizations can maximize the value of their knowledge assets, enhance efficiency, and enable teams to make more informed, data-driven decisions. These agents shift the traditional knowledge management paradigm, transforming knowledge management from a static repository into a dynamic, integral component of business strategy. By harnessing the power of AI agents, companies can enhance their agility, foster innovation, and maintain competition in the rapidly evolving business environment.
This article explores AI knowledge management agents, their key capabilities, use cases and benefits in more detail.
- Understanding AI agents and their types
- What are AI agents in knowledge management?
- How do AI agents address common problems faced in knowledge management?
- Key components of AI knowledge management agent
- How ZBrain’s generative AI agents are transforming enterprise operations
- Key capabilities of AI knowledge management agent
- Use cases and applications of AI agents in knowledge management
- Building LLM-based AI agents for knowledge management: A step-by-step guide
- Key benefits of AI agents in knowledge management
- How can LeewayHertz help you build AI agents for knowledge management?
Understanding AI agents and their types
An AI agent is a highly efficient virtual assistant; it autonomously performs tasks by sensing its environment, interpreting data, making informed decisions, and executing actions to achieve predefined objectives.
A key feature of AI agents is their capacity to adapt and improve continuously. Using technologies like Large Language Models (LLMs), these agents enhance their skills through ongoing interactions, becoming more advanced and effective.
Collaboration plays a crucial role in autonomous AI agents. Multiple agents work together, each with specialized roles, contributing to more effective problem-solving by leveraging their unique expertise toward shared goals.
Core functions of AI agents encompass various tasks, from simple to complex processes:
- Environmental perception: AI agents continuously monitor their operational environment, detecting and analyzing real-time changes. Leveraging large language models (LLMs), they interpret instructions and context autonomously or semi-autonomously based on human prompts.
- Tool utilization: AI agents use various tools, such as calculators, APIs, and search engines, to gather critical information for decision-making and task execution.
- Decision-making: AI agents make informed decisions based on data-driven insights by leveraging LLMs’ deep language understanding. They interpret complex instructions and contextual cues, ensuring actions align with strategic business objectives.
- Adaptive learning: AI agents employ advanced reasoning techniques, including chain-of-thought and tree-of-thought reasoning, facilitated by LLMs, to learn from outcomes and refine strategies. These methods enable agents to draw logical connections and develop solutions to complex problems, continually enhancing efficiency and effectiveness.
- Problem resolution: AI agents identify solutions and preempt issues before escalation. They leverage LLMs to manipulate text and generate specific outputs, such as reports and emails, enhancing their problem-solving capabilities.
- Strategic planning: AI agents contribute significantly to long-term business planning and resource allocation through their forecasting and planning abilities, supported by their deep understanding and manipulation of textual data provided by LLMs.
Optimize Your Operations With AI Agents
Optimize your workflows with ZBrain AI agents that automate tasks and empower smarter, data-driven decisions.
What are AI agents in knowledge management?
AI agents in knowledge management are pivotal tools designed to transform how organizations handle and utilize information and expertise. These agents harness advanced artificial intelligence techniques to automate tasks, facilitate knowledge discovery, and optimize decision-making processes across various domains.
Unlike basic information retrieval systems, AI agents in knowledge management are adept at understanding and analyzing complex data structures and unstructured information. They employ natural language processing (NLP), machine learning, and semantic analysis to extract meaningful insights, categorize knowledge, and recommend relevant resources to users.
AI knowledge management agents play several critical roles like:
- Information discovery and retrieval: AI agents efficiently search and retrieve relevant information from extensive knowledge repositories, databases, and collaborative platforms. They use sophisticated algorithms to understand user queries, suggest relevant documents, and facilitate knowledge sharing.
- Content curation and organization: AI agents curate and organize content by tagging documents, classifying information based on topics or themes, and creating summaries or abstracts. This helps streamline access to critical knowledge assets and enhances organizational content management.
- Knowledge generation and collaboration: AI agents contribute to knowledge creation by generating insights from data, identifying patterns, and synthesizing complex information into actionable knowledge. They also facilitate collaboration by connecting individuals with expertise and fostering discussions around specific topics or projects.
- Decision support and analytics: Leveraging machine learning and predictive analytics, AI agents provide decision support by analyzing trends, predicting outcomes, and offering recommendations based on data-driven insights. This assists decision-makers in making informed choices that align with organizational goals.
- Continuous learning and improvement: AI agents continuously learn from user interactions, feedback, and new data inputs, enhancing their ability to personalize recommendations, refine search results, and adapt to evolving knowledge needs within the organization.
- Automation of knowledge processes: These agents automate routine knowledge management tasks such as content tagging, document summarization, and knowledge base maintenance. This frees up human resources for more strategic activities and accelerates operational efficiency.
AI agents in knowledge management empower organizations to leverage their data more effectively, improve decision-making processes, foster innovation, and gain competitive advantage in a rapidly evolving business landscape. They represent a transformative approach to managing and harnessing organizational knowledge for sustainable growth and success.
How do AI agents address common problems faced in knowledge management?
Here’s how AI agents can address specific challenges in knowledge management:
Manual knowledge extraction is time-consuming and error-prone
Problem: Manual information extraction from large datasets and unstructured sources is slow and prone to human errors, leading to inefficiencies in knowledge base creation.
Solution: Automated knowledge extraction
AI agents with natural language processing (NLP) and machine learning algorithms can automate the knowledge extraction process. They swiftly parse through data, categorize information accurately, and enrich knowledge bases efficiently, reducing manual effort and improving data accuracy.
Difficulty in finding relevant information
Problem: Users struggle to locate specific information within extensive knowledge repositories, impacting productivity and decision-making.
Solution: Intelligent search and retrieval
AI agents enhance information retrieval by understanding user intent and context. These algorithms improve search accuracy and relevance through continuous learning from user interactions, ensuring quick access to pertinent information and boosting user satisfaction.
Maintaining content relevance
Problem: Ensuring that content within knowledge management systems remains current and relevant is challenging and requires manual updates.
Solution: Automated content curation
AI agents autonomously curate and prioritize content based on relevance, popularity, and user feedback. By analyzing trends and user behavior, AI agents ensure the knowledge base is regularly updated with accurate and valuable information, enhancing its usefulness over time.
Ensuring data quality and integrity
Problem: Maintaining data quality and integrity across diverse sources within knowledge management systems is critical but difficult to manage manually.
Solution: Data quality assurance
AI agents implement automated checks and validations to monitor data quality. They detect inconsistencies, errors, and outdated information, ensuring the knowledge base remains reliable. By proactively managing data integrity, AI agents support informed decision-making and enhance operational efficiency.
Reactive approach to knowledge management
Problem: Traditional knowledge management approaches often react to user needs rather than proactively anticipating them, leading to inefficiencies and missed opportunities.
Solution: Continuous learning and improvement
AI agents continuously analyze user interactions and feedback to improve knowledge management strategies. By identifying patterns and trends, AI agents enable proactive content updates, personalized recommendations, and predictive insights, enhancing overall responsiveness and effectiveness in meeting organizational goals.
Key components of AI knowledge management agent
The architecture of AI agents for knowledge management comprises several pivotal components, facilitating the processing of input data, reasoning, action planning, and execution based on contextual requirements.
Input: In knowledge management, the input component captures and processes diverse inputs from users and other agents, primarily in auditory, textual, and visual formats. These inputs guide the agent’s actions and decisions.
Brain: The brain manages reasoning, planning, and decision-making through four modules:
- Profiling: Defines the agent’s role and purpose for specific tasks.
- Memory: Stores past interactions to learn and improve over time.
- Knowledge: Contains domain-specific information to aid in planning and action.
- Planning: Determines appropriate actions based on the task and context.
Action: The action component executes planned actions by breaking down complex tasks into manageable steps and using the right tools at the right times, such as retrieving information, summarizing documents, or connecting experts.
By integrating these components, AI agents for knowledge management can effectively capture, process, and disseminate knowledge, enhancing organizational efficiency and fostering a culture of continuous learning and improvement.
How ZBrain’s generative AI agents are transforming enterprise operations
ZBrain AI agents transform knowledge management by introducing intelligent automation and actionable insights. Powered by large language models (LLMs), these agents go beyond conventional AI, delivering creative, strategic, and operational enhancements. Seamlessly integrating into diverse knowledge ecosystems, they optimize data access, streamline workflows, and foster innovation in managing organizational knowledge.
ZBrain AI agents: Key features and benefits:
- Seamless system integration
ZBrain AI agents integrate effortlessly with knowledge repositories, collaboration tools, and content management systems, ensuring smooth workflows across your existing platforms. - Continuous learning
With a built-in human feedback loop, these agents continuously refine their ability to retrieve, organize, and present information, ensuring accuracy and relevance over time. - Proprietary data integration
By leveraging your organization’s unique data, ZBrain AI agents provide tailored insights and recommendations, aligning with your specific business needs for informed decision-making. - Low-code orchestration with flow
Flow enables the creation of complex workflows for tasks like document categorization, information retrieval, and content dissemination, making it easy to design multi-step knowledge management processes. - End-to-end automation
From organizing unstructured data to delivering contextual insights, ZBrain AI agents autonomously manage the entire knowledge lifecycle, freeing teams to focus on strategic innovation. - Cloud and model agnostic
Designed for flexibility, these agents operate seamlessly on AWS, Azure, Google Cloud, or private cloud platforms, ensuring compatibility with your infrastructure for scalable knowledge management.
Key capabilities of AI knowledge management agent
AI agents designed for knowledge management possess several key capabilities that distinguish them in effectively handling organizational data and information. Here are the capabilities an AI agent in knowledge management typically possesses:
- Natural Language Processing (NLP): Ability to understand and process human language, enabling the AI knowledge management agent to comprehend queries, extract information from text, and generate human-like responses.
- Information retrieval: Proficiency in retrieving relevant information from structured and unstructured data sources based on user requests or predefined tasks.
- Content generation: Capability to create new content such as summaries, reports, or responses based on analyzed data and user needs.
- Knowledge discovery: Utilization of machine learning techniques to discover patterns, trends, and insights within data that contribute to organizational knowledge.
- Task automation: Ability to automate tasks such as data entry, document categorization, and information retrieval processes.
- Decision support: Providing insights and recommendations to support decision-making processes by synthesizing and analyzing data from various sources.
- Continuous learning: Capacity to learn from interactions, user feedback, and new data inputs to improve performance and relevance over time.
- Multimodal capabilities: Capability to process and understand multiple forms of data, including text, images, and audio, depending on the agent’s objectives.
- Integration and scalability: Seamless integration with existing systems and scalability to handle increasing volumes of data and diverse tasks within the organization.
- Personalization: Tailoring interactions and content recommendations based on user profiles, preferences, and historical interactions to enhance user experience and efficiency.
These capabilities enable AI agents in knowledge management to streamline information processes and enhance decision-making, collaboration, and innovation within organizations by leveraging advanced artificial intelligence techniques.
Optimize Your Operations With AI Agents
Optimize your workflows with ZBrain AI agents that automate tasks and empower smarter, data-driven decisions.
Use cases and applications of AI agents in knowledge management
AI agents are pivotal in transforming knowledge management by automating and streamlining key processes. Below are some use cases and how ZBrain supports them:
Use case | Description | How ZBrain helps |
Proactive knowledge discovery | Analyzes patterns in unstructured datasets to update knowledge bases with new and relevant information. | ZBrain can continuously discover new insights, ensuring knowledge bases are relevant. |
Content organization and categorization | Classifies and tags content, improving document accessibility by organizing it into structured categories. | ZBrain can categorize and tag content efficiently, making it easier for users to find relevant information quickly. |
Automated knowledge extraction | Extracts key insights from unstructured data sources, converting them into structured formats for better decision-making. | ZBrain extracts valuable information from documents, emails, and transcripts, providing essential insights for analysis. |
Intelligent search and retrieval | Processes natural language queries to understand user intent and provides relevant results. | ZBrain can refine search results using NLP, improving query accuracy and recommending related content. |
Personalized knowledge recommendations | Offers personalized content suggestions based on roles, interests, and past behavior, enhancing productivity and engagement. | ZBrain delivers personalized recommendations, ensuring users receive timely and relevant information. |
Knowledge gap analysis and training | Analyzes performance and feedback to identify knowledge gaps, recommending tailored training programs. | ZBrain identifies knowledge gaps and suggests personalized development programs to support continuous learning. |
Automated content creation and updates | Generates and updates content, such as reports and summaries, by aggregating information from various sources. | ZBrain can automate content creation and updates, ensuring knowledge bases stay accurate and current. |
Collaboration and knowledge sharing | Facilitates collaboration by suggesting relevant resources and managing knowledge-sharing platforms. | ZBrain enables seamless collaboration by providing suggestions and managing platforms to share valuable insights. |
Compliance and governance | Enforces compliance policies and manages access to sensitive information, ensuring data security and providing audit trails. | ZBrain monitors compliance, tracks knowledge access, and securely handles sensitive information. |
Performance monitoring and reporting | Tracks knowledge management initiatives’ impact, providing insights on utilization, engagement, and ROI. | ZBrain monitors knowledge utilization and generates reports to optimize knowledge management strategies. |
By leveraging AI agents, organizations can create more efficient, responsive, intelligent knowledge management systems that drive innovation and competitive advantage.
Optimize Your Operations With AI Agents
Optimize your workflows with ZBrain AI agents that automate tasks and empower smarter, data-driven decisions.
Building LLM-based AI agents for knowledge management: A step-by-step guide
Large language models (LLMs) are revolutionizing knowledge management practices. With LLM-powered AI agents, professionals can streamline tasks, discover hidden insights, and enhance the speed of information retrieval and decision-making. This section provides a detailed walkthrough of building your LLM-powered knowledge management agent.
Define the knowledge management scope and objectives
Clearly outline the specific domain you want to target (e.g., corporate knowledge, intellectual property, compliance). Identify the key challenges you want the AI agent to address.
- Task-oriented approach: Define the specific knowledge management tasks the AI agent should perform. This might include:
- Information retrieval: Identifying and summarizing relevant documents, articles, and data sources.
- Content categorization: Organizing information into relevant categories and tagging content for easier access.
- Knowledge extraction: Extracting key insights and data points from unstructured text.
- Document drafting: Assisting in drafting reports, summaries, and other documents based on gathered knowledge.
Select an appropriate LLM
Select a base LLM that aligns with your knowledge management needs. Here are some powerful options often favored for such purposes:
- OpenAI’s GPT (GPT-3.5, GPT-4): Renowned for impressive text generation, summarization, translation, and creative writing capabilities. GPT-4, in particular, excels at complex reasoning and understanding context. Access is typically through an API.
- Google’s PaLM 2 (Pathway Language Model 2): This model performs well in reasoning, coding, and multilingual tasks. It’s considered highly versatile for knowledge management. Access is usually via Google’s AI platform or specific services like Vertex AI.
- Meta’s LLaMA (Large Language Model Meta AI): Available in different sizes, making it adaptable to various needs and computational constraints. Known for its performance in text generation and comprehension tasks. Access is often granted through research partnerships or specific releases.
- BLOOM (BigScience Large Open-science Open-access Multilingual Language Model): A collaborative effort designed for open and accessible research. It’s a powerful option for multilingual tasks and has shown potential across various domains. Often accessible for direct download and use.
- Hugging face transformers: This isn’t a single LLM but rather a library (and a community) providing access to a vast collection of pre-trained LLMs, including many mentioned above. This platform makes experimenting with and comparing different LLMs for your needs easier.
Factors to consider:
- Model size: Larger models are generally more capable but require more computational resources.
- Performance: Evaluate the model’s accuracy and efficiency on tasks similar to your knowledge management needs.
- Licensing: Based on your budget and usage requirements, consider open-source options (e.g., BERT) or commercial APIs (e.g., OpenAI’s GPT-3 API).
Data collection and preparation: Fueling the AI engine
Gather relevant and high-quality datasets specific to your knowledge management domain. This might include:
- Knowledge databases: Explore resources like internal databases, industry-specific repositories, and specialized knowledge bases.
- Research papers: Access academic journals, conference proceedings, and whitepapers.
- Industry reports: Utilize market research reports, trend analyses, and case studies.
- Textbooks and articles: Use academic and industry publications to gather comprehensive information.
Data preprocessing: Clean and preprocess the data to ensure it’s in a format the LLM can understand. This involves:
- Cleaning: Removing irrelevant information, correcting errors, and handling missing data.
- Formatting: Consistently structuring the data (e.g., using JSON, CSV) and ensuring consistent text formatting, numbers, and dates.
Train the LLM (for the specific domain/task)
Domain adaptation: This crucial step involves training the pre-trained LLM on your domain-specific datasets. This process tailors the LLM’s knowledge and capabilities to perform exceptionally well on tasks related to your knowledge management needs.
- Prompt engineering: While training, experiment with different ways of phrasing prompts or questions to elicit the most accurate and relevant responses from the LLM. This step helps the LLM understand how to interpret and respond to your inquiries.
Develop the AI agent architecture: Building the brain and body
Design the AI agent as a system with distinct modules, each responsible for a specific function:
- Input processing: Handles user queries and commands.
- LLM interaction: Interacts with the trained LLM to generate responses and insights.
- Output generation: Presents the LLM’s output in a clear and understandable format.
- Memory and context: Incorporate mechanisms for the agent to remember previous interactions and maintain context during multi-turn conversations.
Implement Natural Language Understanding (NLU): Teaching the agent to understand
Develop NLU modules to interpret queries and commands accurately.
- Intent recognition: Train the agent to understand the user’s intent (e.g., finding specific information, summarizing a topic, comparing documents).
- Entity extraction: Enable the agent to identify and extract key entities (e.g., people, organizations, key terms) from text.
Create knowledge integration systems: Connecting to external knowledge
Integrate external knowledge bases and databases to provide the AI agent with a wider range of information to draw upon.
- Fact-checking: Implement mechanisms to verify information against trusted sources and flag potential inaccuracies or inconsistencies.
- Continuous learning: Design systems for the AI agent to continuously learn and update its knowledge base with new findings and data.
Develop reasoning and analysis capabilities: Going beyond information retrieval
Data analysis: Implement algorithms for data analysis, including statistical analysis, pattern recognition, and trend identification.
Hypothesis generation: Develop modules that can generate hypotheses or questions by analyzing data.
Logical reasoning: Enable the agent to perform logical reasoning and inference, concluding available evidence.
Design output generation and summarization: Presenting findings clearly
Natural Language Generation (NLG): Develop NLG capabilities for the agent to generate coherent and human-readable responses, summaries, and reports.
Summarization: Implement techniques for summarizing large volumes of information into concise and informative overviews.
Visualization: Create modules that can generate charts, graphs, and other visualizations to present data and findings in an easily understandable format.
Implement ethical and bias mitigation measures: Ensuring responsible AI
Bias detection: Develop systems to detect and mitigate potential biases in data, algorithms, and outputs.
Transparency: Implement measures to explain the AI agent’s decision-making process, making its reasoning transparent to users.
Ethical guidelines: Ensure compliance with relevant ethical guidelines and data protection regulations.
Create user interface and interaction design: Making the agent user-friendly
Intuitive interface: Develop an intuitive interface that allows users to interact with the AI agent easily and naturally.
Query refinement: Implement features for query refinement, allowing users to refine their questions and receive more precise results iteratively.
Collaborative research: Design systems for collaborative work, enabling AI agents and human professionals to work together seamlessly.
Testing and validation: Ensuring accuracy and reliability
Rigorous testing: Conduct thorough testing of the AI agent’s capabilities across various tasks and scenarios.
Validation studies: Compare the AI agent’s outputs to expert analysis to validate its accuracy and reliability.
Ongoing monitoring: Implement ongoing monitoring and quality control measures to ensure the agent’s performance remains consistent.
Deployment and scaling: Making the agent accessible
Infrastructure: Set up the necessary infrastructure to deploy the AI agent, considering factors like computational resources, storage capacity, and security.
Data security: Implement strong security protocols to safeguard sensitive data.
Scalability: Develop strategies to scale the AI agent’s capabilities to handle increasing demands.
Continuous improvement and updating: An ongoing journey
Feedback loops: Establish feedback loops to gather user input and continuously improve the AI agent’s performance.
Regular updates: Regularly update the agent’s knowledge base with the latest findings, data, and methodologies.
Version control: Implement version control and change management processes to track updates and ensure stability.
Documentation and training: Empowering users
Comprehensive documentation: Create clear and comprehensive documentation to guide users on how to use the AI agent effectively.
Training programs: Develop training programs to help users understand the AI agent’s capabilities, limitations, and ethical considerations.
Best practices: Establish best practices for AI-assisted work within your specific domain.
Platforms for building AI agents
AutoGen: A framework specifically designed for building conversational AI agents using LLMs. It simplifies creating agents to engage in multi-turn conversations, access tools, and perform complex tasks.
CrewAI: A no-code platform for building and deploying AI agents, including those powered by LLMs. It offers a user-friendly interface for defining agent workflows, integrating data sources, and managing agent interactions.
Key considerations
Human-AI collaboration: Remember that AI agents are intended to enhance human intelligence, not substitute it. Foster a collaborative environment where AI agents and human professionals work together to achieve common goals.
Ethical implications: Consider the ethical implications of using AI in knowledge management, ensuring that your AI agent is designed and utilized in a responsible, transparent manner that serves society’s broader interests.
Building LLM-powered AI agents for knowledge management is an iterative, continuous learning and improvement journey. By following this guide, you can create a powerful agent that accelerates discovery, deepens understanding, and helps solve complex problems across diverse fields.
Key benefits of AI agents in knowledge management
The key benefits of AI agents in knowledge management include:
- Efficiency: AI agents automate routine tasks such as data categorization, retrieval, and analysis, significantly reducing the time and effort required compared to manual methods.
- Continuous learning: Through machine learning algorithms, AI agents continuously learn from new data and interactions, improving their ability to provide relevant and up-to-date information.
- Proactive insights: AI agents can proactively discover patterns and trends in large datasets, uncovering insights that may not be immediately apparent through traditional methods.
- Enhanced decision-making: By synthesizing and presenting complex data in a digestible format, AI agents empower decision-makers to quickly make informed and data-driven decisions.
- Personalization: AI agents can personalize interactions by tailoring information based on user preferences and historical interactions, improving user satisfaction and efficiency.
- Risk mitigation: AI agents can identify potential data anomalies, helping organizations mitigate risks proactively and maintain data integrity.
- Cost-effectiveness: By automating tasks and improving efficiency, AI agents reduce operational costs associated with managing and accessing organizational knowledge.
- Breakdown language barriers: AI agents can process and understand multiple languages, facilitating seamless communication and knowledge sharing across global teams. This capability ensures that information is accessible and actionable for users regardless of their native language, enhancing collaboration and expanding organizational reach in diverse markets.
AI agents in knowledge management transform how organizations utilize and benefit from their vast repositories of information, leading to improved operational efficiency, better decision-making, and competitive advantages in rapidly evolving markets.
How can LeewayHertz help you build AI agents for knowledge management?
As a leader in AI development, LeewayHertz is positioned to empower organizations across various sectors, including knowledge management, through advanced AI solutions. Here’s how LeewayHertz can assist your organization in leveraging AI agents effectively for knowledge management:
- Strategic consultation: LeewayHertz offers strategic consultation to help organizations understand how AI agents can transform knowledge management. Our experts collaborate with you to identify key areas where AI can enhance information retrieval, content organization, collaboration, and decision support. We develop tailored strategies that align with your organizational objectives and drive digital transformation in knowledge management.
- Custom AI agent development: Specializing in developing custom AI agents, LeewayHertz creates solutions tailored to the specific needs of knowledge management. Using advanced platforms like AutoGen Studio and CrewAI, we prototype and deploy AI agents capable of handling diverse tasks such as information discovery, content curation, and knowledge retrieval. These agents are designed to optimize workflows and improve the efficiency of knowledge-intensive processes.
- Seamless integration: Our team ensures seamless integration of AI agents into your existing knowledge management systems. Leveraging AutoGen Studio and CrewAI, we integrate these intelligent agents to work with your IT infrastructure. This integration enhances data interoperability, streamlines operations, and boosts productivity without disrupting ongoing workflows.
- Driving innovation in knowledge management: AI agents developed by LeewayHertz offer organizations a competitive edge in a landscape where efficient information handling and decision-making are crucial. Our solutions enable enhanced information discovery, more effective collaboration, and data-driven insights that empower organizations to make informed decisions faster and more accurately.
Partnering with LeewayHertz empowers organizations to harness the full potential of AI agents in knowledge management, driving efficiency, innovation, and strategic growth. Our commitment to delivering cutting-edge AI solutions ensures that your organization remains adaptive and responsive to the evolving demands of knowledge-intensive environments.
Endnote
AI agents represent a transformative leap forward in knowledge management, offering unprecedented capabilities that address the limitations of traditional systems. By leveraging advanced language models and machine learning algorithms, AI agents seamlessly integrate, organize, and retrieve vast amounts of information, ensuring that relevant knowledge is readily accessible when needed. They enhance efficiency by automating routine tasks, providing personalized insights to users, and fostering a collaborative and innovative work environment.
Moreover, AI agents’ ability to continuously learn from interactions and feedback means they constantly evolve and improve, making them invaluable assets in a dynamic business landscape. Their advanced reasoning, decision-making, and planning capabilities enable organizations to make smarter, data-driven decisions, ultimately driving business success.
As organizations navigate an increasingly complex and data-rich world, adopting AI agents in knowledge management will be key to unlocking their full potential, maintaining competition, and fostering a culture of continuous improvement and innovation.
Transform your knowledge management with advanced AI agents today! Explore how LeewayHertz’s AI agent development services streamline operations, enhance collaboration, and optimize efficiency across your organization.
Start a conversation by filling the form
All information will be kept confidential.
FAQs
What are AI agents in knowledge management?
An AI agent in knowledge management is a system or program designed to autonomously perform tasks such as capturing, organizing, sharing, and analyzing knowledge. By creating workflows, AI agents streamline processes, improve accessibility to information, and enhance decision-making capabilities within an organization. These AI agents are instrumental in managing both structured and unstructured data, ensuring that knowledge is readily available and up-to-date.
How do AI agents improve knowledge management systems?
AI agents enhance knowledge management by automating content curation, enabling intelligent search and retrieval, providing personalized recommendations, and ensuring information is up-to-date. This leads to increased efficiency and better utilization of organizational knowledge.
What are some specific tasks AI agents can perform in knowledge management?
Specific tasks performed by AI agents include:
- Answering questions: AI agents can provide quick and accurate answers to user queries, pulling information from various sources within the knowledge base.
- Summarizing documents: They can condense lengthy articles or reports into concise summaries, saving users time.
- Creating new content: Based on existing knowledge, AI agents can generate drafts of documents, presentations, or reports.
- Connecting experts: They can identify individuals with specific expertise within an organization based on their contributions and interactions within the knowledge base.
- Identifying knowledge gaps: By analyzing existing content, AI agents can pinpoint areas where knowledge is missing or incomplete.
- Personalizing knowledge delivery: AI agents can tailor information delivery based on individual user profiles, roles, and past interactions.
- Automating knowledge updates: AI agents can monitor data sources and automatically update the knowledge base with new information.
What are the benefits of using AI agents for knowledge management?
- Improved efficiency: Automation reduces manual effort and speeds up knowledge-related tasks.
- Enhanced accuracy: AI reduces human error in information retrieval and analysis.
- Better decision-making: Access to relevant and timely knowledge supports informed decisions.
- Increased collaboration: Agents facilitate knowledge sharing and connection between individuals.
- Scalability: AI agents can handle large and growing knowledge bases efficiently.
What types of organizations can benefit from AI agents for knowledge management?
Any organization that relies heavily on knowledge can benefit, including corporations, research institutions, government agencies, and educational institutions.
Can AI agents assist in content creation within knowledge bases?
Yes, AI agents can automate content generation by creating new knowledge base articles, FAQs, and other resources based on existing content. This ensures the knowledge base remains current and comprehensive.
What is ZBrain?
ZBrain is a full-stack, enterprise-grade generative AI orchestration platform that helps organizations create custom generative AI applications and AI agents. It enables businesses to optimize processes like capturing, organizing, and sharing knowledge through its advanced capabilities. By seamlessly integrating with existing systems, offering an intuitive interface, and providing access to diverse AI models, ZBrain empowers companies to enhance operational efficiency, improve decision-making, and deliver superior customer experiences in knowledge management.
How can ZBrain’s AI agents benefit knowledge management?
- Seamless integration with knowledge systems: ZBrain AI agents integrate effortlessly into existing knowledge management platforms, such as document repositories, collaboration tools, and enterprise resource planning systems, ensuring a unified knowledge workflow.
- Intelligent information retrieval: By leveraging advanced AI models, ZBrain AI agents can quickly and accurately retrieve relevant information from vast data repositories, improving knowledge accessibility for users.
- Continuous learning: Through a human feedback loop, these AI agents continuously improve their understanding and categorization of knowledge, ensuring the delivery of accurate and up-to-date information.
- Low-code workflow automation: ZBrain’s Flow feature enables businesses to create customized workflows for knowledge tasks, such as content curation, tagging, or archiving, without extensive coding knowledge.
- End-to-end automation: From capturing and organizing information to sharing and analyzing it, ZBrain AI agents autonomously manage the entire knowledge management lifecycle, freeing up teams to focus on innovation and strategy.
- Cloud and model agnostic: The platform’s flexibility allows AI agents to operate across any cloud environment or with various AI models, ensuring compatibility with existing infrastructure and scalability for future needs.
How can an organization partner with LeewayHertz to implement AI agents for knowledge management?
To explore how LeewayHertz can assist in implementing AI agents for knowledge management, contact us at info@leewayhertz.com. Our team will work closely with you to understand your organization’s unique knowledge management requirements and demonstrate how AI agents can optimize your processes. Please provide details such as your organization’s name, industry, and specific knowledge management goals to help us tailor a solution that aligns with your needs. We look forward to collaborating to enhance your knowledge management capabilities efficiently and effectively.
How does LeewayHertz ensure data security when deploying AI agents for knowledge management?
LeewayHertz prioritizes data security by implementing robust measures throughout the AI agent deployment process. These measures include data encryption, secure API integrations, and compliance with regulations to protect sensitive knowledge management data. Our AI agents are designed to operate within secure environments and incorporate Single Sign-On (SSO) for streamlined and secure user authentication. Additionally, role-based permissions are employed to ensure controlled access, granting users only the data and features relevant to their roles. Regular security audits and customizable access controls further safeguard your organization’s proprietary information, ensuring that your data remains secure and confidential at all times.
Insights
AI-driven development: Tools, technologies, advantages and implementation
AI-driven development seamlessly integrates artificial intelligence, particularly through ML algorithms and NLP, to comprehend, assist, and generate code, thereby streamlining a developer’s tasks and fostering the creation of superior-quality software.
Enterprise AI solutions for logistics: Enhancing operational excellence
Building an enterprise AI solution in logistics involves leveraging advanced technologies to automate processes, gain insights, and make data-driven decisions within logistics operations.
AI use cases in the automotive industry
AI in the automotive industry has redefined vehicle technology and driving experiences. Through advanced ML and data analytics, AI enables autonomous driving, enhancing safety and efficiency.